- 1School of Management and Economics, North China University of Water Resources and Electric Power, Zhengzhou, China
- 2Institute of Industrial Economics, Jiangxi University of Finance and Economics, Nanchang, China
The technological source of green innovation has been a persistent theme in environmental economics. In more recent years, digital technology has triggered a new round of social changes and is viewed as the emerging growth engine, leading to significant improvements in productivity and innovation. However, whether digital technology can promote green innovation remains an unknown issue. This study elucidates the causal relationship between digital transformation and green enterprise innovation for Chinese companies during 2009–2019. The findings indicate that digital transformation positively affects the quantity and quality of green innovation performance. Each standard deviation increase in the degree of digital transformation increases the quantity and quality of green enterprise innovation by 2.924% and 2.124%, respectively. Additionally, digital transformation drives green innovation by alleviating financing constraints and information asymmetries and improving human capital. This effect is more pronounced among enterprises in regions with high levels of environmental investment, clean industries, and stringent environmental regulations. By highlighting the linkage between firm-level digital transformation and green innovation, this study contributes to our understanding of the positive environmental externality associated with the diffusion of digital technology and offers valuable insights for the sustainable development of emerging economies.
1 Introduction
Owing to the increasingly severe natural resource constraints and continuous changes in climate risks, countries around the world are seeking circular and low-carbon sustainable development paths, actively addressing environmental challenges while opening up the commanding heights of future economic competition. Green innovation is expected to coordinate environmental protection and economic growth and is key to achieving sustainable development goals (Zhang et al., 2022a). Over the past decade, various determinants of green innovation have been explored, but the main focus has been on environmental regulation (Guo et al., 2018; Fan et al., 2021; Peng and Tao, 2022). However, the effective use of such tools relies on administrative mandatory intervention, which has high regulatory costs and is prone to crowding out high-quality green innovation with high investment and risk, resulting in a “binary paradox” of pursuing quantity and abandoning quality (Guo et al., 2018). With the wave of digital development sweeping the world, the continuous penetration of the new generation of digital technology has promoted enterprises to achieve fundamental changes in production methods, management processes, and organizational structure. Digital transformation has become an important direction for future reform and transformation of enterprises (Wen et al., 2022). Digital transformation is a transformation facilitated by advancements in information technology. It involves introducing digital technology into enterprises, utilizing relevant technologies to process information and data within organizations, and assisting in decision-making (Wu et al., 2019; Tucker and Duval, 2022). Digital transformation has inherent advantages in technology research and development, information sharing, and reducing transaction costs, which is conducive to knowledge diffusion and technology spillover. There is a significant innovation-driving effect (Ferreira et al., 2019), which can provide technical support and new opportunities for green innovation. The 2021 study by the International Energy Agency (IEA, 2021) pointed out that demand-driven solutions based on digital technology will provide significant benefits for reducing costs, increasing efficiency, and reducing carbon emissions in energy use. Some studies have preliminarily explored the relationship between digitalization and green innovation, but no consensus has yet been reached. Based on data from the Middle East and North African region and Gulf-Cooperation countries, research shows that digital technology can exert a positive green innovation effect by creating an R&D environment with high security, low latency, and low energy consumption (El-Kassar and Singh, 2017). However, based on data from 239 managers in the United States, Ghasemaghaei and Calic (2020) suggested that the development of digital technology not only does not contribute to the improvement of green innovation levels but may also have negative impacts. Li et al. (2021) also indicated that digital transformation increases resource extraction and energy consumption, which may lead to a decrease in the green innovation activities of enterprises. It can be seen that further research is needed on how digital transformation affects green innovation. Therefore, our study focuses on digital transformation and green innovation and aims to answer the following three related and progressive questions: 1) can digital transformation effectively promote green innovation in enterprises? It includes the improvement of the quantity and quality of innovation. 2) If there is a promotion effect, how does digital transformation affect green innovation? 3) Is the impact of digital transformation on green innovation the same across different enterprises, industries, and regional characteristics?
A sample of A-share listed enterprises in China’s Shanghai and Shenzhen stock markets was used from 2009 to 2019. The reasons for choosing China as a case study are threefold. First, as the world’s largest emerging economy and one of the world’s largest carbon emitters, China faces enormous environmental challenges (Hu et al., 2022), and improving its green innovation level is crucial for the sustainable development of the global economy. Second, the National School of Development of Peking University and Zhaopin Recruitment (National School of Development of Peking University and Zhaopin Recruitment, 2021) pointed out that, during the study period, China’s digital economy and technology developed rapidly, and more than 80% of Chinese enterprises joined the digital transformation. Therefore, China has abundant sample data for us to quantify digital transformation and make a more detailed investigation. Third, due to China’s massive scale and diversity, we can examine the heterogeneous impact of digital transformation on green innovation from different perspectives. Hence, we can more objectively and comprehensively understand the relationship between digital transformation and green innovation.
We build a comprehensive indicator of digital transformation. Moreover, we distinguish between the quantity and quality of green innovation using green patent data from listed companies. Our research results show that, first, digital transformation can significantly improve the quantity and quality of green innovation. Second, consistent with intuition, digital transformation will bring enterprises lower financing constraints and information asymmetry and higher levels of human capital, thus bringing better green innovation performance. Finally, the promotion effect of digital transformation on green innovation is more significant in enterprises with high levels of environmental protection investment, clean industries, and regions with stronger environmental regulation.
This study has the following contributions. First, the existing literature focusing on the relationship between green innovation and digital transformation is not only controversial but also mostly studies from the perspective of single-digital technologies such as big data and artificial intelligence (El Kassar and Singh, 2019; Ghasemaghaei and Calic, 2020; Mubarak et al., 2021). This study uses a novel and reliable approach that constructs a comprehensive indicator by textual analysis to reflect the general level of firm-level digital transformation, alleviating the problem of variable measurement error as far as possible. In addition, this study also utilizes PSM-DID and instrumental variable methods to address endogeneity issues. The above methodological improvements enable us to derive more robust and accurate empirical estimations, form a good response, and supplement previous research. Second, the potential mechanisms underlying the impact of digital transformation on green innovation have not been fully explored. The existing research is mostly based on the natural resource perspective, which means that digital transformation promotes green innovation by improving enterprise research and development levels and financial capabilities (Feng et al., 2022; He et al., 2023). However, according to the characteristics of green innovation, financing constraints, information asymmetry, and human capital are three very important influencing factors (Liu et al., 2022a; Munawar et al., 2022), but few studies have considered them, especially the human capital channel. Therefore, our research explores potential channels in these three dimensions and opens up the black box of their connections more comprehensively. This deepens our understanding of how digital transformation promotes green innovation within enterprises. Lastly, limited literature examines the differential effects of different environmental factors on the innovation effects of digital transformation, making previous conclusions potentially one-sided (Feng et al., 2022; He et al., 2023). This study incorporates the differences in environmental investment levels, industry pollution intensity, and local environmental regulation levels of enterprises into the research model and systematically evaluates the asymmetric impact of digital transformation on green innovation from both micro and macro levels, expanding the context analysis boundary of the existing literature.
The remainder of this study is categorized as follows: Section 2 discusses the literature review and research hypotheses. Section 3 outlines the data and methodology. Section 4 presents the empirical results and discussion. Section 5 discusses the main mechanism. Section 6 indicates heterogeneity testing. Lastly, Section 7 addresses the main findings and policy recommendations.
2 Literature review and hypothesis development
2.1 Digital transformation and green innovation
With the development of digital technology, scholars have gradually realized the importance of digitization for green innovation (El Kassar and Singh, 2019; Li et al., 2021). EL Kassar and Singh (2019) demonstrated that the application of big data and artificial intelligence technology is one of the important factors driving green innovation. Mubarak et al. (2021) collected data from 217 manufacturing companies in Malaysia and found that applying Industry 4.0 technology can motivate enterprises to carry out green innovation. Ghasemaghaei and Calic (2020) found that an increase in the “volume” of big data technology based on US corporate data may not contribute to the improvement of green innovation efficiency and may even have negative impacts. Li et al. (2021) also supported the adverse effects of digital technology on green innovation. In addition, there are also a few studies exploring the impact of digitalization-related policies on green innovation. Zhang et al. (2022b) used the quasi-natural integration of informatization and industrialization to provide Chinese evidence of digital transformation promoting green innovation. Unfortunately, this study did not examine the potential mechanism between the two. Although Feng et al. (2022) and He et al. (2023) preliminarily discussed the possible mechanisms between digitalization and green innovation, they mainly focused on the perspective of natural resources. It can be found that there are controversies in the literature on digitalization and green innovation, and discussions are mostly focused on digital aspects such as big data or artificial intelligence. The overall exploration of digital transformation in green innovation is relatively insufficient, and there is a lack of research on the possible channels of interaction between the two. In addition, the existing literature is often limited to exploring the quantity of green innovation, but the quality of innovation is the fundamental manifestation of an enterprise’s innovation ability. Therefore, this study conducts further research on digitalization and green innovation, while also considering the impact of digital transformation on the quality and quantity of green innovation.
The advantage of digital transformation is that it can improve the quantity and quality of green innovation in enterprises. First, digital technology emphasizes coordinating production and the environment (Levinson, 2009). It enables digital transformation to provide a strong internal driving force for the “increase in quality and quantity” of enterprises’ green innovation. Moreover, digital technology is a typical common technology (Basu and Fernand, 2007). Hence, digital enterprises can develop numerous new technologies based on existing technologies. It can reduce green R&D costs and green innovation risks, increase the probability of enterprises transforming knowledge into high-quality green innovation achievements, and thereby empower the development of the quantity and quality of green innovation in enterprises (Strambach, 2017). Second, enterprises using digital technology can optimize resource allocation and combine existing products to form new ones with competitive advantages (Yoo et al., 2012). Enterprises can not only integrate digital technology into their existing product production to promote the improvement of green innovation quality but also accelerate product iteration speed and increase the output of green innovation. Finally, digital transformation facilitates enterprises to use the Internet of things, big data, and other channels to obtain customers’ consumption habits and preferences, achieve an accurate understanding of market demand (Bajari et al., 2019), implement consumers’ demand preferences for green products, transform enterprises’ green innovation from experience-driven to data-driven, and lay an intellectual foundation for improving the quality of green innovation. In summary, we propose Hypothesis 1.
H1. Digital transformation can significantly improve the quantity and quality of enterprises’ green innovation.
2.2 Financing constraints, information asymmetry, and human capital
Although research on digitalization and green innovation has gradually enriched, as mentioned earlier, the potential mechanism of correlation between digital transformation and green innovation has not been fully explored (Mubarak et al., 2021; Zhang et al., 2022a; He et al., 2023). Based on the characteristics of green innovation, we attempt to analyze the potential mechanism of digital transformation to promote green innovation from three dimensions: financing constraints, information asymmetry, and human capital.
First, green innovation projects have higher risks and more significant funding requirements than traditional innovation. Therefore, financing difficulties often become a crucial constraint for enterprises implementing green innovation strategies (Liu et al., 2022b). Digital transformation meets the requirements of the national digital economy development strategy, so it is easier for enterprises to get preferential policies and financial subsidies from the government, thus improving the financing constraints of enterprises to promote green innovation. At the same time, enterprises engaged in digital transformation often signal bold change and active deployment to the outside world. Therefore, it is easy to expand the external funding channels required for green innovation in enterprises, providing financial support for the iterative upgrading of green innovation in enterprises (Wynarczyk et al., 2013). Additionally, digital transformation can help enterprises reduce costs and increase efficiency (Peng and Tao, 2022), enable them to obtain richer digital resource services at lower costs, maintain the stability of the innovation capital chain, and enable enterprises to promote green innovation better.
Second, green innovation requires integrating information from multiple links (such as energy consumption, product research and development, and product marketing), often resulting in serious information asymmetry issues, leading to low efficiency in enterprises’ green innovation (Liu et al., 2022a). Digital transformation has advantages in information exchange, which helps alleviate information asymmetries (Zhao et al., 2022). From an internal perspective within the enterprise, digital technology can transform massive unstructured and non-standard data into easily understandable visual data, thereby improving information transmission efficiency (Lin and Kunnathur, 2019). Enterprises can track various internal production links and processes, strengthen their environmental responsibility orientation, and improve environmental innovation strategic decision-making behavior, ultimately driving green technology innovation. From the external perspective of the enterprise, digital transformation can improve the authenticity and accuracy of the public’s access to enterprise environmental information, thus breaking down information barriers and reducing information asymmetry with external stakeholders (Goldstein and spatt, 2021). It is conducive to forming an effective external supervision mechanism, thereby imposing implicit constraints on the irrational behavior of management. This, in turn, reduces executive behaviors that are not conducive to green technology innovation and finally motivate enterprises to engage in green innovation activities.
Finally, human capital has the characteristics of increasing marginal benefits that physical capital lacks (Romer, 1990). It is a key input factor in improving enterprises’ green innovation performance (Munawar et al., 2022). On the one hand, the experience, knowledge, and technology contained in human capital can change enterprises’ existing knowledge foundation and bring new ideas to green innovation. On the other hand, enterprises’ environmental protection awareness is closely related to their human capital status (Chen et al., 2021). The higher the level of human capital in a company, the stronger its overall environmental awareness and the more inclined it is to increase its efforts in green innovation (Yong et al., 2019). Digital transformation has a significant promotional effect on the level of human capital (Smith et al., 2017). It will make the production mode of enterprises intelligent and automated. The widespread use of advanced machinery and equipment will increase the demand for high-tech talents, replace low-end labor forces, ultimately optimize the structure of human capital, and improve the level of human capital (Lordan and Neumark, 2018). With the integration of highly educated and high-quality human and knowledge capital into the entire process of green innovation research and development in enterprises, talents continue to gather in enterprises, forming a diffusion effect conducive to green innovation development.
Based on the above analysis, we propose Hypothesis 2.
H2. Digital transformation reduces financing constraints and information asymmetry and improves the level of human capital to promote enterprises’ green innovation.
Based on the above analysis, the theoretical analysis of the study is shown in Figure 1.
2.3 Heterogeneity of the impact of digital transformation on green innovation
Under varied external circumstances and enterprise attributes, digital transformation’s impact on green innovation may be asymmetric. Thus, we examine the heterogeneity of the role of digital transformation in green innovation from three perspectives: regional environmental protection intensity, industrial pollution attribute differences, and enterprise environmental investment level.
First, according to the theory of environmental regulation and technological innovation, the intensity of environmental protection by local governments is an essential factor influencing local enterprises’ green innovation (Xie et al., 2017). Government environmental regulations profoundly influence and motivate green innovation activities’ direction, focus, and scale. Enterprises in regions with stricter environmental regulations face greater environmental pressure and therefore have greater incentives to engage in green innovation activities. Therefore, the application of digital technology can have a greater impact on green innovation in enterprises. Enterprises in regions with lower environmental regulatory intensity face less environmental pressure and lack incentives for green innovation. The incentive effect of digitalization on green innovation in enterprises is also relatively weak.
Second, the green innovation activities of enterprises are closely related to whether the industry they operate in belongs to heavy polluting industries. Compared to non-heavily polluting enterprises, the difficulty and cost of technological upgrading and transformation for heavily polluting enterprises are high. Business activities in heavily polluting industries have environmental specificity. With the development of big data, the innovation attention of heavily polluting industry enterprises is shifting toward non-green innovation, which in turn hinders the improvement of green innovation quality (Du et al., 2021). Moreover, the business activities of heavy polluting industries themselves have environmental specificity, and digital transformation is mainly reflected in multiple stages such as information exchange, technological learning, and production and sales. It cannot fundamentally change the attributes of heavy polluting industries, so the incentive effect on the green innovation activities of such enterprises is not significant.
Finally, digital transformation can optimize the technological innovation resources of enterprises, thereby motivating them to engage in green innovation. For enterprises with different levels of environmental investment, there is heterogeneity in this incentive effect. If enterprises do not attach importance to environmental investment, even if digital transformation optimizes their technological innovation resources, they will not engage in green innovation. If enterprises attach importance to environmental investment, then digital transformation is more likely to promote the completion of green technology research, shorten the cycle of green innovation research, and accelerate the application of green innovation achievements. Therefore, our study believes that for enterprises with higher levels of environmental investment, digital transformation has a greater incentive effect on their green innovation.
In summary, this article proposes Hypothesis 3.
H3. The promotion effect of digital transformation on green innovation is more significant among enterprises in regions with high levels of environmental investment, clean industries, and stringent environmental regulations.
3 Data and methodology
3.1 Data
This study uses the green patent data and corresponding enterprise financial data of A-share listed companies in China’s Shanghai and Shenzhen stock markets from 2009 to 2019. We obtained enterprise financial data and patent data from the CSMAR database and the State Intellectual Property Office of the People’s Republic of China, respectively. The World Intellectual Property Organization (WIPO) issued an index list of environmentally friendly international patent classification according to the United Nations Framework Convention on Climate Change, which classifies green patents into eight major fields: alternative energy, transportation, waste management, energy conservation, alternative energy production, administrative regulation and design, agriculture and forestry, and nuclear power. This study screened and extracted the green patent data of sample-listed companies based on the green patent standard. Furthermore, we divided green patents into green invention patents and green utility model patents to reflect the different innovations and values of green patents. It is generally believed that the former is more innovative than the latter.
Given that green innovation is mainly concentrated in the manufacturing industry, we excluded financial, real estate, and other service industries and only retained the listed enterprises in the industrial sector: 1) enterprises that have been delisted are excluded; 2) enterprises with missing relevant variables are excluded; 3) ST, PT, and insolvent samples are also excluded; and 4) to reduce the effect of outliers, all micro-level continuous variables are subjected to the upper and lower 1% tail reduction in this study.
3.2 Variables
3.2.1 Green innovation
We used enterprises’ green innovation (GI) as an explained variable in the study. We measured the green innovation performance of enterprises by the number of green patent applications for two main reasons: first, there is a wealth of research on enterprise innovation, with existing studies typically measuring R&D expenses and innovation subsidies (Liu et al., 2021), but it is difficult to distinguish between R&D expenses or innovation subsidies specifically used for green innovation. Green patents can intuitively reflect the output of enterprises’ green technology innovation activities, with quantifiability and spillovers inside and outside the industry. Moreover, compared with R&D investment, patents have a clear technical classification. Data availability and accuracy are guaranteed. According to the nature of different technologies, the patent data can be further classified to reflect the different value connotations and contributions of innovation activities. Second, patent technology will likely impact enterprises during the application process, so the patent application data are more stable, reliable, and timely than the grant data.
Referring to Xiang et al. (2022); Gao et al. (2023); Wang et al. (2023), we use the number of green invention patent applications as a metric for evaluating the quality of green innovation (patent1). Meanwhile, sum up the application numbers for green invention patents and green utility model patents to evaluate enterprises’ green innovation quantity (patent2). Considering the typical “right-skewed” characteristics of green patent data, we add “1” to it and take the natural logarithm.
3.2.2 Digital transformation
Digital transformation (Dig) is used as another explanatory variable. We used textual analysis to construct digital transformation indicators for enterprises. First, drawing on existing digitalization studies and combining a series of important digital economy-related policy documents, we collated and summarized statistics on keywords closely related to digital transformation (it mainly includes artificial intelligence, 5G, fingerprint identification, biometrics, authentication, big data, data visualization, B2C, O2O, mobile payment, digital marketing, smart home, digital currency, physical information system, blockchain, human–computer interaction, and distributed computing). Then, considering that the essence of digital transformation is to introduce digital technology to cope with uncertain technological progress and market fluctuations, it is closely related to the development strategy, business operation, risk response, and other vital features of the enterprise. These features are mainly described and disclosed in the “Management Discussion and Analysis” section of the enterprise’s annual report. Therefore, to ensure that the digital indicators fit the actual situation of the enterprise, we focus on the “Management Discussion and Analysis” part and count the frequency of digital transformation words. 1 Finally, referring to Hassan et al. (2019), we constructed a digital transformation index by dividing the total number of sentences in the text by the total number of words of digital transformation keywords. The larger the indicator, the higher the degree of digitalization of the enterprise will be.
According to the annual statistics of enterprise digitalization during the sample period (Figure 2), at the micro level, the frequency of digitization-related terms disclosed in the annual report of each listed enterprise on average shows a yearly growth trend, from 18.10 times in 2009 to 60.3 times in 2019, i.e., a 3.33 times increase. It indicates the rapid integration of digital technology with the real economy. At the macro level, the White Paper on the Development of China’s Digital Economy (2020), released by the China Academy of Information and Communications Technology (China Academy of Information and Communications Technology, 2021), shows that the scale of China’s digital economy grew from 9.49 trillion yuan in 2011 to 35.7 trillion yuan in 2019, increasing 3.76 times. The growth of the digital economy at the macro and micro levels of statistics is basically comparable, which supports, to a certain extent, the rationality of the micro-enterprise digitization indicators constructed in this paper based on the text analysis method.
3.2.3 Control variables
To overcome the effect of omitted variables, we chose a set of enterprise-level control variables. The specific variables are defined as follows: enterprise size (Size): the natural logarithm of the enterprise’s total assets at year-end; enterprise age (Age): the natural logarithm of the enterprise’s length of time on the market; enterprise social value creation capacity (Tobin’s q): the logarithm of the ratio of the enterprise’s market value to the replacement cost of capital; enterprise debt (Debts): the logarithm of the ratio of the enterprise’s current year loan amount to total assets; enterprise return on total assets (Return on assets): the ratio of the enterprise’s net profit to total assets; capital intensity (Capital intensity): the ratio of the enterprise’s total assets to its operating revenue; share of the first largest shareholder’s shareholding (Largest shareholder): the share of the first largest shareholder’s shareholding to the total equity; share of independent directors (Independent directors): the ratio of the number of independent directors to the total number of board of directors.
The descriptive statistics of the main variables are shown in Table 1.
3.3 Empirical model
We refer to the practices of many scholars (Quan et al., 2021; Zhai et al., 2022), using the two-way fixed effects model to investigate the impact of digital transformation on green innovation in a general sense. Our model is shown as follows.
where i and t are subscripts referring to the enterprise and year, respectively. GIit is the proxy variable for enterprises’ green innovation. Digit refers to the degree of digital transformation in the enterprise. The magnitude of the coefficient measures the impact of digital transformation on enterprises’ green innovation. Xit is an enterprise-level control variable. We also control for year-fixed effects µt and enterprise-fixed effects θi to account for further enterprise- and time-varying factors, and εit is a random disturbance term. Furthermore, to avoid the problem of pseudo-significance and considering that the core explanatory variables are at the enterprise level, the standard errors are clustered at the enterprise level in this study.
4 Results and discussion
4.1 Baseline results
Table 2 reports the regression results of Eq. 1. The first two columns examine the relationship between digital transformation and the quality of green innovation in enterprises, which is strategically crucial for promoting green development and achieving the goal of carbon neutrality. The regression results show that in the case of a single-core explanatory variable and a series of control variables, the coefficients of Dig are 0.109 and 0.113, respectively, both significant at the level of at least 5%. This indicates that digital transformation has significantly improved the quality of enterprises’ green innovation. Patent2 corresponds to a significant positive regression coefficient, indicating that digital development significantly improves the output level of green innovation simultaneously, which is consistent with Li et al. (2021) and Mubarak et al. (2021). Moreover, in an economic sense, the findings reveal that each standard deviation increase in the degree of digital transformation leads to an increase in the quantity and quality of enterprises’ green innovation by 2.924% and 2.124%, respectively, when controlling for the effects of other factors. In conclusion, digital transformation positively impacts enterprises’ green innovation, and this result is economically significant. Thus, Hypothesis 1 is verified.
Improving the degree of enterprise digital transformation can promote the flow and integration of a large amount of data and information within the enterprise and quickly output available information. It sends a positive signal of trust and ability to the demanders and financial institutions, which improves the integration efficiency of R&D resources and knowledge while alleviating the financing constraints of enterprises. Finally, digital transformation promotes the improvement of enterprises’ green innovation transformation level in both quantity and quality, accelerating the reduction of supply-side emissions to achieve sustainable development.
4.2 Endogeneity problem
Although we introduce relevant control variables in the abovementioned benchmark analysis, there may still be unobservable factors, such as enterprise development concept and life cycle, that affect enterprises’ digital development and green innovation activities. It may cause the endogenous problem of missing variables. Additionally, the endogeneity problem of reverse causality is also prominent. On the one hand, improving enterprise digitalization can accelerate the diffusion of knowledge within the enterprise and promote green innovation. On the other hand, as the efficiency of enterprise green innovation development improves, it can force the enterprise to develop and progress toward a higher level of digitalization with stronger sharing, which provides an important source of motivation for better green transformation and upgrading. Based on this, this study adopted the following two approaches (as mentioned in Sections 4.2.1 and 4.2.2) to alleviate the endogeneity problem.
4.2.1 Instrumental variables
This paper constructed instrumental variables (IVs) for enterprise digitization levels based on Lewbel’s (1997) research to address the endogeneity problem. The method of constructing instrumental variables proposed by Lewbel (1997) is a way to construct valid internal instrumental variables without using external factors. Using the above method to construct instrumental variables helps somewhat eliminate endogeneity bias. Specifically, we use the third power of the difference between the digitization level of enterprise and the mean of digitized water by two-digit industry and province as the instrumental variable (Dig_iv). Table 3 reports the regression results of the IV estimation. The Kleibergen–Paap rk LM statistic is significant at the 5% level, rejecting the original hypothesis that the instrumental variable is under-identified. The Cragg–Donald Wald F statistic is greater than the critical value (16.38) of Stock–Yogo at the 10% significance level, rejecting the original hypothesis of weak instrumental variables. The above tests show that the instrumental variables selected in this paper are reasonable and reliable. The coefficients of the core explanatory variables remain significantly positive, indicating that this paper’s main conclusions still hold after eliminating the effect of endogeneity.
One may wonder why our coefficients for IV regression are larger than those in baseline regression. This is due to the problem of “local average treatment effect” (abbreviated as LATE) in instrumental variable analysis, which is generated by heterogeneity, meaning that each individual’s treatment effect is different. In this case, the use of instrumental variables may result in measuring the average processing effect of only a portion of individuals in the treatment group rather than the average processing effect of the treatment group. In this study, the instrumental variable Dig_iv only affects the choices of sensitive individuals in the sample companies who are undergoing digital transformation in the same province and industry. For individuals who engage in digital transformation, regardless of whether other companies in the same province or industry engage, digital transformation does not change their decision. Therefore, the green innovation incentive effect measured by Dig_iv may be much greater than the sample average level. That is why IV regressions produce larger coefficients.
4.2.2 PSM-DID
This paper re-estimates the impact of digital transformation on enterprises’ green innovation using difference-in-differences-based propensity score matching (PSM-DID). There were a total of 3,517 listed companies during the study period, of which 701 have never undergone digital transformation. We first construct a digital transformation dummy variable (Dig_dum) based on whether Dig is greater than 0. The value is 1 if the enterprise has undergone the digital transformation during the sample period (i.e., treatment group) and the observation time is in the year of the first digital transformation year and after. Otherwise, it is 0. Then, we select the control variables as covariates, calculating the propensity scores using the logit model. Using the one-to-one nearest-neighbor matching method, we have matched control groups with similar characteristics to those of enterprises that have never undergone digital transformation. Finally, difference-in-differences (DID) is used to estimate. The regression results are shown in Table 4. After matching, it turns out that the coefficient of the core explanatory variable is still significantly positive, and there is no significant difference from the previous results. This shows that the findings of this study are robust.
The parallel trend hypothesis is an important premise of DID. Referring to Deschênes et al. (2017), this paper uses the event study method to construct the following regression equation:
where
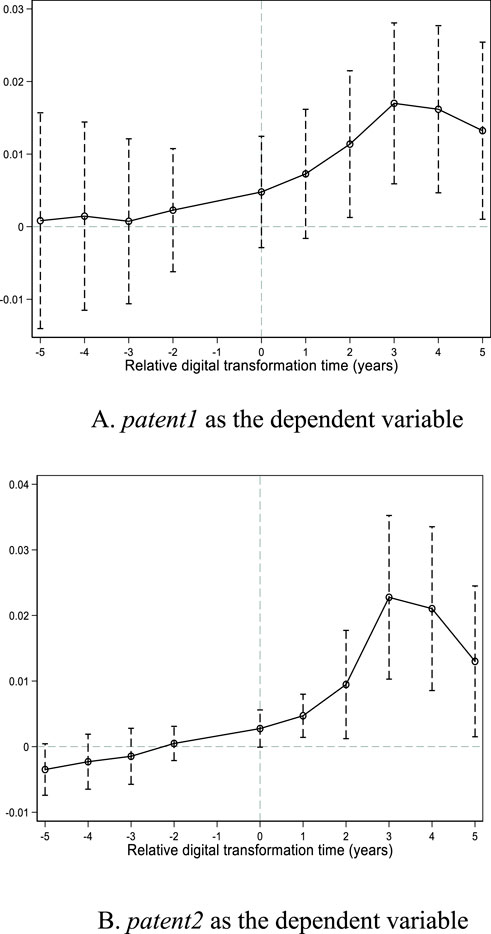
Figure 3. Parallel trend chart. Notes: Using n = −1 as the base year, (A) 95% confidence interval of the coefficient when patent1 is the dependent variable. (B) 95% confidence interval of the coefficient when patent2 is the dependent variable. We control for enterprise- and year-fixed effects for manufacturing enterprises. Standard errors are clustered at the enterprise level.
4.3 Robustness checks
To further improve the reliability of empirical results, we conducted the following robustness tests. First, we replaced the explanatory variables. We adopted the proportion of digitization-related intangible assets to total enterprise intangible assets (Dig1) as a measure of enterprise digitization. Second, we controlled for joint fixed effects. Considering that there may be differences in digital transformation and innovation incentive policies in different regions, industries, and years, we control the high-level joint fixed effects of province-year and industry-year based on benchmark regression. It alleviates the impact of changes in the macro-systemic environment on the regression results to a certain extent. Lastly, we replaced the regression model. Because the number of enterprises’ green innovations has the characteristic of left truncation at 0, it can lead to the problem of model regression bias. Therefore, this paper uses the Tobit model. Table 5 reports the robustness tests’ results, which are consistent with the benchmark results mentioned earlier.
5 Mechanism analysis
In this section, we will investigate why digital transformation is related to the increase in green innovation activities among enterprises. We considered three functional channels: first, digital transformation can ease the financing constraints of enterprises, thereby increasing enterprises’ capital investments in green research and development. Second, their increased green innovation activities may result from the reduced information constraints due to digital transformation. Finally, human capital may play an important role in the interlinkage between digital transformation and the improvement of green innovation performance among enterprises. Next, the abovementioned mechanisms are tested to explore the internal mechanisms of enterprises’ digitalization to promote their green innovation performance by “improving quality and efficiency.”
5.1 Financing constraint mitigation effect
The financing constraints faced by enterprises are an important reason for crowding out green innovation (Liu et al., 2022b). Unlike general innovation activities, green innovation emphasizes the realization of economic performance goals and the effective reduction of environmental pollution with new technologies and concepts. Enterprises need to invest in innovation in green product research and development, green process upgrading, end-of-pipe treatment, and other aspects with higher risks and more significant capital requirements. Once enterprises face severe financing constraints, they may actively reduce investment in green technology innovation. This paper illustrates the path for digital transformation necessary to improve enterprises’ green innovation level by examining whether digital transformation can affect enterprise financing constraints.
In an imperfect capital market, the difference between internal and external financing costs can cause the investment expenditure of enterprises to rely significantly on their internal cash flows. Investment cash flow sensitivity can measure the financing constraints of enterprises to a certain extent (Fazarri et al., 1988). Therefore, this paper uses the investment cash flow sensitivity (Fin_con) as the measurement index of the financing constraints of enterprises. The greater the sensitivity of investment cash flow, the higher the degree of financing constraints for enterprises. The regression results are shown in column (1) of Table 6. The coefficient value of the core explanatory variable is significantly negative, indicating that the digital transformation has significantly eased the constraints on enterprise financing. Thus, Hypothesis 2 is verified. The possible reason for this result is that applying big data, intelligent algorithms, cloud computing, and other digital technologies can help improve enterprise credit evaluation and increase information transparency for both credit parties. Furthermore, real-time decisions can be made according to the short-term financing needs of enterprises. Thus, enterprises can obtain financing support faster. Additionally, it can also drive enterprises to deleverage, stabilize financial conditions, and reduce financial risks. It better matches the risk characteristics of resources and enterprise innovation projects (Demertzis et al., 2018), enabling enterprises to choose financing channels. At the same time, digital systems can help enterprises establish credit records and drive the availability of traditional financing with data, thus effectively easing the financing constraints of enterprises.
5.2 Information asymmetry mitigation effect
We use the log of one plus the number of analysts tracking a particular firm to measure the overall information asymmetry (Infor) faced by enterprises. The information asymmetry is lower if the number of enterprises tracked by analysts is greater. The regression results are shown in column 2 of Table 6. The coefficient of Dig is significantly positive, indicating that digital transformation has somewhat alleviated information asymmetry. This is because, with digital technology enabled, the production data initially deposited in the enterprise can be collected and processed for practical mining and utilization. It is conducive to improving the efficiency of information exchange between all levels of the enterprise and effective communication with the outside. Therefore, the problem of information asymmetry in enterprises can be alleviated after implementing digital transformation. With the relaxation of information constraints, enterprises can effectively integrate innovation resources and the efficient cooperation of R&D personnel. It reduces green R&D costs and risks, giving enterprises more efficient ways to achieve green innovation activities. Moreover, improving external information disclosures by digital enterprises is conducive to the strict supervision of consumers and the government. It further enhances the sense of corporate environmental responsibility and reduces the ambiguity and uncertainty in green innovation decisions. It also helps form a trust mechanism between enterprises and external investors, which overcomes the problem of blocked capital sources when enterprises invest in innovation, thus promoting the green innovation activities of enterprises.
5.3 Human capital enhancement effect
Increasing human capital can effectively promote enterprise innovation. Excellent talent teams are the source and power of enterprises’ sustainable competitive advantage. Achieving green innovation goals not only requires enterprises to carry out pollution control at the end of production but also requires the development of new green technologies, processes, and management models to be integrated into the whole production process to balance enterprises’ green innovation targets. Therefore, to achieve the “quantity and quality” of green innovation, it is necessary to continuously introduce and cultivate high-technology talents with innovative thinking and abilities and then promote enterprises to shift from passive to active participation in the green innovation competition, thereby improving the quantity and quality of green innovation. In this paper, we took the log of the number of employees with bachelor’s degrees or higher (Edu1) and the proportion of the number of employees with bachelor’s degrees or higher among all employees (Edu2) as proxy indicators to measure the level of human capital. We examined the mechanism of the digital transformation mechanism to improve enterprises’ green innovation performance. The last two columns of the core explanatory variables in Table 6 are significantly positive, indicating that digital transformation significantly contributes to the level of human capital in enterprises. This is because digital development facilitates human capital to rapidly update its knowledge base and structure, which activates existing static human capital. Relying on the digital system, employees can quickly master new skills and accumulate new knowledge. It increases the knowledge reserve of human capital and thus improves the level of human capital within enterprises.
Overall, Hypothesis 2 is verified.
6 Heterogeneity testing
6.1 Regional heterogeneity: regional environmental protection intensity
We use the log of the frequency of words related to “environmental protection” in the annual provincial government work report as a proxy variable for the government’s environmental protection intensity. We divided the sample data into areas with low environmental protection intensity and areas with high environmental protection intensity based on the median environmental protection intensity. The regression results in Table 7 show that the coefficients are significantly positive for both high- and low-environmental protection areas. However, the effect of “quality and efficiency” of green innovation is much greater in high-intensity areas than in low-intensity areas. It implies that the impact of digitalization on green innovation is more significant in regions with high-environmental protection intensity.
6.2 Industry heterogeneity: heavy-polluting industry or not
According to the “List of Listed Companies’ Environmental Protection Verification Industry Classification and Management” formulated by the Ministry of Environmental Protection of China in 2008, 16 industries such as coal, mining, textile, petrochemical, pharmaceutical, chemical, metallurgy, and thermal power are classified as heavy-polluting industries. Others are classified as light-polluting industries. Next, according to the above criteria, the whole sample is divided into two groups of subsamples of the heavy- and non-heavy polluting industries, and the regression is again performed. Table 8 reports the results of the differential effects of belonging to the heavy-polluting industries on the relationship between digital transformation and green innovation. It can be found that the coefficients of Dig are positive and remain highly significant in the light-polluting industries. On the contrary, the coefficients of Dig are positive but insignificant in the heavy-polluting industries. It shows that, compared to the heavy-polluting industry, the digital transformation of enterprises in the light-polluting industry can produce a greater incentive effect for green innovation.
6.3 Enterprise heterogeneity: level of environmental investment
For enterprises with different levels of environmental investment, there are differences in the incentive effects of enterprise digitalization on their green technology innovation. We divide the sample data into companies with high and low levels of environmental investment and perform group regressions based on the median level of enterprise environmental investment. The results are presented in Table 9. The regression results show that for enterprises with high investment levels in environmental protection, improving enterprise digitalization levels can significantly encourage enterprises to engage in green innovation, whereas for those with low investment levels in environmental protection, improving enterprise digitalization levels cannot significantly impact enterprises’ green technology innovation levels. The above results show that, for enterprises paying more attention to green development, improving the digital level can better optimize their innovative technology resources. This promotes the improvement of the green innovation level of enterprises.
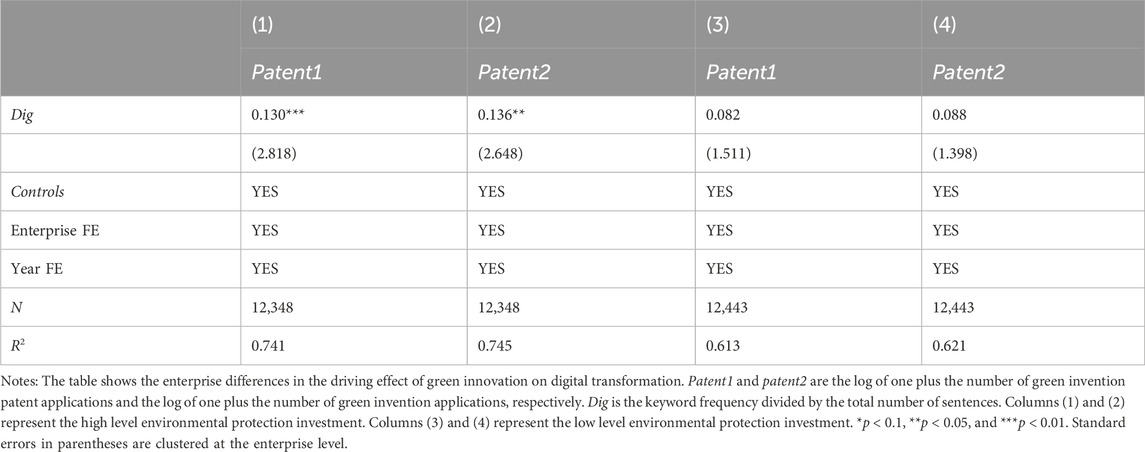
Table 9. Heterogeneous green innovation effects by enterprise environmental protection investment levels.
Overall, Hypothesis 3 is verified.
7 Conclusion and policy implications
Green innovation is an important way to realize the symbiotic and integrated development of enterprises and the social environment. Enterprises’ digitalization level is constantly improving in the context of the vigorous development of the digital economy. Studying whether digital transformation can promote green innovation in enterprises has important implications for the green development of the global economy. Using the A-share listed companies in the Shanghai and Shenzhen stock markets of China from 2009 to 2019, this paper examines the impact of digitalization on the green innovation of enterprises and its mechanisms. The study found that digital transformation has significantly improved enterprises’ green innovation levels in quantity and quality. The quantity and quality of green innovation in enterprises increased by 2.924% and 2.124%, respectively, for each standard deviation of the degree of digital transformation. The mechanism test shows that digital transformation has promoted the green innovation performance of enterprises mainly by easing the financing constraints and information asymmetry and improving the level of human capital of enterprises. Furthermore, heterogeneity analysis also found that the incentive effect of digitalization on enterprises’ green innovation is more significant in enterprises with higher investment levels in environmental protection, enterprises in non-heavy polluting industries, and regions with stronger environmental regulation.
Based on the empirical analysis, this study provides favorable evidence that digital development stimulates green innovation in enterprises and proposes the following policy implications: first, we should actively adapt to the digital transformation wave and fully grasp the opportunities created by it to promote economic structure optimization and kinetic energy. The government should speed up the construction of “new infrastructure” to build a complete digital infrastructure system and increase investment in 5G, industrial Internet, and big data industries. The government can also promote the continuous penetration of the digital economy into traditional industries and provide external technical support for the green transformation of industrial structures. Moreover, they can actively guide enterprises to consolidate the dividend advantage of digitalization for green and high-quality development. Second, digital finance should be used to unblock digital transformation’s information transmission mechanisms, alleviate enterprises’ internal and external information asymmetries, and guide the flow of financial resources to enterprises with green R&D motivation and conditions. Positive interaction should be promoted between enterprises, investors, and consumers by effectively breaking corporate financing constraints. Third, the differential impact of digital development on the green innovation performance of enterprises of different types, industries, and regions should be considered. The digital development strategy of differentiation, dynamism, and personalization should be implemented. Enterprises with low environmental protection investment levels should be encouraged to increase capital investment through fiscal policies. The government should moderately strengthen the intensity of environmental regulation for heavily polluting enterprises, encouraging enterprises to actively and voluntarily participate in environmental governance. Digital development can become the “hardware” technical support to effectively improve the quality of enterprise development and realize industrial upgrading.
Although this study draws reliable conclusions on the impact of digital transformation on green innovation, there are still some limitations that point to future research directions. First, for the measurement of digital transformation indicators, we use the text analysis method to measure digital transformation by counting the frequency of words related to “digitization” in enterprise annual reports, which can relatively objectively reflect more information. However, the implicit premise of this measurement method is the reliability and usefulness of annual report information. Hence, the measurement results are easily affected by false advertising, keyword selection, or other factors. Therefore, more efforts need to be made in future research to continuously improve the indicators. Second, our discussion on the impact of digital transformation on green innovation mechanisms lacks a more rigorous mathematical model analysis. In the future, we can consider constructing a theoretical model framework for three mechanisms to obtain more reliable conclusions. Third, our study did not consider the spillover effects of digital transformation and green innovation, which may overestimate the results of this article. Therefore, future research can consider introducing the spatial Durbin model (SDM) to obtain a deeper and more comprehensive understanding. Finally, this study examines the impact of overall digitization, but the impact of different dimensions of digital technology choices on green innovation may vary. Future research can incorporate analyses of different levels of digital technology based on this study.
Data availability statement
The original contributions presented in the study are included in the article/Supplementary Material; further inquiries can be directed to the corresponding author.
Author contributions
XW: writing–original draft and writing–review and editing. XZ: conceptualization, data curation, investigation, software, and writing–review and editing.
Funding
The authors declare financial support was received for the research, authorship, and/or publication of this article. Project of National Social Science Fund of China (21BGL251); Project of Henan Province Soft Science Research (232400411106); Open Bidding for Selecting the Best Candidates (OPSBC) of SSWR (24JB-03-09).
Conflict of interest
The authors declare that the research was conducted in the absence of any commercial or financial relationships that could be construed as a potential conflict of interest.
Publisher’s note
All claims expressed in this article are solely those of the authors and do not necessarily represent those of their affiliated organizations, or those of the publisher, the editors, and the reviewers. Any product that may be evaluated in this article, or claim that may be made by its manufacturer, is not guaranteed or endorsed by the publisher.
Footnotes
1First, we use Python’s crawler technology to extract the text content of “Management Discussion and Analysis” from the annual report. Then, use the “Jieba” Chinese word segmentation library of the Python software package to segment the above text and finally obtain the frequency of digital transformation keywords appearing in the annual report.
References
Bajari, P., Chernozhukov, V., Hortaçsu, A., and Suzuki, J. (2019). The impact of big data on firm performance: an empirical investigation. AEA Pap. Proc. 109, 33–37. doi:10.1257/pandp.20191000
Basu, S., and Fernald, J. (2007). Information and communications technology as a general-purpose technology: evidence from US industry data. Ger. Econ. Rev. 8, 146–173. doi:10.1111/j.1468-0475.2007.00402.x
Chen, S., Song, H., and Wu, C. (2021). Human capital investment and firms’ industrial emissions: evidence and mechanism. J. Econ. Behav. Organ. 182, 162–184. doi:10.1016/j.jebo.2020.12.002
China Academy of Information and Communications Technology (2021). The "white paper on the development of China's digital economy"[EB/OL]. Available at: http://www.caict.ac.cn/kxyj/qwfb/bps/202304/t20230427_419051.htm.
Demertzis, M., Merler, S., and Wolff, G. B. (2018). Capital markets union and the fintech opportunity. J. Financ. Regul. Compliance 4, 157–165. doi:10.1093/jfr/fjx012
Deschenes, O., Greenstone, M., and Shapiro, J. S. (2017). Defensive investments and the demand for air quality: evidence from the NOx budget program. Am. Econ. Rev. 107, 2958–2989. doi:10.1257/aer.20131002
Du, K., Cheng, Y., and Yao, X. (2021). Environmental regulation, green technology innovation, and industrial structure upgrading: the road to the green transformation of Chinese cities. Energy Econ. 98, 105247. doi:10.1016/j.eneco.2021.105247
El-Kassar, A. N., and Singh, S. K. (2019). Green innovation and organizational performance: the influence of big data and the moderating role of management commitment and HR practices. Technol. Forecast Soc. Change 144, 483–498. doi:10.1016/j.techfore.2017.12.016
Fan, F., Lian, H., Liu, X., and Wang, X. (2021). Can environmental regulation promote urban green innovation Efficiency? An empirical study based on Chinese cities. J. Clean. Prod. 287, 125060. doi:10.1016/j.jclepro.2020.125060
Fazzari, S., Hubbard, R. G., Petersen, B. C., Blinder, A. S., and Poterba, J. M. (1988). Financing constraints and corporate investment. Brookings Pap. Econ. Act. 1988, 141–406. doi:10.2307/2534426
Feng, H., Wang, F., Song, G., and Liu, L. (2022). Digital transformation on enterprise green innovation: effect and transmission mechanism. Int. J. Environ. Res. public health 19 (17), 10614. doi:10.3390/ijerph191710614
Ferreira, J. J., Fernandes, C. I., and Ferreira, F. A. (2019). To be or not to be digital, that is the question: firm innovation and performance. J. Bus. Res. 101, 583–590. doi:10.1016/j.jbusres.2018.11.013
Gao, Q., Cheng, C., and Sun, G. (2023). Big data application, factor allocation, and green innovation in Chinese manufacturing enterprises. Technol. Forecast. Soc. Change 192, 122567. doi:10.1016/j.techfore.2023.122567
Ghasemaghaei, M., and Calic, G. (2020). Assessing the impact of big data on firm innovation performance: big data is not always better data. J. Bus. Res. 108, 147–162. doi:10.1016/j.jbusres.2019.09.062
Goldstein, I., and Spatt, C. S. Ye M. (2021). Big data in finance. Rev. Financ. Stud. 34, 3213–3225. doi:10.1093/rfs/hhab038
Guo, Y., Xia, X., Zhang, S., and Zhang, D. (2018). Environmental regulation, government R&D funding and green technology innovation: evidence from China provincial data. Sustainability 10 (4), 940. doi:10.3390/su10040940
Hassan, T. A., Hollander, S., Van Lent, L., and Tahoun, A. (2019). Firm-level political risk: measurement and effects. Q. J. Econ. 134, 2135–2202. doi:10.3386/w24029
He, Q., Ribeiro-Navarrete, S., and Botella-Carrubi, D. (2023). A matter of motivation: the impact of enterprise digital transformation on green innovation. Rev. Manag. Sci. 18, 1489–1518. doi:10.1007/s11846-023-00665-6
Hong, M., Li, Z., and Drakeford, B. (2021). Do the green credit guidelines affect corporate green technology innovation? Empirical research from China. Int. J. Environ. Res. Public Health 18 (4), 1682. doi:10.3390/ijerph18041682
Hu, J., Wu, H., and Ying, S. X. (2022). Environmental regulation, market forces, and corporate environmental responsibility: evidence from the implementation of cleaner production standards in China. J. Bus. Res. 150, 606–622. doi:10.1016/j.jbusres.2022.06.049
IEA (2021). Digital demand-driven electricity networks initiative. Available at: https://www.iea.org/programmes/digital-demand-driven-electricity-networks-initiative.
Levinson, A. (2009). Technology, international trade, and pollution from US manufacturing. Am. Econ. Rev. 99, 2177–2192. doi:10.1257/aer.99.5.2177
Lewbel, A. (1997). Constructing instruments for regressions with measurement error when no additional data are available, with an application to patents and R&D. Econ. J. Econ. Soc. 65, 1201–1213. doi:10.2307/2171884
Li, X., Liu, J., and Ni, P. (2021). The Impact of the digital economy on CO2 emissions: a theoretical and empirical analysis. Sustainability 13, 7267. doi:10.3390/su13137267
Lin, C., and Kunnathur, A. (2019). Strategic orientations, developmental culture, and big data capability. J. Bus. Res. 105, 49–60. doi:10.1016/j.jbusres.2019.07.016
Liu, J., Jiang, Y., Gan, S., He, L., and Zhang, Q. (2022a). Can digital finance promote enterprise green innovation? Environ. Sci. Pollut. Res. 29, 35828–35840. doi:10.1007/s11356-022-18667-4
Liu, M., Liu, Y., and Zhao, Y. (2021). Environmental compliance and enterprise innovation: empirical evidence from Chinese manufacturing enterprises. Int. J. Environ. Res. Public Health18 18, 1924. doi:10.3390/ijerph18041924
Liu, Y., Xu, H., and Wang, X. (2022b). Government subsidy, asymmetric information and green innovation. Kybernetes 51, 3681–3703. doi:10.1108/K-05-2021-0422
Lordan, G., and Neumark, D. (2018). People versus machines: the impact of minimum wages on automatable jobs. Labour Econ. 52, 40–53. doi:10.1016/j.labeco.2018.03.006
Mubarak, M. F., Tiwari, S., Petraite, M., Mubarik, M., and Raja Mohd Rasi, R. Z. (2021). How Industry 4.0 technologies and open innovation can improve green innovation performance? Manag. Environ. Qual. Int. J. 32 (5), 1007–1022. doi:10.1108/meq-11-2020-0266
Munawar, S., Yousaf, H. Q., Ahmed, M., and Rehman, S. (2022). Effects of green human resource management on green innovation through green human capital, environmental knowledge, and managerial environmental concern. J. Hosp. Tour. Management52:52, 141–150. doi:10.1016/j.jhtm.2022.06.009
National School of Development of Peking University and Zhaopin Recruitment (2021). The "2022 employment relationship trends report - diversified employment relationships in the digital era. Available at: https://mp.weixin.qq.com/s/2pKifvKh7OkfLtsO0M4dbA.
Peng, Y., and Tao, C. (2022). Can digital transformation promote enterprise performance? from the perspective of public policy and innovation. J. Innovation Knowl. 7, 100198. doi:10.1016/j.jik.2022.100198
Quan, X., Ke, Y., Qian, Y., and Zhang, Y. (2021). CEO foreign experience and green innovation: evidence from China. J. Bus. Ethics 182, 535–557. doi:10.1007/s10551-021-04977-z
Romer, P. M. (1990). Endogenous technological change. J. Polit. Econ. 98, S71–S102. doi:10.1086/261725
Smith, C., Smith, J. B., and Shaw, E. (2017). Embracing digital networks: entrepreneurs' social capital online. J. Bus. Ventur 32 (1), 18–34. doi:10.1016/j.jbusvent.2016.10.003
Strambach, S. (2017). Combining knowledge bases in transnational sustainability innovation: microdynamics and institutional change. Econ. Geogr. 93, 500–526. doi:10.1080/00130095.2017.1366268
Tucker, P., and Duval, D. (2022). Why digital transformation needs to be at the heart of any mining rebrand strategy. Can. Min. J. (1), 143.
Wang, A., Si, L., and Hu, S. (2023). Can the penalty mechanism of mandatory environmental regulations promote green innovation? Evidence from China's enterprise data. Energy Econ. 125, 106856. doi:10.1016/j.eneco.2023.106856
Wu, L., Lou, B., and Hitt, L. (2019). Data analytics supports decentralized innovation. Manag. Sci. 65 (10), 4863–4877. doi:10.1287/mnsc.2019.3344
Wynarczyk, P., Piperopoulos, P., and McAdam, M. (2013). Open innovation in small and medium-sized enterprises: an overview. Int. Small Bus. J. 31, 240–255. doi:10.1177/0266242612472214
Xiang, X., Liu, C., and Yang, M. (2022). Who is financing corporate green innovation? Int. Rev. Econ. Finance 78, 321–337. doi:10.1016/j.iref.2021.12.011
Xie, R. H., Yuan, Y. J., and Huang, J. J. (2017). Different types of environmental regulations and heterogeneous influence on “green” productivity: evidence from China. Ecol. Econ. 132, 104–112. doi:10.1016/j.ecolecon.2016.10.019
Yong, J. Y., Yusliza, M. Y., Ramayah, T., and Fawehinmi, O. (2019). Nexus between green intellectual capital and green human resource management. J. Clean. Prod. 215, 364–374. doi:10.1016/j.jclepro.2018.12.306
Yoo, Y., Boland, Jr R. J., Lyytinen, K., and Majchrzak, A. (2012). Organizing for innovation in the digitized world. Organ. Sci. 23, 1398–1408. doi:10.1287/orsc.1120.0771
Zhai, H., Yang, M., and Chan, K. C. (2022). Does digital transformation enhance a firm's performance? Evidence from China. Technol. Soc. 68, 101841. doi:10.1016/j.techsoc.2021.101841
Zhan, L., Guo, P., and Pan, G. (2023). The effect of mandatory environmental regulation on green development efficiency: evidence from China. Environ. Sci. Pollut. 30 (4), 9782–9792. doi:10.1007/s11356-022-22815-1
Zhang, Q., Yang, M., and Lv, S. (2022b). Corporate digital transformation and green innovation: a quasi-natural experiment from integration of informatization and industrialization in China. Int. J. Environ. Res. Public Health 19 (20), 13606. doi:10.3390/ijerph192013606
Zhang, Y., Liu, H., and Li, M. (2022a). Does chief executive officer turnover affect green innovation quality and quantity? Evidence from China’s manufacturing enterprises. Environ. Sci. Pollut. Res. 30, 81760–81782. doi:10.1007/s11356-022-20365-0
Keywords: digital transformation, green innovation, information asymmetry, financing constraints, human capital
Citation: Wang X and Zhong X (2024) Digital transformation and green innovation: firm-level evidence from China. Front. Environ. Sci. 12:1389255. doi: 10.3389/fenvs.2024.1389255
Received: 21 February 2024; Accepted: 09 May 2024;
Published: 30 May 2024.
Edited by:
Abdul Majeed, Huanggang Normal University, ChinaReviewed by:
Shaham Saleem, Beijing Institute of Technology, ChinaYao Wang, University of International Business and Economics, China
Copyright © 2024 Wang and Zhong. This is an open-access article distributed under the terms of the Creative Commons Attribution License (CC BY). The use, distribution or reproduction in other forums is permitted, provided the original author(s) and the copyright owner(s) are credited and that the original publication in this journal is cited, in accordance with accepted academic practice. No use, distribution or reproduction is permitted which does not comply with these terms.
*Correspondence: Xiangfei Zhong, MjgxMzI0MjM3MUBxcS5jb20=