- Liaoning Provincial Key Laboratory for Hydrobiology, College of Fisheries and Life Science, Dalian Ocean University, Dalian, China
Freshwater biodiversity has been declining in urban areas, which may threaten ecosystem functions. Although many studies have demonstrated a positive correlation between biodiversity and ecosystem functioning (BEF) in terrestrial and marine ecosystems, little is known about the BEF relationship in freshwater environments, especially in highly urbanized regions where water pollution is a major concern. Eutrophication in urban water bodies may trigger algae blooms, decreasing the evenness or functional divergence (FDiv) of phytoplankton communities, thus negatively affecting ecosystem functioning. Through an annual field investigation, we clarified the relationship between phytoplankton diversity and ecosystem functioning, represented as resource use efficiency (RUE), in an urban river in northern China. Results indicated that evenness in the phytoplankton community contributes most to driving ecosystem functioning compared to environmental factors. The relative abundance of dominant Bacillariophyta was positively correlated with the resource use efficiency of phytoplankton (RUEpp) but negatively correlated with the resource use efficiency of zooplankton (RUEzp). Both phytoplankton evenness and functional divergence were negatively linked to RUEpp but positively to RUEzp. Our findings suggest that the reduction of phytoplankton evenness and functional divergence may seriously threaten resource use efficiency (RUE), and its potential mechanism can provide a crucial reference for water quality protection and sustainable water resource utilization in the basin.
1 Introduction
Environmental pressures resulting from intensified human activities severely threaten biodiversity and ecosystem structure, with freshwater ecosystems being particularly affected (Yi et al., 2014; Newbold et al., 2015; Zhao et al., 2019; Wang J. et al., 2021; Moi et al., 2022; Polazzo et al., 2022). One major threat is eutrophication (Rosset et al., 2014), which disrupts the natural balance of nutrients crucial for the stability of aquatic ecosystems (Huisman et al., 2018). Excessive nutrient inputs can trigger algal bloom outbreaks, reduce planktonic biodiversity (Wang et al., 2022), alter community structure (McQuatters-Gollop et al., 2009; Bužančić et al., 2016; Wang H. et al., 2021), reduce ecological niche differentiation, and intensify interspecific competition, thereby affecting ecosystem functions (Amorim and Moura, 2021). These blooms, often dominated by species such as Cyanobacteria, Chlorophyta, and Bacillariophyta, increase water turbidity and pH, resulting in anoxia and organism mortality (Visser et al., 2016). Furthermore, the intensity of the bloom also increases phytoplankton nutrient utilization but blocks the energy transfer from primary producers to zooplankton (Grey et al., 2000), which is not conducive to the acquisition of nutrients by zooplankton and negatively affects ecosystem functioning (Tian et al., 2017).
The relationship between biodiversity and ecosystem functioning (BEF) has long been a central theme in ecology. Considerable evidence suggests that biodiversity is critical for maintaining ecosystem functioning, and a loss of biodiversity reduces the capacity of ecosystems to provide multiple services (Cardinale et al., 2012; Naeem et al., 2012; Hagan et al., 2021; Mitchell et al., 2024), i.e., biodiversity positively influences ecosystem functioning (Cardinale et al., 2011; Tilman et al., 2014; Oliver et al., 2015; Soliveres et al., 2016; Slade et al., 2017). However, this relationship may also exhibit a non-linear pattern influenced by interspecific interactions and environmental heterogeneity (Cardinale et al., 2011; Thompson et al., 2017). Most of the early research on BEF relationships has focused on terrestrial ecosystems (Soliveres et al., 2016; Schuldt et al., 2018), particularly terrestrial plants, while aquatic ecosystems have received less attention (Daam et al., 2019). Globally, the biodiversity of freshwater systems is declining faster than that of terrestrial and marine systems (Vaughn, 2010; Zhang et al., 2019), and its loss will seriously threaten ecosystem stability and productivity.
The BEF relationship of phytoplankton was first proposed by Ptacnik et al. (2008), who used resource use efficiency (RUE) to indicate ecosystem functioning. RUE quantifies ecosystem functioning by measuring the ratio of resources acquired by organisms to the amount of biomass they transform and serves as a better indicator of the level of resource use by organisms (Hodapp et al., 2019). As one of the most important primary producers in river ecosystems (Varol, 2019), phytoplankton communities reflect their fundamental and critical role in material cycling, pollutant degradation, water self-purification, and ecosystem stability (Yang et al., 2022). Due to their tiny unicellular size and extreme sensitivity to environmental changes (Feio et al., 2009), phytoplankton are susceptible to both abiotic factors, such as nutrient salinity and water temperature, as well as biotic factors, such as grazing and interspecific competition. Consequently, they are considered ideal aquatic ecological indicator organisms (Wojciechowski et al., 2017). In the context of China’s rapid urbanization and industrialization, changes in phytoplankton diversity significantly reflect the response and adaptation of river ecosystems to environmental stress. In particular, increased nutrients from industrial wastewater and urban runoff cause eutrophication of water bodies, affecting phytoplankton community structure and function, reducing water quality, and threatening the overall aquatic biodiversity (Mustapha et al., 2013; Barakat et al., 2016). Therefore, monitoring and analyzing changes in phytoplankton diversity not only assesses the health of ecosystems but also provides a critical scientific basis for developing effective environmental management strategies.
While numerous studies have confirmed the overall positive correlation between BEF, most have focused solely on taxonomic diversity metrics such as species richness (Duffy et al., 2017; Amorim and Moura, 2021). In contrast, evenness metrics, which reflect the uniformity of species distribution within communities, and functional diversity metrics, which describe the functional traits of species, have received limited attention. Recent studies have concluded that the relationship between phytoplankton evenness and ecosystem functioning (measured by resource use efficiency) generally shows a negative effect in highly disturbed ecosystems, i.e., dominant species with superior resource utilization capabilities outcompete others (Filstrup et al., 2019; Otero et al., 2020). Furthermore, evenness is considered a sensitive indicator of changes in biodiversity, often reflecting the impacts of anthropogenic activities or environmental changes better than species richness and eliciting rapid responses in ecosystem functioning (Chapin III et al., 2000; Wilsey and Potvin, 2000; Filstrup et al., 2014; Hodapp et al., 2015). In contrast, species richness ignores the effects of differences in relative abundance among species on interspecific interactions and overemphasizes the importance of rare species (Zhang et al., 2012; Filstrup et al., 2014), which does not adequately represent the diversity associated with ecosystem functioning. In particular, changes in evenness often occur with little or no change in species richness, i.e., species tend to decline in number before going extinct, and evenness can capture such species declines (Wilsey and Potvin, 2000; Hillebrand et al., 2008). Consequently, the effects of evenness on ecosystem functioning warrant further in-depth investigation (Wilsey and Potvin, 2000).
Field and experimental studies have demonstrated that functional diversity is a highly effective predictor of ecosystem functioning, making it a critical aspect of contemporary biodiversity research (Griffin et al., 2009; Abonyi et al., 2017). In this context, the functional diversity indices proposed by Villéger et al. (2008), which comprehensively assess functional traits such as growth forms, habitat preferences, and resource use strategies, have received widespread recognition in the international scientific community (Eisenhauer et al., 2019). Compared to traditional research focused on taxonomic diversity, this species trait-based method of analysis more accurately reflects the actual functional roles of species within ecosystems. This approach highlights the functional differences among species and addresses the problem of traditional biodiversity indices that treat the contributions of each species equally (Cadotte et al., 2011), making it a promising tool for biological monitoring and ecosystem management. In particular, in the study of phytoplankton, by analyzing the relationship between their functional diversity and ecosystem functions, we can better understand the critical contributions of these microorganisms to primary productivity and nutrient cycling in water bodies. This will further elucidate the complex response mechanisms of aquatic ecosystems to environmental change, providing a solid foundation for the formulation of scientific ecological conservation and management strategies.
Currently, studies of aquatic biodiversity tend to focus on biodiversity effects within individual trophic levels, with less attention paid to vertical biodiversity effects across multiple trophic levels (Duffy et al., 2007). This approach may overlook potential trophic connectivity effects during urbanization, resulting in a lack of understanding of the role of individual taxa in food webs and their impact on ecosystem functioning (Eisenhauer et al., 2013; Li et al., 2021; Moi et al., 2021). Organisms drive ecosystem functioning at multiple trophic levels, and considering trophic complexity is critical to understanding the role of biodiversity at different trophic levels (Li et al., 2020). Primary producers can have different effects on ecosystem functioning within and across trophic levels (Filstrup et al., 2014). Results that consider only a single trophic group are likely to underestimate the importance of biodiversity and fail to effectively reflect the complexity of river and lake ecosystems (Weijters et al., 2009; Soliveres et al., 2016; Li et al., 2020). Therefore, it is necessary to integrate data from multiple trophic levels and horizontally explore the ecosystem functions of communities at different trophic levels to improve our understanding of the ecological health of rivers.
The Xiaoqing River, situated in northern China, is a highly urbanized area where intensive human activities have led to eutrophication of the water and the outbreaks of algal blooms. This makes it an ideal ecosystem to empirically test the relationship between biodiversity and river ecosystem functioning (Wang and Yin, 2023). We used phytoplankton taxonomic diversity (evenness) and functional diversity (functional divergence) as indicators of biodiversity, and resource use efficiency of phytoplankton (RUEpp) and zooplankton (RUEzp) communities as measures of ecosystem functioning. As one of the most important primary producers in river ecosystems, the diversity change of phytoplankton can reflect the response of rivers to environmental stress, while RUE can comprehensively reflect the level of resource utilization by biomes, and the correlation analysis between the two can help to elucidate the intrinsic mechanism underlying the biodiversity-ecosystem functioning relationship. We tested the following research hypotheses: (1) Phytoplankton evenness would be negatively correlated with RUEpp but positively correlated with RUEzp. (2) Functional divergence (FDiv) of the phytoplankton community would be negatively correlated with RUEpp but positively correlated with RUEzp. (3) The increase of dominant species caused by algal blooms would lead to an increase in RUEpp and a decrease in RUEzp.
2 Materials and methods
2.1 Study area
The Xiaoqing River, with a length of approximately 240 km, originates in Jinan City (He et al., 2018), Shandong Province, northern China (Figure 1). It spans five cities in Shandong Province (Jinan, Binzhou, Zibo, Dongying, and Weifang) (Jiang et al., 2017), with a watershed area of approximately 10,336 km2 (Zhang et al., 2020). This area has a temperate monsoon climate, with an average annual mean temperature of 14.7°C and an average annual precipitation of 1,043.4 mm. The Xiaoqing River is mainly used for flood control, irrigation, and navigation (He et al., 2020). However, with the rapid economic and social development of Jinan City, the land use in the basin has changed significantly (Liu et al., 2019), leading to the worsening of eutrophication in the Xiaoqing River and severe damage to the ecosystem (Zhang et al., 2023). In the present study, we monitored ten fixed sites along the Jinan section of the Xiaoqing River on a monthly basis from April 2020 to March 2021.
2.2 Collection and measurement of environment parameters
A portable multi-parameter water quality monitor (AZ86031) was used in the field to measure water temperature (WT), water depth (D), pH, dissolved oxygen (DO), transparency (Trans), turbidity (Tur), suspended solids (SS) and conductivity (EC) at each sampling site (Jiang et al., 2017). We collected 2 L of water samples at a depth of 0.5 m below the surface. The water samples were stored in a cold, dark environment, transported to the laboratory for further analysis, and then divided into two subsamples: one for water chemistry analysis and the other for quantitative phytoplankton analysis. The following indicators were determined according to the national environmental protection standards issued by the Ministry of Environmental Protection: ammonia nitrogen (NH3−-N), nitrate nitrogen (NO3−-N), nitrite nitrogen (NO2−-N), and phosphate (PO43–-P) were determined by ultraviolet spectrophotometry, the permanganate index (CODMn) was determined by the permanganate method (GB11892-89), total nitrogen (TN) was determined by UV spectrophotometry using alkaline potassium persulphate digestion, and total phosphorus (TP) was determined by the ammonium molybdate spectrophotometric method (Zhou et al., 2022). Chlorophyll-a content was determined by the hot ethanol method: the samples were extracted with 90% ethanol solution after filtration, and the supernatant was taken after a water bath, shaking, centrifugation, and the chlorophyll-a concentration was determined spectrophotometrically (Chen and Gao, 2000).
2.3 Plankton sampling and analysis
The collected phytoplankton quantitative samples were placed in 1 L plastic bottles, labeled, and fixed in the field by adding 1%–1.5% (v/v) of Lugol’s reagent. After 24 h of sedimentation, the samples were concentrated to 100 mL (Zhang et al., 2021). Quantitative samples of zooplankton were collected with a water sampler at different water levels in each section, with 50 L of mixed water samples filtered through a 25# plankton mesh (64 μm mesh diameter) (Lu et al., 2021). The filtered samples were then preserved in 100 mL sample bottles with 4% formaldehyde solution.
Referring to the relevant diagrams (Wang, 1961; Jiang, 1979; Zhu and Chen, 2000; Hu and Wei, 2006; Wang, 2020), phytoplankton and zooplankton samples were identified and analyzed to the lowest possible taxonomic level (i.e., species level when possible) using the field-of-view method under a 400x light microscope (SOPTOP, EX20). Density and biomass of phytoplankton and zooplankton in quantitative samples were calculated based on the identification results (Supplementary Appendix S1, S2), where biomass was derived from density multiplied by the average individual weight (biomass = density x weight).
The density of phytoplankton (i.e., the number of phytoplankton per liter of water, Np) was calculated as follows:
Where:
Np is the number of phytoplankton per liter of water;
Cs is the area of the counting frame (mm2);
Fs is the area of one field of view;
Fn is the number of fields of view counted;
V is the volume of 1 L of water sample after sedimentation and concentration (100 mL);
v is the volume of the counting frame (0.1 mL); Pn is the number of individual phytoplankton counted in Fn fields of view.
The density of zooplankton (i.e., the number of zooplankton per liter of water, Nz) was calculated as follows:
Where:
Nz is the number of zooplankton per liter of water;
Vs is the volume after sedimentation (100 mL);
N is the number of individuals obtained;
V is the sampled volume (50L);
Va is the volume of the counting frame (1 mL).
The Technical Specification for Classification and Monitoring of Algal Blooms, issued by Guangdong Province in December 2020, indicates that the common phylum of water blooms, including Cyanobacteria and Bacillariophyta, can be classified into five categories. The results of the phytoplankton density calculations conducted in this study indicate that the present study area can be defined as Class III Bacillariophyta bloom (density >5 × 106cells/L).
2.4 Phytoplankton taxonomic and functional diversity
In this study, taxonomic diversity and functional diversity were used to investigate BEF relationships in the Xiaoqing River across different seasons. Measures of phytoplankton taxonomic diversity included species richness and evenness. Species richness was quantified as the number of species in a community, while evenness was a measure of the uniformity in the distribution of individual species abundances within a community. A higher value of evenness indicates a more balanced distribution of individuals among species and a more stable community. Evenness is calculated as J′ = H′ /ln S, where H′ is the Shannon-Wiener diversity index and S is the number of species in the sample.
With reference to previous studies (Litchman and Klausmeier, 2008; Otero et al., 2020; Zhou et al., 2022), we selected ten categories of traits related to phytoplankton morphology, growth and reproduction, and habitat characteristics, including algal size, morphology, motility, reproduction, nitrogen-fixing capacity, and habitat suitability traits. These traits are closely related to phytoplankton resource acquisition, predator avoidance, and reproduction (Table 1). Phytoplankton trait data and density data were then used to calculate functional richness (FRic), functional evenness (FEve), and functional divergence (FDiv) of the phytoplankton community in the “FD” package of the R language. Among the indices, FRic refers to the volume of multidimensional space occupied by all species in the functional space of the community. It reflects the degree of utilization of ecological space, with a larger index indicating a higher degree of utilization. (2) FEve is defined as the uniformity of the distribution of functional traits of organisms in a community in an ecological space. The larger the index, the more comprehensive the utilization of effective resources, and the higher the efficiency of resource utilization. (3) FDiv reflects changes in biological characteristics within a community, as well as the degree of ecological niche differentiation and the degree of competition for resources. A higher FDiv indicates a higher degree of complementarity among the organisms within the community, accompanied by a weaker degree of competition.
2.5 Resource use efficiency
We used resource use efficiency (RUE) to quantify the ratio of realized resources to potential productivity as a metric for river ecosystem functioning (Hodapp et al., 2019). Total phosphorus (TP) was used as a proxy for potential phytoplankton productivity, while phytoplankton biomass represented the realized resources. Phytoplankton resource use efficiency (RUEpp) was expressed as the ratio of phytoplankton biomass to TP concentration. On the other hand, zooplankton resource use efficiency (RUEzp) was calculated as the ratio of zooplankton biomass (realized resource) to phytoplankton biomass (potential productivity) (Ptacnik et al., 2008; Filstrup et al., 2014; 2019). The specific equations are as follows:
2.6 Statistical analyses
Two multiple linear regression models were constructed using the “lm” function in R to assess the impact of the predictor variables—environmental factors and biodiversity—on the response variables, specifically RUEpp and RUEzp. Sixteen environmental variables and five biodiversity indicators were included in the models: water temperature (WT), water depth (D), transparency (Trans), turbidity (Tur), pH, electrical conductivity (EC), dissolved oxygen (DO), ammonia nitrogen (NH3−-N), nitrite nitrogen (NO2−-N), nitrate nitrogen (NO3−-N), total nitrogen (TN), total phosphorus (TP), phosphate (PO43–-P), permanganate index (CODMn), suspended solids (TSS), chlorophyll-a (Chl-a), species richness, evenness, functional divergence (FDiv), functional evenness (FEve), and functional richness (FRic). The analysis quantified the overall explanatory power of these variables on RUE and determined the relative contribution of each predictor, calculated as the square of its regression coefficient divided by the sum of the squares of all regression coefficients (Johnson and Lebreton, 2004). The Shapiro-Wilk normality test of normality indicated a non-normal distribution of RUEpp and RUEzp, which prompted a logarithmic transformation. All variables were standardized using Z-scores prior to regression analysis. The vif function of the “car” package assessed multicollinearity through variance inflation factor (VIF) values, and Pearson correlation coefficients were calculated to assess relationships between variables, facilitating the identification and resolution of multicollinearity.
Based on the Bray-Curtis distance calculated for plankton community composition, we used Principal Coordinate Analysis (PCoA) and PERMANOVA in the “vegan” package in R to test whether there were significant multivariate differences in phytoplankton and zooplankton community structure and assembly between seasons. The post hoc function pairwiseAdonis was used for multiple comparisons between seasons in the PERMANOVA analysis (Sun et al., 2023). One-way ANOVA was used to assess variability in RUE between seasons, while the Kruskal-Wallis test was used to assess differences in relative phytoplankton abundance between seasons. Results were presented as box plots. Watershed boundaries and stream systems were depicted using digital elevation modeling in ArcGIS version 10.8. The calculation of phytoplankton evenness and species richness was conducted in the R language “vegan” package, and the phytoplankton functional richness (FRic), functional evenness (FEve), and functional divergence (FDiv) were implemented in the package “FD”. We used Pearson’s correlation analyses to determine the relationship between dominant algae and RUE, and the direction and strength of the BEF relationship, with predictor variables standardized for Z-scores before analysis. Pearson’s correlation analyses, one-way ANOVA, Kruskal-Wallis tests, correlation heatmaps, and box plots were implemented in the Origin 2023 software, with significance levels set at p < 0.05, and the rest of the analyses were performed in R language (4.3.1).
3 Results
3.1 Contribution of environmental factors and biodiversity
After calculating the VIF values and the correlation coefficients between the variables, we found that the VIF values of all 21 variables were less than 10. Therefore, we addressed multicollinearity based on the correlation coefficients by discarding one of the variables with correlation coefficients higher than 0.5 to ensure the model’s robustness (Appendix S3). The removed variables included turbidity (Tur), pH, electrical conductivity (EC), ammonia nitrogen (NH3−-N), total nitrogen (TN), chlorophyll-a (Chl-a), and species richness (Richness). The results of the multiple linear regression showed that the adjusted R2 of the RUEpp and RUEzp models were 0.658 and 0.499, respectively, indicating that the models were able to explain a considerable portion of the variation in the data. The higher the contribution, the more the independent variable explains the variation in the dependent variable. In the models, evenness had the most significant explanatory effect on both RUEpp and RUEzp, with contributions as high as 66.933% and 71.535%, respectively, which were statistically highly significant (p < 0.001). Among the functional diversity indicators, functional divergence made the largest contribution, with the degree of explanation for RUEpp being particularly important. Additionally, TP contributed the most to RUEpp among environmental factors, while NO3−-N contributed the most to RUEzp (Tables 2, 3).
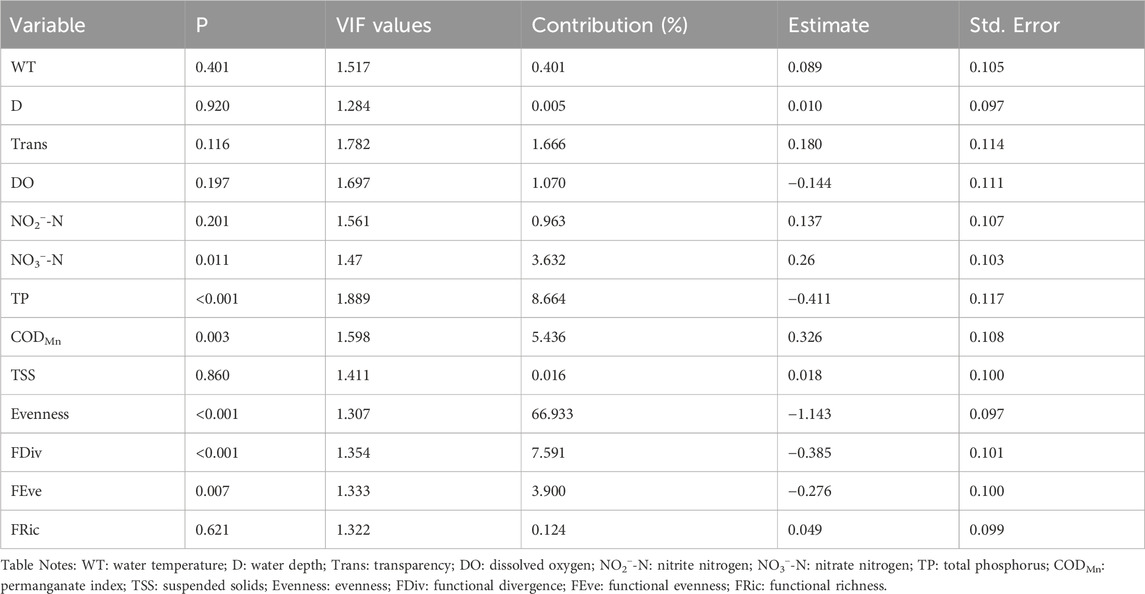
Table 2. Multiple regression analysis models based on predictor variables (numerous environmental factors and biodiversity indexes) and response variable RUEpp. The “Contribution” column in the table quantifies the contribution of a single explanatory variable to the multiple linear regression model of the dependent variables RUEpp.
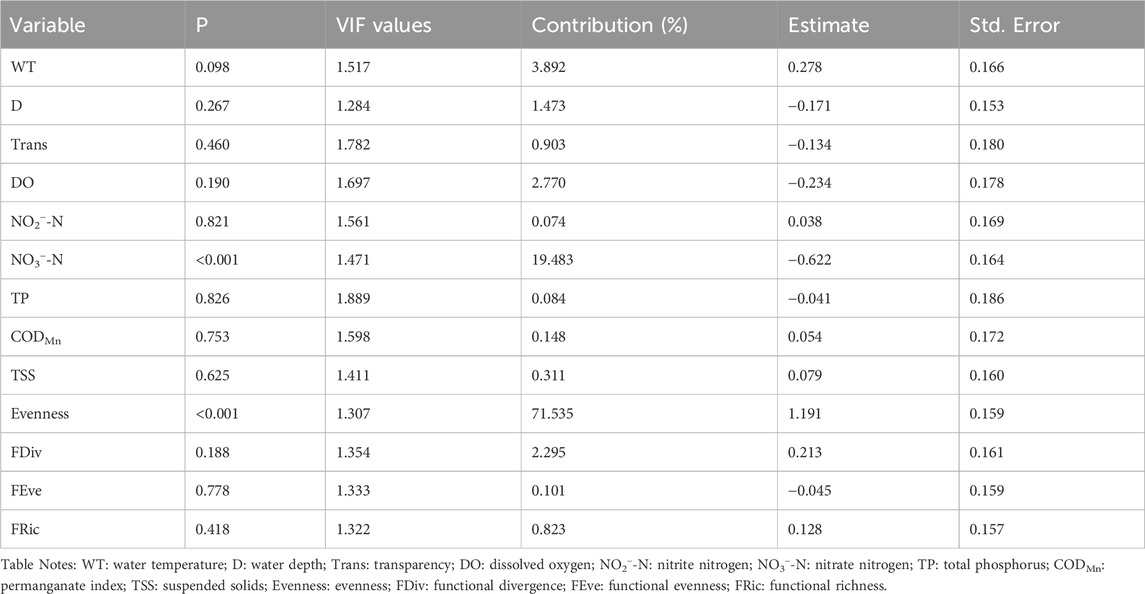
Table 3. Multiple regression analysis models based on predictor variables (numerous environmental factors and biodiversity indexes) and response variable RUEzp. The “Contribution” column in the table quantifies the contribution of a single explanatory variable to the multiple linear regression model of the dependent variables RUEzp.
3.2 Differences in plankton community composition and RUE across seasons
The plankton community composition collected from the Xiaoqing River during the four seasons tended to cluster separately. The phytoplankton and zooplankton community compositions differed significantly among seasons (R2 = 0.113, p < 0.001; R2 = 0.117, p < 0.001), and the pairwise differences in phytoplankton community composition among all four seasons were also significant (p < 0.05), indicating that structure of phytoplankton and zooplankton assemblages was significantly affected by seasonal changes (Figure 2).
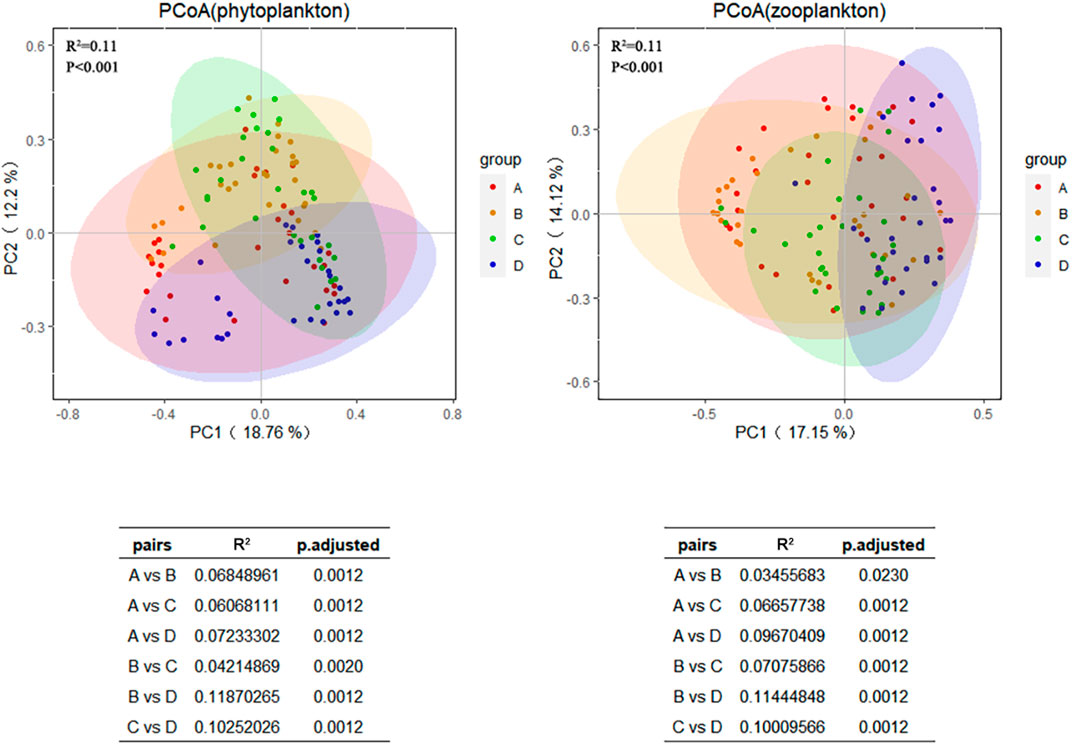
Figure 2. Principal coordinate analysis (PCoA) and PERMANOVA tests for phytoplankton and zooplankton communities in four seasons based on Bray-Curtis distance. Different graph colors represent different seasons: A is spring, B is summer, C is autumn, and D is winter, and p < 0.05 is considered significant. The horizontal and vertical axes represent the first and second principal coordinates and their contribution to community differences.
Significant differences were observed in RUEpp and RUEzp across seasons (Figure 3), with RUEpp being significantly higher in spring than in the other seasons. However, RUEzp was higher in summer and autumn compared to winter. In addition, the relative abundance of Bacillariophyta was significantly higher than other phyla in all four seasons, and they dominated the phytoplankton community (Figure 4), followed by the Chlorophyta and Cyanobacteria, whereas the species of Chrysophyta, Xanthophyta, Cryptophyta, Euglenophyta, and Dinophyceae accounted for a smaller total proportion. As the relative abundance of Bacillariophyta increased, RUE showed an increasing trend (R2 = 0.111, p < 0.001), while RUEzp was negatively correlated (R2 = 0.164, p < 0.001) (Figure 5). This finding indicates that the increase in the Bacillariophyta population significantly contributed to RUEpp, while it significantly reduced RUEzp.
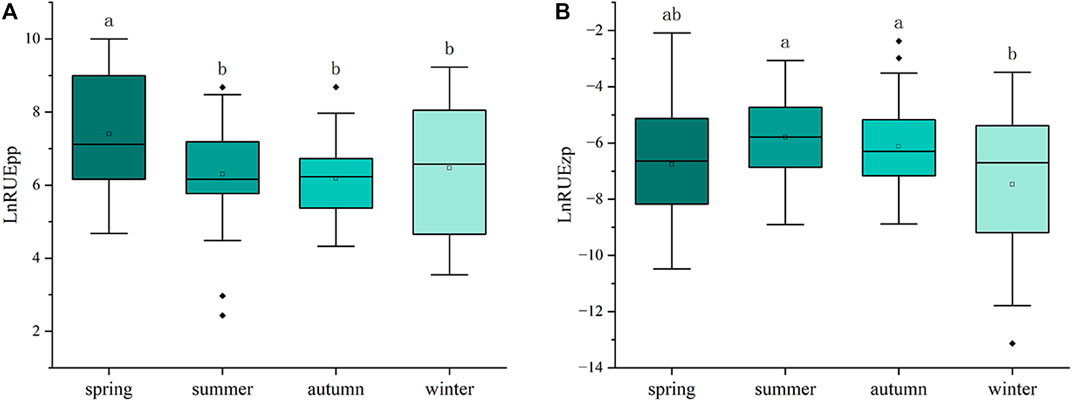
Figure 3. Box plots depicting the Resource Use Efficiency (RUE) of phytoplankton (A) and zooplankton (B) across spring, summer, autumn, and winter. One-way ANOVA was employed to assess the seasonal differences in RUE. To further determine specific differences between seasons, the Least Significant Difference (LSD) post-hoc test was performed. Different lowercase letters above each season indicate statistically significant differences (p < 0.05).
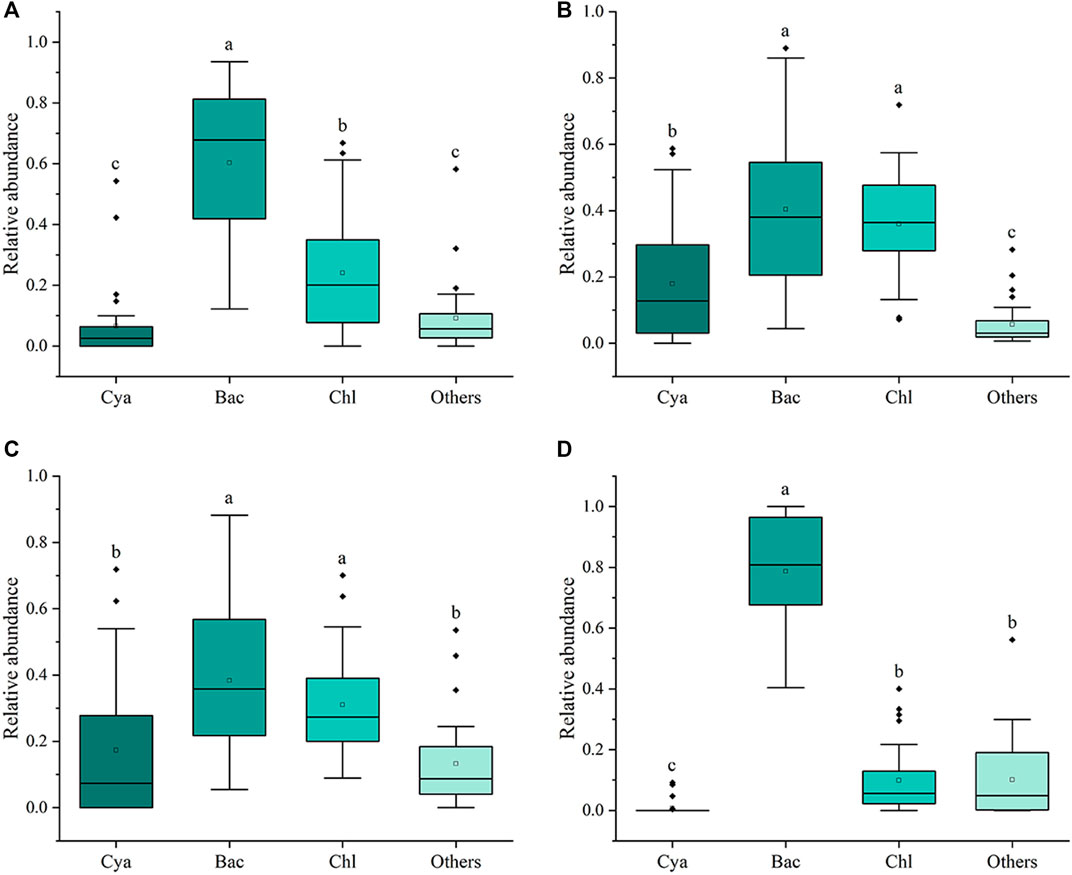
Figure 4. Box plot showing the relative abundance of phytoplankton phyla under four seasons: spring (A), summer (B), autumn (C), and winter (D), where Cya represents the Cyanobacteria, Bac means the Bacillariophyta, Chl means the Chlorophyta, and Others means the total relative abundance of the Chrysophyta, Xanthophyta, Cryptophyta, Euglenophyta, and Dinophyceae. Significant differences are indicated by different lowercase letters (p < 0.05).
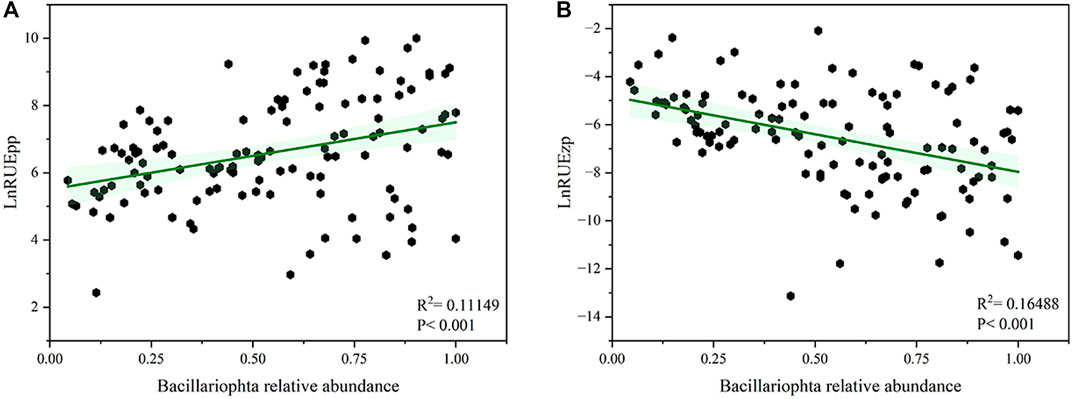
Figure 5. Relative abundance of dominant algae about phytoplankton resource use efficiency [RUEpp; (A)] and zooplankton resource use efficiency [RUEzp; (B)]. The predictor variable was the relative abundance of Bacillariophyta, and the response variable was transformed to a natural logarithm before analysis, and p < 0.05 was considered a significant difference.
3.3 Effect of taxonomic diversity on resource use efficiency
RUEpp showed a decreasing trend with increasing evenness in all seasons except autumn (spring: R2 = 0.672, p < 0.001; summer: R2 = 0.372, p < 0.001; winter: R2 = 0.671, p < 0.001). In contrast, RUEzp exhibited an increasing trend with increasing evenness in spring, summer, and winter (spring: R2 = 0.488, p < 0.001; summer: R2 = 0.208, p = 0.006; winter: R2 = 0.557, p < 0.001) (Figure 6). These results suggest that the dominant phytoplankton species (e.g., Bacillariophyta) exhibit specific physiological characteristics and thus utilize resources more efficiently, but the acquired resources were not effectively transferred to the next trophic level.
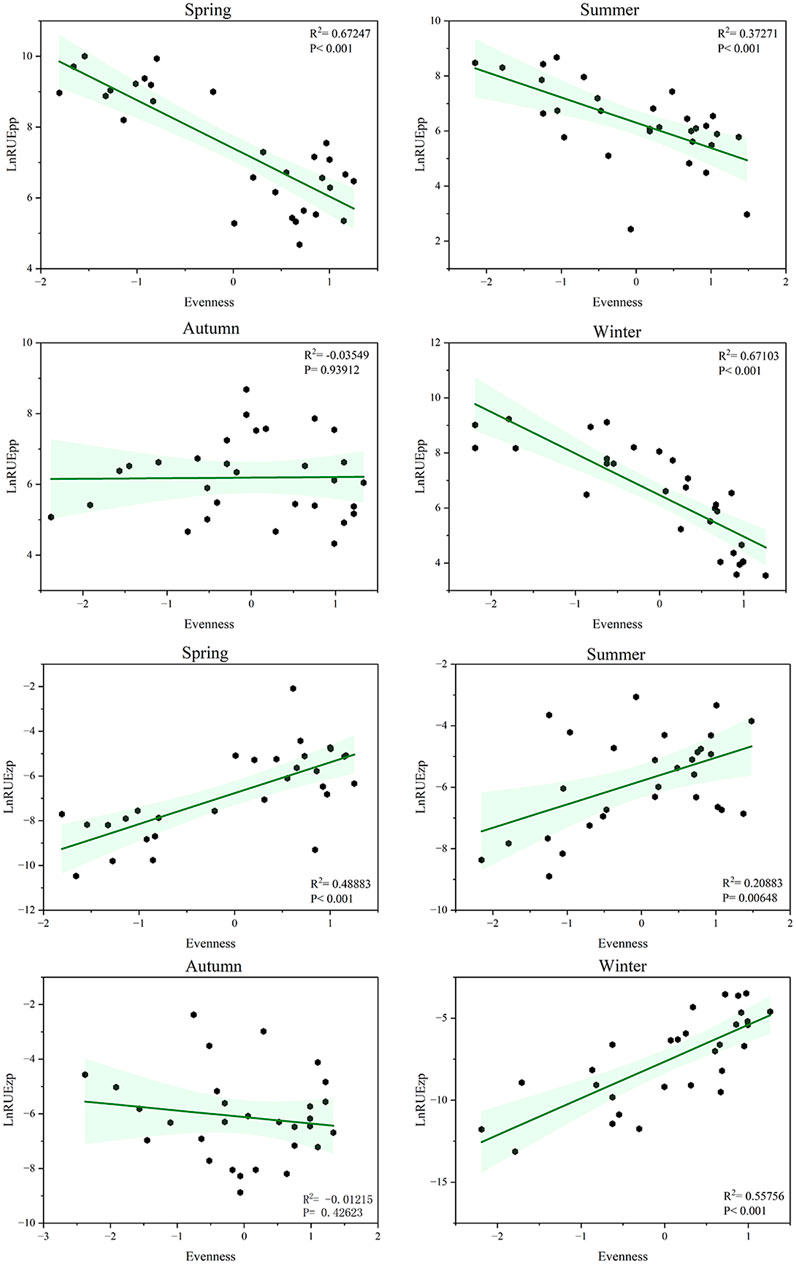
Figure 6. Relationship between taxonomic diversity and ecosystem function in Xiaoqing River in each season. The response variables were phytoplankton resource use efficiency (RUEpp) and zooplankton resource use efficiency (RUEzp), and the explanatory variable was phytoplankton evenness. Green shading represents 95% confidence intervals, and the response variables were transformed to natural logarithms before analysis.
3.4 Effect of functional diversity on resource use efficiency
Except in winter, RUEpp showed a significant negative correlation with FDiv (spring: R2 = 0.258, p = 0.002; summer: R2 = 0.216, p = 0.005; autumn: R2 = 0.167, p = 0.014), whereas RUEzp was significantly positively correlated (spring: R2 = 0.183, p = 0.010; summer: R2 = 0.167, p = 0.014; autumn: R2 = 0.221, p = 0.005) (Figure 7). These results suggest that the higher the degree of divergence in phytoplankton functional traits, the lower the RUEpp, whereas zooplankton feeding on a more functionally diverse phytoplankton assemblage exhibited a higher RUE.
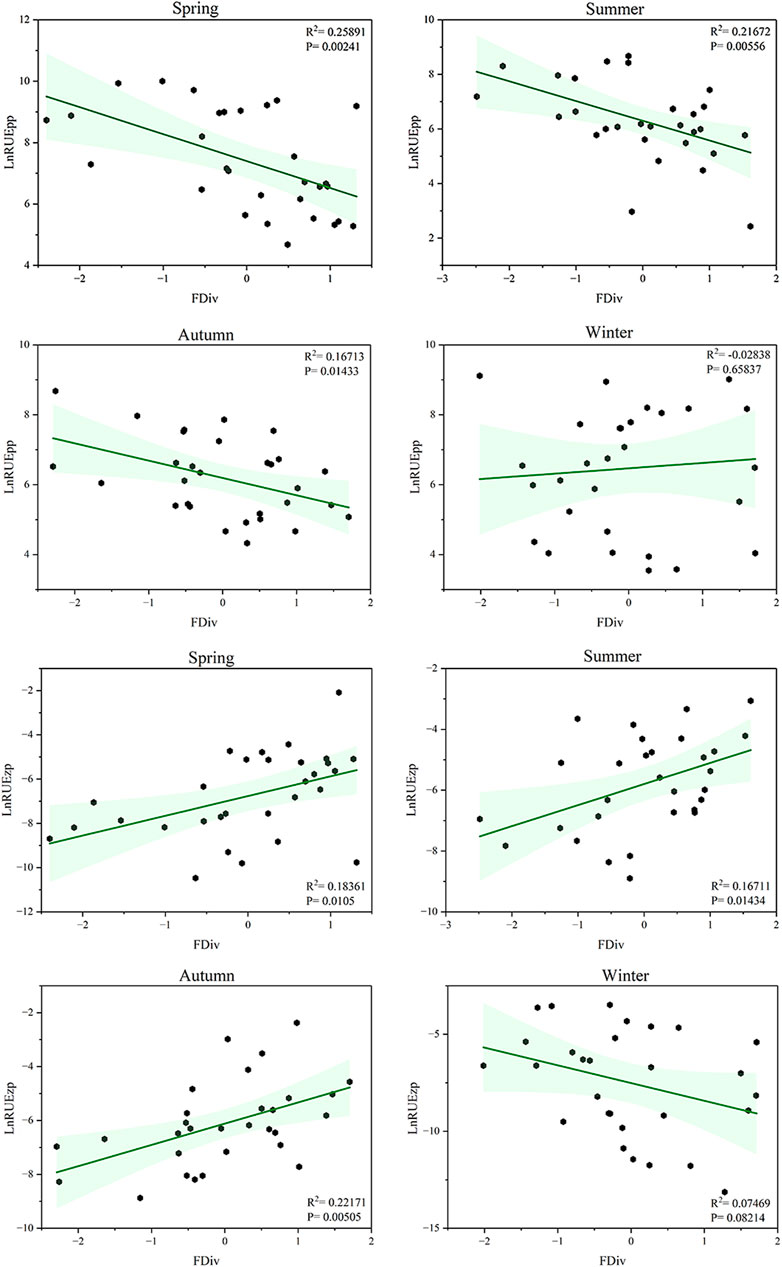
Figure 7. Relationship between functional diversity and ecosystem function in Xiaoqing River in each season. The response variables were phytoplankton resource use efficiency (RUEpp) and zooplankton resource use efficiency (RUEzp), and the explanatory variable was phytoplankton functional divergence index. Green shading represents 95% confidence intervals, and the response variables were transformed to natural logarithms before analysis.
4 Discussion
Although most BEF-related studies have focused on single sampling and single taxa, our study investigated the BEF relationship across trophic levels under different seasons. Our results demonstrated that the increase in the relative abundance of dominant species caused by Bacillariophyta blooms significantly impacted the RUEpp and RUEzp. Meanwhile, phytoplankton evenness negatively affected RUEpp but positively affected RUEzp. Similar correlation trends were also reflected in the functional divergence index. We evaluated the roles of environmental factors and biodiversity in explaining variations in RUE, among which evenness emerged as the most significant contributor.
The results of the multiple linear regression modeling showed that evenness had a significant effect in both RUEpp and RUEzp models and was the main factor explaining the variation. This is in agreement with Hodapp et al. (2015) who found that evenness was a key driver of phytoplankton productivity and RUE compared to environmental factors such as temperature and light. In communities with lower evenness, a few dominant species have a competitive advantage and are able to use resources more efficiently due to their higher physiological or chemical adaptations (Hodapp et al., 2015). In contrast, higher phytoplankton evenness may mean that zooplankton have a more diverse food source, which not only provides richer nutrients, but also improves zooplankton growth and reproductive efficiency (Wang et al., 2024).
In the RUEpp model, NO3−-N and CODMn had positive effects on resource use efficiency, while total phosphorus (TP), evenness, functional divergence and functional evenness all had negative effects. High phosphorus levels in eutrophic waters can make nitrate a limiting factor, and as nitrate levels increase, resource use efficiency can be improved by supporting more phytoplankton growth (He et al., 2021). Meanwhile, higher CODMn values indicate the presence of more biodegradable substances in the water body, which may release additional nutrients (e.g., nitrogen and phosphorus) during degradation that can be used by phytoplankton to further improve resource use efficiency (Reinl et al., 2022). The possible reason for the negative correlation of functional divergence is that under eutrophication conditions, functional convergence of algae reduces ecological niche occupancy and ecosystem resources cannot be fully utilized (Zhang et al., 2019; Dunck et al., 2019). In contrast, in the RUEzp model, NO3−-N had a significant negative effect on RUEzp, except for phytoplankton evenness, which had a significant positive effect. We hypothesize that the possible reason is that elevated nitrate-nitrogen concentrations usually contribute to the occurrence of algal blooms, in which some harmful algal species bloom and may not be consumed by zooplankton. In addition, algal blooms can cause rapid changes in water quality (e.g., oxygen depletion and toxin release) that stress zooplankton populations and reduce their growth and reproductive success (He et al., 2021).
Principal coordinate analysis revealed significant seasonal variations in the composition of phytoplankton and zooplankton communities. This finding indicates that the structure of riverine organisms is largely influenced by seasonal differences, such as temperature and flow. The results of the one-way ANOVA showed that RUEpp and RUEzp also exhibited seasonal differences. Among the variables, RUEpp exhibited a significantly higher value in spring than in other seasons. This indicates that phytoplankton growth activity was higher in the spring, probably due to increased light and temperature, which significantly increased resource use efficiency. Furthermore, the higher RUEzp observed in summer may be attributed to the availability of phytoplankton as a food source, which in turn facilitates the growth and reproduction of zooplankton. For the study of algal bloom conditions in the Xiaoqing River, Bacillariophyta dominated the phytoplankton community during the sampling period and formed high-density algal blooms (density >5 × 106 cells/L) several times. The strongest bloom was found in spring, which could reach up to class Ⅳ, followed by class Ⅲ in summer, and the weakest degree of bloom was found in autumn and winter. Compared with Chlorophyta and other higher aquatic plants, Bacillariophyta have a more efficient photosynthetic rate, faster exponential growth rate, and more robust salinity tolerance, which allows them to fully utilize water resources. In contrast, zooplankton feeding strategies favor algae such as Chlorophyta, which are more palatable, and avoid Bacillariophyta (Vincent and Bowler, 2020). Possible mechanisms include the release of substances by Bacillariophyta that can inhibit zooplankton predation (Malej and Harris, 1993), their hard siliceous shells, and the production of toxic aldehydes, which are also important predation avoidance mechanisms (Miralto et al., 1999). Furthermore, studies of eutrophic lakes have found that when blooms occur, RUEpp often increases while RUEzp decreases (Filstrup et al., 2014; Gao et al., 2022). If bloom algae with higher RUE and competitive advantages are well adapted to environmental conditions, they may produce greater RUEpp; simultaneously, zooplankton may reduce predation on these dominant species with lower nutrient quality (Hassett et al., 1997), thereby decreasing RUEzp.
The positive correlation between phytoplankton species richness and RUE has been widely demonstrated in the assessment of ecosystem functioning (Ptacnik et al., 2008; Striebel et al., 2009). However, evenness tends to have a better effect on ecosystem functioning than species richness, because it captures changes in the abundance of each species rather than just the loss and gain of species (Filstrup et al., 2019). Meanwhile, under dramatic environmental change, evenness can better reflect the rapid changes in the external environment, and higher evenness means that the abundance of species in the community tends to be more balanced and the whole tends to be more stable (Otero et al., 2020). Most current studies have focused more on the relationship between primary producer diversity and RUE and less on the RUE of herbivores and even higher trophic levels. Therefore, a comprehensive understanding of the effects of biodiversity requires the integration of both horizontal and vertical dimensions of biodiversity, especially when specific taxa with unique traits dominate primary producers (Duffy et al., 2007). Our results consistently showed that phytoplankton evenness is significantly correlated with RUEpp and RUEzp across seasons (although the strength of the correlation may be differ) and exhibits opposite trends, which is consistent with the findings of Filstrup et al. (2014) in eutrophic lakes. These findings can also be explained by ecological stoichiometry, i.e., when RUEpp is high, RUEzp decreases due to reduced trophic quality (Hassett et al., 1997). Notably, in contrast to other seasons, we found that the relationship between phytoplankton evenness and RUE was not significant in the autumn, speculating that this finding may be related to the large amount of tree litter input to the river from the riparian zone. As an essential source of carbon, nitrogen, and phosphorus, leaf litter can significantly increase phytoplankton biomass and chlorophyll content and promote photosynthesis (Zhang et al., 2018). However, large amounts of leaf litter can also form a cover on the water surface that reduces light transmittance, diminishes the amount of light available to phytoplankton in the water column, and reduces photosynthetic efficiency (Domingues et al., 2014). Some light-loving taxa, such as Microcystis and Scenedesmus, are reduced, while shade-tolerant species, such as Cryptomonas and Euglena, are benefitted (Reynolds et al., 2002; Padisák et al., 2009). Furthermore, leaf litter accumulation may lead to localized stagnation of the water column, affecting mobility, nutrient transport, and light distribution, disrupting vertical migration of phytoplankton, and ultimately limiting their distribution and growth (Li et al., 2013).
Individual ecology-based classification of phytoplankton functional groups can more accurately characterize habitats, reveal dynamic relationships between phytoplankton functional groups and the aquatic environment, and predict changes in ecosystem function (Abrantes et al., 2006; Borics et al., 2021). Our results are consistent with the hypothesis that FDiv has some explanatory power in predicting ecosystem functioning. Its response pattern is consistent with taxonomic diversity (evenness), i.e., FDiv has a negative correlation effect on RUEpp and a positive correlation on RUEzp, indicating that FDiv well reflects the distribution of species in the functional trait space and their effects on RUE. The higher the FDiv of phytoplankton, the weaker the competition for resources among species, the more fully utilized the ecological niche space, and the higher the primary productivity generated, so that RUEzp exhibited an upward trend (Ptacnik et al., 2010). Several studies have demonstrated that functional diversity can be a better predictor of ecosystem properties because adding or subtracting species with similar functions has little effect on ecosystem functioning (Carmona et al., 2016; Abonyi et al., 2017; Gross et al., 2017; Ye et al., 2019). Due to the heterogeneity of ecosystem habitats and resources, communities with similar functions cannot achieve maximum resource utilization. In contrast, communities with greater functional differences can improve RUE and enhance ecosystem functioning (Cadotte et al., 2011). Therefore, the functional characterization approach is considered a more promising tool in stream ecosystem biomonitoring and management (Abonyi et al., 2017; Breton et al., 2017; Leruste et al., 2018). The present study demonstrated that both taxonomic and functional diversity were significantly correlated with ecosystem functioning. However, the model fit for taxonomic diversity was slightly better than that for functional diversity. This observation contradicts the results of previous studies, and this discrepancy may stem from the fact that our ability to quantify traits remains limited, and the trait profiles covered need to adequately capture slight differences in species at specific ecological niches. For instance, differences in light requirements, which may be a key trait in determining species’ efficient use of heterogeneous resources within the environment, may need to be better captured, thus reducing the percentage of variance explained by functional diversity in RUE. Furthermore, trait-mediated effects due to interactions among taxa must be considered, which may limit the applicability of currently used traits (Klais et al., 2017).
Our study supports a bottom-up ecosystem regulatory mechanism in which resource use drives competition among species at the primary producer level, with more competitive populations gaining a resource advantage and dominating the community, i.e., selection effects. At the herbivore level, resource heterogeneity and differences in food selectivity drive changes in trophic transfer efficiency (Filstrup et al., 2019), and such interactions across trophic levels form a more complex and rich BEF relationship. In this study, only two groups of organisms were involved, and we should consider expanding the scope of the study based on field observations to investigate the BEF mechanism of organisms at higher trophic levels. Considering the advantages of studying BEF relationships based on functional traits, our results improve the quantitative description of phytoplankton functional traits in subsequent studies. This study contributes to a more comprehensive identification of various ecologically relevant traits for a more comprehensive assessment of river ecosystem functioning (Abonyi et al., 2017; Kremer et al., 2017). Furthermore, the findings of this study will provide theoretical guidance for the protection and management of eutrophic waters. Specifically, we propose the implementation of measures to control nutrient inputs, maintain the structural stability of phytoplankton communities, and strengthen ecosystem monitoring and assessment. These measures aim to slow down the eutrophication process, maintain the functional balance and diversity of primary producer communities, and thus improve the resource use efficiency and ecological service function of the whole ecosystem. These protection strategies should be further refined and a comprehensive eutrophication water management plan should be formulated and implemented with the ultimate goal of curbing eutrophication and realizing the improvement and restoration of the aquatic ecosystem.
5 Conclusion
In summary, this study has established the patterns and mechanisms between biodiversity and ecosystem function in natural phytoplankton communities under eutrophic conditions. The results verified our preliminary hypothesis that both phytoplankton evenness and FDiv significantly affect RUE and have opposite patterns at the different trophic levels. Due to the increase of dominant species caused by algal blooms, the abundance of each species tends to be unbalanced, the occupation of the trophic ecological niche decreases, and it becomes difficult to effectively transfer and utilize resources to the upper trophic level. When the dominant harmful algal blooms declined, a relative equilibrium state was reached among the species, the whole ecological niche was gradually utilized more comprehensively, and the ecosystem function was enhanced. Therefore, in order to effectively manage and protect the health of water bodies, we suggest controlling the input of nutrients and slowing down the eutrophication process; taking measures to restore and maintain an appropriate phytoplankton community structure and avoiding the absolute dominance of a single species; and strengthening the monitoring and assessment of the structural and functional indicators of phytoplankton communities and formulating corresponding protection management and strategies. This study provides important insights into the study of ecosystem functioning across trophic scales. It supports the view that increased ecological niche utilization can improve resource use efficiency, providing an important reference for subsequent studies of BEF mechanisms at higher trophic levels.
Data availability statement
The raw data supporting the conclusions of this article will be made available by the authors, without undue reservation.
Author contributions
MM: Conceptualization, Formal Analysis, Methodology, Software, Visualization, Writing–original draft, Writing–review and editing. JL: Data curation, Methodology, Software, Writing–review and editing. AL: Data curation, Methodology, Software, Writing–review and editing. PZ: Investigation, Visualization, Writing–review and editing. XY: Funding acquisition, Project administration, Supervision, Writing–review and editing.
Funding
The author(s) declare that financial support was received for the research, authorship, and/or publication of this article. This work was funded by the National Natural Science Foundation of China (41977193) and the National Science and Technology Basic Resources Survey Program of China (2019FY101700).
Conflict of interest
The authors declare that the research was conducted in the absence of any commercial or financial relationships that could be construed as a potential conflict of interest.
Publisher’s note
All claims expressed in this article are solely those of the authors and do not necessarily represent those of their affiliated organizations, or those of the publisher, the editors and the reviewers. Any product that may be evaluated in this article, or claim that may be made by its manufacturer, is not guaranteed or endorsed by the publisher.
Supplementary material
The Supplementary Material for this article can be found online at: https://www.frontiersin.org/articles/10.3389/fenvs.2024.1389220/full#supplementary-material
References
Abonyi, A., Horváth, Z., and Ptacnik, R. (2017). Functional richness outperforms taxonomic richness in predicting ecosystem functioning in natural phytoplankton communities. Freshw. Biol. 63, 178–186. doi:10.1111/fwb.13051
Abrantes, N., Antunes, S. C., Pereira, M. J., and Gonçalves, F. (2006). Seasonal succession of cladocerans and phytoplankton and their interactions in a shallow eutrophic lake (Lake Vela, Portugal). Acta Oecol. 29, 54–64. doi:10.1016/j.actao.2005.07.006
Amorim, C. A., and Moura, A. do N. (2021). Ecological impacts of freshwater algal blooms on water quality, plankton biodiversity, structure, and ecosystem functioning. Sci. Total Environ. 758, 143605. doi:10.1016/j.scitotenv.2020.143605
Barakat, A., El Baghdadi, M., Rais, J., Aghezzaf, B., and Slassi, M. (2016). Assessment of spatial and seasonal water quality variation of Oum Er Rbia River (Morocco) using multivariate statistical techniques. Int. Soil Water Conservation Res. 4, 284–292. doi:10.1016/j.iswcr.2016.11.002
Borics, G., Abonyi, A., Salmaso, N., and Ptacnik, R. (2021). Freshwater phytoplankton diversity: models, drivers and implications for ecosystem properties. Hydrobiologia 848, 53–75. doi:10.1007/s10750-020-04332-9
Breton, E., Christaki, U., Bonato, S., Didry, M., and Artigas, L. F. (2017). Functional trait variation and nitrogen use efficiency in temperate coastal phytoplankton. Mar. Ecol. Prog. Ser. 563, 35–49. doi:10.3354/meps11974
Bužančić, M., Ninčević Gladan, Ž., Marasović, I., Kušpilić, G., and Grbec, B. (2016). Eutrophication influence on phytoplankton community composition in three bays on the eastern Adriatic coast. Oceanologia 58, 302–316. doi:10.1016/j.oceano.2016.05.003
Cadotte, M. W., Carscadden, K., and Mirotchnick, N. (2011). Beyond species: functional diversity and the maintenance of ecological processes and services. J. Appl. Ecol. 48, 1079–1087. doi:10.1111/j.1365-2664.2011.02048.x
Cardinale, B. J., Duffy, J. E., Gonzalez, A., Hooper, D. U., Perrings, C., Venail, P., et al. (2012). Biodiversity loss and its impact on humanity. Nature 486, 59–67. doi:10.1038/nature11148
Cardinale, B. J., Matulich, K. L., Hooper, D. U., Byrnes, J. E., Duffy, E., Gamfeldt, L., et al. (2011). The functional role of producer diversity in ecosystems. Am. J. Bot. 98, 572–592. doi:10.3732/ajb.1000364
Carmona, C. P., De Bello, F., Mason, N. W. H., and Lepš, J. (2016). Traits without borders: integrating functional diversity across scales. Trends Ecol. Evol. 31, 382–394. doi:10.1016/j.tree.2016.02.003
Chapin III, F. S., Zavaleta, E. S., Eviner, V. T., Naylor, R. L., Vitousek, P. M., Reynolds, H. L., et al. (2000). Consequences of changing biodiversity. Nature 405, 234–242. doi:10.1038/35012241
Chen, Y., and Gao, X. (2000). Comparison of two methods for phytoplankton chlorophyll-a concentration measurement. J. Lake Sci. 12, 185–188. doi:10.18307/2000.0215
Daam, M. A., Teixeira, H., Lillebø, A. I., and Nogueira, A. J. A. (2019). Establishing causal links between aquatic biodiversity and ecosystem functioning: status and research needs. Sci. Total Environ. 656, 1145–1156. doi:10.1016/j.scitotenv.2018.11.413
Domingues, R. B., Guerra, C. C., Barbosa, A. B., Brotas, V., and Galvão, H. M. (2014). Effects of ultraviolet radiation and CO2 increase on winter phytoplankton assemblages in a temperate coastal lagoon. J. Plankton Res. 36, 672–684. doi:10.1093/plankt/fbt135
Duffy, J. E., Cardinale, B. J., France, K. E., McIntyre, P. B., Thébault, E., and Loreau, M. (2007). The functional role of biodiversity in ecosystems: incorporating trophic complexity. Ecol. Lett. 10, 522–538. doi:10.1111/j.1461-0248.2007.01037.x
Duffy, J. E., Godwin, C. M., and Cardinale, B. J. (2017). Biodiversity effects in the wild are common and as strong as key drivers of productivity. Nature 549, 261–264. doi:10.1038/nature23886
Dunck, B., Felisberto, S. A., and de Souza Nogueira, I. (2019). Effects of freshwater eutrophication on species and functional beta diversity of periphytic algae. Hydrobiologia 837, 195–204. doi:10.1007/s10750-019-03971-x
Eisenhauer, N., Dobies, T., Cesarz, S., Hobbie, S. E., Meyer, R. J., Worm, K., et al. (2013). Plant diversity effects on soil food webs are stronger than those of elevated CO2 and N deposition in a long-term grassland experiment. Proc. Natl. Acad. Sci. 110, 6889–6894. doi:10.1073/pnas.1217382110
Eisenhauer, N., Schielzeth, H., Barnes, A. D., Barry, K. E., Bonn, A., Brose, U., et al. (2019). “Chapter One - a multitrophic perspective on biodiversity–ecosystem functioning research,” in Advances in ecological research. Editors N. Eisenhauer, D. A. Bohan, and A. J. Dumbrell (Academic Press), 1–54. doi:10.1016/bs.aecr.2019.06.001
Feio, M. J., Almeida, S. F. P., Craveiro, S. C., and Calado, A. J. (2009). A comparison between biotic indices and predictive models in stream water quality assessment based on benthic diatom communities. Ecol. Indic. 9, 497–507. doi:10.1016/j.ecolind.2008.07.001
Filstrup, C. T., Hillebrand, H., Heathcote, A. J., Harpole, W. S., and Downing, J. A. (2014). Cyanobacteria dominance influences resource use efficiency and community turnover in phytoplankton and zooplankton communities. Ecol. Lett. 17, 464–474. doi:10.1111/ele.12246
Filstrup, C. T., King, K. B. S., and McCullough, I. M. (2019). Evenness effects mask richness effects on ecosystem functioning at macro-scales in lakes. Ecol. Lett. 22, 2120–2129. doi:10.1111/ele.13407
Gao, X., Wang, W., Ndayishimiye, J. C., Govaert, L., Chen, H., Jeppesen, E., et al. (2022). Invasive and toxic cyanobacteria regulate allochthonous resource use and community niche width of reservoir zooplankton. Freshw. Biol. 67, 1344–1356. doi:10.1111/fwb.13921
Grey, J., Jones, R. I., and Sleep, D. (2000). Stable isotope analysis of the origins of zooplankton carbon in lakes of differing trophic state. Oecologia 123, 232–240. doi:10.1007/s004420051010
Griffin, J. N., Méndez, V., Johnson, A. F., Jenkins, S. R., and Foggo, A. (2009). Functional diversity predicts overyielding effect of species combination on primary productivity. Oikos 118, 37–44. doi:10.1111/j.1600-0706.2008.16960.x
Gross, N., Bagousse-Pinguet, Y. L., Liancourt, P., Berdugo, M., Gotelli, N. J., and Maestre, F. T. (2017). Functional trait diversity maximizes ecosystem multifunctionality. Nat. Ecol. Evol. 1, 0132–0139. doi:10.1038/s41559-017-0132
Hagan, J. G., Vanschoenwinkel, B., and Gamfeldt, L. (2021). We should not necessarily expect positive relationships between biodiversity and ecosystem functioning in observational field data. Ecol. Lett. 24, 2537–2548. doi:10.1111/ele.13874
Hassett, R. P., Cardinale, B., Stabler, L. B., and Elser, J. J. (1997). Ecological stoichiometry of N and P in pelagic ecosystems: comparison of lakes and oceans with emphasis on the zooplankton-phytoplankton interaction. Limnol. Oceanogr. 42, 648–662. doi:10.4319/lo.1997.42.4.0648
He, D., Zhang, K., Cui, X., Tang, J., and Sun, Y. (2018). Spatiotemporal variability of hydrocarbons in surface sediments from an intensively human-impacted Xiaoqing River-Laizhou Bay system in the eastern China: occurrence, compositional profile and source apportionment. Sci. Total Environ. 645, 1172–1182. doi:10.1016/j.scitotenv.2018.07.193
He, H., Chen, K., Du, Y., Li, K., Liu, Z., Jeppesen, E., et al. (2021). Increased nitrogen loading boosts summer phytoplankton growth by alterations in resource and zooplankton control: a mesocosm study. Front. Environ. Sci. 9. doi:10.3389/fenvs.2021.772314
He, X., Chen, G., Fang, Z., Liang, W., Li, B., Tang, J., et al. (2020). Source identification of chromium in the sediments of the Xiaoqing River and Laizhou Bay: a chromium stable isotope perspective. Environ. Pollut. 264, 114686. doi:10.1016/j.envpol.2020.114686
Hillebrand, H., Bennett, D. M., and Cadotte, M. W. (2008). Consequences of dominance: a review of evenness effects on local and regional ecosystem processes. Ecology 89, 1510–1520. doi:10.1890/07-1053.1
Hodapp, D., Hillebrand, H., and Striebel, M. (2019). “Unifying” the concept of resource use efficiency in ecology. Front. Ecol. Evol. 6, 233. doi:10.3389/fevo.2018.00233
Hodapp, D., Meier, S., Muijsers, F., Badewien, T. H., and Hillebrand, H. (2015). Structural equation modeling approach to the diversity-productivity relationship of Wadden Sea phytoplankton. Mar. Ecol. Prog. Ser. 523, 31–40. doi:10.3354/meps11153
Hu, H., and Wei, Y. (2006). The Freshwater Algae of China Systematics, Taxonomy and Ecology. Beijing, China: Science Press. Available at: http://ir.ihb.ac.cn/handle/342005/10582.
Huisman, J., Codd, G. A., Paerl, H. W., Ibelings, B. W., Verspagen, J. M. H., and Visser, P. M. (2018). Cyanobacterial blooms. Nat. Rev. Microbiol. 16, 471–483. doi:10.1038/s41579-018-0040-1
Jiang, T., Skyllberg, U., Björn, E., Green, N. W., Tang, J., Wang, D., et al. (2017). Characteristics of dissolved organic matter (DOM) and relationship with dissolved mercury in Xiaoqing River-Laizhou Bay estuary, Bohai Sea, China. Environ. Pollut. 223, 19–30. doi:10.1016/j.envpol.2016.12.006
Jiang, X. (1979). Zoology of China. Arthropoda. Crustacea. Freshwater Cladocera. Beijing, China: Science Press.
Johnson, J. W., and Lebreton, J. M. (2004). History and use of relative importance indices in organizational research. Organ. Res. Methods 7, 238–257. doi:10.1177/1094428104266510
Klais, R., Norros, V., Lehtinen, S., Tamminen, T., and Olli, K. (2017). Community assembly and drivers of phytoplankton functional structure. Funct. Ecol. 31, 760–767. doi:10.1111/1365-2435.12784
Kremer, C. T., Williams, A. K., Finiguerra, M., Fong, A. A., Kellerman, A., Paver, S. F., et al. (2017). Realizing the potential of trait-based aquatic ecology: new tools and collaborative approaches. Limnol. Oceanogr. 62, 253–271. doi:10.1002/lno.10392
Leruste, A., Villéger, S., Malet, N., De Wit, R., and Bec, B. (2018). Complementarity of the multidimensional functional and the taxonomic approaches to study phytoplankton communities in three Mediterranean coastal lagoons of different trophic status. Hydrobiologia 815, 207–227. doi:10.1007/s10750-018-3565-4
Li, F., Altermatt, F., Yang, J., An, S., Li, A., and Zhang, X. (2020). Human activities’ fingerprint on multitrophic biodiversity and ecosystem functions across a major river catchment in China. Glob. Change Biol. 26, 6867–6879. doi:10.1111/gcb.15357
Li, F., Zhang, H., Zhu, Y., Xiao, Y., and Chen, L. (2013). Effect of flow velocity on phytoplankton biomass and composition in a freshwater lake. Sci. Total Environ. 447, 64–71. doi:10.1016/j.scitotenv.2012.12.066
Li, F., Zhang, Y., Altermatt, F., and Zhang, X. (2021). Consideration of multitrophic biodiversity and ecosystem functions improves indices on river ecological status. Environ. Sci. Technol. 55, 16434–16444. doi:10.1021/acs.est.1c05899
Litchman, E., and Klausmeier, C. (2008). Trait-based community ecology of phytoplankton. Annu. Rev. Ecol. Evol. Syst. 39, 615–639. doi:10.1146/annurev.ecolsys.39.110707.173549
Liu, Y., Cao, S., Li, X., Li, F., and Zhang, X. (2019). Combined effect of climate change and human activities on the hydrological processes of an urban river: case study of the Xiaoqing River, China. Hydrological Sci. J. 64, 2105–2114. doi:10.1080/02626667.2019.1618463
Lu, Q., Liu, X., Qiu, X., Liang, T., Chen, J., Zhao, S., et al. (2021). Changes and drivers of zooplankton diversity patterns in the middle reach of Yangtze River floodplain lakes, China. Ecol. Evol. 11, 17885–17900. doi:10.1002/ece3.8353
Malej, A., and Harris, R. P. (1993). Inhibition of copepod grazing by diatom exudates: a factor in the development of mucus aggregates? Mar. Ecol. Prog. Ser. 96, 33–42. doi:10.3354/meps096033
McQuatters-Gollop, A., Gilbert, A. J., Mee, L. D., Vermaat, J. E., Artioli, Y., Humborg, C., et al. (2009). How well do ecosystem indicators communicate the effects of anthropogenic eutrophication? Estuar. Coast. Shelf Sci. 82, 583–596. doi:10.1016/j.ecss.2009.02.017
Miralto, A., Barone, G., Romano, G., Poulet, S. A., Ianora, A., Russo, G. L., et al. (1999). The insidious effect of diatoms on copepod reproduction. Nature 402, 173–176. doi:10.1038/46023
Mitchell, M. G. E., Qiu, J., Cardinale, B. J., Chan, K. M. A., Eigenbrod, F., Felipe-Lucia, M. R., et al. (2024). Key questions for understanding drivers of biodiversity-ecosystem service relationships across spatial scales. Landsc. Ecol. 39, 36–17. doi:10.1007/s10980-024-01842-y
Moi, D. A., Lansac-Tôha, F. M., Romero, G. Q., Sobral-Souza, T., Cardinale, B. J., Kratina, P., et al. (2022). Human pressure drives biodiversity–multifunctionality relationships in large Neotropical wetlands. Nat. Ecol. Evol. 6, 1279–1289. doi:10.1038/s41559-022-01827-7
Moi, D. A., Romero, G. Q., Antiqueira, P. A. P., Mormul, R. P., Teixeira de Mello, F., and Bonecker, C. C. (2021). Multitrophic richness enhances ecosystem multifunctionality of tropical shallow lakes. Funct. Ecol. 35, 942–954. doi:10.1111/1365-2435.13758
Mustapha, A., Aris, A. Z., Juahir, H., Ramli, M. F., and Kura, N. U. (2013). River water quality assessment using environmentric techniques: case study of Jakara River Basin. Environ. Sci. Pollut. Res. 20, 5630–5644. doi:10.1007/s11356-013-1542-z
Naeem, S., Duffy, J. E., and Zavaleta, E. (2012). The functions of biological diversity in an age of extinction. Science 336, 1401–1406. doi:10.1126/science.1215855
Newbold, T., Hudson, L. N., Hill, S. L. L., Contu, S., Lysenko, I., Senior, R. A., et al. (2015). Global effects of land use on local terrestrial biodiversity. Nature 520, 45–50. doi:10.1038/nature14324
Oliver, T. H., Isaac, N. J. B., August, T. A., Woodcock, B. A., Roy, D. B., and Bullock, J. M. (2015). Declining resilience of ecosystem functions under biodiversity loss. Nat. Commun. 6, 10122. doi:10.1038/ncomms10122
Otero, J., Álvarez-Salgado, X. A., and Bode, A. (2020). Phytoplankton diversity effect on ecosystem functioning in a coastal upwelling system. Front. Mar. Sci. 7. doi:10.3389/fmars.2020.592255
Padisák, J., Crossetti, L. O., and Naselli-Flores, L. (2009). Use and misuse in the application of the phytoplankton functional classification: a critical review with updates. Hydrobiologia 621, 1–19. doi:10.1007/s10750-008-9645-0
Polazzo, F., dos Anjos, T. B. O., Arenas-Sánchez, A., Romo, S., Vighi, M., and Rico, A. (2022). Effect of multiple agricultural stressors on freshwater ecosystems: the role of community structure, trophic status, and biodiversity-functioning relationships on ecosystem responses. Sci. Total Environ. 807, 151052. doi:10.1016/j.scitotenv.2021.151052
Ptacnik, R., Moorthi, S. D., and Hillebrand, H. (2010). “Chapter 1 - hutchinson reversed, or why there need to Be so many species,” in Advances in ecological research. Editor G. Woodward (Academic Press), 1–43. doi:10.1016/B978-0-12-385005-8.00001-0
Ptacnik, R., Solimini, A. G., Andersen, T., Tamminen, T., Brettum, P., Lepistö, L., et al. (2008). Diversity predicts stability and resource use efficiency in natural phytoplankton communities. Proc. Natl. Acad. Sci. 105, 5134–5138. doi:10.1073/pnas.0708328105
Reinl, K. L., Harris, T. D., Elfferich, I., Coker, A., Zhan, Q., De Senerpont Domis, L. N., et al. (2022). The role of organic nutrients in structuring freshwater phytoplankton communities in a rapidly changing world. Water Res. 219, 118573. doi:10.1016/j.watres.2022.118573
Reynolds, C. S., Huszar, V., Kruk, C., Naselli-Flores, L., and Melo, S. (2002). Towards a functional classification of the freshwater phytoplankton. J. Plankton Res. 24, 417–428. doi:10.1093/plankt/24.5.417
Rosset, V., Angélibert, S., Arthaud, F., Bornette, G., Robin, J., Wezel, A., et al. (2014). Is eutrophication really a major impairment for small waterbody biodiversity? J. Appl. Ecol. 51, 415–425. doi:10.1111/1365-2664.12201
Schuldt, A., Assmann, T., Brezzi, M., Buscot, F., Eichenberg, D., Gutknecht, J., et al. (2018). Biodiversity across trophic levels drives multifunctionality in highly diverse forests. Nat. Commun. 9, 2989. doi:10.1038/s41467-018-05421-z
Slade, E. M., Kirwan, L., Bell, T., Philipson, C. D., Lewis, O. T., and Roslin, T. (2017). The importance of species identity and interactions for multifunctionality depends on how ecosystem functions are valued. Ecology 98, 2626–2639. doi:10.1002/ecy.1954
Soliveres, S., van der Plas, F., Manning, P., Prati, D., Gossner, M. M., Renner, S. C., et al. (2016). Biodiversity at multiple trophic levels is needed for ecosystem multifunctionality. Nature 536, 456–459. doi:10.1038/nature19092
Striebel, M., Behl, S., and Stibor, H. (2009). The coupling of biodiversity and productivity in phytoplankton communities: consequences for biomass stoichiometry. Ecology 90, 2025–2031. doi:10.1890/08-1409.1
Sun, Y., Li, H., Wang, X., Jin, Y., Nagai, S., and Lin, S. (2023). Phytoplankton and microzooplankton community structure and assembly mechanisms in northwestern pacific ocean estuaries with environmental heterogeneity and geographic segregation. Microbiol. Spectr. 11, e0492622. doi:10.1128/spectrum.04926-22
Thompson, P. L., Rayfield, B., and Gonzalez, A. (2017). Loss of habitat and connectivity erodes species diversity, ecosystem functioning, and stability in metacommunity networks. Ecography 40, 98–108. doi:10.1111/ecog.02558
Tian, W., Zhang, H., Zhang, J., Zhao, L., Miao, M., and Huang, H. (2017). Biodiversity effects on resource use efficiency and community turnover of plankton in Lake Nansihu, China. Environ. Sci. Pollut. Res. 24, 11279–11288. doi:10.1007/s11356-017-8758-2
Tilman, D., Isbell, F., and Cowles, J. M. (2014). Biodiversity and ecosystem functioning. Annu. Rev. Ecol. Evol. Syst. 45, 471–493. doi:10.1146/annurev-ecolsys-120213-091917
Varol, M. (2019). Phytoplankton functional groups in a monomictic reservoir: seasonal succession, ecological preferences, and relationships with environmental variables. Environ. Sci. Pollut. Res. 26, 20439–20453. doi:10.1007/s11356-019-05354-0
Vaughn, C. C. (2010). Biodiversity losses and ecosystem function in freshwaters: emerging conclusions and research directions. BioScience 60, 25–35. doi:10.1525/bio.2010.60.1.7
Villéger, S., Mason, N. W. H., and Mouillot, D. (2008). New multidimensional functional diversity indices for a multifaceted framework in functional ecology. Ecology 89, 2290–2301. doi:10.1890/07-1206.1
Vincent, F., and Bowler, C. (2020). Diatoms are selective segregators in global ocean planktonic communities. mSystems 5, e00444. doi:10.1128/msystems.00444-19
Wang, B., and Yin, X. (2023). Homogenization of functional diversity of rotifer communities in relation to eutrophication in an urban river of north China. Biology 12, 1488. doi:10.3390/biology12121488
Wang, H., García Molinos, J., Heino, J., Zhang, H., Zhang, P., and Xu, J. (2021a). Eutrophication causes invertebrate biodiversity loss and decreases cross-taxon congruence across anthropogenically-disturbed lakes. Environ. Int. 153, 106494. doi:10.1016/j.envint.2021.106494
Wang, H., Zhang, X., Shan, H., Chaochao, L. V., Ren, W., Wen, Z., et al. (2022). Biodiversity buffers the impact of eutrophication on ecosystem functioning of submerged macrophytes on the Yunnan-Guizhou Plateau, Southwest China. Environ. Pollut. 314, 120210. doi:10.1016/j.envpol.2022.120210
Wang, J., Soininen, J., and Heino, J. (2021b). Ecological indicators for aquatic biodiversity, ecosystem functions, human activities and climate change. Ecol. Indic. 132, 108250. doi:10.1016/j.ecolind.2021.108250
Wang, L., Liu, J., Bao, Z., Ma, X., Shen, H., Chen, J., et al. (2024). Predictable shifts in diversity and ecosystem function in phytoplankton and zooplankton communities along thermocline stratification intensity continua. Sci. Total Environ. 912, 168981. doi:10.1016/j.scitotenv.2023.168981
Wang, Y. (2020). Atlas of common aquatic organisms in Chinese watersheds. Beijing, China: Science Press.
Weijters, M. J., Janse, J. H., Alkemade, R., and Verhoeven, J. T. A. (2009). Quantifying the effect of catchment land use and water nutrient concentrations on freshwater river and stream biodiversity. Aquatic Conservation Mar. Freshw. Ecosyst. 19, 104–112. doi:10.1002/aqc.989
Wilsey, B. J., and Potvin, C. (2000). Biodiversity and ecosystem functioning: importance of species evenness in an old field. Ecology 81, 887–892. doi:10.1890/0012-9658(2000)081[0887:BAEFIO]2.0.CO;2
Wojciechowski, J., Heino, J., Bini, L. M., and Padial, A. A. (2017). Temporal variation in phytoplankton beta diversity patterns and metacommunity structures across subtropical reservoirs. Freshw. Biol. 62, 751–766. doi:10.1111/fwb.12899
Yang, Y., Chen, H., Abdullah Al, M., Ndayishimiye, J. C., Yang, J. R., Isabwe, A., et al. (2022). Urbanization reduces resource use efficiency of phytoplankton community by altering the environment and decreasing biodiversity. J. Environ. Sci. 112, 140–151. doi:10.1016/j.jes.2021.05.001
Ye, L., Chang, C.-W., Matsuzaki, S. S., Takamura, N., Widdicombe, C. E., and Hsieh, C. (2019). Functional diversity promotes phytoplankton resource use efficiency. J. Ecol. 107, 2353–2363. doi:10.1111/1365-2745.13192
Yi, Z.-F., Cannon, C. H., Chen, J., Ye, C.-X., and Swetnam, R. D. (2014). Developing indicators of economic value and biodiversity loss for rubber plantations in Xishuangbanna, southwest China: a case study from Menglun township. Ecol. Indic. 36, 788–797. doi:10.1016/j.ecolind.2013.03.016
Zhang, F., Fu, H., Lou, H., Sun, X., Zhang, D., Sun, P., et al. (2023). Assessment of eutrophication from Xiaoqing River estuary to Laizhou Bay: further warning of ecosystem degradation in typically polluted estuary. Mar. Pollut. Bull. 193, 115209. doi:10.1016/j.marpolbul.2023.115209
Zhang, G., Yu, X., Liu, Y., Zhang, H., Zhang, Q., Li, Y., et al. (2018). Accumulation effect of litter decomposition and water level on carbon and nitrogen in shallow lake water of Lake Poyang. J. Lake Sci. 30, 668–679. doi:10.18307/2018.0309
Zhang, M., Shi, X., Chen, F., Yang, Z., and Yu, Y. (2021). The underlying causes and effects of phytoplankton seasonal turnover on resource use efficiency in freshwater lakes. Ecol. Evol. 11, 8897–8909. doi:10.1002/ece3.7724
Zhang, Q., Liu, Y.-P., Luo, F.-L., Dong, B.-C., and Yu, F.-H. (2019a). Does species richness affect the growth and water quality of submerged macrophyte assemblages? Aquat. Bot. 153, 51–57. doi:10.1016/j.aquabot.2018.11.006
Zhang, S., Xu, H., Zhang, Y., Li, Y., Wei, J., and Pei, H. (2020). Variation of phytoplankton communities and their driving factors along a disturbed temperate river-to-sea ecosystem. Ecol. Indic. 118, 106776. doi:10.1016/j.ecolind.2020.106776
Zhang, Y., Chen, H. Y. H., and Reich, P. B. (2012). Forest productivity increases with evenness, species richness and trait variation: a global meta-analysis. J. Ecol. 100, 742–749. doi:10.1111/j.1365-2745.2011.01944.x
Zhang, Y., Cheng, L., Li, K., Zhang, L., Cai, Y., Wang, X., et al. (2019b). Nutrient enrichment homogenizes taxonomic and functional diversity of benthic macroinvertebrate assemblages in shallow lakes. Limnol. Oceanogr. 64, 1047–1058. doi:10.1002/lno.11096
Zhao, X., Ren, B., Li, D., Xiang, Z., Garber, P. A., and Li, M. (2019). Effects of habitat fragmentation and human disturbance on the population dynamics of the Yunnan snub-nosed monkey from 1994 to 2016. PeerJ 7, e6633. doi:10.7717/peerj.6633
Zhou, Q., Zhang, Y., Tao, J., Ye, L., Wang, H., Shan, K., et al. (2022). Water depth and land-use intensity indirectly determine phytoplankton functional diversity and further regulate resource use efficiency at a multi-lake scale. Sci. Total Environ. 834, 155303. doi:10.1016/j.scitotenv.2022.155303
Keywords: ecosystem functioning, evenness, functional traits, algae blooms, functional divergence
Citation: Ma M, Li J, Lu A, Zhu P and Yin X (2024) Effects of phytoplankton diversity on resource use efficiency in a eutrophic urban river of Northern China. Front. Environ. Sci. 12:1389220. doi: 10.3389/fenvs.2024.1389220
Received: 21 February 2024; Accepted: 13 June 2024;
Published: 04 July 2024.
Edited by:
Angela Helen Arthington, Griffith University, AustraliaReviewed by:
Ruineris Almada Cajado, Federal University of Pará, BrazilBasanta Kumar Das, Central Inland Fisheries Research Institute (ICAR), India
Jun Xu, Chinese Academy of Sciences (CAS), China
Copyright © 2024 Ma, Li, Lu, Zhu and Yin. This is an open-access article distributed under the terms of the Creative Commons Attribution License (CC BY). The use, distribution or reproduction in other forums is permitted, provided the original author(s) and the copyright owner(s) are credited and that the original publication in this journal is cited, in accordance with accepted academic practice. No use, distribution or reproduction is permitted which does not comply with these terms.
*Correspondence: Xuwang Yin, yinxuwang@dlou.edu.cn