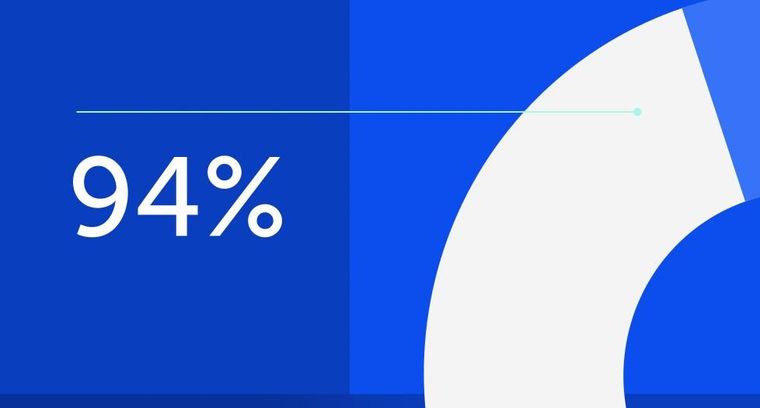
94% of researchers rate our articles as excellent or good
Learn more about the work of our research integrity team to safeguard the quality of each article we publish.
Find out more
ORIGINAL RESEARCH article
Front. Environ. Sci., 12 June 2024
Sec. Environmental Economics and Management
Volume 12 - 2024 | https://doi.org/10.3389/fenvs.2024.1385363
This article is part of the Research TopicEnergy-Environment Sustainability: Progresses and Impacts of Clean Energy System ConstructionView all 8 articles
Different from the previous studies that mainly focus on the environmental and economic benefits of the total R&D, we specifically focus on the R&D activities in the energy field, namely, energy-saving R&D in this study, and explored the spatial impacts of energy-saving R&D on urban carbon emission performance and its internal transmission mechanisms for the first time. The heterogeneity analysis from the perspectives of different-types of R&D and different urban groups were also conducted. Results indicate that: Energy-saving R&D has obvious promoting effect on the improvement of urban carbon emission performance, among which the utility-type energy-saving R&D plays a more important role than the invention-type R&D. Energy-saving R&D promotes urban carbon performance mainly through the transmission mechanism of technical effects, while structural effect and urbanization effect negatively affects carbon emission performance due to the existence of energy rebound. Furthermore, there exists significant spatial spillover effects of energy-saving R&D on urban carbon emission performance, and economic factors play a more important role in this spatial effect. In addition, the impact of energy-saving R&D on carbon emission performance has obvious urban group heterogeneity. Finally, we proposed several policy suggestions based on the main conclusions of this study.
The rising global temperature has become a major concern for human community in recent years (Bilgili et al., 2021; Kuşkaya, 2022). Carbon dioxide, as the main factor causing temperature rise, is considered the key to addressing global climate change issues by reducing its emissions. To jointly solve these problems, countries around the world made their own carbon reduction commitments at the United Nations Climate Change Conference held in 2015. As a big carbon emitter and energy consumer, China has taken active actions to reduce carbon emissions and proposed the goal of “Carbon peaking by 2030 and Carbon neutrality by 2060". The achievement of this goal requires all sectors and subjects of the economy to take effective measures to reduce carbon dioxide emissions and improve their carbon emission performance.
As an important pillar of China’s future economic growth, the low-carbon and green development of urban community is of great significance to the realization of the “double carbon” goals (Fang et al., 2023a). In light of this, increasing attentions have been given from academia to the exploration of the driving factors that contribute to improving urban carbon emission performance in recent years. A number of studies have examined the various drivers of carbon emission performance from different insights, including economic growth (Schröder and Storm, 2020; Yang and Zhao, 2023), energy efficiency (Bilgili et al., 2023; Lu et al., 2023), foreign direct investment (FDI) (Zhu et al., 2016; Song et al., 2021), internet development (Lin and Zhou, 2021; Pan et al., 2023), corporate governance (Ferrat, 2021; Lv et al., 2021), and digital economy (Zhang et al., 2022; Zhao et al., 2023). However, it is worth noting that there is a scarcity of studies that have specifically examined the potential impacts of domestic research and development (R&D) activities, particularly in the energy field, on the improvement of urban carbon emission performance. As an important driving force of the energy-saving technology progress and innovations, energy-saving R&D has an important impact on the improvement of urban carbon emission performance. Besides, a limited number of studies have examined the heterogeneous impacts of different-types of R&D activities (i.e., utility-type and invention-type) on carbon emissions performance (Lee and Min, 2015; Alam et al., 2019). Considering the purpose of the different functionally-oriented R&D activities varies from each other (CNIPA, 2020), they might produce different environmental outcomes in relation to the improvement of carbon emission performance. However, these aspects are often ignored in the existing studies. Therefore, it is necessary to systematically investigate the potential impacts of energy-saving R&D and its heterogeneity on urban carbon emission performance.
Energy-saving R&D may bring three effects on urban carbon emission performance (i.e., technical effect, structural effect, and urbanization effect) (Lee et al., 2015; Churchill et al., 2019). First, the technological progress and innovations brought about by energy-saving R&D can help to improve energy utilization efficiency of enterprises, reduce energy resource waste, and enhance the efficiency of production factors, thereby contributing to the improvement of urban carbon emission performance (Fang et al., 2024). This effect is defined as the “technical effect” of energy-saving R&D. Second, the technological innovations of energy-saving R&D facilitate the transformation of production from low value-added and heavily polluting industries to high-value-added and environmentally friendly industries, thereby reducing the share of pollution-intensive industries in the overall economic structure, which is conducive to the improvement of urban carbon emission performance (Koçak and Ulucak, 2019; Yu et al., 2022). This is defined as the “structural effect”. However, it is worth noting that due to the existence of the energy rebound effect, energy consumption and output growth of energy-intensive industries may grow faster than that of other industries, thus increasing the share of energy-intensive industries’ output in the overall economic output, which results in a reduction of urban carbon emission performance (Alam et al., 2021). Therefore, the net effect of structural effects on carbon emission performance is uncertain, and further empirical verification is needed. Third, the rigid demand for energy consumption and corresponding carbon emissions generated from the urbanization process may weaken the carbon reduction effect of energy-saving R&D (Xu et al., 2021a), this is defined as the “urbanization effect”. On the other hand, the economies of scale brought about by urbanization are conducive to promoting the carbon reduction role of energy-saving R&D (Jiao et al., 2018). Therefore, the direction of “urbanization effect” is uncertain and still needs to be examined. In summary, energy-saving R&D indirectly affects urban carbon emission performance mainly through the transmission mechanisms of technical effect, structural effect, and urbanization effect. The technical effect can help improve urban carbon emission performance, whereas the net effects of structural effect and urbanization effect are uncertain and further empirical research is needed to clarify the underlying influence mechanism.
Accordingly, to fill the above research gaps, we systematically investigated the impacts of energy-saving R&D on urban carbon emission performance based on a panel dataset of 218 prefecture-level cities in China. The main contributions of this study are as follows. Firstly, different from the previous studies that mainly focus on the total R&D (Churchill et al., 2019; Petrović and Lobanov, 2020), we specifically focus on the R&D activities in the energy field, namely, energy-saving R&D, and explored the potential impacts of energy-saving R&D on urban carbon emission performance and its internal transmission mechanisms for the first time. This is of great significance in accurately identifying the specific impacts of R&D activities on carbon emission performance, and offers new insights into providing more targeted policy recommendations to strengthen the carbon reduction role of energy-saving R&D. Secondly, we creatively examined the heterogeneous impacts of the different functional-oriented R&D (i.e., utility-type and invention-type) on urban carbon emission performance, rather than taking R&D as uniform (Lee and Min, 2015; Alam et al., 2021). This is much more helpful for better understanding the actual impacts of energy-saving R&D on carbon emission performance, and provides practical references for decision-makers to formulate insightful schemes to improve urban carbon emission performance from the perspectives of energy-saving R&D. To fill this research gap, we further explored the spatial effects of energy-saving R&D on urban carbon emission performance for the first time by constructing the spatial weight matrices of geographic and economic. This not only can provide new insights into improving carbon emission performance from the aspects of the spillover effects of energy-saving R&D, but also can help government officials and policymakers formulate targeted strategies and policies to enhance the role of energy-saving R&D in carbon reduction.
The remaining part is organized as follows. Section 2 provides the literature review on the driving factors of carbon emission performance and energy-saving R&D. Section 3 introduces the econometric model and data sources. In section 4, we carry out the benchmark estimation and robustness check. Section 5 displays the mechanism analysis. In section 6, we further discuss the spatial impacts and urban group heterogeneity of energy-saving R&D on carbon emission performance. Last section provides the main conclusions of this study and corresponding policy suggestions. The specific research framework of this study is shown in Figure 1.
As the global climate continues to warm, the imperative to reduce CO2 emissions, the leading cause of climate change, has become a pressing concern on a global scale (Fang et al., 2023b; Magazzino et al., 2023). In light of this, improving carbon emission performance has become an important task for local governments and enterprises in countries worldwide. In response, the exploration on the influencing factors of carbon emission performance has attracted increasing attentions from academia in recent years. A number of meaningful studies have examined the drivers of carbon emission performance from different insights, including new-type urbanization (Cui and Cao, 2023; Zhang et al., 2023), energy efficiency (Li et al., 2022; Bilgili et al., 2023), internet development (Lin and Zhou, 2021), foreign direct investment (FDI) (Song et al., 2021; Yue et al., 2023), development zones (Chang et al., 2020; Gao et al., 2023), and corporate governance (Narsa Goud, 2022; Oyewo, 2023). To be specific, on the basis of a panel data from 283 Chinese cities over 2006–2019, Chen et al. (2023) investigated the impact of new urbanization on the carbon emission performance and found that new-type urbanization contributes to the improvement of urban carbon emission performance, and this effect is more powerful in the later stage of new-type urbanization construction. The study indicates that new-type urbanization impacts carbon emission performance mainly through the transmission mechanism of economic agglomeration effect, government leading effect and resource allocation effect. Research results of Cui and Cao (2023) and Zhang et al. (2023) also came to similar conclusions by studying the panel data of prefecture-level cities in China.
Besides, scholars also examined the influences of development zones on carbon emission performance. For example, based on a panel dataset of 182 Chinese prefecture-level cities from 1997 to 2017, Gao et al. (2023) investigated the impact of development zones on carbon emission performance. Their research results show that the establishment of development zones has a positive impact on carbon emission performance, and this effect is more significant in the eastern large cities or coastal cities due to the mature economic foundation and preferably business environment of these cities. Similarly, Chang et al. (2020) also discovered a positive effect from development zone to urban carbon emission performance in the Bohai Rim Economic Circle. Moreover, with the rapid development of the Internet, the impact of the Internet development on carbon emission performance has also attracted increasing attention from the scholars. For instance, by utilizing a two-way fixed-effect approach, Lin and Zhou (2021) investigated the impacts of Internet development on carbon emission performance based on a panel data of China over 2006–2017. Their findings revealed that Internet development plays an active role in enhancing carbon emission performance, primarily by facilitating the upgrade of industrial structure and the diffusion of technologies, and highlighted that a market-oriented development is conducive to strengthening the positive role of the Internet on carbon emission performance improvement. Research results of Kou and Xu (2022) and Zhang et al. (2022) also supported this conclusion. Furthermore, some studies have also focused on the impact of foreign direct investment (FDI) on carbon emission performance. Specifically, Song et al. (2021) investigated the impacts of FDI on carbon emission performance based on a panel data of China from 2007 to 2018 and found that there exists opposing two-sided effects between them. Interestingly, during the sample period, promoting effect (0.1065) was greater than the inhibiting effect (0.0402), resulting in an overall positive promoting effect on carbon emission performance.
In addition, scholars also discussed the impact of corporate governance on carbon emission performance. For example, based on the data of 336 multinational enterprises in 32 countries, Oyewo (2023) explored the potential impact of corporate governance on carbon emission performance. His research results indicate that board diversity, CEO duality, and ESG committees negatively affect carbon emission performance, whereas board independence and ESG-based compensation have significant positive impacts. Narsa Goud (2022) came to similar conclusions by studying the 220 non-financial listed companies in India. Besides, an increasing number of studies also investigated the impacts of economic growth (Wang et al., 2019; Ding et al., 2020), industrial structure (Abudureheman et al., 2023b; Honma et al., 2023), population density (Hong et al., 2022), digital economy (Wu et al., 2021; Zhao et al., 2023), carbon market (Torres and Pinho, 2011; Zhou et al., 2022) and green innovations (Xu et al., 2021b; Chen et al., 2021) on carbon emission performance, however, it is worth noting that very few studies have paid attention to the potential impacts of energy-saving R&D, as a main source of energy technology progress, on urban carbon emission performance and its internal transmission mechanism, especially for the case of China.
As the main source of technological progress, R&D activities have a significant impact on both the economy and the environment (Acheampong and Boateng, 2019; Huang et al., 2020). Most of the previous studies have primarily focused on the environmental and economic benefits of the total R&D (Lee and Min, 2015; Jiao et al., 2018; Huang and Chen, 2020; Shahbaz et al., 2020). For example, based on a panel dataset of 30 OECD countries, Alam et al. (2020) explored the impacts of R&D investment on clean-energy consumption and found that R&D activities play a significant role in promoting the clean energy consumption and helps to reduce CO2 emissions. By applying a non-parametric model, Awaworyi Churchill et al. (2019) investigated the potential impacts of R&D intensity on CO2 emissions in G7 countries. Their findings reveal that there exists a negative nexus between R&D and CO2 emissions and highlighted that enhanced cooperation among countries is beneficial for promoting R&D’s effectiveness in mitigating climate change. Moreover, by introducing the total R&D intensity as an important control variable into the multi-objective optimization model, He et al. (2021) carried out a simulation prediction on the impact of R&D intensity on energy conservation and emission reduction in China’s future industrial sector. His research shows that under the optimal scenario, the R&D intensity of China’s industrial sector will increase by an average annual rate of 11.14% during the 2017–2030, and the resulting decline in energy intensity will reach 0.98 tons/million yuan in 2030, a decrease of 78.4% compared with 2005, emphasizing the importance of strengthening R&D intensity to reduce energy intensity and improve energy efficiency. Although these studies provide a general understanding of the environmental impacts of total R&D activities, however, they take R&D activities as uniform and few of them have tried to narrow the total R&D to a more specific area, which results in a limited and vague understanding of the environmental innovation outcomes of the R&D. Different from these studies, we specifically focus on the R&D activities in the energy field—energy-saving R&D, rather than treating it uniform, which is of great significance in accurately identifying the specific impacts of R&D on carbon emission performance and provide more targeted policy implications to strengthen the carbon reduction role of R&D.
In this study, we classify the total R&D into mainly two-types (i.e., invention-type and utility-type) according to the different purposes of the R&D activities (CNIPA, 2020). The invention-type R&D mainly comprises the intellectual property, such as the proposal of an innovative technique for products, methods, or their improvements; While the utility-type R&D mainly includes the new technical solutions proposed for the shape, structure, or combination of products that are suitable for practical use (CNIPA, 2020). As the utility-type R&D functionally oriented and aimed at solving practical problems, there should be more commercial use of it. Considering the different purposes within the invention-type and utility-type R&D activities, the impacts of these two types of energy-saving R&D on carbon emission performance may be heterogeneous, however, this heterogeneity often be ignored in the previous studies. For this reason, we propose the following hypothesis:
H1. Utility-type energy-saving R&D plays a more important role in improving carbon emission performance than the invention-type energy-saving R&D.
Furthermore, the progress of energy technologies brought about by energy-saving R&D in the region may also exert a positive effect to the improvement of energy technologies in the surrounding areas through inter-regional industrial cooperation, talent flow and technical exchanges, thereby indirectly affecting the carbon emission performance of neighboring areas. From this perspective, it can be assumed that energy-saving R&D may have a spatial impact on regional carbon emission performance. However, to the best of our knowledge, there has been no studies have explored the spatial impacts of energy-saving R&D on carbon emission performance to date. Accordingly, to fill this research gap, this study tries to examine the spatial impacts of energy-saving R&D on carbon emission performance for the first time by proposing the following hypothesis:
H2. Energy-saving R&D has a spatial impact on the improvement of urban carbon emission performance.
To effectively evaluate the potential impacts of the energy-saving R&D on urban carbon emission performance, this study establishes the benchmark model as follows in Eq. 1. Considering the natural logarithm processing can effectively solve the heteroscedasticity problem, we perform natural logarithm of each variable for the benchmark model:
Where i and t represent the city and year, respectively; CEP represents the core explained variable—urban carbon emission performance; ERD represents the key explanatory variable—energy-saving R&D; The remaining variables are control variables that may affect urban carbon emission performance, including economic growth (EG), energy consumption structure (ECS), industrial structure (INS), urbanization (URB), and foreign direct investment (FDI).
Considering the strong continuity of the environmentally-relevant variables in the time dimension, we further constructed the following dynamic econometric model:
Where,
In Section 2.2, we classified the total energy-saving R&D into two types: invention-type and utility-type according to the different purposes of R&D activities. To examine their different impacts on carbon emission performance (i.e., Hypothesis 1), we perform the following econometric model:
Where, IERD indicates invention-type energy-saving R&D, and UERD indicates utility-type energy-saving R&D. The interpretation of other variables is same as Eq. 1.
In addition, to further examine whether there exists a complementary effect between these two types of energy-saving R&D, we added their interaction terms (i.e., lnIERD * lnUERD) to the model as follows:
Where,
This study explores the potential impacts of energy-saving R&D on urban carbon emission performance based on a panel dataset from 218 prefecture level cities in China over the period 2007–2019. We calculated the carbon emission performance of each city by using the Super-Slacks Based Measure (Super-SBM). Compared with the traditional data envelopment method (DEA), the Super-SBM method has obvious advantages in solving the problem of input and output slackness by introducing undesirable outputs and modified relaxation variables (Iftikhar et al., 2018; Lin and Zhu, 2021). Furthermore, it also can effectively evaluate the factor efficiency in the presence of unexpected outputs (Li and Shi, 2014; Abudureheman et al., 2023a). Therefore, this study employs the Super-SBM method to measure the carbon emission performance of each city. The main input variables are including capital, labor, and energy consumption. The actual GDP and carbon emissions represent the expected and unexpected outputs, respectively. The specific expression of the Super-SBM model is as follows:
Where, j represents each decision-making unit (DMU), n is the number of DMU; m, q1, q2 denote the input variable, expected and unexpected output respectively.
Regarding the key explanatory variable—energy-saving R&D, as the invention of new technologies is a challenging and unpredictable process, some energy-saving R&D investments may not necessarily lead to the successful generation of new energy-saving technologies (Bye and Jacobsen, 2011; Huang et al., 2020), and energy-saving R&D investment data is difficult to obtain (Chen et al., 2022), therefore, this study uses the number of energy-saving patents to represent the innovative output of energy-saving R&D activities. Patent data is sourced from the National Patent Database (CNIPA, 2020). According to China’s patent law, patents are divided into three types: invention-type, utility-type, and design-type patents (CNIPA, 2020). The invention-type mainly refers to the intellectual property, such as the proposal of an innovative technique for products, methods, or their improvements; While the utility-type mainly includes the new technical solutions proposed for the shape, structure, or combination of products that are suitable for practical use. The design-type is mainly aimed at protecting the appearance of the products, therefore, design patents are not considered in this study. Based on this, the energy-saving patents in this study includes mainly two types: invention-type and utility-type.
In addition, to ensure the validity and reliability of the estimation results, we also selected some control variables which may affect urban carbon emission performance including economic growth, industrial structure, energy consumption structure, foreign direct investment and urbanization level. Economic growth (GDP) is represented by the GDP of each city. Industrial structure (INS) is denoted by the proportion of the added value of the secondary industry to GDP. As for the energy consumption structure (ECS), due to the lack of renewable energy consumption data at the urban level, this study uses the proportion of electricity consumption to total energy consumption to represent the energy consumption structure of each city. Foreign direct investment (FDI) is expressed by the proportion of the total output value of foreign-invested enterprises to GDP. In addition, we proxied the urbanization level of each city by using the urban nighttime lighting data released by the National Geophysical Data Center (NGDC, 2020). The data for the other related variables mentioned above are all sourced from the China Urban Statistical Yearbook (CUSY, 2020) and the statistical yearbooks of each city (CSY, 2020). Table 1 reports the descriptive statistics of the above variables.
Before the benchmark regression, the stationarity and cross-sectional correlation of the variables should be tested to ensure the validity and reliability of the estimated results. For this purpose, this study employs the Breusch-Pagan LM test (Breusch and Pagan, 1980), Pesaran C-D test (Pesaran, 2004) and Friedman test (Friedman, 1937) to investigate the cross-sectional correlation between different units of the sample data. The corresponding results are reported in Table 2, from which we can see that all the three tests have rejected the null hypothesis of no cross-sectional correlation at the 1% significance level, implying a significant correlation among the cross-sectional units in the sample panel data. Therefore, it is necessary to incorporate this correlation between cross-sectional units in the subsequent empirical estimation.
Moreover, we further carry out the unit root test for each variable by employing the Pesaran CADF & CIPS test (Pesaran, 2007) to keep the validity of the estimation results. Table 3 shows the corresponding results. We can see from Table 3 that only some variables are significant at the original level, therefore, a first-order difference is required for each variable. It can be seen that all variables after first-order difference pass the significance test and rejected the null hypothesis of the existence of unit root, which means that the variables used in this study need to perform first-order difference.
Considering the benchmark model (i.e., Eq. 2) is a dynamic model, traditional static estimation methods such as fixed effects (FE) and feasible generalized least squares (FGLS) may lead to biased estimation results. Arellano and Bover (1995) and Blundell and Bond (2023) propose two moment estimation methods suitable for dynamic models: differential GMM and system GMM. Compared with differential GMM (DIF-GMM) estimation, the system GMM (SYS-GMM) can estimate both horizontal and difference equation at the same time, and introduce more instrumental variables into the model to effectively improve the estimation efficiency. Therefore, this study takes the SYS-GMM method as the benchmark method to estimate the Eq. 2, and the corresponding results are shown in column four of the Table 4. From Table 4, we can see that the values of AR 1) are less than 0.1, while the values of AR 2) are larger than 0.1, indicating that there is no autocorrelation in the disturbance term, that is, the estimation results meet the exogenous condition. The values of Sargan tests are greater than 0.1, which implies that the instrumental variables of SYS-GMM technique is valid. Furthermore, to ensure the credibility and robustness of the benchmark results, the estimation results of DIF-GMM, FE and FGLS are also reported in Table 4.
The SYS-GMM estimation results in Table 4 shows that, energy-saving R&D (denoted as lnERD) has significant positive impacts on urban carbon emission performance. Besides, the estimation results of DIF-GMM, FE and FGLS are also exhibited a significant positive value of energy-saving R&D on urban carbon emission performance, which further supports the robustness and credibility of SYS-GMM estimation results. The significant positive coefficients of energy-saving R&D implies that energy-saving R&D contributes significantly to the improvement of urban carbon emission performance. To be specific, a 1% increase in energy-saving R&D investment will promote the carbon emission performance of each city by 0.260%. This is because energy-saving R&D activities facilitates the progress and innovations of energy-saving technologies, improves energy utilization efficiency of enterprises, reduces production costs and energy wastes, which in turn contributes to the improvement of urban carbon emission performance.
Regarding the control variables, it can be seen that both urbanization (URB) and economic growth (GDP) have a negative impact on the urban carbon emissions performance, and both are statistically significant at 1% level. One possible reason may be that China’s economy and urbanization process are undergoing rapid growth in the past decades, while growing urban economies and urbanization process both require large amounts of energy input, and increasing energy consumption generates greenhouse gas emissions, which is not conducive to the improvement of urban carbon emission performance. The energy consumption structure (ECS) exhibits a positive impact on urban carbon emission performance at a significance level of 5%, indicating that the transformation of energy consumption structure to electricity will help to improve urban carbon emission performance. In March 2022, the National Development and Reform Commission and the National Energy Administration jointly issued the " Guiding Opinions on Further Promoting Electric Energy Substitution”, and stressed the further expansion and deepening of electricity substitution during the “14th Five-Year Plan” period, with the goal of achieving a 30% share of electricity in the structure of end-use energy consumption by 2025. In the context of a low-carbon economy, electricity has become an important direction for the transformation of terminal energy consumption structure with the advantages of environmental protection, simplicity, and high-efficiency, and this will help to improve the carbon emission performance of cities. The coefficient of industrial structure (INS) is significantly negative at the 1% statistical level, indicating that the increase in the proportion of urban secondary industry to GDP will have a negative impact on urban carbon emission performance. As an energy intensive industry, the secondary industry is the main energy consumer and source of carbon emissions. Therefore, increases in the share of secondary industry in the economy will not be conducive to the improvement of urban carbon emission performance. In addition, the impact of foreign direct investment (FDI) on carbon emission performance is significantly positive, indicating that increased foreign direct investment will help to improve urban carbon emission performance. The possible reason may be that FDI will bring in the introduction of capital and technology, which is not only conducive to improving the carbon emission performance of the local area, but also helps to improve the carbon emission performance of surrounding cities through the spatial spillover effect of technology.
In Section 3.1, we have discussed the overall impacts of energy-saving R&D on urban carbon emission performance based on the benchmark model (i.e., Eq. 2) and find a positive significant nexus between energy-saving R&D and carbon emission performance. To this end, another interesting question sparked our interests: how different types of energy-saving R&D affect urban carbon emission performance? Whether there exists a significant difference in their impact on carbon emission performance? Do they complement each other and synergistically promote urban carbon emission performance? To clarify these questions, we divided the total energy-saving R&D into two types according to their different use purpose: invention-type and utility-type, and examined their heterogenous impacts on urban carbon emission performance. Table 5 displays the corresponding results. The main results of columns (1)–(2) of Table 5 shows that only the utility-type energy-saving R&D (denoted as lnUERD) exhibited a significant positive impact on carbon emission performance at a 1% statistical level, while the invention-type energy-saving R&D (denoted as lnIERD) presented a non-significant positive result, implying that utility-type energy-saving R&D plays a more important role in improving carbon emission performance than the invention-type energy-saving R&D. However, it is worth noting that we cannot conclude the invention-type energy-saving R&D is not important just based on this alone. In the next step, we tried to introduce the cross terms of the two types of energy-saving R&D (lnIERD * lnUERD) into the benchmark model (i.e., Eq. 3), and examined whether the invention-type energy saving R&D can serve as a supplement for the utility-type energy-saving R&D to improve carbon emission performance more effectively. Column 3) of the Table 5 reports the corresponding results, we can see that the estimated coefficient of the cross term (lnIERD * lnUERD) is 0.209 and significant at the 1% statistical level, indicating that invention-type energy-saving R&D helps to strengthen the carbon reduction role of utility-type energy-saving R&D, thereby promoting urban carbon emission performance more effectively. Thus, the Hypothesis one is confirmed. Huang et al. (2021) came to similar conclusions by studying the spatial impacts of energy-saving R&D on China’s provincial energy consumption.
To ensure the reliability and robustness of the above empirical results, we perform robustness checks from the following three perspectives: policy change, adding new control variables, and different sample perspectives, in the next step.
Since the United Nations Climate Conference in 2015, global climate change has become a major concern for countries worldwide, and many countries have put forward ambitious goals aimed at addressing and mitigating climate change issues. As a big carbon emitter in the world, China has made active actions to reduce CO2 emissions and proposed a series of energy conservation and emission reduction policies and targets in the 13th Five-Year Plan (2016–2020) released in 2016. To check whether the policy change brings bias to the sample estimation results, this study introduces a dummy variable “Treat” to represent the 13th Five-Year Plan period in the benchmark model (Eq. 2). We set “Treat = 1” to denote the “13th Five-Year Plan” period, and use “Treat = 0” to indicate other periods. Columns (1)–(3) of Table 6 shows the corresponding results, it can be seen that the coefficient of the key explanatory variable—energy-saving R&D (i.e., lnERD) is significantly positive at 1% statistical level, which is consistent with the benchmark estimation results in Table 4. Regarding the two different types of energy-saving R&D (i.e., lnIERD and lnUERD), lnUERD exhibits a significant positive value, while lnIERD shows an insignificant estimation result, and their cross terms presents a significant positive value, which also keep accordance with the benchmark estimation results in Table 5. The above results indicate that the benchmark regression is robust and exclude the impacts of policy change.
In this step, we introduce new control variable PGDP (denoted as per capita GDP) to the benchmark model (i.e., Eq. 2) and re-estimate it by using the SYS-GMM technique. Columns (4)–(6) of the Table 6 shows the corresponding results, it can be seen that after adding the new control variable (i.e., PGDP), the signs and significance of the total energy-saving R&D and its two categories (i.e., lnIERD and lnUERD) still remain consistent with the baseline regression results in Tables 4, 5. Furthermore, the coefficient of the cross term (i.e., lnIERD*lnUERD) is also statistically significant and positive, further supporting the robustness of the benchmark estimation results.
Considering the economy of China’s four largest municipalities (i.e., Beijing, Shanghai, Tianjin and Chongqing) is at the top of the country, their energy-saving R&D may have a different impact on carbon emission performance than that of other cities. To avoid the bias of estimation results caused by city specificity, we re-estimated the benchmark model (i.e., Eq. 2) after removing the municipalities and Columns (7)–(9) of Table 6 shows the corresponding results. We can see that after removing the special values, the signs and significance of the core explanatory variable (i.e., lnERD), two different types of energy-saving R&D (i.e., lnIERD and lnUERD), and their cross terms still keep accordance with the results of the benchmark regression in Table 4 and Table 5, which excludes estimation bias caused by urban specificity, once again supporting the robustness of the benchmark estimation results.
According to the primary results in Section 4, energy-saving R&D has a significant positive impact on urban carbon emission performance. To this end, another interesting question sparks our interest: What is the internal transmission mechanism of energy-saving R&D on urban carbon emission performance? To systematically investigate how energy-saving R&D affects urban carbon emission performance, we established the following mediation effect model:
Where,
Table 7 shows the estimation results of mediating effect model. It can be seen that the coefficients of energy-saving R&D in models 1), 3), and 5) are significantly positive, indicating that energy-saving R&D helps to improve energy efficiency, optimize industrial structure, and promote urbanization process. To be specific, a 1% increase in energy-saving R&D promotes energy efficiency by 0.618%, industrial structure by 0.063% and urbanization level by 0.136%, indicating that energy-saving R&D has the most prominent role in improving energy efficiency. Meanwhile, the positive effects of energy-saving R&D on the optimization and upgrading of industrial structure and the promotion of urbanization have also been verified. In addition, in models 2), 4), and 6), the coefficients of technical effect (Tech), structural effect (Struc), and urbanization effect (URB) are all significant, implying that the mediation effects exist and are effective. Specifically, the coefficient of technical effect (Tech) is 0.189 at the 1% significance level, which indicates that the enhancement of energy efficiency significantly promotes the improvement of urban carbon emission performance. Technological progresses and innovations brought about by energy-saving R&D are conducive to improving energy utilization efficiency, reducing energy resource wastes and carbon emissions, and thus contributing to the improvement of urban carbon emission performance. Furthermore, the coefficients of structural effect (Struc) and urbanization effect (URB) are significantly negative at the 5% statistical level, with values of −0.077 and −0.058, respectively. As discussed in Section 1, technological progress and improved energy efficiency may prompt enterprises to use more energy to expand production scale and increase profits, which will cause energy rebound effect. This will weaken the positive role of energy-saving R&D on optimizing and upgrading the industrial structures, and ultimately resulting in a negative overall effect of industrial structure on carbon emission performance. Regarding the urbanization effect, due to the rigid demand for energy consumption and the corresponding carbon emissions caused by urbanization, the total effect of urbanization on carbon emission performance is also negative. In summary, the positive effects of technological effect on carbon emission performance exceeds the negative effects of structural effect and urbanization effect, ultimately leading to a positive promotion effect of energy-saving R&D on urban carbon emission performance. This transmission mechanisms are illustrated in Figure 2 more intuitively.
Figure 2. Transmission mechanism of energy-saving R&D on carbon emission performance. Transmission mechanism of energy-saving R&D on carbon emission performance.
Considering the main output of R&D activities, such as technological progress, has significant spillover effects (Awaworyi Churchill et al., 2019; Alam et al., 2020; Gu et al., 2021), a meaningful question sparks our interest: Does energy-saving R&D has spatial spillover effect on urban carbon emission emissions? If so, do different spatial factors affect this spillover effect? To the best of our knowledge, there has been no studies systematically examined the spatial impacts of energy-saving R&D on urban carbon emission performance and the driving factors of this spatial effect, especially for the case of China. Neglecting the spatial interaction effect into consideration will lead to a biased estimation result, therefore, it is necessary to conduct a spatial econometric analysis to examine the spatial impacts of energy-saving R&D on urban carbon emission performance.
Notably, a spatial autocorrelation test must be performed before constructing the spatial econometric model. We applied the commonly used Moran’s I and Geary’s C index tests for spatial autocorrelation check (Liu and Yang, 2021; Abudureheman et al., 2022b). The specific calculation formulas of the Moran’s I and Geary’s C indexes are as follows:
Where,
We constructed the spatial Durbin model (SDM) based on the approach introduced by LeSage and Pace (2014) to effectively capture the spillover effects of energy-saving R&D on urban carbon emission performance across both time and space dimensions. The specific expression of SDM model is as follows:
Where,
Table 8 shows the estimation results of the spatial Durbin model (SDM) (i.e., Eq. 8) under the geographical (W1) and economic (W2) spatial weight matrices, in which the direct effect denotes the influences of main explanatory variable on the explained variable in the local region, while the indirect effect (also known as spatial spillover effect) indicates the influences of key explanatory variable on the explained variable in the neighboring region. We can see from Table 8 that both the direct and indirect effects of the energy-saving R&D exhibits significant positive estimation results under the geographical (W1) and economic (W2) spatial weight matrices, indicating that energy-saving R&D not only contributes to the improvement of carbon emission performance in the local region, but also helps to the promote the carbon emission performance of neighboring areas; that is, there exists significant spatial spillover effects of energy-saving R&D on urban carbon emission performance, which supported the Hypothesis 2. One possible explanation is that the advancements in energy-saving technologies and innovations resulting from energy-saving R&D not only enhance the carbon emission performance within a region but also create positive externalities to the improvement of energy technologies in the surrounding areas through inter-regional industrial cooperation, talent flow and technical exchanges, which is helpful for promoting the carbon emission performance of neighboring areas. On the other hand, in the context of a low-carbon economy, the carbon emission performance has become an important indicator for both local governments and enterprises (Ferrat, 2021). Thus, there exists a competition effect among different regions to enhance their carbon emission performance (Abudureheman et al., 2022a). To be specific, improvements in the carbon emission performance of enterprises in neighboring cities may reduce the competitive advantages of enterprises in the local region, thus prompting local enterprises in the region to strengthen their carbon emission performance by taking effective measures, such as increasing energy-saving R&D investments, so as to improve their overall carbon emission performance.
In addition, we discovered another interesting finding from the estimation results in Table 6 that the elastic coefficients of energy-saving R&D and other control variables under the economic weight matrix (W2) are larger than that of the geographical weight matrix (W1), implying that economic factors play a more important role in the spatial spillover effects of energy-saving R&D than geographical factors. One possible explanation for this is that a close economic correlation between different regions is conducive to facilitate the cooperation among different regions in energy-saving research and development, promote the flow of energy-saving technologies and human resources, thereby promoting the carbon emission performance of both local region and spatially correlated regions.
Due to the differences in economic development and resource availability across cities, the effects of energy-saving R&D on carbon emission performance may vary among different types of urban groups. To understand this heterogeneity, we further classified cities from the following two perspectives. First, it is categorized into four groups according to city size, which includes megacities, metropolises, medium-sized cities and small cities. Second, it is classified into two groups according to the characteristics of resource endowment, which includes resource-based cities and non-resource-based cities. We conduct heterogeneity analysis on these different urban groups separately.
Table 9 shows the corresponding estimation results, from which we can see that there exists significant heterogeneity in the impact of energy-saving R&D on carbon emission performance among different urban groups. Specifically, the elasticity coefficients of energy-saving R&D in megacities and metropolises are significantly positive at the 1% statistical level, while it is comparatively weaker in medium-sized cities, being only significantly positive at the 10% level. However, the elasticity coefficients for small cities are not statistically significant. The possible reason may be that, as an economically developed urban group, megacities and metropolises have a solid economic foundation and abundant human capital, and their energy-saving R&D investment intensity is higher than that of medium and small cities with relatively underdeveloped economy. Therefore, energy-saving R&D in megacities and metropolises shows a significant promotion role in improving the cities’ carbon emission performance. However, due to the weak economic conditions, lack of human capital and other factors, medium-sized and small cities have relatively low energy-saving R&D investment intensity and the technology absorption and innovation capabilities are weaker than big cities. Therefore, during the sample period, energy-saving R&D in medium-sized and small cities did not show significant improvement in carbon emission performance. Regarding the resource-type urban groups, it is worth noting that energy-saving R&D significantly contributes to the carbon emission performance of both resource-based and non-resource-based cities at least at the 5% statistical significance level. The National Sustainable Development Plan for Resource-Based Cities (2013–2020), issued by the State Council in 2013, points out that resource-based cities are important strategic energy and resource bases in China, and it is necessary to improve energy and resources utilization efficiency of these cities, strengthen environmental governance and ecological protection, and realize green and low-carbon development in resource-based cities. Through the advancement and innovation of energy-saving technologies, the energy-saving R&D plays a crucial role in enhancing the energy utilization efficiency of resource-based cities. This, in turn, leads to a reduction in resource wastage, pollution emissions, and an improvement in carbon emission performance. Consequently, energy-saving R&D serves as a significant pathway towards achieving the low-carbon transformation and development of resource-based cities. At the same time, due to the high energy consumption demand and high carbon emission reduction pressure in most non-resource-based cities (such as Beijing, Shanghai, etc.), it is still necessary to strengthen energy-saving R&D investments in non-resource-based cities. This will facilitate the progress of energy-saving technologies, enhance energy efficiency, and ultimately improve the carbon emission performance of non-resource-based cities.
This study explores the potential impacts of energy-saving R&D on urban carbon emission performance based on a balanced panel dataset of 218 prefecture-level cities in China over the period 2007–2019. Different from previous studies that mainly focus on the total R&D, we differentiated the energy-saving R&D from the total R&D and categorized it two different types according to the purpose of energy-saving R&D, and further examined its heterogenous impacts on urban carbon emission performance for the first time. The internal mechanisms and spatial spillover effects of energy-saving R&D on urban carbon emission performance is also have been discussed. Lastly, the regional heterogeneity between different types of city group is further investigated. The main conclusions are as follows:
(1) Energy-saving R&D has obvious promoting effect on the improvement of urban carbon emission performance, among which the utility-type energy-saving R&D plays a more important role in improving carbon emission performance than the invention-type energy-saving R&D. At the same time, invention-type energy-saving R&D helps to strengthen the carbon reduction role of utility-type energy-saving R&D, thereby promoting urban carbon emission performance more effectively.
(2) The mechanism analysis based on the mediation effect model shows that energy-saving R&D promotes urban carbon performance mainly through the transmission mechanism of technical effects, while structural and urbanization effects weaken the positive benefits of energy-saving R&D due to the presence of energy rebound, which ultimately negatively affect carbon emission performance.
(3) Estimation results of spatial econometric model indicates that there exist significant spatial spillover effects of energy-saving R&D on urban carbon emission performance. That is, the energy-saving R&D not only contributes to the improvement of carbon emission performance in the local region, but also helps to promote the carbon emission performance of neighboring areas and economic factors play a more important role in this spatial effect.
(4) The impact of energy-saving R&D on carbon emission performance has obvious urban group heterogeneity; Specifically, energy-saving R&D in megacities and metropolises have a strong promotion effect on improving carbon emission performance, while it is weaker in medium-sized cities and small cities. Furthermore, energy-saving R&D in both the resource-based and non-resource-based cities significantly promoted the carbon emission performance of the cities.
In light of the main research findings above, this study highlights the following policy recommendations:
Firstly, the primary findings of the benchmark model shows that energy-saving R&D has a significant improvement in urban carbon emission performance. Therefore, it is necessary to strengthen investments in energy-saving R&D and promote the progress and innovations in energy-saving technologies. China is currently in the stage of high-quality new industrialization and urbanization, and its demand for energy consumption continues to be high. In this context, it is particularly important to reduce carbon emissions through energy-saving R&D and its innovation outcomes. The heterogeneous impact of different types of energy-saving R&D on carbon emission performance indicates that it is important to consider not only the up-front investments in energy-saving R&D but also the specific characteristics of different types of energy-saving R&D activities when formulating energy conservation and emission reduction policies. Local governments should provide financial supports for energy-saving R&D investments by establishing incentive mechanisms.
Secondly, the heterogeneous impact of different types of energy-saving R&D on carbon emission performance indicates that it is important to consider not only the up-front investments in energy-saving R&D but also the specific characteristics of different types of energy-saving R&D activities when formulating energy conservation and emission reduction policies. Given the evident role of utility-type energy-saving R&D in promoting urban carbon emission performance, energy conservation and emission reduction policies should give more prioritize to the utility-type energy-saving R&D and encourage enterprises to invest in these R&D activities. Furthermore, considering the synergistic effect of invention-type energy-saving R&D on the utility-type energy-saving R&D, attentions should also be given to the synchronous development of the invention-type energy-saving R&D. Local governments should provide financial supports for energy-saving R&D investments by establishing incentive mechanisms, such as subsidies, tax concessions and spatial funds.
Thirdly, the mediation effect mechanism analysis indicates that energy-saving R&D promotes carbon emission performance mainly through the technological effects, while structural effects and urbanization effects exhibit negative impacts on the carbon emission performance due to the existence of energy rebound effect. Therefore, while strengthening the technological effects of energy-saving R&D, attentions should also be paid to controlling the energy rebound effect brought about by technological progress. Reasonable energy pricing mechanisms and stringent environmental regulations can help reduce energy rebound effects and enhance industrial structure, thereby improving carbon emission performance. Furthermore, it is necessary to strengthen the high-quality development of urbanization, promote urban economic agglomeration, and improve the environmental awareness of urban residents, so as to improve urban carbon emission performance.
Fourthly, attentions should also be given to the heterogeneity of energy-saving R&D in different types of urban groups on carbon emission performance. According to the research findings of this study, energy-saving R&D in megacities and metropolises have a strong promotion effect on improving carbon emission performance, while it is weaker in medium-sized cities and small cities. Therefore, local governments should give policy preference to medium-sized and small cities and set up special funds to support energy-saving R&D investments. Meanwhile, medium-sized and small cities should strengthen their own technological innovation and absorption capabilities, increase investments in energy-saving R&D activities, and enhance energy-saving technology levels so as to improve their carbon emission performance.
The data that support the findings of this study are available from the corresponding author upon reasonable request.
The studies involving humans were approved by the Xinjiang University, Urumqi, China. The studies were conducted in accordance with the local legislation and institutional requirements. The participants provided their written informed consent to participate in this study.
MA: Data curation, Investigation, Methodology, Writing–original draft, Writing–review and editing. AY: Conceptualization, Supervision, Validation, Writing–original draft, Writing–review and editing.
The author(s) declare financial support was received for the research, authorship, and/or publication of this article. This work was supported from the Basic Research Business Expenses and Research Projects of Universities in Xinjiang Autonomous Region, China (NO. XJEDU 2024P002).
The authors declare that the research was conducted in the absence of any commercial or financial relationships that could be construed as a potential conflict of interest.
All claims expressed in this article are solely those of the authors and do not necessarily represent those of their affiliated organizations, or those of the publisher, the editors and the reviewers. Any product that may be evaluated in this article, or claim that may be made by its manufacturer, is not guaranteed or endorsed by the publisher.
Abudureheman, M., Jiang, Q., Dong, X., and Dong, C. (2022a). CO2 emissions in China: does the energy rebound matter? Energies 15, 4279. doi:10.3390/en15124279
Abudureheman, M., Jiang, Q., Dong, X., and Dong, C. (2022b). Spatial effects of dynamic comprehensive energy efficiency on CO2 reduction in China. Energy Policy 166, 113024. doi:10.1016/j.enpol.2022.113024
Abudureheman, M., Jiang, Q., Gong, J., and Yiming, A. (2023a). Estimating and decomposing the tfp growth of service-oriented manufacturing in China: a translogarithmic stochastic frontier approach. Sustainability 15, 6027. doi:10.3390/su15076027
Abudureheman, M., Jiang, Q., Gong, J., and Yiming, A. (2023b). Technology-driven smart manufacturing and its spatial impacts on carbon emissions: evidence from China. Comput. Industrial Eng. 181, 109283. doi:10.1016/j.cie.2023.109283
Acheampong, A. O., and Boateng, E. B. (2019). Modelling carbon emission intensity: application of artificial neural network. J. Clean. Prod. 225, 833–856. doi:10.1016/j.jclepro.2019.03.352
Alam, M. S., Apergis, N., Paramati, S. R., and Fang, J. (2020). The impacts of R&D investment and stock markets on clean-energy consumption and CO<sub>2</sub> emissions in OECD economies. Int. J. Finance Econ. 26, 4979–4992. doi:10.1002/ijfe.2049
Alam, M. S., Apergis, N., Paramati, S. R., and Fang, J. (2021). The impacts of R&D investment and stock markets on clean-energy consumption and CO<sub>2</sub> emissions in OECD economies. Int. J. Finance Econ. 26, 4979–4992. doi:10.1002/ijfe.2049
Alam, M. S., Atif, M., Chien-Chi, C., and Soytaş, U. (2019). Does corporate R&D investment affect firm environmental performance? Evidence from G-6 countries. Energy Econ. 78, 401–411. doi:10.1016/j.eneco.2018.11.031
Arellano, M., and Bover, O. (1995). Another look at the instrumental variable estimation of error-components models. J. Econ. 68, 29–51. doi:10.1016/0304-4076(94)01642-D
Awaworyi Churchill, S., Inekwe, J., Smyth, R., and Zhang, X. (2019). R&D intensity and carbon emissions in the G7: 1870–2014. Energy Econ. 80, 30–37. doi:10.1016/j.eneco.2018.12.020
Bilgili, F., Balsalobre-Lorente, D., Kuşkaya, S., Alnour, M., Önderol, S., and Hoque, M. E. (2023). Are research and development on energy efficiency and energy sources effective in the level of CO2 emissions? Fresh evidence from EU data. Environ. Dev. Sustain., 1–37. doi:10.1007/s10668-023-03641-y
Bilgili, F., Lorente, D. B., Kuşkaya, S., Ünlü, F., Gençoğlu, P., and Rosha, P. (2021). The role of hydropower energy in the level of CO2 emissions: an application of continuous wavelet transform. Renew. Energy 178, 283–294. doi:10.1016/j.renene.2021.06.015
Blundell, R., and Bond, S. (2023). Reprint of: initial conditions and moment restrictions in dynamic panel data models. J. Econ. 234, 38–55. doi:10.1016/j.jeconom.2023.03.001
Breusch, T. S., and Pagan, A. R. (1980). The Lagrange multiplier test and its applications to model specification in econometrics. Rev. Econ. Stud. 47, 239–253. doi:10.2307/2297111
Bye, B., and Jacobsen, K. (2011). Restricted carbon emissions and directed R&D support; an applied general equilibrium analysis. Energy Econ. 33, 543–555. doi:10.1016/j.eneco.2010.12.007
Chang, L., Hao, X., Song, M., Wu, J., Feng, Y., Qiao, Y., et al. (2020). Carbon emission performance and quota allocation in the Bohai Rim economic Circle. J. Clean. Prod. 258, 120722. doi:10.1016/j.jclepro.2020.120722
Chen, J., Sun, C., Shi, J., and Liu, J. (2022). Technology R&D and sharing in carbon emission reduction in a duopoly. Technol. Forecast. Soc. Change 183, 121936. doi:10.1016/j.techfore.2022.121936
Chen, W., Wang, G., Xu, N., Ji, M., and Zeng, J. (2023). Promoting or inhibiting? New-type urbanization and urban carbon emissions efficiency in China. Cities 140, 104429. doi:10.1016/j.cities.2023.104429
Chen, Z., Zhang, X., and Chen, F. (2021). Do carbon emission trading schemes stimulate green innovation in enterprises? Evidence from China. Technol. Forecast. Soc. Change 168, 120744. doi:10.1016/j.techfore.2021.120744
Churchill, S. A., Inekwe, J., Smyth, R., and Zhang, X. (2019). R&D intensity and carbon emissions in the G7: 1870–2014. Energy Econ. 80, 30–37. doi:10.1016/j.eneco.2018.12.020
CNIPA (2020) China national intellectual property administration. Available at: https://www.cnipa.gov.cn/.
CSY (2020) China statistical yearbook. Available at: http://www.stats.gov.cn/.
Cui, H., and Cao, Y. (2023). China's cities go carbon neutral: how can new-type urbanization policies improve urban carbon performance? Sustain. Prod. Consum. 42, 74–94. doi:10.1016/j.spc.2023.09.011
CUSY (2020) China urban statistical yearbook. Available at: http://www.stats.gov.cn/.
Ding, L.-L., Lei, L., Zhao, X., and Calin, A. C. (2020). Modelling energy and carbon emission performance: a constrained performance index measure. Energy 197, 117274. doi:10.1016/j.energy.2020.117274
Fang, G., Chen, G., Yang, K., Yin, W., and Tian, L. (2023a). Can green tax policy promote China's energy transformation? a nonlinear analysis from production and consumption perspectives. Energy 269, 126818. doi:10.1016/j.energy.2023.126818
Fang, G., Huang, M., Zhang, W., and Tian, L. (2024). Exploring global embodied carbon emissions transfer network—an analysis based on national responsibility. Technol. Forecast. Soc. Change 202, 123284. doi:10.1016/j.techfore.2024.123284
Fang, G., Yang, K., Chen, G., and Tian, L. (2023b). Environmental protection tax superseded pollution fees, does China effectively abate ecological footprints? J. Clean. Prod. 388, 135846. doi:10.1016/j.jclepro.2023.135846
Ferrat, Y. (2021). Carbon emissions and firm performance: a matter of horizon, materiality and regional specificities. J. Clean. Prod. 329, 129743. doi:10.1016/j.jclepro.2021.129743
Friedman, M. (1937). The use of ranks to avoid the assumption of normality implicit in the analysis of variance. J. Am. Stat. Assoc. 32, 675–701. doi:10.2307/2279372
Gao, S., Sun, D., and Wang, S. (2023). Do development zones increase carbon emission performance of China's cities? Sci. Total Environ. 863, 160784. doi:10.1016/j.scitotenv.2022.160784
Gu, G., Wang, Z., and Wu, L. (2021). Carbon emission reductions under global low-carbon technology transfer and its policy mix with R&D improvement. Energy 216, 119300. doi:10.1016/j.energy.2020.119300
He, Y., Fu, F., and Liao, N. (2021). Exploring the path of carbon emissions reduction in China’s industrial sector through energy efficiency enhancement induced by R&D investment. Energy 225, 120208. doi:10.1016/j.energy.2021.120208
Hong, S., Hui, E. C. M., and Lin, Y. (2022). Relationships between carbon emissions and urban population size and density, based on geo-urban scaling analysis: a multi-carbon source empirical study. Urban Clim. 46, 101337. doi:10.1016/j.uclim.2022.101337
Honma, S., Ushifusa, Y., Okamura, S., and Vandercamme, L. (2023). Measuring carbon emissions performance of Japan's metal industry: energy inputs, agglomeration, and the potential for green recovery reduction. Resour. Policy 82, 103519. doi:10.1016/j.resourpol.2023.103519
Huang, J., and Chen, X. (2020). Domestic R&D activities, technology absorption ability, and energy intensity in China. Energy Policy 138, 111184. doi:10.1016/j.enpol.2019.111184
Huang, J., Chen, X., Cai, X., and Zou, H. (2021). Assessing the impact of energy-saving R&D on China’s energy consumption: evidence from dynamic spatial panel model. Energy 218, 119443. doi:10.1016/j.energy.2020.119443
Huang, J., Luan, B., Cai, X., and Zou, H. (2020). The role of domestic R&D activities played in carbon intensity: evidence from China. Sci. Total Environ. 708, 135033. doi:10.1016/j.scitotenv.2019.135033
Iftikhar, Y., Wang, Z., Zhang, B., and Wang, B. (2018). Energy and CO2 emissions efficiency of major economies: a network DEA approach. Energy 147, 197–207. doi:10.1016/j.energy.2018.01.012
Jiao, J., Jiang, G., and Yang, R. (2018). Impact of R&D technology spillovers on carbon emissions between China’s regions. Struct. Change Econ. Dyn. 47, 35–45. doi:10.1016/j.strueco.2018.07.002
Koçak, E., and Ulucak, Z. Ş. (2019). The effect of energy R&D expenditures on CO 2 emission reduction: estimation of the STIRPAT model for OECD countries. Environ. Sci. Pollut. Res. 26, 14328–14338. doi:10.1007/s11356-019-04712-2
Kou, J., and Xu, X. (2022). Does internet infrastructure improve or reduce carbon emission performance? --A dual perspective based on local government intervention and market segmentation. J. Clean. Prod. 379, 134789. doi:10.1016/j.jclepro.2022.134789
Kuşkaya, S. (2022). Residential solar energy consumption and greenhouse gas nexus: evidence from Morlet wavelet transforms. Renew. Energy 192, 793–804. doi:10.1016/j.renene.2022.04.107
Lee, K.-H., and Min, B. (2015). Green R&D for eco-innovation and its impact on carbon emissions and firm performance. J. Clean. Prod. 108, 534–542. doi:10.1016/j.jclepro.2015.05.114
Lee, K.-H., Min, B., and Yook, K.-H. (2015). The impacts of carbon (CO2) emissions and environmental research and development (R&D) investment on firm performance. Int. J. Prod. Econ. 167, 1–11. doi:10.1016/j.ijpe.2015.05.018
LeSage, J. P., and Pace, R. K. (2014). The biggest myth in spatial econometrics. Econometrics 2, 217–249. doi:10.3390/econometrics2040217
Li, H., and Shi, J.-f. (2014). Energy efficiency analysis on Chinese industrial sectors: an improved Super-SBM model with undesirable outputs. J. Clean. Prod. 65, 97–107. doi:10.1016/j.jclepro.2013.09.035
Li, J., Shi, J., Duan, K., Li, H., Zhang, Y., and Xu, Q. (2022). Efficiency of China's urban development under carbon emission constraints: a city-level analysis. Phys. Chem. Earth, Parts A/B/C 127, 103182. doi:10.1016/j.pce.2022.103182
Lin, B., and Zhou, Y. (2021). Does the Internet development affect energy and carbon emission performance? Sustain. Prod. Consum. 28, 1–10. doi:10.1016/j.spc.2021.03.016
Lin, B., and Zhu, R. (2021). Energy efficiency of the mining sector in China, what are the main influence factors? Resour. Conservation Recycl. 167, 105321. doi:10.1016/j.resconrec.2020.105321
Liu, M., and Yang, L. (2021). Spatial pattern of China’s agricultural carbon emission performance. Ecol. Indic. 133, 108345. doi:10.1016/j.ecolind.2021.108345
Lu, H., Meng, H., Lu, C., Shang, D., Wang, D., and Jin, H. (2023). The mechanism for selecting low carbon urban experimentation cases in the literature and its impact on carbon emission performance. J. Clean. Prod. 420, 138191. doi:10.1016/j.jclepro.2023.138191
Lv, Y., Liu, J., Cheng, J., and Andreoni, V. (2021). The persistent and transient total factor carbon emission performance and its economic determinants: evidence from China's province-level panel data. J. Clean. Prod. 316, 128198. doi:10.1016/j.jclepro.2021.128198
Magazzino, C., Mele, M., Drago, C., Kuşkaya, S., Pozzi, C., and Monarca, U. (2023). The trilemma among CO2 emissions, energy use, and economic growth in Russia. Sci. Rep. 13, 10225. doi:10.1038/s41598-023-37251-5
Narsa Goud, N. (2022). Corporate governance: does it matter management of carbon emission performance? An empirical analyses of Indian companies. J. Clean. Prod. 379, 134485. doi:10.1016/j.jclepro.2022.134485
NGDC (2020). National Geophysical Data Center. Available at: http://www.ngdc.noaa.gov/index.html.
Oyewo, B. (2023). Corporate governance and carbon emissions performance: international evidence on curvilinear relationships. J. Environ. Manag. 334, 117474. doi:10.1016/j.jenvman.2023.117474
Pan, M., Zhao, X., lv, K., Rosak-Szyrocka, J., Mentel, G., and Truskolaski, T. (2023). Internet development and carbon emission-reduction in the era of digitalization: where will resource-based cities go? Resour. Policy 81, 103345. doi:10.1016/j.resourpol.2023.103345
Pesaran, M. H. (2004) General diagnostic tests for cross section dependence in panels (IZA Discussion Paper No. 1240). Institute for the Study of Labor IZA. doi:10.17863/CAM.5113
Pesaran, M. H. (2007). A simple panel unit root test in the presence of cross-section dependence. J. Appl. Econ. 22, 265–312. doi:10.1002/jae.951
Petrović, P., and Lobanov, M. M. (2020). The impact of R&D expenditures on CO2 emissions: evidence from sixteen OECD countries. J. Clean. Prod. 248, 119187. doi:10.1016/j.jclepro.2019.119187
Schröder, E., and Storm, S. (2020). Economic growth and carbon emissions: the road to “hothouse earth” is paved with good intentions. Int. J. Political Econ. 49, 153–173. doi:10.1080/08911916.2020.1778866
Shahbaz, M., Nasir, M. A., Hille, E., and Mahalik, M. K. (2020). UK's net-zero carbon emissions target: investigating the potential role of economic growth, financial development, and R&D expenditures based on historical data (1870–2017). Technol. Forecast. Soc. Change 161, 120255. doi:10.1016/j.techfore.2020.120255
Song, W., Mao, H., and Han, X. (2021). The two-sided effects of foreign direct investment on carbon emissions performance in China. Sci. Total Environ. 791, 148331. doi:10.1016/j.scitotenv.2021.148331
Torres, M., and Pinho, P. (2011). Encouraging low carbon policies through a local emissions trading scheme (LETS). Cities 28, 576–582. doi:10.1016/j.cities.2011.06.005
Wang, S., Wang, J., Fang, C., and Li, S. (2019). Estimating the impacts of urban form on CO2 emission efficiency in the Pearl River Delta, China. Cities 85, 117–129. doi:10.1016/j.cities.2018.08.009
Wu, H., Xue, Y., Hao, Y., and Ren, S. (2021). How does internet development affect energy-saving and emission reduction? Evidence from China. Energy Econ. 103, 105577. doi:10.1016/j.eneco.2021.105577
Xu, L., Fan, M., Yang, L., and Shao, S. (2021a). Heterogeneous green innovations and carbon emission performance: evidence at China's city level. Energy Econ. 99, 105269. doi:10.1016/j.eneco.2021.105269
Xu, L., Fan, M., Yang, L., and Shao, S. (2021b). Heterogeneous green innovations and carbon emission performance: evidence at China's city level. Energy Econ. 99, 105269. doi:10.1016/j.eneco.2021.105269
Yang, C., and Zhao, S. (2023). Scaling of Chinese urban CO2 emissions and multiple dimensions of city size. Sci. Total Environ. 857, 159502. doi:10.1016/j.scitotenv.2022.159502
Yu, P., Hao, R., Cai, Z., Sun, Y., and Zhang, X. (2022). Does emission trading system achieve the win-win of carbon emission reduction and financial performance improvement? evidence from Chinese A-share listed firms in industrial sector. J. Clean. Prod. 333, 130121. doi:10.1016/j.jclepro.2021.130121
Yue, W., Li, Y., Su, M., Chen, Q., and Rong, Q. (2023). Carbon emissions accounting and prediction in urban agglomerations from multiple perspectives of production, consumption and income. Appl. Energy 348, 121445. doi:10.1016/j.apenergy.2023.121445
Zhang, W., Liu, X., Wang, D., and Zhou, J. (2022). Digital economy and carbon emission performance: evidence at China's city level. Energy Policy 165, 112927. doi:10.1016/j.enpol.2022.112927
Zhang, W., Xu, Y., Streets, D. G., and Wang, C. (2023). Can new-type urbanization realize low-carbon development? A spatiotemporal heterogeneous analysis in 288 cities and 18 urban agglomerations in China. J. Clean. Prod. 420, 138426. doi:10.1016/j.jclepro.2023.138426
Zhao, H., Chen, S., and Zhang, W. (2023). Does digital inclusive finance affect urban carbon emission intensity: evidence from 285 cities in China. Cities 142, 104552. doi:10.1016/j.cities.2023.104552
Zhou, A., Xin, L., and Li, J. (2022). Assessing the impact of the carbon market on the improvement of China's energy and carbon emission performance. Energy 258, 124789. doi:10.1016/j.energy.2022.124789
Zhu, H. M., Duan, L. J., Guo, Y. W., and Yu, K. M. (2016). The effects of FDI, economic growth and energy consumption on carbon emissions in ASEAN-5: evidence from panel quantile regression. Econ. Model. 58, 237–248. doi:10.1016/j.econmod.2016.05.003
Keywords: energy-saving R&D, urban carbon emission performance, heterogeneity analysis, mediating effect, China
Citation: Abudureheman M and Yiming A (2024) The impact of energy-saving R&D on urban carbon emission performance: evidence from 218 prefecture-level cities in China. Front. Environ. Sci. 12:1385363. doi: 10.3389/fenvs.2024.1385363
Received: 12 February 2024; Accepted: 13 May 2024;
Published: 12 June 2024.
Edited by:
Shigeyuki Hamori, Kobe University, JapanReviewed by:
Sevda Kuşkaya, Erciyes University, TürkiyeCopyright © 2024 Abudureheman and Yiming. This is an open-access article distributed under the terms of the Creative Commons Attribution License (CC BY). The use, distribution or reproduction in other forums is permitted, provided the original author(s) and the copyright owner(s) are credited and that the original publication in this journal is cited, in accordance with accepted academic practice. No use, distribution or reproduction is permitted which does not comply with these terms.
*Correspondence: Abulaiti Yiming, YWltaW5AeGpudS5lZHUuY24=
Disclaimer: All claims expressed in this article are solely those of the authors and do not necessarily represent those of their affiliated organizations, or those of the publisher, the editors and the reviewers. Any product that may be evaluated in this article or claim that may be made by its manufacturer is not guaranteed or endorsed by the publisher.
Research integrity at Frontiers
Learn more about the work of our research integrity team to safeguard the quality of each article we publish.