- 1Zukunftskolleg, University of Konstanz, Konstanz, Germany
- 2Independent Research, Köln, Germany
- 3Department of Ecological Sciences, Faculty of Sciences, University of Chile, Santiago, Chile
- 4Department of Computer and Information Science, University of Konstanz, Konstanz, Germany
Sorption is a key process to understand the environmental fate of pollutants on soils, conduct preliminary risk assessments and fill information gaps. Quantitative Structure-Activity Relationships (QSAR) and Pedotransfer Functions (PTF) are the most common approaches used in the literature to predict sorption. Both models use different outcomes and follow different simplification strategies to represent data. However, the impact of those differences on the interpretation of sorption trends and application of models for regulatory purposes is not well understood. We conducted a systematic review to contextualize the requirements for developing, interpreting, and applying predictive models in different scenarios of environmental concern by using pesticides as a globally relevant organic pollutant model. We found disagreements between predictive model assumptions and empirical information from the literature that affect their reliability and suitability. Additionally, we found that both model procedures are complementary and can improve each other by combining the data treatment and statistical validation applied in PTF and QSAR models, respectively. Our results expose how relevant the methodological and environmental conditions and the sources of variability studied experimentally are to connect the representational value of data with the applicability domain of predictive models for scientific and regulatory decisions. We propose a set of empirical correlations to unify the sorption mechanisms within the dataset with the selection of a proper kind of model, solving apparent incompatibilities between both models, and between model assumptions and empirical knowledge. The application of our proposal should improve the representativity and quality of predictive models by adding explicit conditions and requirements for data treatment, selection of outcomes and predictor variables (molecular descriptors versus soil properties, or both), and an expanded applicability domain for pollutant-soil interactions in specific environmental conditions, helping the decision-making process in regard to both scientific and regulatory concerns (in the following, the scientific and regulatory dimensions).
1 Introduction
Sorption is a key process in tracking the environmental fate of pollutants on soils due to its role in increasing the persistence and accumulation (Dollinger et al., 2015; Rybacka and Andersson, 2016; Zhu et al., 2017; De Gerónimo et al., 2018; Cai et al., 2019; Conde-Cid et al., 2019; Pandey and Roy, 2021) by reducing their transport (Kodešová et al., 2015; Wang et al., 2015; Sidoli et al., 2016; De Gerónimo et al., 2018; Zhang et al., 2018; Cai et al., 2019; Conde-Cid et al., 2019; Conde-Cid et al., 2020; Pandey and Roy, 2021; Hu et al., 2022) and bioavailability (Kodešová et al., 2015; Zhang et al., 2018; Cai et al., 2019; Conde-Cid et al., 2020; Cantwell et al., 2022; Hu et al., 2022), and negatively affecting their biodegradation (Dollinger et al., 2015; Aranda et al., 2016; Zhang et al., 2018; Cai et al., 2019; Pandey and Roy, 2021; Cantwell et al., 2022) and bioremediation (Cantwell et al., 2022). This has led to the development of various models for the prediction of sorption, with two taking a dominant position in the literature: quantitative structure-activity relationships (QSAR) and pedotransfer functions (PTF). Both models are considered an efficient option for conducting preliminary risk assessment, filling information gaps, identify soil issues and guide soil management and sustainability in different soils of interest (e.g., agricultural, polluted, and vulnerable soils) when the production of experimental data may be infeasible for real time and large-scale decision-making (Wang et al., 2015; Aranda et al., 2016; Sidoli et al., 2016; Singh et al., 2016; Berthod et al., 2017; Sabour and Moftakhari Anasori Movahed, 2017; Zhu et al., 2017; De Gerónimo et al., 2018; Zhang et al., 2018; Cai et al., 2019; Conde-Cid et al., 2019; Conde-Cid et al., 2020; Kobayashi et al., 2020; Kobayashi and Yoshida, 2021; Muhire et al., 2021; Pandey and Roy, 2021; Cantwell et al., 2022; Hu et al., 2022; Jiang et al., 2022). Additionally, both models predict sorption on soils through sorption coefficients, which represent the linear/nonlinear distribution between the retained and the aqueous concentration of pollutant in chemical equilibrium in sorption isotherm studies (Neira-Albornoz et al., 2022).
Despite the fact that both kinds of models seem applicable under similar conditions and have been scientifically validated in their predictive ability, their shared goal is pursued by employing different procedures and assumptions that entail different chemical, computational, and regulatory implications. We therefore identified three factors that may affect their reliability and suitability for regulatory purposes: (i) outcome selection, which depends on the experimental design and conditions as well as methodological considerations due to the complexity of environmental systems (Neira-Albornoz et al., 2022); (ii) simplification strategy, with QSAR models describing the sorption process through molecular properties of pollutants (Wang et al., 2015; Aranda et al., 2016; Berthod et al., 2017; Sabour and Moftakhari Anasori Movahed, 2017; Zhu et al., 2017; Zhang et al., 2018; Cai et al., 2019; Kobayashi et al., 2020; Kobayashi and Yoshida, 2021; Muhire et al., 2021; Pandey and Roy, 2021; Cantwell et al., 2022; Jiang et al., 2022), and PTF assuming that the sorption depends on the local soil properties instead (Sidoli et al., 2016; Singh et al., 2016; De Gerónimo et al., 2018; Conde-Cid et al., 2019; Conde-Cid et al., 2020; Hu et al., 2022); and (iii) institutionalization, where only QSAR models have been promoted by the Organization for Economic Co-operation and Development (OECD), the Registration, Evaluation, Authorization, and Restriction of Chemicals (REACH) and the U.S. Environmental Protection Agency (USEPA), based on institutional requirements and guidelines (OECD, 2014; Card et al., 2017; Kar et al., 2018; Thomas et al., 2019; Chinen and Malloy, 2020).
For instance, different, non-equivalent sorption coefficients are used to represent the sorption process (Mamy et al., 2015; Nolte and Ragas, 2017; Neira-Albornoz et al., 2022), which are interpreted as physicochemical properties of pollutants (QSAR) or of soils (PTF), affecting the data collection, data treatment and the reliability, comparability, and applicability of their predictions in real scenarios. Therefore, understanding the background of QSAR and PTF models is crucial to determine when and how to apply them in environmental contexts of concern. However, this comparative background is not fully understood, creating uncertainty in both scientific and decision-making practice.
In this work, we assess the links between the underlying assumptions of predictive models and the state-of-the-art based on experimental studies to develop a conceptual background for improving the use of models for the prediction of the sorption of pollutants on soils for regulatory purposes. We contribute to the scientific practice and decision-making processes in three ways: (i) we develop a comprehensive analysis of two contrasting approaches for predicting sorption coefficients for regulatory purposes, (ii) we connect the QSAR and PTF theories to current experimental trends, and (iii) we propose a procedure that unifies and improves the contextualization and interpretability of predictive models.
2 Methodology
Our methodology included three stages: (i) the presentation of an explicit scope to introduce our audience and contextualize the outcomes and pollutants assessed in our study, (ii) the description of the systematic review, and (iii) the extraction of information from the literature. We divided the last step into (i) QSAR and PTF models to describe and compare their development backgrounds, and (ii) empirical findings from the literature to contrast them with the development and interpretation of predictive models.
2.1 Scope of the study
2.1.1 Sorption coefficients
Our study is focused on three sorption coefficients used as outcomes in QSAR and PFT models to describe the sorption of pollutants of environmental concern: (i) the linear coefficient,
2.1.2 Approach
Our analysis involved three layers (Engeström, 2011; Jensen, 2022): (i) an interpretative layer, where assumptions used by scientists and predictive models to make decisions were explicitly defined and addressed, (ii) a contradictory layer, where contradictions among QSAR and PTF assumptions were analyzed, and (iii) an agentive layer, involving different actors that transform scientific practices, including regulatory agencies (e.g., OECD guidelines for the development of predictive models) (OECD, 2014).
The interpretative layer has already been addressed in critical reviews for QSAR (Hansen et al., 1999; Mamy et al., 2015; Nolte and Ragas, 2017) and PTF (McBratney et al., 2002; Van Looy et al., 2017) models, independently. However, they were not contrasted with each other (QSAR vs. PTF) nor with experimental studies. In this article, we compared both models and included three scientific actors (QSAR developers, PTF developers, and soil scientists conducting experimental research), their assumptions as well as potential contradictions, and the impact of OECD guidelines on the scientific practices.
2.1.3 Decision-making process
We defined two dimensions: scientific and regulatory (Figure 1). The scientific dimension is composed of soil scientists who conduct experimental research or develop and use predictive models (scientific decisions). The regulatory dimension involves environmental entities that apply those models in different socio-environmental contexts at local or global scale (regulatory decisions).
Our study considers scientific decisions as model development, where the interpretative layer consist of (i) experimental designs used by soil scientists to produce data, and (ii) theoretical background and assumptions applied during the predictive model development. Additionally, a contradictory layer was addressed through the comparison of (i) QSAR and PTF models, and (ii) predictive models versus empirical information.
In addition, we considered regulatory decisions as model implementation through a contradictory layer that considered the concord between experimental designs and predictive model assumptions to evaluate the representational value of data and reliability of predictions. In addition, we included an agentive layer based on the OECD principles (OECD, 2014), which are used in QSAR models and equivalent to some REACH conditions (Chinen and Malloy, 2020).
In this sense, our approach (Figure 1) shows how different scientific and regulatory decisions are mediated by predictive models that act as an interface (e.g., science-policy interaction) and whose understanding requires a holistic approach.
2.2 Systematic literature review
As the basis for our investigation, we conducted two systematic literature reviews about research into the sorption of organic pollutants on soils: one for QSAR and PTF models (Figure 2A) and another for experimental sorption studies (Figure 2B).
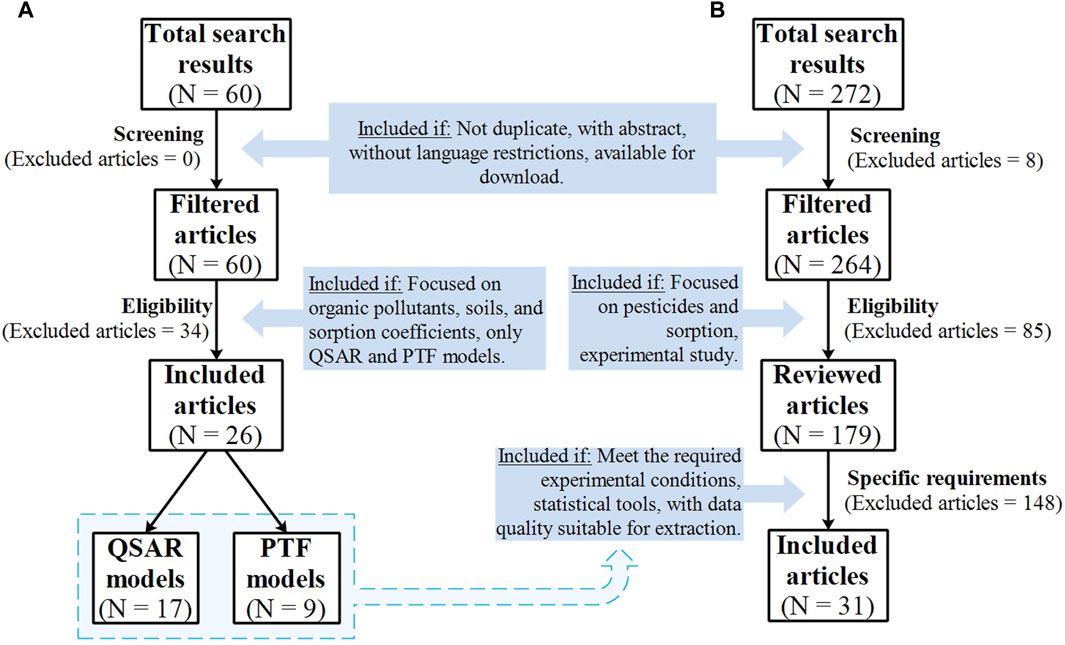
Figure 2. Flow chart and procedure for the analysis of recent articles that (A) develop predictive models, and (B) conduct experimental studies.
Based on the number of published articles, we used two intervals of recent years to represent research and trends conducted with comparable and currently validated procedures: 5 years (2017–2021) for experimental studies, and (ii) 8 years (2015–2022) for QSAR and PTF models.
We identified numerous experimental studies, which exceeded the feasible amount for manual annotation, and consequently selected pesticides as a globally relevant organic pollutant model of focus. While reducing the manual data extraction to a scope that was feasible to conduct, this also ensured a sufficiently diverse number of pollutants in the considered studies, allowing us to extrapolate our findings to other organic pollutants assessed by QSAR and PTF models.
A schematic overview is shown in Figure 2. A detailed description of our search strategy (keywords, search date, dataset used) and the application of inclusion criteria for each individual article are listed in Supplementary Table S1 for QSAR and PTF models, and Supplementary Table S2 for experimental sorption studies. In screening the related work, we discarded duplicates, articles without abstract, with language restrictions, or those that were not downloadable. We then applied eligibility criteria for each literature review. For predictive models, we included articles focused on QSAR or PTF models to predict sorption coefficients of organic pollutants on soils, describing their procedure (Figure 2A; Supplementary Table S1). For experimental studies, we included articles conducting experimental studies on the sorption of pesticides on soils and soil-related sorbents (Figure 2B; Supplementary Table S2).
We only included experimental studies that meet three specific requirements based on the selected predictive models (Figure 2B; Supplementary Table S2). First, experimental conditions that ensure data comparability and representativeness: the corroboration of equilibrium condition through kinetic studies, mention of the isotherm shape, and description of the quantification method (e.g., mass balance to quantify the sorbed concentration from the aqueous concentration). Second, the use of statistical tools to validate sorption coefficients: Pearson coefficient >0.95 and five or more concentration points to produce one sorption coefficient, based on the minimum observation:descriptor ratio proposed for QSAR models to avoid overfitting (Roy et al., 2015). Finally, the suitability and comprehensiveness of the provided data descriptions for data extraction: an explicit calculation of sorption coefficients including clear values, units and nomenclature along the article, and explicit conditions where data were quantified, such as solution, sorbent:solution ratio, and interval of concentrations.
All selection criteria were applied successively.
2.3 Description of QSAR and PTF development procedures
We conducted a descriptive analysis of scientific articles producing predictive models through the following process: First, we divided their procedures into shared steps (Figure 3). Then, we extracted information related to each step: outcome selection (outcome, units, accomplishment of the OECD principle P1), data collection and treatment (data production, data mining, diversity of data, experimental conditions used to quantify data within the dataset, data treatment, kind of sorption coefficients included in the dataset), predictor variables (kind of predictor, quantification method), model equation and validation (splitting procedures, algorithms linked to the OECD principles P2 and P4), and applicability and regulatory purposes (considering the OECD principles P3 and P5). This information is shown in Supplementary Table S3. Later, we compared the approaches both models followed, and finally we identified and described similarities and differences, summarizing relevant patterns for the development of predictive models.
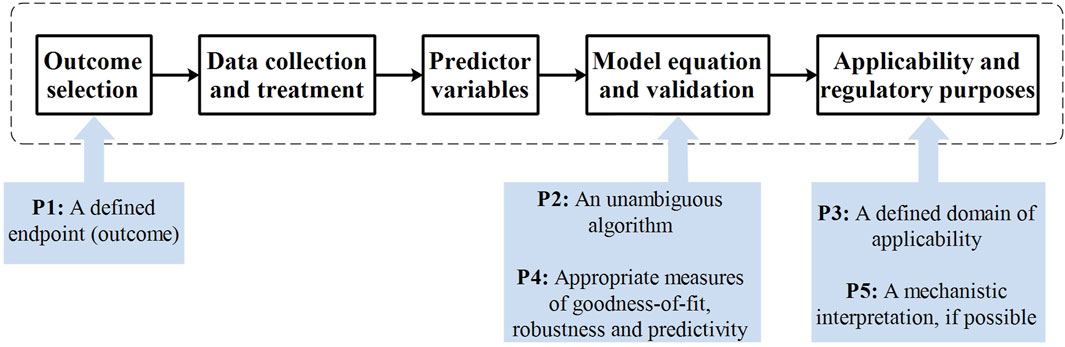
Figure 3. General procedure for developing predictive models, including the OECD principles (from P1 to P5) (OECD, 2014).
Additionally, we proposed QSAR and PTF assumptions for explaining common procedures during the model development procedures. Assumptions are (i) implicit within each article, (ii) derived from general observations during the development of predictive models, and (iii) conceptually linked to the simplification strategies followed by both predictive models.
2.4 Extraction and classification of the empirical information
The following information was extracted from the experimental studies (Figure 2B): (i) data per article, (ii) sources of variability explored during the experimental design within each article, (iii) pesticides studied per article (to evaluate frequency and consistency among studies), and (iv) characterization of pesticides studied within an article by name, target, acid-base activity, and chemical class.
The information extracted from articles is shown in Supplementary Table S4, where we classified the sources of variability addressed within each article into two groups: soil variability (SV) and other sources of variability (OV). SV included the use of different soils (SV [soil]), treatments applied to the soils prior to the experiment (SV [treat]), spatial variability during the soil sampling process (SV [spatial]), and the lack of soil variability (SV [none]). On the other hand, OV included the use of different pesticides (OV [pollut]), control and analysis of specific experimental conditions (OV [exp]), and the lack of other sources of variability (OV [none]). The effect of time (OV [time]) was investigated in a few reviewed articles and discarded during the application of specific requirements for the included articles (Figure 2B).
During the classification of soil variability, we considered soil-related sorbents (e.g., soils, sediments, microplastics) as independent. Additionally, we defined SV [treat] as treatments made to one soil that modifies its structural composition, such as additions (e.g., application of organic amendments, biochar at one or different temperatures, lime, fertilizers, adjuvants, microplastics), removals (e.g., removal of organic matter), isolation of components (e.g., extraction of clay fractions), and management practices. In this sense, different sorbents obtained from one soil were considered as SV [treat] instead of SV [soil]. Finally, SV [spatial] encodes topographic variations in which all samples belong to the same soil but at different depths, horizons, or surface location in one plot.
For other sources of variability, OV [pollut] represents the quantification of the sorption coefficient of different chemicals in the same article, while OV [exp] represents the application of different approaches to describe the behavior of the same pollutant, such as sorption studies (e.g., one-point and sorption isotherm), experimental conditions (e.g., pure pesticides, mixture of pesticides, commercial formulations) and control of variables (e.g., the effect of temperature, pH, salinity, soil solution composition and sterilization of soils in the sorption experiment).
Pesticide names were homogenized when the same pesticide had different names among the articles, and their attributes were derived from the Pesticide Properties Database, PPDB (Lewis et al., 2016). Then, the most relevant functional groups of every pesticide were obtained from the database (Lewis et al., 2016). The groups were ordered according to the number of apparitions among the studies, and pesticides were successively classified considering their most frequent group. The compounds with low-frequent groups or lack of information on the PPDB website were classified within the groups in which they fit the best after a structural analysis. Finally, we created four sub-groups according to common characteristics within the groups, whose chemical structures are shown in Supplementary Figure S1. The information about pesticides is shown in Supplementary Table S5.
The combination of articles studying the same pesticide was used to evaluate (i) sources of variability available in the literature per pesticide, (ii) number of articles studying a pesticide, and (iii) characterization of individual pesticides. Then, the distribution of information was analyzed among articles and pesticides, and the contrast with QSAR and PTF assumptions and the impact for scientific and regulatory decisions was discussed.
Finally, we analyzed the interpretation of current QSAR models through the following procedure: (i) we considered the averaged sorption coefficient values for one pesticide among different soils (called
3 Literature review results
In this section we present our results for predictive models and empirical findings. Table 1 is a condensed version of Supplementary Table S3 and acts as a guide for reader in this section, showing information from Figure 3 for each article, such as outcome, data collection and treatment (type and diversity), predictor variables, model equation and validation, and applicability domain.
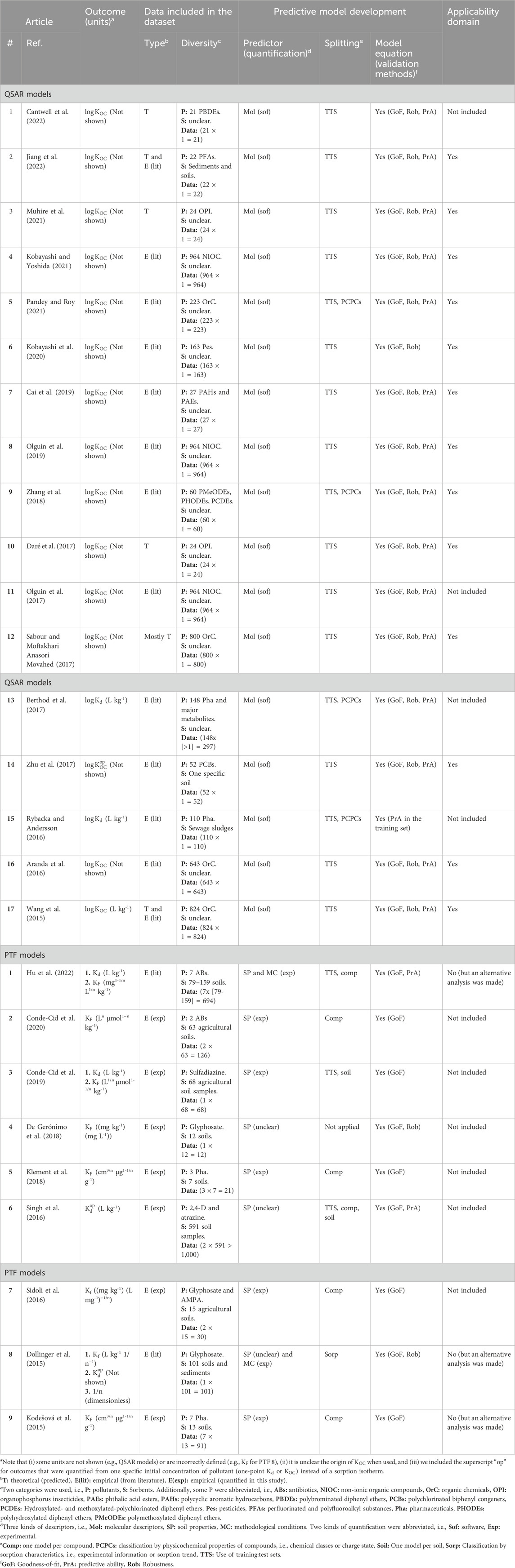
Table 1. Summary of the information extracted from QSAR and PTF models from the literature (presented in the same order as in Supplementary Table S3).
3.1 Literature on predictive model development
Based on the articles found in the literature (17 QSAR and 9 PTF models, Figure 2A), we provide a comprehensive analysis by considering the key development steps of QSAR and PTF models (Figure 3). Furthermore, we include the five principles proposed by the OECD to guide the development procedure of QSAR models for regulatory purposes (P1-5, Figure 3) (OECD, 2014), to enable a comparison between QSAR and PTF models.
3.1.1 Outcome selection
3.1.1.1 QSAR models
According to the QSAR model theory, activities or properties of pollutants (e.g., sorption coefficients) are explained by their chemical structure (Aranda et al., 2016; Olguin et al., 2019). This implies that the outcome is exclusively dependent on pollutants, or put another way, independent of soil properties.
In the literature, a high correlation between soil organic carbon content (OC) and sorption coefficients has been found for non-ionizable or neutral pollutants sorbed on soils with OC > 0.1% (Olguin et al., 2017; Olguin et al., 2019). This minimizes the soil variability (only OC is important) and allows the extrapolation of trends to other soils having the same sorption mechanism, especially if the mechanism is unspecific (i.e., pollutants may interact with different types of sorption sites) and generalizable (i.e., a specific soil component may represent the whole sorption), such as hydrophobic. Under this scenario, the sorption coefficient is a property of pollutants (Olguin et al., 2017; Cai et al., 2019).
In this sense, most QSAR models predicted
3.1.1.2 PTF models
In contrast to QSAR, PTF theory considers that correlational associations among soil properties have explanatory implications for other soil properties (Sidoli et al., 2016; Singh et al., 2016; De Gerónimo et al., 2018; Conde-Cid et al., 2020). Therefore, sorption coefficients of one pollutant among different soils must depend on soil properties.
In the literature, different sorption mechanisms and trends have been found for the same pollutant, e.g., a major and a minor role of OC in the sorption of oxytetracycline and chlortetracycline across different soils (Conde-Cid et al., 2020) or non-hydrophobic sorption mechanisms (Dollinger et al., 2015; Kodešová et al., 2015; Sidoli et al., 2016; De Gerónimo et al., 2018; Klement et al., 2018), in accordance with the PTF theory.
Additionally, the outcome selection process was linked to the evaluation of sorption linearity during the data treatment (Kodešová et al., 2015; Sidoli et al., 2016; Klement et al., 2018) and the analysis of predictor variables related to the environmental and methodological conditions, such as tillage (Singh et al., 2016) or the presence of phosphate (Sidoli et al., 2016) in agricultural soils.
In this sense, PTF models studied one pollutant at a time, including pesticides (Dollinger et al., 2015; Sidoli et al., 2016; Singh et al., 2016; De Gerónimo et al., 2018), pharmaceuticals (Kodešová et al., 2015; Klement et al., 2018), and antibiotics (Conde-Cid et al., 2019; Conde-Cid et al., 2020; Hu et al., 2022), generally ionizable (Dollinger et al., 2015; Kodešová et al., 2015; Sidoli et al., 2016; De Gerónimo et al., 2018; Klement et al., 2018; Conde-Cid et al., 2019; Conde-Cid et al., 2020; Hu et al., 2022), and using non-normalized sorption coefficients (
3.1.1.3 Key insights
We found different strategies that were employed to simplify the sorption process. QSAR models considered the sorption coefficient as a physicochemical property of pollutants, independent of soils and are therefore valid for all soils, simplifying the outcome selection (P1, Figure 3). PTF models evaluated one pollutant per model, considering that the sorption coefficient is a soil property. This implies that QSAR models, when applicable, are broad and general, while PTF models tend to be specific, acquiring local relevance. Figure 4 shows conditions and assumptions that we propose to understand both kinds of predictive models.
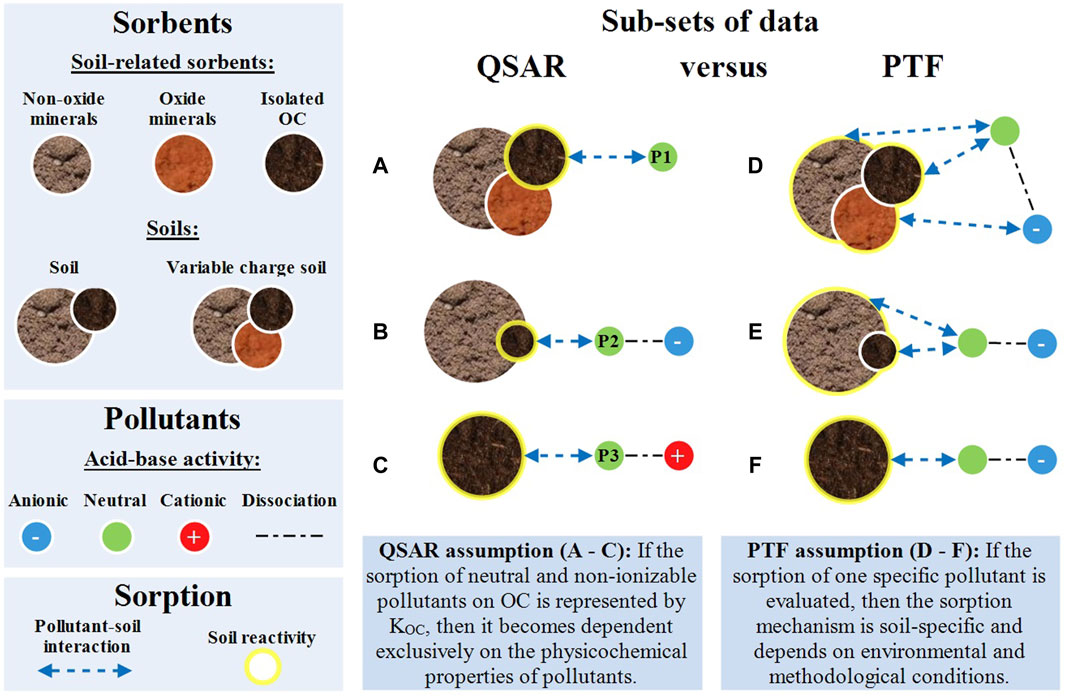
Figure 4. Assumptions considered during the development of predictive models. QSAR examples (A–C) represent the sorption of the neutral form of three different pollutants on soils, specifically on the OC fraction. PTF examples (D–F) represent diverse pollutant-soil interactions for the same hypothetical pollutant on different soils.
3.1.2 Data collection and treatment
3.1.2.1 QSAR models
Physicochemical information of sorbents was typically not included in QSAR studies (Wang et al., 2015; Aranda et al., 2016; Rybacka and Andersson, 2016; Berthod et al., 2017; Daré et al., 2017; Olguin et al., 2017; Sabour and Moftakhari Anasori Movahed, 2017; Zhu et al., 2017; Zhang et al., 2018; Cai et al., 2019; Olguin et al., 2019; Kobayashi et al., 2020; Kobayashi and Yoshida, 2021; Muhire et al., 2021; Pandey and Roy, 2021; Cantwell et al., 2022; Jiang et al., 2022). Additionally, methodological conditions used to obtain the experimental sorption coefficient values were neglected during the development of the datasets. Moreover, some sorption coefficients were theoretically derived from other predictive models using the octanol/water partition coefficient (
The previous findings impact the applicability of QSAR models in two ways: (i) empirical values were used together, independent of their quantification method (e.g., one-point vs. isotherm, batch vs. field-based) or sorption trend (e.g., sorption isotherm linearity); and (ii) datasets in the considered literature were built using mean or median sorption coefficient values such that the amount of data is equivalent to the number of pollutants and soil variability was part of the experimental error. Both issues increase the uncertainty when trying to assess the representational value of data for reliable predictions.
Furthermore, findings impact the reproducibility of QSAR models: (i) only three QSAR models provided the units of sorption coefficients (Wang et al., 2015; Rybacka and Andersson, 2016; Berthod et al., 2017), necessary to evaluate the comparability among empirical values obtained from different studies due to possible changes in the isotherm shape (linearity,
3.1.2.2 PTF models
In contrast to QSAR, articles concerning PTF included the description of the site and procedure for soil sampling, with most of the sorbents being agricultural soils. Generally speaking, soil samples were superficial, from 0 to 5 (De Gerónimo et al., 2018), 20 (Conde-Cid et al., 2019; Conde-Cid et al., 2020) or 25 cm depth (Kodešová et al., 2015; Klement et al., 2018), with few cases addressing the spatial variability (Kodešová et al., 2015; Singh et al., 2016). In some cases, confounding variables were minimized using soils without application of the pollutants or fertilizers in previous years (De Gerónimo et al., 2018) or by evaluating the presence of pollutants before the sorption study (Conde-Cid et al., 2020).
Additionally, methodological and environmental conditions such as soil:solution ratio, interval of concentrations, background electrolyte, temperature, and contact and equilibrium time impacted the magnitude and interpretation of sorption coefficients and therefore were included during the data collection and treatment. For instance, the selection of the interval of concentrations or the soil:solution ratio impacted the isotherm shape (Dollinger et al., 2015), with several nonlinear sorption isotherms fitting to the Freundlich model (Dollinger et al., 2015; Kodešová et al., 2015; Sidoli et al., 2016; De Gerónimo et al., 2018; Klement et al., 2018; Conde-Cid et al., 2019; Conde-Cid et al., 2020; Hu et al., 2022), including the prediction of the linearity coefficient (
All sorption coefficients used in PTF models were quantified experimentally. Most of the PTF studies produced their own data using the same methodological conditions (Kodešová et al., 2015; Sidoli et al., 2016; Singh et al., 2016; De Gerónimo et al., 2018; Klement et al., 2018; Conde-Cid et al., 2019; Conde-Cid et al., 2020). Only in two cases data were collected from other studies (Dollinger et al., 2015; Hu et al., 2022), where methodological differences among studies were included as predictor variables and analyzed during the mechanistic interpretation of predictive models. Additionally, data treatment included a homogenization step when (i) sorption was approximately linear, so
Another homogenization technique involved the simplification of the sorption mechanisms by minimizing the variability (i) between pollutants, e.g., using pH-dependent pollutants at similar pH values among soils (Conde-Cid et al., 2019) or avoiding mixtures between ionic and non-ionic forms (Sidoli et al., 2016); or (ii) between sorbents, e.g., using unmodified soils or sediments with OC < 20% and comparable background electrolytes (Dollinger et al., 2015).
3.1.2.3 Key insights
QSAR models were focused on a common sorption mechanism applicable through several soils, while PTF models considered local variability and specific sorption mechanisms, in accordance with our proposed assumptions (Figure 4). This affected the data collection and treatment. For QSAR models, data lack methodological and environmental context because they are assumed as independent of soils (e.g., methodological conditions such as soil:solution ratio could be perceived as soil variability and therefore irrelevant). On the other hand, PTF models implemented a highly detailed procedure, including (i) description of soils, (ii) impacts of methodological and environmental conditions, and (iii) classification and treatment of data in agreement with the previous steps.
3.1.3 Predictor variables
3.1.3.1 QSAR models
Only molecular properties were used by QSAR models to predict sorption coefficients. Those predictor variables, called “descriptors” in QSAR models, were generally related to hydrophobicity, such as
Despite the previously described procedure, (i) hydrophobicity was in some cases inadequate for representing the sorption of ionic species, affecting the statistical quality of QSAR models (Rybacka and Andersson, 2016; Berthod et al., 2017), and (ii) the use of molecular descriptors (hydrophobic, hydrogen-bonding and charge-related interactions) to address soil variability produced poor predictive ability (Berthod et al., 2017). Both cases support our proposed assumption (Figure 4) as a requisite to apply QSAR models.
3.1.3.2 PTF models
Physicochemical properties of soils were used as predictor variables for PTF models, including environmental and methodological conditions that varied within the dataset. The most common properties were pH in different solutions, OC, soil texture, and cation exchange capacity (CEC). More properties were added to represent specific soil orders or land uses, such as variable charge (e.g., exchangeable aluminum, crystallized and amorphous oxy-hydroxides) (Sidoli et al., 2016; De Gerónimo et al., 2018; Conde-Cid et al., 2019), salinity (e.g., CaCO3 content, hydrolytic and exchangeable acidity, base cation saturation) (Kodešová et al., 2015; Klement et al., 2018), or agricultural context (e.g., total organic N content, available P) (Sidoli et al., 2016; Conde-Cid et al., 2019). Some properties showed statistically significant correlations, such as OC with CEC and clay content with iron and aluminum oxides (De Gerónimo et al., 2018). Since these correlations depend on soils they cannot be generalized. Additionally, the quantification method varied among articles. In these cases, different methodological conditions within a dataset were (i) tested as descriptors, e.g., soil:solution ratio and maximum initial concentration of pollutants (Dollinger et al., 2015; Hu et al., 2022) or (ii) used as part of the data splitting (Dollinger et al., 2015).
3.1.3.3 Key insights
According to our proposed assumptions (Figure 4), if the sorption mechanism is independent of soils, then it is not necessary to contextualize predictor variables or include soil descriptors. This explains why QSAR models generally follow an a posteriori approach, where a massive pool of molecular descriptors is used, and the mechanistic explanation is derived from the algorithmically selected descriptors to represent the outcome. On the other hand, PTF models explicitly address the complexity of the sorption process, following an a priori approach, where they explain the conceptual background of the sorption process and consequently propose a few predictor variables to develop predictive models.
3.1.4 Model equation
3.1.4.1 QSAR models
Databases were split into a training and a test set, used to develop the QSAR model and assess its predictive performance, respectively. In this sense, data splitting helped to evaluate the reliability of QSAR models when applied to external data, providing an estimate of the performance for new pollutants. Ideally, data are uniformly distributed between training and test set. In the literature, the process was random (Wang et al., 2015; Berthod et al., 2017; Sabour and Moftakhari Anasori Movahed, 2017; Muhire et al., 2021; Cantwell et al., 2022; Jiang et al., 2022) or rational, depending on the splitting algorithm, where Y-ranking is the most frequent approach (Zhu et al., 2017; Cai et al., 2019; Olguin et al., 2019; Kobayashi and Yoshida, 2021). Considered training:test set ratios include 14:86 (Aranda et al., 2016), 66:33 (Olguin et al., 2017; Olguin et al., 2019; Kobayashi and Yoshida, 2021; Cantwell et al., 2022), 70:30 (Sabour and Moftakhari Anasori Movahed, 2017), 75:25 (Wang et al., 2015; Daré et al., 2017; Muhire et al., 2021; Pandey and Roy, 2021), 80:20 (Berthod et al., 2017; Zhu et al., 2017; Cai et al., 2019; Pandey and Roy, 2021; Jiang et al., 2022), and 88:12 (Kobayashi et al., 2020). In one case, the effect of dataset size was also evaluated, showing that the division of the training set (N = 643) into eight subsets (N = 79 - 81) produced equivalent QSAR models to those for the whole dataset (Olguin et al., 2019).
Some articles applied mechanistic criteria for splitting, such as the development of different QSAR models based on the whole dataset and specific chemical classes (aliphatic groups, monoaromatic hydrocarbons, diphenyl ethers, polyaromatic hydrocarbons and plant protection products), with their respective training and test sets (Pandey and Roy, 2021), the use of different test sets based on intervals of low, medium and high sorption coefficient values (Daré et al., 2017), the use of several training and test sets to evaluate the stability of the mathematical equation and its predictability (Berthod et al., 2017), and the development of QSAR models based on the charge of the pollutants (neutral, positively, and negatively charged) (Rybacka and Andersson, 2016; Berthod et al., 2017).
Different algorithms were used to develop the QSAR models, most of them assuming a linear relationship between descriptors and outcome, such as multiple linear regression (MLR) (Wang et al., 2015; Aranda et al., 2016; Berthod et al., 2017; Kobayashi et al., 2020; Kobayashi and Yoshida, 2021; Pandey and Roy, 2021; Jiang et al., 2022), partial least squares regression (PLSR) (Rybacka and Andersson, 2016; Berthod et al., 2017; Daré et al., 2017; Zhu et al., 2017; Cai et al., 2019; Pandey and Roy, 2021), support vector machines (SVM) (Kobayashi et al., 2020; Kobayashi and Yoshida, 2021), and univariate linear regression (ULR) (Berthod et al., 2017; Olguin et al., 2017; Zhang et al., 2018; Olguin et al., 2019). Nonlinear models included gradient boosting decision tree (Kobayashi et al., 2020; Kobayashi and Yoshida, 2021) and neural network-based models (Berthod et al., 2017; Sabour and Moftakhari Anasori Movahed, 2017) algorithms.
MLR was the most frequently used algorithm due to the simple mechanistic explanation derived from those QSAR models. However, previous treatments were required due to the large number of descriptors, e.g., stepwise selection (Wang et al., 2015; Berthod et al., 2017; Pandey and Roy, 2021; Jiang et al., 2022), best subset selection (Pandey and Roy, 2021) and replacement method (Aranda et al., 2016). Additionally, PLSR was useful for dimensionality reduction and to avoid multicollinearity (Zhu et al., 2017; Cai et al., 2019; Pandey and Roy, 2021). On the other hand, nonlinear regressions were able to represent complex relationships between sorption coefficients and descriptors. Finally, ULR was used to assess simple relationships between sorption coefficients and hydrophobicity.
3.1.4.2 PTF models
Data splitting was always related to mechanistic issues, such as the use of different outcomes (Hu et al., 2022), the use of one pollutant per model (Kodešová et al., 2015; Sidoli et al., 2016; Singh et al., 2016; Klement et al., 2018; Conde-Cid et al., 2020) and/or per site or plot from which the data were obtained (Singh et al., 2016), and the methodological conditions and available information about specific physicochemical properties (Dollinger et al., 2015). However, only three articles included training and test sets (Singh et al., 2016; Conde-Cid et al., 2019; Hu et al., 2022), negatively affecting the validation of most of the PTF models.
Algorithms for developing PTF models included MLR (Dollinger et al., 2015; Kodešová et al., 2015; Sidoli et al., 2016; De Gerónimo et al., 2018; Klement et al., 2018; Conde-Cid et al., 2019; Conde-Cid et al., 2020; Hu et al., 2022) with stepwise selection (Dollinger et al., 2015; Sidoli et al., 2016; De Gerónimo et al., 2018), PLSR (Singh et al., 2016) and nonlinear algorithms (Sidoli et al., 2016). One study used principal component analysis (PCA) to guide the interpretation of descriptors for the MLR (De Gerónimo et al., 2018). Some MLR algorithms included the transformation of physicochemical soil properties that did not follow a normal distribution (Conde-Cid et al., 2020), or the use of exponential relationships between sorption coefficients and predictor variables to improve the statistical quality (from R2 < 0.75 to > 0.92) but changing the mechanistic interpretation (Sidoli et al., 2016).
3.1.4.3 Key insights
Data splitting preceded the generation of a mathematical equation. This had a statistical explanation in QSAR models (i.e., reliability by using training and test sets), with a few articles including characteristics of pollutants. The procedure and the use of several explicit algorithms for developing the QSAR models agrees with the OECD principle P2 (Figure 3). Inversely, PTF models lacked a statistically validated procedure but evaluated diversity and complexity of data and used the data splitting to better represent sorption mechanism, according to pollutant-soil interactions. This procedure is not included in QSAR models because the sorption mechanism was assumed as generalizable and independent of soils (Figure 4).
3.1.5 Model validation
3.1.5.1 QSAR models
The validation of QSAR models was based on the OECD principle P4 (Wang et al., 2015; Sabour and Moftakhari Anasori Movahed, 2017; Kobayashi et al., 2020; Kobayashi and Yoshida, 2021), considering several statistical parameters and procedures classified in two steps: internal validation (training set) related to the goodness-of-fit and robustness, and external validation (test set) to evaluate the predictive performance.
Common statistical parameters for goodness-of-fit included R2 (Berthod et al., 2017; Daré et al., 2017; Olguin et al., 2017; Sabour and Moftakhari Anasori Movahed, 2017; Zhu et al., 2017; Zhang et al., 2018; Cai et al., 2019; Olguin et al., 2019; Kobayashi et al., 2020; Kobayashi and Yoshida, 2021; Muhire et al., 2021; Pandey and Roy, 2021; Cantwell et al., 2022) and adjusted R2 (Wang et al., 2015; Muhire et al., 2021; Jiang et al., 2022), F-Test value (Zhang et al., 2018; Muhire et al., 2021; Cantwell et al., 2022), standard error of the estimate (Zhu et al., 2017; Zhang et al., 2018; Cai et al., 2019; Kobayashi et al., 2020; Cantwell et al., 2022), residual sum of squares (Olguin et al., 2017; Kobayashi et al., 2020), root mean square error (RMSE) (Wang et al., 2015; Daré et al., 2017; Olguin et al., 2017; Sabour and Moftakhari Anasori Movahed, 2017; Olguin et al., 2019; Kobayashi and Yoshida, 2021; Muhire et al., 2021; Jiang et al., 2022), p-value of descriptors contained in the QSAR model (Zhu et al., 2017; Cai et al., 2019; Muhire et al., 2021; Jiang et al., 2022), variance inflation coefficient to evaluate multicollinearity (Jiang et al., 2022), mean absolute error (Pandey and Roy, 2021), and concordance correlation coefficient (CCC) (Olguin et al., 2017; Sabour and Moftakhari Anasori Movahed, 2017; Olguin et al., 2019; Kobayashi and Yoshida, 2021).
Robustness was typically addressed through one of the following procedures: “leave-one-out” cross-validation (Wang et al., 2015; Aranda et al., 2016; Berthod et al., 2017; Daré et al., 2017; Olguin et al., 2017; Sabour and Moftakhari Anasori Movahed, 2017; Zhang et al., 2018; Cai et al., 2019; Olguin et al., 2019; Kobayashi et al., 2020; Kobayashi and Yoshida, 2021; Muhire et al., 2021; Pandey and Roy, 2021; Cantwell et al., 2022; Jiang et al., 2022), “leave-many-out” cross-validation (Olguin et al., 2017; Olguin et al., 2019), and bootstrapping (Wang et al., 2015). Additionally, Y-scrambling was used to detect or discard chance correlations (Aranda et al., 2016; Daré et al., 2017; Olguin et al., 2017; Sabour and Moftakhari Anasori Movahed, 2017; Zhang et al., 2018; Olguin et al., 2019; Kobayashi and Yoshida, 2021; Muhire et al., 2021; Pandey and Roy, 2021). Other statistical parameters such as RMSE and CCC for the internal validation were also used (Daré et al., 2017; Olguin et al., 2017; Zhang et al., 2018; Olguin et al., 2019; Kobayashi and Yoshida, 2021; Muhire et al., 2021).
External validation was evaluated through different indicators, such as variance explained in external prediction (Wang et al., 2015; Daré et al., 2017; Olguin et al., 2017; Sabour and Moftakhari Anasori Movahed, 2017; Zhu et al., 2017; Zhang et al., 2018; Cai et al., 2019; Olguin et al., 2019; Kobayashi and Yoshida, 2021; Muhire et al., 2021; Pandey and Roy, 2021; Cantwell et al., 2022; Jiang et al., 2022), modified coefficient of determination of the external validation (Daré et al., 2017; Olguin et al., 2017; Sabour and Moftakhari Anasori Movahed, 2017; Zhang et al., 2018; Olguin et al., 2019; Kobayashi et al., 2020; Muhire et al., 2021; Pandey and Roy, 2021), standard error of prediction (Zhu et al., 2017; Cai et al., 2019; Cantwell et al., 2022), RMSE of validation (Wang et al., 2015; Sabour and Moftakhari Anasori Movahed, 2017; Zhang et al., 2018; Olguin et al., 2019; Kobayashi and Yoshida, 2021; Muhire et al., 2021), external CCC (Zhang et al., 2018; Olguin et al., 2019; Kobayashi and Yoshida, 2021), and mean absolute error (Pandey and Roy, 2021).
3.1.5.2 PTF models
Validation of PTF models was scarce and focused on goodness-of-fit, using R2 or adjusted R2 (Kodešová et al., 2015; Sidoli et al., 2016; Singh et al., 2016; Klement et al., 2018; Conde-Cid et al., 2019; Conde-Cid et al., 2020), p-value (Kodešová et al., 2015; Klement et al., 2018; Conde-Cid et al., 2019) and standard error (Singh et al., 2016), but not including an analysis of overfitting (e.g., observation:descriptor ratio). Two articles assessed the model robustness through cross-validation (Dollinger et al., 2015) and bootstrap methods (De Gerónimo et al., 2018). Articles that considered validation sets followed different approaches to validate their models: (i) developing the PTF model using the training set but quantifying R2, Nash-Sutcliffe efficiency, RMSE, and absolute error only for the validation set (Hu et al., 2022), thereby causing the model to lack goodness-of-fit and robustness, (ii) plotting the measured vs. estimated sorption coefficient values for the training and test set and checking how many data fell within the interval of the measured value
3.1.5.3 Key insights
QSAR models present a higher statistical quality than PFT models, due to the application of training and test sets, the high number of statistical parameters applied to the data, and their consistency among different studies. This is not related to our proposed assumptions (Figure 4) but the institutionalization of QSAR models with respect to PTFs (P2 and P4, Figure 3).
3.1.6 Applicability of predictive models
3.1.6.1 QSAR models
The applicability domain (AD) is a theoretical chemical space where QSAR models make reliable predictions (Kobayashi et al., 2020; Kobayashi and Yoshida, 2021). This region is defined by the diversity of pollutants (molecular structures) in the training set and the descriptors that are used to predict their endpoints (Aranda et al., 2016; Zhu et al., 2017). The AD is specific to each QSAR model, and reliability is only possible to assess for molecules and properties that fall within this chemical space, based on similarity (Daré et al., 2017; Zhu et al., 2017). Otherwise, a prediction is an unreliable model extrapolation (Aranda et al., 2016; Zhu et al., 2017). Without an explicit definition of the AD, a predictive model does not meet the OECD principle P3 (Zhang et al., 2018).
AD was quantified by standardization (Wang et al., 2015; Daré et al., 2017; Sabour and Moftakhari Anasori Movahed, 2017; Zhu et al., 2017; Zhang et al., 2018; Cai et al., 2019; Olguin et al., 2019; Kobayashi et al., 2020; Kobayashi and Yoshida, 2021; Pandey and Roy, 2021), leverage (Wang et al., 2015; Aranda et al., 2016; Daré et al., 2017; Sabour and Moftakhari Anasori Movahed, 2017; Zhu et al., 2017; Zhang et al., 2018; Cai et al., 2019; Olguin et al., 2019; Muhire et al., 2021), Euclidean distance (Kobayashi et al., 2020; Kobayashi and Yoshida, 2021), and one-class support vector machines (Kobayashi and Yoshida, 2021). These methods were applied to detect outliers (usually defined as > 3.0σ from the mean) and/or influential points (leverage > threshold). AD was commonly visualized through a Williams plot considering the standardized cross-validated residuals versus leverage values of pollutants (Wang et al., 2015; Sabour and Moftakhari Anasori Movahed, 2017; Zhu et al., 2017; Zhang et al., 2018; Cai et al., 2019; Olguin et al., 2019; Muhire et al., 2021; Jiang et al., 2022). Here, outliers and influential points were molecular structures, and their analysis was based on molecular descriptors, delimiting the scope and interpretability of QSAR models (Cai et al., 2019; Jiang et al., 2022).
3.1.6.2 PTF models
The considered PTF models did not include an AD. Therefore, PTF model applicability was not statistically validated.
3.1.6.3 Key insights
QSAR models presented a clearly defined AD, guided by the OECD (P3, Figure 3). AD was independent of methodological or environmental conditions from which the empirical values are obtained, which fits with the QSAR assumption that we proposed (Figure 4) and impacts the OECD principle P5 (Figure 3). On the other hand, PTF models lack a defined AD but used soil physicochemical properties and methodological conditions as predictor variables, implying that their AD would be defined by the diversity of pollutant-soil interactions, whose interpretability depends on soils and local conditions.
3.1.7 Regulatory purposes
3.1.7.1 QSAR models
In general, QSAR models were considered as successful for estimating sorption coefficients and were applied to environmental risk assessment (Berthod et al., 2017; Sabour and Moftakhari Anasori Movahed, 2017; Zhang et al., 2018; Cai et al., 2019; Kobayashi et al., 2020; Kobayashi and Yoshida, 2021; Muhire et al., 2021; Jiang et al., 2022), helping the design of new molecules with less environmental impact (Sabour and Moftakhari Anasori Movahed, 2017; Kobayashi et al., 2020; Muhire et al., 2021; Jiang et al., 2022), providing objective decisions (Kobayashi et al., 2020; Kobayashi and Yoshida, 2021) and assessing soil remediation and potential leaching (Sabour and Moftakhari Anasori Movahed, 2017).
3.1.7.2 PTF models
PTF models were also considered successful and useable for environmental fate and risk assessment (Dollinger et al., 2015; Kodešová et al., 2015; Singh et al., 2016; Hu et al., 2022), and identification of vulnerable soils (Conde-Cid et al., 2020; Hu et al., 2022), helping the developing of mitigation strategies and management practices when necessary (Conde-Cid et al., 2020; Hu et al., 2022). However, the use of PTF models was generally suggested more cautiously than for QSARs. For instance, PTF models were proposed as screening methods for regional approaches if local soils were included (Singh et al., 2016). So, their validity depends on non-soil variables such as the pollutants and environmental conditions (Hu et al., 2022). Furthermore, their descriptors and the interval of initial concentration for calculating sorption coefficients should be verified with new datasets if used on different soils (Dollinger et al., 2015; Kodešová et al., 2015).
3.1.7.3 Key insights
QSAR models can be used for regulatory purposes for any soil at any condition. However, QSAR reliability is restricted to the validity of their assumption (Figure 4), which in turn depends on the main sorption mechanism within the dataset. On the other hand, PTF models represent diverse sorption mechanisms at local scale. In this sense, QSAR and PTF models are not exclusive but complementary.
3.2 Literature describing empirical findings
Here, we describe the main trends found in empirical studies. We focused the results on pesticides attributes and sources of variability explored within the studies, shown per article and pesticide in Tables 2, 3, respectively. Information in Table 2 shows the sources of variability addressed per article, characterization of pesticides (name of pesticides studied per article, targets, acid-base activities, chemical classes, and chemical forms in the study), and main trends found in the study. Additionally, the name of the pesticides is shown in Table 3, including their characterization per pesticide, the articles that studied every pesticide and the characterization of those articles (sum of sources of variability addressed per pesticide).
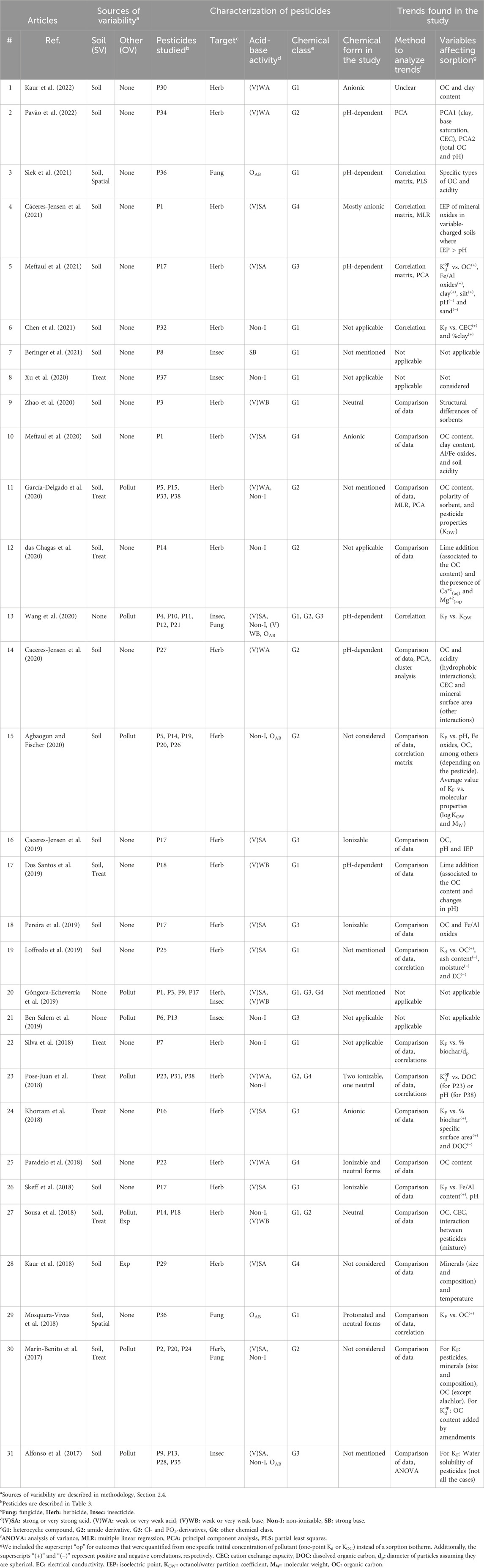
Table 2. Summary of the information extracted from the experimental articles from the literature (presented in the same order as in Supplementary Table S4).
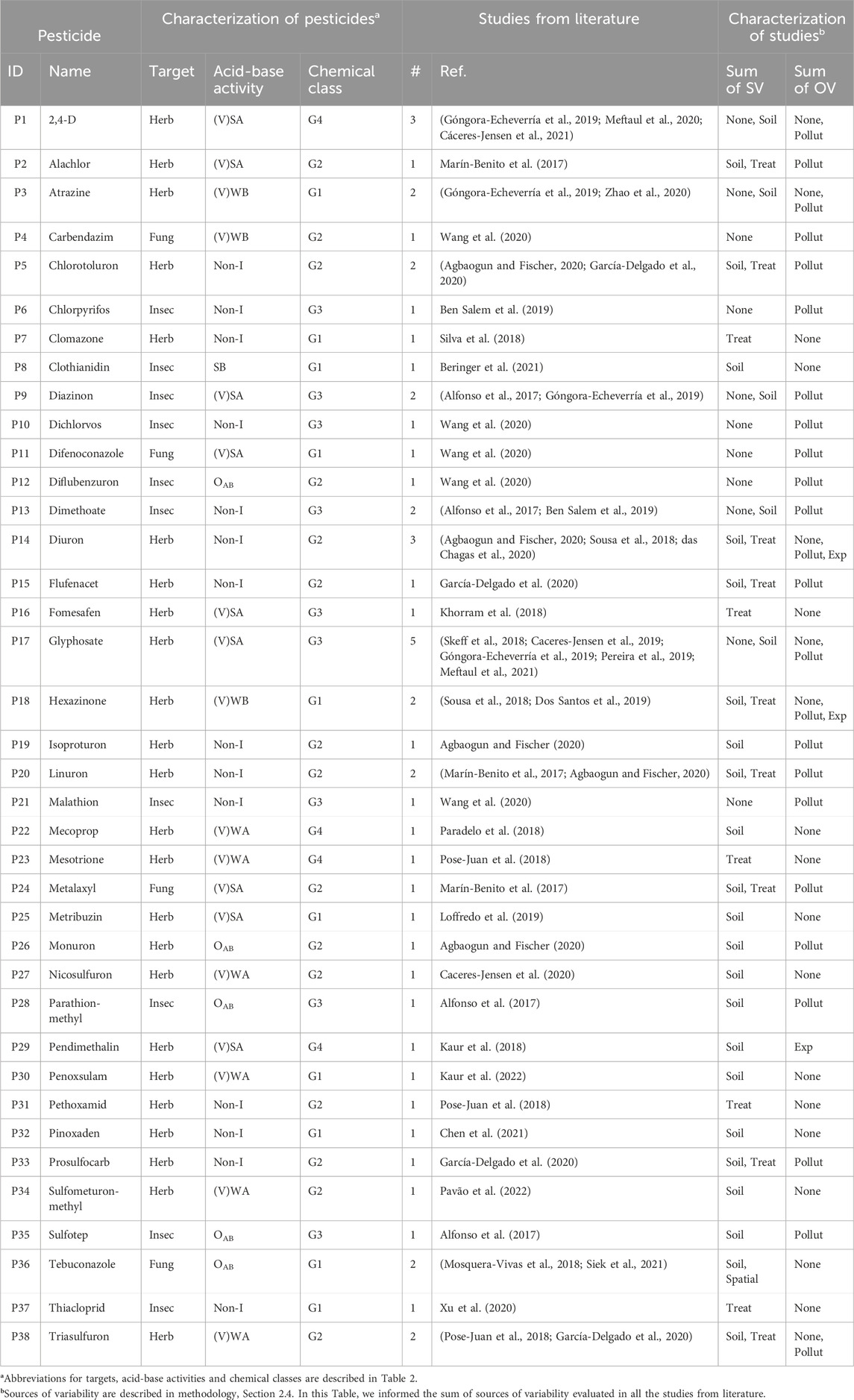
Table 3. Summary of the information about pesticides extracted from the experimental articles from the literature.
3.2.1 Pesticide attributes
3.2.1.1 Target
We identified three pesticide types in the literature: herbicides (N = 24, 63% of studied pesticides), insecticides (N = 10, 26%), and fungicides (N = 4, 11%), which reflects the trend in global usage (De et al., 2014). Among all articles, N = 24 (77%) included herbicides.
3.2.1.2 Acid-base activity
A large percentage of pesticides had no or low pH-dependence: N = 14 (37%) non-ionizable, N = 6 (16%) (very) weak acid, and N = 3 (8%) (very) weak base. Furthermore, acids were more common than bases, with only one article studying a strong base.
3.2.1.3 Chemical class
Heterocyclic compounds and amide derivatives were frequent among pesticides N = 10 (26%) and articles N = 13 (42%).
3.2.1.4 Key insights
A heterogeneous distribution of targets, acid-base activities and chemical classes was found in the literature for articles and pesticides. We related trends to the occurrence of the most frequent pesticides: glyphosate, 2,4-D and diuron (Table 3; Supplementary Figure S2), which are strong acid herbicides and are present in 10 out of 31 articles (32%). These pesticides have been found frequently in water bodies in North America (glyphosate) and Australia (2,4-D and diuron) (Sharma et al., 2019). Furthermore, glyphosate and 2,4-D are commonly used in Argentina, North/Central America, and Africa (Sharma et al., 2019), and studied using PTF models (Dollinger et al., 2015; Sidoli et al., 2016; Singh et al., 2016; De Gerónimo et al., 2018).
3.2.2 Sources of variability
Some of the 31 articles studied one source of variability, while others addressed combinations. Their distribution is shown in Figure 5.
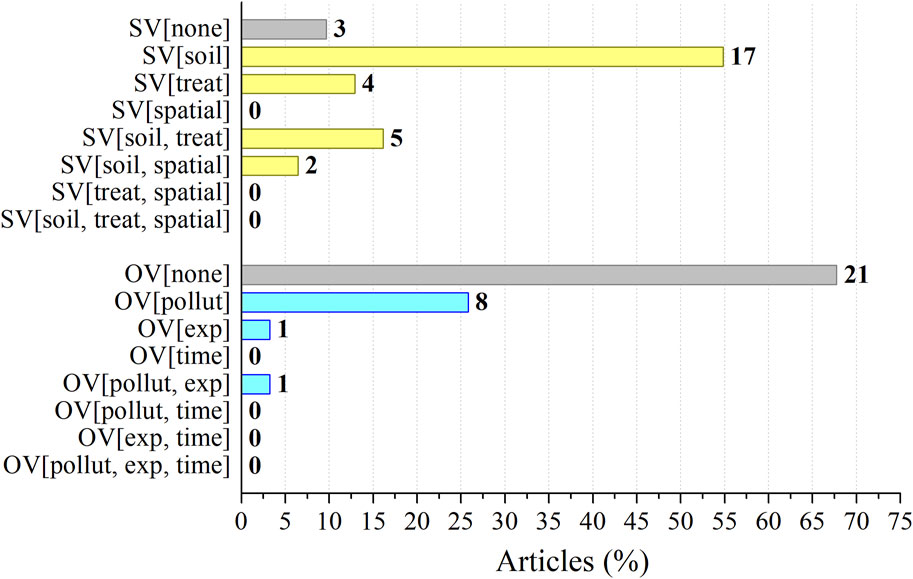
Figure 5. Soil variability (SV, yellow) and other sources of variability (OV, light blue) studied in the literature. Gray bars represent a lack of variability (SV [none] and OV [none]). Numbers to the right of each bar represent the number of articles.
In descending order, we found that articles studied (i) SV [soil] > SV [soil, treat] > SV [treat] > SV [none] > SV [soil, spatial] for soil variability, with seven out of 31 articles studying different sources of soil variability together, and (ii) OV [none] > OV [pollut] > OV [exp] = OV [pollut, exp] for other sources of variability, with only one out of 31 articles studying different sources of variability.
3.2.2.1 Complexity and uncertainty
Sources of variability contrasted in complexity: (i) soil variability involved several sorbents, treatments and/or topographic conditions. Samples had different physicochemical properties, so the interpretation of trends may involve multiple potentially valid explanations, including the presence of confounding variables. Then, these studies are useful to represent real scenarios of environmental concern, but their evaluation of sorption mechanisms is limited due to the inherent uncertainty of the experimental design. On the other hand, (ii) other sources of variability generally involved one sorbent, modifying one specific variable at controlled values (e.g., temperature, pH), giving simpler interpretations that are only locally valid but have potential to be extrapolated in future research.
Three different approaches were used to interpret results, based on their complexity and limitations: (i) conceptual comparisons without statistical tools when only two contrasting cases were studied, e.g., two soils, treatments, or pollutants; (ii) correlations between selected physicochemical properties of sorbents or pollutants (Khorram et al., 2018; Pose-Juan et al., 2018; Silva et al., 2018; Ben Salem et al., 2019; Góngora-Echeverría et al., 2019; Wang et al., 2020; Xu et al., 2020); and (iii) statistical tools applied to all possible variables with the purpose of reducing uncertainty when interpreting experimental studies. Common statistical tools were similar to those used in predictive models: PCA (Caceres-Jensen et al., 2020; García-Delgado et al., 2020; Meftaul et al., 2021; Pavão et al., 2022), regression models (García-Delgado et al., 2020; Cáceres-Jensen et al., 2021; Siek et al., 2021), cluster analysis (Caceres-Jensen et al., 2020), multivariate ANOVA analysis (Alfonso et al., 2017), and correlation matrices between (i) sorption coefficients and physicochemical properties (Mosquera-Vivas et al., 2018; Loffredo et al., 2019; Agbaogun and Fischer, 2020; Cáceres-Jensen et al., 2021; Chen et al., 2021; Siek et al., 2021), (ii) sorption coefficients and pairwise interactions to represent the effect of the interaction between physicochemical properties on sorption (Cáceres-Jensen et al., 2021), and (iii) physicochemical properties to explore multicollinearity and avoid biased interpretations (Agbaogun and Fischer, 2020; Cáceres-Jensen et al., 2021; Siek et al., 2021).
3.2.2.2 Key insights
Soil variability, especially SV [soil], was more frequent in the literature than other sources of variability (Table 2; Figure 5). Additionally, differences in complexity produced different approaches to interpret results, where the use of statistical tools within studies and comparison among studies affect their reliability and extrapolation.
3.3 Evidence-based analysis of the predictive model assumptions
This section explores the QSAR and PTF assumptions from an empirical perspective to simplify their analysis and discussion in Section 4.
3.3.1 Analysis of QSAR assumptions
Sorption coefficients correlated positively with OC content and composition for non-ionizable or neutral pesticides in articles that investigated SV [soil] (Paradelo et al., 2018; Sousa et al., 2018; Agbaogun and Fischer, 2020; Caceres-Jensen et al., 2020; García-Delgado et al., 2020; Pavão et al., 2022). The same trend was observed for various aromatic pesticides (Mosquera-Vivas et al., 2018; Paradelo et al., 2018; Sousa et al., 2018; Dos Santos et al., 2019; Loffredo et al., 2019; Meftaul et al., 2020; Siek et al., 2021), including a positive correlation between sorption coefficients and exogenous OC such as biochar (Silva et al., 2018). These correlations were associated with hydrophobic and polar sorption mechanisms.
When sorption on OC was exclusively hydrophobic, the previous trend was accompanied for (i) a negative correlation between sorption coefficients and pH for non-ionizable or neutral pesticides (Paradelo et al., 2018; Caceres-Jensen et al., 2020; Pavão et al., 2022), and (ii) a negative or positive correlation between sorption coefficients and solubility or lipophilicity (e.g.,
However, the previous trend is not always valid. For non-ionizable aromatic and heterocyclic compounds, physicochemical properties such as pH (Dos Santos et al., 2019; Siek et al., 2021), CEC (Sousa et al., 2018; Chen et al., 2021), oxide mineral content (Meftaul et al., 2020), size of particles (Silva et al., 2018; Meftaul et al., 2020; Chen et al., 2021), among others (Loffredo et al., 2019; Agbaogun and Fischer, 2020; Chen et al., 2021; das Chagas et al., 2020) were found to be relevant. This was explained by different sorption mechanisms (hydrophobic > polar > others), which may occur together. For example, π-interactions can be hydrophobic (e.g., n-π and π-π stacking of heterocyclic pollutants on aromatic-C from soil OC) (Agbaogun and Fischer, 2020; García-Delgado et al., 2020; Zhao et al., 2020) or polar (e.g., π-π electron-donor-acceptor) (Zhao et al., 2020), and the affinity difference between aromatic and aliphatic interactions may be relevant (García-Delgado et al., 2020). Additionally, the addition of biochar or the presence of competitive sorption induced changes in the isotherm shape (Silva et al., 2018; Sousa et al., 2018) (i.e., linearity coefficients (
In addition, we found four scenarios in the literature where
3.3.1.1 Key insights
The sorption of neutral and non-ionizable pollutants occurs preferably on OC and could be represented by
3.3.2 Analysis of PTF assumptions
The hydrophobic sorption of non-ionizable and neutral pollutants was predicted by PTF models using soil descriptors and always following the same trends: positive and negative correlation with OC and pH, respectively (Kodešová et al., 2015; Singh et al., 2016; Klement et al., 2018; Hu et al., 2022). However, the magnitude of the effect of each descriptor depended on the pollutant.
The sorption of ionic pesticides (anions, cations and zwitterions) in empirical studies in the literature correlated with OC and pH (Skeff et al., 2018; Caceres-Jensen et al., 2019; Loffredo et al., 2019; Meftaul et al., 2020; Meftaul et al., 2021), but observed trends varied among the experimental studies. The same occurred in PTF models, where correlations with OC were positive (Klement et al., 2018; Conde-Cid et al., 2019), negative (Dollinger et al., 2015; Klement et al., 2018) or negligible (Kodešová et al., 2015; Sidoli et al., 2016; De Gerónimo et al., 2018), and other soil variables such as clay content, Fe and Al oxide content, CEC and base-cation saturation were more relevant than OC (Dollinger et al., 2015; Kodešová et al., 2015; Sidoli et al., 2016; De Gerónimo et al., 2018; Klement et al., 2018).
The variability explained by soil or pollutant properties was also variable. In studies including strong acids, the soil variability was negligible (Alfonso et al., 2017) or defined by clay content and specific minerals (Marín-Benito et al., 2017). Additionally, the contribution of minerals, the lack of correlation between sorption coefficients and OC, or the high variability of
The pH-dependent surface charge was a particular soil characteristic impacting the sorption of ionic pesticides. Sorption coefficients of anionic pesticides were explained by soil texture, content of Fe and Al oxides and isoelectric point of soils in experimental studies (Skeff et al., 2018; Caceres-Jensen et al., 2019; Meftaul et al., 2020; Cáceres-Jensen et al., 2021; Meftaul et al., 2021) and PTF models (Sidoli et al., 2016; De Gerónimo et al., 2018). For example, glyphosate sorption was mainly non-hydrophobic and higher in variable charge soils than in permanent charge soils, depending on the isoelectric point and content of Fe and Al oxides (Skeff et al., 2018; Caceres-Jensen et al., 2019; Pereira et al., 2019; Meftaul et al., 2021) (Figure 4D versus 4E). This behavior made
Finally, articles that studied OV [exp] showed that the sorption coefficients and nonlinearity (
3.3.2.1 Key insights
Sorption followed diverse trends depending on the acid-base activity of pollutants and kind of soil (permanent and variable charge soils). PTF models are applicable for predicting hydrophobic sorption, conceptually linked to the QSAR assumption, but soil properties (OC, pH) had different impacts (e.g., correlations) depending on the pollutant. Furthermore, PTF models can represent sorption mechanisms in specific scenarios beyond QSAR assumption.
4 Discussion
In this section we discuss our findings, considering requirements for developing and unifying QSAR and PTF models from an empirical perspective, including recommendations for scientific and regulatory decisions. We used headers to simplify the understanding of our proposals.
4.1 Requirements for developing and applying predictive models
Based on findings from the literature, we found three topics required for applying predictive models for regulatory purposes: (i) an explicit connection between simplification strategies (QSAR and PTF assumptions, Figure 4) and the representational value of data, (ii) tools and procedures for validating predictive models and their applicability, and (iii) practical needs covered by predictive models for regulatory purposes.
4.1.1 Representational value
Production, collection and treatment of data determine their representational value and potential use as evidence of the phenomenon they are intended to represent (Leonelli, 2019). Therefore, QSAR and PTF assumptions (Figure 4; Section 3.1) should be evaluated and validated to minimize biases. For instance, the idea that the hydrophobic sorption mechanism is generalizable (QSAR assumption) might be enhanced by the distribution of acid-base activities (Tables 2, 3), where most of the current information in the literature is focused on sorption mechanisms independent or only slightly dependent on pH. Moreover, most of the pesticides studied were aromatic (Supplementary Figure S1), which mainly present hydrophobic mechanisms despite other interactions (e.g., polar). In this sense, we recommend validating the assumptions based on the empirical findings applied to the dataset used to develop predictive models.
We can further generalize the QSAR assumption to the following statement: “Predictions can be extrapolated among soils when they share an unspecific sorption mechanism occurring in a unique and common soil component”. If that soil component is OC (see Figures 4A–C, F), and the soil variability (including environmental and methodological conditions) is so low that it can be neglected compared to the variability among pollutants, then it is possible to quantify an average sorption coefficient value per pollutant and create QSAR models.
The notion of generalization has three implications: (i) Both QSAR and PTF can share data (see Figures 4C, F, belonging to different kinds of model despite being equivalent), (ii) the generalization of QSAR assumption may help to develop new QSAR models or simplify PTF models, e.g., if the common soil component is different than OC, and (iii) all models (even QSAR) are applicable to soils with properties similar to those used during the data collection and treatment, so soils should necessarily be included in the AD.
4.1.2 Validation tools
The validation of predictive models should consider their representational value and statistical parameters. Both kinds of validation are necessary and complementary, addressing methodological and environmental conditions affecting the sorption coefficients and isotherm shape (as PTF models) and giving the models a statistical and reproducible quality in accordance with the OECD principles (as QSAR models).
Validation should be considered in all steps, such as data splitting (e.g., splitting pure and mixed pollutants into different datasets, and afterwards dividing them again into their respective training and test sets) or selection of predictor variables (e.g., proposing them based on the sources of variability and then evaluating different algorithms to produce the mathematical equation).
For regulatory decisions, the validation process should improve the applicability of predictive models in environmental scenarios of concern with relevant but rarely studied sources or variability, especially in agricultural contexts where (i) commercial formulations may contain mixtures, (ii) pesticides can be added in previously polluted soils, or (iii) the seasonality could produce relevant temperature variations.
From an institutional perspective, only QSAR models are used or promoted as reliable tools (OECD, 2014; Nolte and Ragas, 2017; Chi et al., 2018; Kar et al., 2018; Thomas et al., 2019), probably due to the generalizability and simplicity of these models in comparison with PTF models, which depend on local conditions as well as specific procedures and predictor variables. Following the QSAR assumption (Figure 4), the OECD assumes that sorption coefficients are physicochemical properties of pollutants (Kar et al., 2018) so its principles have more impact on statistical validation than the outcome selection (always
Considering the above, procedures derived from PTF models may strengthen the application of OECD principles P1 and P5 (Figure 3) by giving a mechanistic context, while statistical tools used in QSAR models support the principles P2, P4 and P3. In this sense, future predictive models could combine both practices and be mechanistically and statistically reliable from an institutional perspective.
4.1.3 Usability for regulatory purposes
The AD of predictive models represents the variability boundaries in which the model was built (e.g., structural diversity, methodological and environmental conditions, predictor variables), required to interpolate new cases, avoiding the uncertainty of extrapolations. In this sense, applicability depends on the dataset. However, it is usually impossible to determine sorption mechanisms in real systems. Notably, sorption experiments are made in ideal conditions, where sorption is isolated from competitive processes such as biological (Kaur et al., 2018; Silva et al., 2018; Skeff et al., 2018; Xu et al., 2020; Beringer et al., 2021; Chen et al., 2021; Siek et al., 2021) or chemical degradation (Góngora-Echeverría et al., 2019; Loffredo et al., 2019; Zhao et al., 2020; Beringer et al., 2021). Furthermore, methodological conditions are not representative of real conditions, such as soil:solution ratio <1 (saturated soil), the usage of a background solution in all studies, controlled pH and temperature (e.g., OV [exp]), or the scarce connection between the interval of concentrations used to quantify sorption coefficients and the field dosages (only done in (Pavão et al., 2022; Mosquera-Vivas et al., 2018; Dos Santos et al., 2019; das Chagas et al., 2020. Predictive models should therefore be used cautiously. What they represent is useful for researchers but not necessarily reflective of regulatory needs, meaning that a clearly defined AD (P3, Figure 3) is necessary but not sufficient to ensure regulatory purposes.
The representational value is key to connect data with real scenarios and establish the correct questions that predictive models can answer for making decisions (Figure 1). For example, it is inappropriate to use sorption coefficients (quantified in equilibrium condition) to represent non-equilibrium scenarios such as environmental fate at non-saturated or variably saturated conditions, e.g., during irrigation, heavy rain, or flooding. However, scientists and environmental entities could use those sorption coefficients to estimate the maximum sorption (at equilibrium) and then the minimum transport of pollutants in ideal conditions. Furthermore, the relevance of sorption with respect to transport in the long term may help to understand the implications of using predictive models for sorption in a specific site or situation.
Additionally, the usability of predictive models depends on their simplicity when used by non-experts, especially when they present predictor variables that are easy to understand and quantify (Berthod et al., 2017; Conde-Cid et al., 2020). If QSAR and PTF models are equally applicable in a hypothetical situation, then QSAR models are easy to implement due to the use of molecular and theoretical descriptors, whose quantification is independent of local conditions, while PTF models have simpler interpretations due to their contextualized predictor variables, helping to understand the meaning of predictions.
4.2 Unifying QSAR and PTF models backgrounds
Following from the above, it is difficult to know a priori how complex the sorption is, and it is difficult to determine sorption mechanisms when several pollutant-soil interactions are possible (see Figures 4D, E). In more complex scenarios, even interactions among soil components or pollutants, such as OC-oxide minerals and multilayer sorption (linearity coefficient
C1. A positive correlation between the sorption coefficient and the percentage of the neutral (uncharged) form of pollutants (100% if the pollutant is non-ionizable).
C2. An exclusive and positive correlation between sorption coefficients and OC (i.e., lack of correlation with other soil properties).
C3. Positive correlations between hydrophobic OC components and sorption coefficients.
4.2.1 Interpretation
These three previous correlations are presented from general to specific. We initially assume that any sorption trend is possible within a dataset. Then, we may simplify the development of predictive models without losing representational value depending on the findings about C1, C2 and C3. If C1 is true, then the sorption is mainly non-ionic (see Figures 4A–C, E, F). If C2 is true, then OC is the most relevant sorbent (see Figures 4A–C, F). Finally, if C3 is also true, then sorption may be represented as hydrophobic on OC (impossible to determine from Figure 4, because it involves the OC composition).
In this sense, an agreement with C1, C2 and C3 represents the hydrophobic sorption assumed in QSAR models, while the non-compliance with any correlation involves the relevance of non-hydrophobic sorption mechanisms, which account for mechanistic diversity (i.e., evidence of several kinds of pollutant-sorbent interaction) instead of fixed statements (QSAR and PTF assumptions, Figure 4). Additionally, these correlations are sensitive to different acid-base activities, chemical classes and soils. Finally, they may be validated experimentally through (i) the changes in sorption of pollutants on each soil at different pH values (C1), (ii) sorption observed in isolated non-organic components of soils and detection of multicollinearity with OC in case other correlations are detected (C2), and (iii) sorption trends on isolated specific OC components, e.g., aliphatic-C, aromatic-C (C3).
Note that C1 and C3 can be fulfilled without C2. This case implies that (i) the main sorbent is OC, but the correlations are hidden by changes in the OC composition among sorbents or interactions between OC and other soil components (e.g., Figures 4C, F versus Figure 4A, B if OC interacts with minerals), or (ii) other sorption mechanisms are relevant, but were eliminated during the experimental procedure to assess C3 (Figure 4E could potentially be an example). To minimize this or other sources of uncertainty or misrepresentations of the sorption coefficient, the corroboration of correlations should follow the specific order: first C1, then C2, and finally C3.
4.2.2 Connection with predictive models
If C1, C2 and C3 are true, then OV [pollut] is sufficient to represent the variability among sorption coefficients (i.e., SV [soil], SV [treat], SV [spatial], and OV [exp] are negligible in the dataset). Therefore, QSAR models are valid approaches to represent the variability within the dataset. On the other hand, PTF models are representative of the dataset if OV [pollut] is negligible (e.g., only one pollutant is analyzed) and SV [soil], SV [spatial], OV [exp] and SV [treat] can be fully explained by physicochemical soil properties. Finally, researchers should use PTF models or hybrid models including soil and pollutant properties as predictor variables when correlations C1, C2 and C3 are false or partially true.
4.2.3 Conditions
Model development (Figure 1) should consider whether the data meet the correlations C1, C2 and C3 to decide an adequate strategy (QSAR, PTF). Afterwards, outcome selection, data treatment, proposal of predictor variables and AD should include the following empirical information of the studies used for obtaining the data: (i) physicochemical properties of pollutants and soils, (ii) methodological and environmental conditions, and (iii) shape of the isotherm. The third topic is especially relevant for QSAR models (i.e., C1 to C3 are met) built from data with different sorption shapes, so the sorption coefficients associated with one pollutant are similar in magnitude but have different interpretations. Finally, the model implementation (Figure 1) should judge if their scenario of concern is included in the model AD (e.g., inclusion of soil samples from different depths, use of fertilized soils).
4.3 Recommendations
In this section, we propose best practices for developing, interpreting, and using current and future predictive models, especially QSAR models due to their institutionalization.
4.3.1 Using empirical information for developing predictive models
The reliability of predictive models and their connection with representational value of data may improve by using sources of variability and our proposed correlations jointly in various stages of the development of predictive models (Figure 3), helping and complementing OECD and REACH guidelines through the following approaches and steps.
4.3.1.1 Exploring sorption mechanisms
Sources of variability can improve the connection between representational value of data and predictive models when used to (i) explore the correlations C1 to C3 (SV [soil]), (ii) detect properties of pollutants and soils affecting sorption (OV [pollut], SV [soil]), and (iii) describe the impact of methodological and environmental conditions in the sorption process in specific contexts (e.g., climate, agricultural practices; OV [exp], SV [treat]). As a result, empirical findings may guide the selection of suitable approaches (QSAR or PTF), outcomes and predictor variables to avoid misrepresentations (e.g., unexplored or underrepresented but relevant sorption mechanisms).
4.3.1.2 Scoping the model
We suggest having a clear objective according to the expected performance and applicability of predictive models, especially the scale and degree of specificity. For instance, variable charge soils (Figures 4A, D) are not common in the literature and their sorption behavior may be hidden among the most common trends (Figures 4B, C, E, F). However, variable charge soils possess a high agricultural productivity, which makes them relevant from a regulatory perspective, specifically for agriculture-based economies from emerging and developing countries (Caceres-Jensen et al., 2019). Then, a predictive model created from large datasets without a mechanistic treatment of data will have general applicability, making the conventional sorption mechanisms and predictive models less useful to predict and interpret specific and important scenarios (e.g., Figure 4D). In this sense, models that have different scope or address infrequent cases provide information that complements our understanding of the sorption process.
4.3.1.3 Dataset
Predictive models should represent the variability among data in the simplest way possible, considering the available information under comparable conditions. As an example, changes in sorption coefficients of soil samples at different depths (SV [spatial]) or influenced by different treatments (SV [treat]) were generally explained by changes in the physicochemical properties of soils (e.g., OC content (endogenous + exogenous), kind of OC, pH, salinity) (Khorram et al., 2018; Pose-Juan et al., 2018; Silva et al., 2018; García-Delgado et al., 2020; Siek et al., 2021; Mosquera-Vivas et al., 2018; Sousa et al., 2018; Dos Santos et al., 2019; das Chagas et al., 2020; Marín-Benito et al., 2017) (see Table 2). Thus, they behaved as different (but conceptually connected) soils and could be included in the same dataset without requiring predictor variables accounting for depth or treatment information. Nevertheless, the extent of change in the soil properties varied among pesticides (Marín-Benito et al., 2017; Pose-Juan et al., 2018) and soils (Pavão et al., 2022; das Chagas et al., 2020), indicating that specific pesticide-sorbent and soil-amendment interactions were relevant when SV [treat]+OV [pollut] and SV [soil, treat] were addressed, respectively.
4.3.1.4 Data treatment
The data treatment should consider sources of variability together with statistical tools to decide whether the findings are reliable, especially if the same pollutant was addressed at different levels of complexity and uncertainty (e.g., SV [soil] versus OV [exp]). Those cases require a well-defined method to compare studies with different experimental designs to analyze the information and improve our comprehension of the environmental fate.
4.3.1.5 Model performance
Sources of variability are helpful for exploring new predictor variables and improving the AD of models in specific scenarios. The high presence of SV [soil] in the literature may be used to improve the predictability of QSAR models by incorporating soil descriptors based on empirical trends when hydrophobic sorption is dominant but not unique, while SV [treat] and SV [spatial] are helpful for exploring the impact of physicochemical properties of soils on pesticide sorption in agricultural contexts.
4.3.1.6 Scientific interpretation
We recommend explicitly assessing sources of variability within the data and doing so from the simplest to the most complex (e.g., starting with OV [exp], finishing with soil variability). Thus, the first (simple) findings act as a conceptual basis to contextualize and simplify the exploration of later (more complex) trends.
4.3.1.7 Model implementation
Scientific interpretation should guide the decision-making process (Figure 1) when selecting scientific information for regulatory purposes. For example, if the agricultural impact of sorption studies is required to propose, apply or evaluate an environmental policy for soil productivity, then it is necessary to consider SV [spatial] and SV [treat], i.e., models that explicitly included those sources of variability and their interpretation, both related to management practices and application of amendments in agricultural soils. As a result, QSAR and PTF models act as a bridge among scientific and regulatory dimensions, involving complex and diverse decisions to help environmental entities to promote and select strategies for applying adequate models in proper scenarios.
4.3.1.8 Adaptable proposal
Correlations C1 to C3 represent the most probable scenarios we found in the literature, but other simplifications could be applied to non-organic soil components if they are relevant in a subgroup of pollutant-soil systems. To this end, we propose to assess (i) pollutant properties that seem relevant (acid-base activity, chemical class, etc.), (ii) a set of relevant soil components, and (iii) relevant functional groups in the selected soil component. For instance, an alternative sorption mechanism could consider correlations of sorption coefficients and anionic pollutants (alternative C1) when sorption occurs mainly in oxide minerals (alternative C2), specifically in aluminum oxides (alternative C3), which fits with Figure 4D if pollutant-soil interactions involving OC and non-oxide minerals are negligible or less relevant.
4.3.2 How to interpret current QSAR models
It is interesting that current QSAR models are statistically validated and offer mechanistic interpretations, even when their assumptions do not necessarily fit with the empirical findings. We propose three possible explanations (not mutually exclusive) related to (i) subsets of data involving hydrophobic sorption, (ii) the contrast between SV [soil] and OV [pollut], and (iii) overall sorption mechanisms.
4.3.2.1 Subsets of data involving hydrophobic sorption
Let us suppose that non-hydrophobic interactions are relevant (e.g., sorption of glyphosate). In that case, OC and pH do not necessarily correlate with sorption coefficients. Moreover, correlations may be positive or negative depending on the pollutant-soil interaction. However, correlations C1 to C3 might be applicable in a subset of data, given specific experimental conditions (e.g., inclusion of ionizable compounds in their neutral form, quantification of average
From a QSAR perspective, sorption is independent of soils and therefore, QSAR models are assumed to be generalizable (see their assumption, Figure 4). In this case, the extrapolation of the subset of data used for developing the model as if they represent the entire dataset (or available information from the literature) produces a hasty generalization fallacy, with the consequent risk of bias when making decisions in soils whose properties were not considered in the subset of data.
The data selection process may help us to address this issue. For example, a wide interval of
Data selection should be described in terms of soil physicochemical properties instead of minimization of the standard deviation only. Otherwise, this practice may support the belief that sorption is strictly hydrophobic and unexplained variability is attributable to incorrect experimental values instead of other sorption mechanisms (Olguin et al., 2019). In this scenario, the explicit description of mechanistic limitations should help environmental agencies to use predictive models for regulatory purposes in a narrow but valid group of pollutant-soil systems, based on the methodological and environmental conditions used to develop the model.
4.3.2.2 Soil versus pollutant variability
In the literature, both soil and pollutant properties produced changes in sorption coefficients. Furthermore, most of the empirical studies were focused on variability among soils, with only a few addressing different pesticides (SV [soil] versus OV [pollut]) (see Table 2). However, no study contrasted both sources of variability.
We analyzed two studies with non-normalized sorption coefficient values at comparable conditions (R2 ≥ 0.95, same units) addressing SV [soil] and OV [pollut] for >2 soils and >2 pesticides (Agbaogun and Fischer, 2020; García-Delgado et al., 2020).
We calculated five
Another study quantified the sorption of four herbicides in two soils, four amendment materials, and the amended soils (García-Delgado et al., 2020). We quantified two
Considering soils (control + amended), the obtained COV were
The applicability and performance of QSAR models increase when (i) SV [soil] or COV value of
4.3.2.3 Overall sorption mechanisms
A third option is that QSAR models represent unrealistic but useful overall trends using average values as outcomes. Consider the following two studies.
A study quantified the sorption of four herbicides in four Mexican soils (Alfonso et al., 2017). We quantified
The opposite situation occurs when the structural variability produces important changes on sorption. From another study involving five pesticides and 18 soils (Agbaogun and Fischer, 2020), we obtained similar
Similarity among pollutants and soils within the dataset affects their variability and mechanistic interpretation. If sorption is generally represented by one overall sorption mechanism, then the use of average values reduces the variability. Moreover, the normalization of sorption coefficients to relevant soil properties (i.e., correlation C2) makes their variability dependent on pollutant properties (case 2). In this scenario, QSAR models have an interpretation and physicochemical meaning, despite their conceptual issues: they represent a sum of unknown trends with compensatory effects that hide specific sorption mechanisms and methodological differences, showing general trends that do not represent local pollutant-soil interactions but allow to approximate them around a probable value. This might explain the unclear impact of some molecular descriptors when complex sorption mechanisms appear (Rybacka and Andersson, 2016). Therefore, these QSAR models cannot predict sorption coefficients in local contexts and should be useful as exploratory analysis prior to the screening step in risk assessment.
4.4 Limitations
Regarding our findings, the main limitation is related to the heterogeneity of information, affecting the extrapolation of our findings and our conceptual proposal in three ways: (i) sources of variability, (ii) distribution of data, and (iii) scarcity of information.
SV [spatial] and OV [exp] involve the study of several samples, which caused authors to simplify logistics, mainly by using one-point sorption coefficients and/or assuming an equilibrium time of 24 h (Figure 5). As a result, we excluded most of these articles from our review (Figure 5), affecting the confidence of the findings for these sources of variability.
If we consider all combinations in which SV [soil] and OV [pollut] were present (SV [soil], SV [soil, treat], SV [soil, spatial] or SV [soil, treat, spatial] together with OV [pollut], OV [pollut, exp]), we find that <20% of the articles studied SV [soil] and OV [pollut], but they covered >60% of the pesticides (5 articles, 27 pesticides). Therefore, the impact of SV [soil] and OV [pollut] might be overrepresented.
Absent sources of variability such as SV [treat, spatial] and those that involve OV [time] produce uncertainty with regard to the generalizability of simplifications proposed during the data treatment. For instance, we do not know if aging, seasonality, or any other time-dependent source of variability is explained by changes in the physicochemical properties of soils, just like SV [spatial], or have more complex effects on sorption, like SV [treat]. This information might help to understand if predictive models are valid in the long term or require empirical time-dependent descriptors to potentially be used in environmental baseline studies or included in local environmental policies.
Regarding the focus of our analysis, three issues affect the interpretation and extrapolation of our results: (i) selection of pollutants, (ii) correlational analysis of sorption mechanisms, and (iii) strategy to unify QSAR and PTF assumptions.
We used pesticides as a globally relevant organic pollutant model of focus due to their structural diversity and reactivity in combination with the large amount of information available in the literature (Neira-Albornoz et al., 2022). This approach is supported by the equivalent findings from different QSAR models using pesticides versus broader ranges of pollutants (Table 1; Section 3.1). However, predictive models and empirical trends for specific non-pesticides compounds might have a different behavior. An example are pharmaceuticals that generally were pH-dependent (PTF models), while pesticides used to be non-ionic (QSAR and literature), affecting the generalizability of our study.
We based our analysis on correlations and connecting both interpretations from theoretical and experimental studies. However, the distribution of data, different experimental designs and collinearity among molecular and soil properties could produce biases when using correlations in the interpretative layer. Biases also have a social explanation, mainly related to global agricultural needs (e.g., the heterogeneous distribution of pesticide usage and the scarcity of studies made on variable charge soils). In this sense, an exhaustive analysis of the context and validity of the empirical trends on a case-by-case basis should minimize biases and oversimplifications of sorption mechanisms.
We proposed hybrid models involving QSAR and PTF assumptions. Considering the lack of mixed models and the lack of experimental studies combining SV [soil] with OV [pollut], our analysis was qualitative. Future research could include molecular and soil descriptors to quantitatively address the feasibility of our proposal and the improvement in explanatory power (statistically and contextually).
Considering the above, our proposal is a first endeavor to understand the implementation of QSAR and PTF models for decision-making considering the representational value of data and should be tested and adapted in future studies according to new evidence.
5 Conclusion
In this article, we developed a comprehensive contextualization of QSAR and PTF models by evaluating the validity of their assumptions and procedures from an evidence-based perspective using empirical results from the literature. Based on our findings, we proposed the analysis of different (i) requirements, such as the selection of appropriate outcomes and kind of model before developing the model itself, (ii) limitations related to the representational value of data and the simplification strategies followed by QSAR and PTF models, and (iii) applicability conditions at local and global scale (Figure 1). This contextualization involves experimental designs, sources of variability, and methodological procedures used during the quantification of empirical data used in the dataset, whose explicit analysis is key to improve the reliability, interpretation and applicability of predictive models. As a result, our work is intended to help scientists and environmental agencies such as OECD and REACH to (i) adapt the development and use of future predictive models to individual contexts of environmental relevance for regulatory purposes, and (ii) interpret and improve current QSAR and PTF models.
Data availability statement
The original contributions presented in the study are included in the article/Supplementary Material, further inquiries can be directed to the corresponding authors.
Author contributions
AN-A: Conceptualization, Formal Analysis, Investigation, Methodology, Project administration, Validation, Visualization, Writing–original draft, Writing–review and editing. MM-P-M: Validation, Visualization, Writing–review and editing. MG: Validation, Writing–review and editing. AS: Conceptualization, Project administration, Visualization, Writing–review and editing.
Funding
The author(s) declare financial support was received for the research, authorship, and/or publication of this article. AN-A was funded by a ZUKOnnect Fellowship of the Zukunftskolleg, University of Konstanz.
Conflict of interest
The authors declare that the research was conducted in the absence of any commercial or financial relationships that could be construed as a potential conflict of interest.
Publisher’s note
All claims expressed in this article are solely those of the authors and do not necessarily represent those of their affiliated organizations, or those of the publisher, the editors and the reviewers. Any product that may be evaluated in this article, or claim that may be made by its manufacturer, is not guaranteed or endorsed by the publisher.
Supplementary material
The Supplementary Material for this article can be found online at: https://www.frontiersin.org/articles/10.3389/fenvs.2024.1379283/full#supplementary-material
References
Agbaogun, B. K., and Fischer, K. (2020). Adsorption of phenylurea herbicides by tropical soils. Environ. Monit. Assess. 192 (4), 212. doi:10.1007/s10661-020-8160-2
Alfonso, L.-F., Germán, G. V., María del Carmen, P. C., and Hossein, G. (2017). Adsorption of organophosphorus pesticides in tropical soils: the case of karst landscape of northwestern Yucatan. Chemosphere 166, 292–299. doi:10.1016/j.chemosphere.2016.09.109
Aranda, J. F., Garro Martinez, J. C., Castro, E. A., and Duchowicz, P. R. (2016). Conformation-independent QSPR approach for the soil sorption coefficient of heterogeneous compounds. Int. J. Mol. Sci. 17 (8), 1247. doi:10.3390/ijms17081247
Ben Salem, A., Chaabane, H., Caboni, P., Angioni, A., Salghi, R., and Fattouch, S. (2019). Environmental fate of two organophosphorus insecticides in soil microcosms under mediterranean conditions and their effect on soil microbial communities. Soil Sediment Contam. An Int. J. 28 (3), 285–303. doi:10.1080/15320383.2018.1564733
Beringer, C. J., Goyne, K. W., Lerch, R. N., Webb, E. B., and Mengel, D. (2021). Clothianidin decomposition in Missouri wetland soils. J. Environ. Qual. 50 (1), 241–251. doi:10.1002/jeq2.20175
Berthod, L., Whitley, D. C., Roberts, G., Sharpe, A., Greenwood, R., and Mills, G. A. (2017). Quantitative structure-property relationships for predicting sorption of pharmaceuticals to sewage sludge during waste water treatment processes. Sci. Total Environ. 579, 1512–1520. doi:10.1016/j.scitotenv.2016.11.156
Caceres-Jensen, L., Rodriguez-Becerra, J., Escudey, M., Joo-Nagata, J., Villagra, C. A., Dominguez-Vera, V., et al. (2020). Nicosulfuron sorption kinetics and sorption/desorption on volcanic ash-derived soils: proposal of sorption and transport mechanisms. J. Hazard. Mater. 385, 121576. doi:10.1016/j.jhazmat.2019.121576
Cáceres-Jensen, L., Rodríguez-Becerra, J., Garrido, C., Escudey, M., Barrientos, L., Parra-Rivero, J., et al. (2021). Study of sorption kinetics and sorption–desorption models to assess the transport mechanisms of 2,4-dichlorophenoxyacetic acid on volcanic soils. Int. J. Environ. Res. Public Health 18 (12), 6264. doi:10.3390/ijerph18126264
Caceres-Jensen, L., Rodríguez-Becerra, J., Sierra-Rosales, P., Escudey, M., Valdebenito, J., Neira-Albornoz, A., et al. (2019). Electrochemical method to study the environmental behavior of Glyphosate on volcanic soils: proposal of adsorption-desorption and transport mechanisms. J. Hazard. Mater. 379, 120746. doi:10.1016/j.jhazmat.2019.120746
Cai, J., Gu, C., Ti, Q., Liu, C., Bian, Y., Sun, C., et al. (2019). Mechanistic studies of congener-specific adsorption and bioaccumulation of polycyclic aromatic hydrocarbons and phthalates in soil by novel QSARs. Environ. Res. 179, 108838. doi:10.1016/j.envres.2019.108838
Cantwell, C., Song, X., Li, X., and Zhang, B. (2022). Prediction of adsorption capacity and biodegradability of polybrominated diphenyl ethers in soil. Environ. Sci. Pollut. Res. 30, 12207–12222. doi:10.1007/s11356-022-22996-9
Card, M. L., Gomez-Alvarez, V., Lee, W.-H., Lynch, D. G., Orentas, N. S., Lee, M. T., et al. (2017). History of EPI Suite™ and future perspectives on chemical property estimation in US Toxic Substances Control Act new chemical risk assessments. Environ. Sci. Process. Impacts 19 (3), 203–212. doi:10.1039/C7EM00064B
Chen, D., Liu, Z., Han, J., Chen, Y., Zhang, K., and Hu, D. (2021). Dissipation, adsorption–desorption, and potential transformation products of pinoxaden in soil. Biomed. Chromatogr. 35 (7), e5097. doi:10.1002/bmc.5097
Chi, Y., Zhang, H., Huang, Q., Lin, Y., Ye, G., Zhu, H., et al. (2018). Environmental risk assessment of selected organic chemicals based on TOC test and QSAR estimation models. J. Environ. Sci. 64, 23–31. doi:10.1016/j.jes.2016.11.018
Chinen, K., and Malloy, T. (2020). QSAR use in REACH analyses of alternatives to predict human health and environmental toxicity of alternative chemical substances. Integr. Environ. Assess. Manag. 16 (5), 745–760. doi:10.1002/ieam.4264
Conde-Cid, M., Fernández-Calviño, D., Núñez-Delgado, A., Fernández-Sanjurjo, M. J., Arias-Estévez, M., and Álvarez-Rodríguez, E. (2020). Estimation of adsorption/desorption Freundlich's affinity coefficients for oxytetracycline and chlortetracycline from soil properties: experimental data and pedotransfer functions. Ecotoxicol. Environ. Saf. 196, 110584. doi:10.1016/j.ecoenv.2020.110584
Conde-Cid, M., Nóvoa-Muñoz, J. C., Fernández-Sanjurjo, M. J., Núñez-Delgado, A., Álvarez-Rodríguez, E., and Arias-Estévez, M. (2019). Pedotransfer functions to estimate the adsorption and desorption of sulfadiazine in agricultural soils. Sci. Total Environ. 691, 933–942. doi:10.1016/j.scitotenv.2019.07.166
Daré, J. K., Silva, C. F., and Freitas, M. P. (2017). Revealing chemophoric sites in organophosphorus insecticides through the MIA-QSPR modeling of soil sorption data. Ecotoxicol. Environ. Saf. 144, 560–563. doi:10.1016/j.ecoenv.2017.06.072
das Chagas, P. S. F., Souza, M. F., Freitas, C. D. M., de Mesquita, H. C., Silva, T. S., dos Santos, J. B., et al. (2020). Increases in pH, Ca2+, and Mg2+ alter the retention of diuron in different soils. CATENA 188, 104440. doi:10.1016/j.catena.2019.104440
De, A., Bose, R., Kumar, A., and Mozumdar, S. (2014). “Worldwide pesticide use,” in Targeted delivery of pesticides using biodegradable polymeric nanoparticles. Editors A. De, R. Bose, A. Kumar, and S. Mozumdar (New Delhi: Springer India), 5–6. doi:10.1007/978-81-322-1689-6_2
De Gerónimo, E., Aparicio, V. C., and Costa, J. L. (2018). Glyphosate sorption to soils of Argentina. Estimation of affinity coefficient by pedotransfer function. Geoderma 322, 140–148. doi:10.1016/j.geoderma.2018.02.037
Dollinger, J., Dagès, C., and Voltz, M. (2015). Glyphosate sorption to soils and sediments predicted by pedotransfer functions. Environ. Chem. Lett. 13 (3), 293–307. doi:10.1007/s10311-015-0515-5
Dos Santos, L. O. G., Souza, M. F., Das Chagas, P. S. F., Fernandes, B. C. C., Silva, T. S., Dallabona Dombroski, J. L., et al. (2019). Effect of liming on hexazinone sorption and desorption behavior in various soils. Archives Agron. Soil Sci. 65 (9), 1183–1195. doi:10.1080/03650340.2018.1557323
Engeström, Y. (2011). From design experiments to formative interventions. Theory and Psychol. 21 (5), 598–628. doi:10.1177/0959354311419252
García-Delgado, C., Marín-Benito, J. M., Sánchez-Martín, M. J., and Rodríguez-Cruz, M. S. (2020). Organic carbon nature determines the capacity of organic amendments to adsorb pesticides in soil. J. Hazard. Mater. 390, 122162. doi:10.1016/j.jhazmat.2020.122162
Góngora-Echeverría, V. R., Martin-Laurent, F., Quintal-Franco, C., Lorenzo-Flores, A., Giácoman-Vallejos, G., and Ponce-Caballero, C. (2019). Dissipation and adsorption of 2,4-D, atrazine, diazinon, and glyphosate in an agricultural soil from yucatan state, Mexico. Water, Air, and Soil Pollut. 230 (6), 131. doi:10.1007/s11270-019-4177-y
Hansen, B. G., Paya-Perez, A. B., Rahman, M., and Larsen, B. R. (1999). QSARs for KOW and KOC of PCB congeners: a critical examination of data, assumptions and statistical approaches. Chemosphere 39 (13), 2209–2228. doi:10.1016/S0045-6535(99)00145-9
Hu, J., Tang, X., Qi, M., and Cheng, J. (2022). New models for estimating the sorption of sulfonamide and tetracycline antibiotics in soils. Int. J. Environ. Res. Public Health 19 (24), 16771. doi:10.3390/ijerph192416771
Jensen, R. (2022). Exploring causal relationships qualitatively: an empirical illustration of how causal relationships become visible across episodes and contexts. J. Educ. Change 23 (2), 179–196. doi:10.1007/s10833-021-09415-5
Jiang, L., Xu, Y., Zhang, X., Xu, B., Xu, X., and Ma, Y. (2022). Developing a QSPR model of organic carbon normalized sorption coefficients of perfluorinated and polyfluoroalkyl substances. Molecules 27 (17), 5610. doi:10.3390/molecules27175610
Kar, S., Roy, K., and Leszczynski, J. (2018). “Impact of pharmaceuticals on the environment: risk assessment using QSAR modeling approach,” in Computational toxicology: methods and protocols. Editor O. Nicolotti (New York, NY: Springer New York), 395–443. doi:10.1007/978-1-4939-7899-1_19
Kaur, P., Makkar, A., Kaur, P., and Shilpa, E. (2018). Temperature dependent adsorption–desorption behaviour of pendimethalin in Punjab soils. Bull. Environ. Contam. Toxicol. 100 (1), 167–175. doi:10.1007/s00128-017-2235-y
Kaur, P., Shilpa, K. H., and Bhullar, M. S. (2022). Equilibrium, kinetic and thermodynamic studies on adsorption of penoxsulam in Punjab soils. Soil Sediment Contam. An Int. J. 31 (5), 611–632. doi:10.1080/15320383.2021.1992608
Khorram, M. S., Sarmah, A. K., and Yu, Y. (2018). The effects of biochar properties on fomesafen adsorption-desorption capacity of biochar-amended soil. Water, Air, and Soil Pollut. 229 (3), 60. doi:10.1007/s11270-017-3603-2
Klement, A., Kodešová, R., Bauerová, M., Golovko, O., Kočárek, M., Fér, M., et al. (2018). Sorption of citalopram, irbesartan and fexofenadine in soils: estimation of sorption coefficients from soil properties. Chemosphere 195, 615–623. doi:10.1016/j.chemosphere.2017.12.098
Kobayashi, Y., Uchida, T., and Yoshida, K. (2020). Prediction of soil adsorption coefficient in pesticides using physicochemical properties and molecular descriptors by machine learning models. Environ. Toxicol. Chem. 39 (7), 1451–1459. doi:10.1002/etc.4724
Kobayashi, Y., and Yoshida, K. (2021). Quantitative structure–property relationships for the calculation of the soil adsorption coefficient using machine learning algorithms with calculated chemical properties from open-source software. Environ. Res. 196, 110363. doi:10.1016/j.envres.2020.110363
Kodešová, R., Grabic, R., Kočárek, M., Klement, A., Golovko, O., Fér, M., et al. (2015). Pharmaceuticals' sorptions relative to properties of thirteen different soils. Sci. Total Environ. 511, 435–443. doi:10.1016/j.scitotenv.2014.12.088
Leonelli, S. (2019). What distinguishes data from models? Eur. J. Philosophy Sci. 9 (2), 22. doi:10.1007/s13194-018-0246-0
Lewis, K. A., Tzilivakis, J., Warner, D. J., and Green, A. (2016). An international database for pesticide risk assessments and management. Hum. Ecol. Risk Assess. An Int. J. 22 (4), 1050–1064. doi:10.1080/10807039.2015.1133242
Loffredo, E., Parlavecchia, M., Perri, G., and Gattullo, R. (2019). Comparative assessment of metribuzin sorption efficiency of biochar, hydrochar and vermicompost. J. Environ. Sci. Health, Part B 54 (8), 728–735. doi:10.1080/03601234.2019.1632643
Mamy, L., Patureau, D., Barriuso, E., Bedos, C., Bessac, F., Louchart, X., et al. (2015). Prediction of the fate of organic compounds in the environment from their molecular properties: a review. Crit. Rev. Environ. Sci. Technol. 45 (12), 1277–1377. doi:10.1080/10643389.2014.955627
Marín-Benito, J. M., Herrero-Hernández, E., Rodríguez-Cruz, M. S., Arienzo, M., and Sánchez-Martín, M. J. (2017). Study of processes influencing bioavailability of pesticides in wood-soil systems: effect of different factors. Ecotoxicol. Environ. Saf. 139, 454–462. doi:10.1016/j.ecoenv.2017.02.012
McBratney, A. B., Minasny, B., Cattle, S. R., and Vervoort, R. W. (2002). From pedotransfer functions to soil inference systems. Geoderma 109 (1), 41–73. doi:10.1016/S0016-7061(02)00139-8
Meftaul, I. M., Venkateswarlu, K., Annamalai, P., Parven, A., and Megharaj, M. (2021). Glyphosate use in urban landscape soils: fate, distribution, and potential human and environmental health risks. J. Environ. Manag. 292, 112786. doi:10.1016/j.jenvman.2021.112786
Meftaul, I. M., Venkateswarlu, K., Dharmarajan, R., Annamalai, P., and Megharaj, M. (2020). Movement and fate of 2,4-D in urban soils: a potential environmental health concern. ACS Omega 5 (22), 13287–13295. doi:10.1021/acsomega.0c01330
Mosquera-Vivas, C. S., Martinez, M. J., García-Santos, G., and Guerrero-Dallos, J. A. (2018). Adsorption-desorption and hysteresis phenomenon of tebuconazole in Colombian agricultural soils: experimental assays and mathematical approaches. Chemosphere 190, 393–404. doi:10.1016/j.chemosphere.2017.09.143
Muhire, J., Li, S. S., Yin, B., Mi, J. Y., and Zhai, H. L. (2021). A simple approach to the prediction of soil sorption of organophosphorus pesticides. J. Environ. Sci. Health, Part B 56 (6), 606–612. doi:10.1080/03601234.2021.1934358
Neira-Albornoz, A., Fuentes, E., and Cáceres-Jensen, L. (2022). Connecting the evidence about organic pollutant sorption on soils with environmental regulation and decision-making: a scoping review. Chemosphere 308, 136164. doi:10.1016/j.chemosphere.2022.136164
Nolte, T. M., and Ragas, A. M. J. (2017). A review of quantitative structure–property relationships for the fate of ionizable organic chemicals in water matrices and identification of knowledge gaps. Environ. Sci. Process. Impacts 19 (3), 221–246. doi:10.1039/C7EM00034K
OECD (2014). Guidance document on the validation of (quantitative) structure-activity relationship [(Q)SAR] models. doi:10.1787/9789264085442-en
Olguin, C. J. M., Sampaio, S. C., and dos Reis, R. R. (2017). Statistical equivalence of prediction models of the soil sorption coefficient obtained using different log P algorithms. Chemosphere 184, 498–504. doi:10.1016/j.chemosphere.2017.06.027
Olguin, C. J. M., Sampaio, S. C., dos Reis, R. R., Remor, M. B., and Olguin, C. F. A. (2019). QSPR modelling of the soil sorption coefficient from training sets of different sizes. SAR QSAR Environ. Res. 30 (5), 299–311. doi:10.1080/1062936X.2019.1586759
Pandey, S. K., and Roy, K. (2021). QSPR modeling of octanol-water partition coefficient and organic carbon normalized sorption coefficient of diverse organic chemicals using Extended Topochemical Atom (ETA) indices. Ecotoxicol. Environ. Saf. 208, 111411. doi:10.1016/j.ecoenv.2020.111411
Paradelo, R., Conde-Cid, M., Martin Abad, E., Novoa-Munoz, J. C., Fernandez-Calvino, D., and Arias-Estevez, M. (2018). Retention and transport of mecoprop on acid sandy-loam soils. Ecotoxicol. Environ. Saf. 148, 82–88. doi:10.1016/j.ecoenv.2017.10.007
Pavão, Q. S., Freitas Souza, M., Teófilo, T. M. S., Lins, H. A., Borges, M. P. S., Silva, T. S., et al. (2022). Understanding the behavior of sulfometuron-methyl in soils using multivariate analysis. Int. J. Environ. Sci. Technol. 19 (1), 95–106. doi:10.1007/s13762-021-03161-0
Pereira, E. A. O., Melo, V. F., Abate, G., and Masini, J. C. (2019). Adsorption of glyphosate on Brazilian subtropical soils rich in iron and aluminum oxides. J. Environ. Sci. Health, Part B 54 (11), 906–914. doi:10.1080/03601234.2019.1644947
Pose-Juan, E., Marín-Benito, J. M., Sánchez-Martín, M. J., and Rodríguez-Cruz, M. S. (2018). Dissipation of herbicides after repeated application in soils amended with green compost and sewage sludge. J. Environ. Manag. 223, 1068–1077. doi:10.1016/j.jenvman.2018.07.026
Roy, K., Kar, S., and Das, R. N. (2015). “Chapter 6 - selected statistical methods in QSAR,” in Understanding the basics of QSAR for applications in pharmaceutical sciences and risk assessment. Editors K. Roy, S. Kar, and R. N. Das (Boston: Academic Press), 191–229. doi:10.1016/B978-0-12-801505-6.00006-5
Rybacka, A., and Andersson, P. L. (2016). Considering ionic state in modeling sorption of pharmaceuticals to sewage sludge. Chemosphere 165, 284–293. doi:10.1016/j.chemosphere.2016.09.014
Sabour, M. R., and Moftakhari Anasori Movahed, S. (2017). Application of radial basis function neural network to predict soil sorption partition coefficient using topological descriptors. Chemosphere 168, 877–884. doi:10.1016/j.chemosphere.2016.10.122
Sharma, A., Kumar, V., Shahzad, B., Tanveer, M., Sidhu, G. P. S., Handa, N., et al. (2019). Worldwide pesticide usage and its impacts on ecosystem. SN Appl. Sci. 1 (11), 1446. doi:10.1007/s42452-019-1485-1
Sidoli, P., Baran, N., and Angulo-Jaramillo, R. (2016). Glyphosate and AMPA adsorption in soils: laboratory experiments and pedotransfer rules. Environ. Sci. Pollut. Res. 23 (6), 5733–5742. doi:10.1007/s11356-015-5796-5
Siek, M., Paszko, T., Jerzykiewicz, M., Matysiak, J., and Wojcieszek, U. (2021). Mechanisms of tebuconazole adsorption in profiles of mineral soils. Molecules 26 (16), 4728. doi:10.3390/molecules26164728
Silva, M., Queiroz, M., Neves, A. A., Silva, A. A. D., Oliveira, A. F., Oliveira, R. L., et al. (2018). Impact of percentage and particle size of sugarcane biochar on the sorption behavior of clomazone in Red Latosol. An. Acad. Bras. Ciencias 90 (4), 3745–3759. doi:10.1590/0001-3765201820180135
Singh, B., Farenhorst, A., McQueen, R., and Malley, D. F. (2016). Near-infrared spectroscopy as a tool for generating sorption input parameters for pesticide fate modeling. Soil Sci. Soc. Am. J. 80 (3), 604–612. doi:10.2136/sssaj2015.03.0118
Skeff, W., Recknagel, C., Düwel, Y., and Schulz-Bull, D. E. (2018). Adsorption behaviors of glyphosate, glufosinate, aminomethylphosphonic acid, and 2-aminoethylphosphonic acid on three typical Baltic Sea sediments. Mar. Chem. 198, 1–9. doi:10.1016/j.marchem.2017.11.008
Sousa, G. V., Pereira, G., Teixeira, M. F. F., Faria, A. T., Paiva, M. C. G., and Silva, A. (2018). Sorption and desorption of diuron, hexazinone and mix (diuron + hexazinone) in soils with different attributes. Planta Daninha 36. doi:10.1590/s0100-83582018360100097
Thomas, P. C., Bicherel, P., and Bauer, F. J. (2019). How in silico and QSAR approaches can increase confidence in environmental hazard and risk assessment. Integr. Environ. Assess. Manag. 15 (1), 40–50. doi:10.1002/ieam.4108
Van Looy, K., Bouma, J., Herbst, M., Koestel, J., Minasny, B., Mishra, U., et al. (2017). Pedotransfer functions in earth system science: challenges and perspectives. Rev. Geophys. 55 (4), 1199–1256. doi:10.1002/2017RG000581
Wang, T., Yu, C., Chu, Q., Wang, F., Lan, T., and Wang, J. (2020). Adsorption behavior and mechanism of five pesticides on microplastics from agricultural polyethylene films. Chemosphere 244, 125491. doi:10.1016/j.chemosphere.2019.125491
Wang, Y., Chen, J., Yang, X., Lyakurwa, F., Li, X., and Qiao, X. (2015). In silico model for predicting soil organic carbon normalized sorption coefficient (KOC) of organic chemicals. Chemosphere 119, 438–444. doi:10.1016/j.chemosphere.2014.07.007
Xu, Z., Qian, X., Wang, C., Zhang, C., Tang, T., Zhao, X., et al. (2020). Environmentally relevant concentrations of microplastic exhibits negligible impacts on thiacloprid dissipation and enzyme activity in soil. Environ. Res. 189, 109892. doi:10.1016/j.envres.2020.109892
Zhang, X., Cheng, D., Shi, J., Qin, L., Wang, T., and Fang, B. (2018). QSPR modeling of the logKow and logKoc of polymethoxylated, polyhydroxylated diphenyl ethers and methoxylated-hydroxylated-polychlorinated diphenyl ethers. J. Hazard. Mater. 353, 542–551. doi:10.1016/j.jhazmat.2018.03.043
Zhao, L., Rong, L., Xu, J., Lian, J., Wang, L., and Sun, H. (2020). Sorption of five organic compounds by polar and nonpolar microplastics. Chemosphere 257, 127206. doi:10.1016/j.chemosphere.2020.127206
Keywords: environmental fate, organic pollutants, pesticides, decision-making, model interpretation
Citation: Neira-Albornoz A, Martínez-Parga-Méndez M, González M and Spitz A (2024) Understanding requirements, limitations and applicability of QSAR and PTF models for predicting sorption of pollutants on soils: a systematic review. Front. Environ. Sci. 12:1379283. doi: 10.3389/fenvs.2024.1379283
Received: 30 January 2024; Accepted: 19 July 2024;
Published: 13 August 2024.
Edited by:
Rui Zhang, University of Jinan, ChinaReviewed by:
Manuel Garcia-Jaramillo, Oregon State University, United StatesSen Li, Beijing University of Chinese Medicine, China
Copyright © 2024 Neira-Albornoz, Martínez-Parga-Méndez, González and Spitz. This is an open-access article distributed under the terms of the Creative Commons Attribution License (CC BY). The use, distribution or reproduction in other forums is permitted, provided the original author(s) and the copyright owner(s) are credited and that the original publication in this journal is cited, in accordance with accepted academic practice. No use, distribution or reproduction is permitted which does not comply with these terms.
*Correspondence: Angelo Neira-Albornoz, angelo-javier.neira-albornoz@uni-konstanz.de; Andreas Spitz, andreas.spitz@uni-konstanz.de