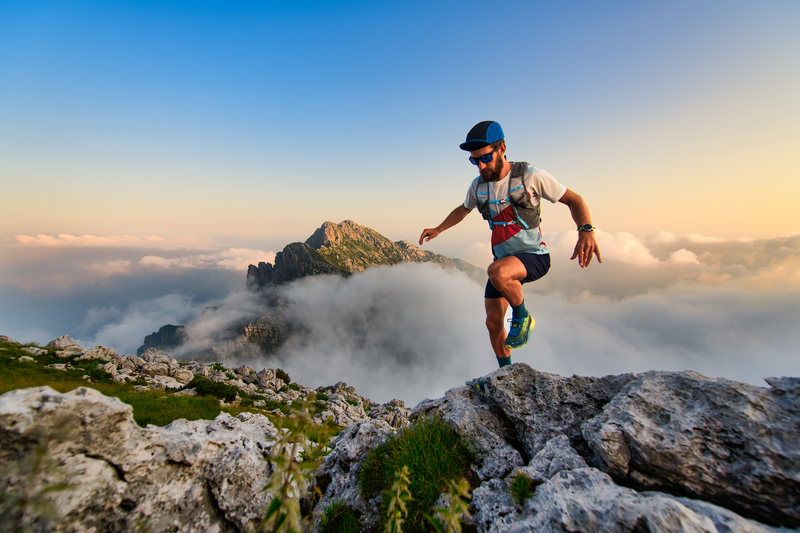
94% of researchers rate our articles as excellent or good
Learn more about the work of our research integrity team to safeguard the quality of each article we publish.
Find out more
REVIEW article
Front. Environ. Sci. , 25 July 2024
Sec. Big Data, AI, and the Environment
Volume 12 - 2024 | https://doi.org/10.3389/fenvs.2024.1375193
The digitalization of agriculture is rapidly changing the way farmers do business. With the integration of advanced technology, farmers are now able to increase efficiency, productivity, and precision in their operations. Digitalization allows for real-time monitoring and management of crops, leading to improved yields and reduced waste. This paper presents a review of some of the use cases that digitalization has made an impact in the automation of open-field and closed-field cultivations by means of collecting data about soils, crop growth, and microclimate, or by contributing to more accurate decisions about water usage and fertilizer application. The objective was to address some of the most recent technological advances that are leading to increased efficiency and sustainability of crop production, reduction in the use of inputs and environmental impacts, and releasing manual workforces from repetitive field tasks. The short discussions included at the end of each case study attempt to highlight the limitations and technological challenges toward successful implementations, as well as to introduce alternative solutions and methods that are rapidly evolving to offer a vast array of benefits for farmers by influencing cost-saving measures. This review concludes that despite the many benefits of digitalization, there are still a number of challenges that need to be overcome, including high costs, reliability, and scalability. Most of the available setups that are currently used for this purpose have been custom designed for specific tasks and are still too expensive to be implemented on commercial scales, while others are still in their early stages of development, making them not reliable or scalable for widespread acceptance and adoption by farmers. By providing a comprehensive understanding of the current state of digitalization in agriculture and its impact on sustainable crop production and food security, this review provides insights for policy-makers, industry stakeholders, and researchers working in this field.
Digital Agriculture (DA) deals with the practice of advanced technological solutions such as sensors, robotics, and data analysis for improving the ecological and economic viability of agricultural operations, and simultaneously elevating crop output and quality. Conventional farming methods have faced significant challenges in the past three decades to respond to the increasing demand for food, rising labor costs, reducing carbon footprint, and climate change (Abbas et al., 2022a; Abbas et al., 2022b; Elahi et al., 2022; Elahi et al., 2024). On the other hand, improving long-term efficiency and maintaining the viability of crop production requires adaptations of digital technologies to reduce input costs and increase profit margins. Digitalization of agriculture benefits from a wide range of automation software and hardware platforms to contribute to replacing tedious manual operations with continuous automated processes with the ultimate objective of securing food production for the increasing world population. In modern farms, multiple ground-based sensors combined with maps and drone-generated images, as well as artificial intelligence (AI) and prediction models are delivering detailed agronomic data on crop conditions to support farmers with short-term and long-term decision-making. With the advances in wireless communication and high-performance data processing hardware, the farms of the future are expected to be entirely connected (Figure 1). In this regard, digital agriculture is offering significant potential for innovative solutions toward automation and robotics, which in return frees human force from fieldwork, providing farmers with time to focus on developing scientific cultivation methods and agribusiness. In addition, the demands for the use of robots in agriculture to eliminate repetitive and dull tasks have introduced an exciting high-tech market that is constantly gaining attention from startup companies and investors. Some of the technology and methods involved in these practices, such as Geographic Information System (GIS), yield monitoring platforms, and variable-rate applications have been studied and covered in numerous published materials under the title of Precision Agriculture (PA) (Cisternas et al., 2020; Pathak et al., 2019). However, their impact on the entire agri-food value chain, as well as the relatively newer concepts such as the Internet-of-Things (IoT), mobile apps, robotics, Artificial Intelligence (AI), Unmanned Aerial Vehicles (UAV), big data analysis, digital twins, and Blockchain fall under the umbrella of digital agriculture (Fielke et al., 2020).
Figure 1. A conceptual view of data-sharing in connected farms for the implementation of digital agriculture.
Digital agriculture is being practiced in many regions, either on commercial scales or in pilot plants. The fundamentals for DA however began to shape after 2010, with the popularity of some of the core technologies such as low-power wide area network (LPWAN) for IoT applications (Klaina et al., 2022), open-source software for robotics (Mier et al., 2023), and machine learning tools for data processing (Sharma et al., 2020; Sharma et al., 2021), which redefined the existing concepts of precision agriculture and smart farming. In the past 10 years, low-resolution satellite-based maps have been frequently replaced with affordable UAVs that are equipped with sophisticated sensors for generating high-spatial and temporal resolution maps that can better support site-specific applications such as early disease detection and variable rate sprayers. In addition to the improvement in the hardware, DA is taking advantage of the advances in data processing and data management tools, deep learning, and cloud-based computing resources. A review of the published literature in the last decade shows that DA has employed a wide range of available technology to enable automation and create added value for sustainable food production. The inputs and outputs of DA have evolved based on data streams, reliable data-sharing services, and flexible data analysis tools that lead toward optimum automation processes and decision-makings. From an agronomic point of view, the digitalization of agriculture has widely supported farmers and researchers with solutions for a better understanding of different crop varieties and species that are more resistant to specific climate conditions or can be adapted to different soil conditions. For example, automated classification of seeds and plants was nearly impossible before the existence of software and high-performance computers to accomplish massive computations and to recommend which genome is suitable for breeding. In some cases such as plant health assessment or early disease detection, it is required to compare and categorize samples according to their colors, leaf morphology, shapes, or invisible symptoms using Support Vector Machine (SVM) classifiers or wavelet analysis methods. Digital agriculture is a growing research field that offers a wide variety of techniques to eliminate uncertainties involved with farming and food production.
The objective of this review is to present some of the use cases of digital technologies in agriculture and their impact on sustainable crop production and food security. The primary focus of each section of this review is to assess the benefits and challenges of digitalization in agriculture and how it can be leveraged to enhance the efficiency and productivity of crop production. The sections of the paper are organized to highlight and examine some of the core technological solutions in this field, including variable rate application, computer vision, UAV imagery, agricultural robotics, wireless sensing, and IoT monitoring. In the last section, the use of 5G network, Digital Twins, and Blockchain are covered as future scenarios. The objective is to provide a comprehensive understanding of the current state of digitalization in agriculture, its potential to address the challenges faced by the industry, and the role it can play in ensuring food security for the future.
The integration of automation and control systems alongside data processing software, web-based applications, and mobile tools has significantly influenced farming practices over the past three decades, largely aiming to enhance efficiency in land and resource utilization. Prior to 2010, farmers relied on technologies such as the Global Positioning System (GPS) (Shamshiri et al., 2013; Shamshiri and Ismail, 2013), ground-based sensing platforms, satellite maps, and local sensing devices like data loggers to monitor fields and identify deficiencies. However, the introduction of more compact technological solutions, such as autonomous drones, LiDAR sensors, high-resolution cameras, small-scale robots, and long-range wireless transmitters, has led to a shift in precision agriculture and smart farming methods towards digitization. These advancements have played a crucial role in fostering economic growth and promoting sustainability in food production. In its workflow, precision agriculture utilizes data from different resources, such as satellite images, in-situ sensors, and mobile sensing platforms, to identify deficiencies and enhance crop yield through improved resource management, including the application of variable rate technology (Shamshiri et al., 2018a). In contrast, digital agriculture encompasses a broader spectrum of technological innovations aimed at the ongoing monitoring, assessment, and management of soil conditions, water resources, and fluctuations in weather patterns across farmlands (Balasundram et al., 2023). These solutions are geared towards boosting field productivity while simultaneously minimizing operational expenses (Basso and Antle, 2020; Sparrow and Howard, 2021), mitigating climate change, and ensuring food security (Balasundram et al., 2023). Example cases include leveraging satellites and high-resolution UAV imagery to monitor crop water levels and quality, assessment of soil moisture and salinity, generating NDVI and yield maps, conducting health assessments for early disease detection, and identifying crop stress. In terms of automation, the integration of wireless sensors and IoT devices has facilitated the implementation of smart irrigation systems, management of water loss, and continuous assessment of soil nutrient levels in remote regions. Additionally, DA offers a diverse array of software applications accessible via smartphones and tablets. These tools empower farmers to benefit from live monitoring of field variabilities (Shamshiri and Weltzien, 2021), implement remote automation (Ahamed et al., 2023), and employ systematic management techniques.
Fixed-wing and multi-rotor drones that are equipped with high-resolution imaging sensors provide farmers with more accurate datasets when compared to satellite-based images. UAV-based remote sensing platforms are mainly used to monitor soil properties and crop stress, creating valuable information for developing decision support systems in pest control applications, smart fertilization, and irrigation management (Lajoie-OMalley et al., 2020). Although satellite images can also provide information about the existing of such variability in the fields in a shorter period of time, however the quality of their images depends on a cloud-free view, which limits their applications at any time and location. In addition, they do not offer a flexible and affordable platform for experimenting with multiple sensors. On the other hand, UAVs offer higher spatial and temporal resolution data which makes them a versatile remote sensing platform in different season and growth stages for supporting a wide variety of applications such as plant phenotyping (Shamshiri et al., 2018c; Comba et al., 2020), Leaf Area Density (LAD) estimation (Garcerá et al., 2021; Bates et al., 2021), determination of Leaf Chlorophyll Content (LCC) (Vergara-Díaz et al., 2016), and plant breeding (Guo W. et al., 2021). A conceptual illustration of a UAV-based image acquisition system with different sensors that are used in digital agriculture for estimation of crop parameters along with other in situ sensors and manual measurements is shown in Figure 2.
Figure 2. UAV-based remote sensing with different sensors and mapping software for digital agriculture (Li et al., 2021).
Utilizing UAV imagery to estimate the height and density of plant canopies offers valuable insights into the growth status of field plants. This method can be outlined in three main steps as (i) generating a digital surface model (DSM), (ii) creating a digital terrain model (DTM), and (iii) determining plant height by subtracting the DTM from the DSM. This approach holds particular significance in crop management decisions reliant on site-specific canopy characterization. The information generated through this method find applications across various domains of DA and PA, including leaf area index evaluation (Comba et al., 2020), precision crop protection (Garcerá et al., 2021), site-specific irrigation (Jiménez-Brenes et al., 2017), nutrient management (Tee et al., 2023), yield prediction (Gené-Mola et al., 2020), autonomous navigation (Pathak et al., 2019; Fielke et al., 2020), and early disease detection (Jurado et al., 2020). Moreover, detailed and reliable canopy information aids farmers in making timely and site-specific management decisions, underscoring the potential of 3D point cloud datasets for economic and environmental conservation strategies. Leaf area index estimation is crucial for enhancing crop growth models and addressing field uncertainties such as terrain erosion (Rodrigo-Comino, 2018), soil organic carbon problems (Chen et al., 2021), and climate change impacts (Balasundram et al., 2023). Collecting LAI data traditionally involves manual measurements using in-field portable instruments (Mourad et al., 2020) such as LI-3000C (LI-COR Biosciences GmbH, Homburg, Germany) or AccuPAR LP-80 (Metergroup, Pullman, WA, United States). However, UAVs equipped with high-resolution imaging sensors, LiDAR, multi-spectral, and hyperspectral cameras (Zhang et al., 2009; Hardin and Jensen, 2011; Wallace et al., 2012; Knoth et al., 2013; Shahbazi et al., 2014; Whitehead et al., 2014; Linchant et al., 2015) have proven successful in estimating LAI for various crops, including maize (Han et al., 2018), berries (Herrero-Huerta et al., 2015), almonds (Torres-Sánchez et al., 2018), olives (Jiménez-Brenes et al., 2017), grapes (Mathews and Jensen, 2013), apples (Hobart et al., 2020), and pears (Guo Y. et al., 2021). UAV remote sensing also shows promise in estimating LAI and canopy coverage ratio at the plant and canopy levels (Lei et al., 2019), essential components for estimating evapotranspiration, surface energy, and water balance (Mourad et al., 2020).
Several studies have demonstrated the effectiveness of UAV-based LAI estimation methods across different crop types and environmental conditions. For instance, Córcoles et al. (Córcoles et al., 2013) employed a UAV-based automated infrared imaging system to estimate LAI for onion crops, showing a linear correlation between canopy cover and LAI. Lendzioch et al. (Lendzioch et al., 2019) successfully estimated winter LAI and snow depth in a spruce forest using UAV-based imagery, while Sha et al. (Sha et al., 2018) compared UAV-based LAI estimation with field measurements for grassland pastures in China, revealing inconsistencies in near-infrared spectrum measurements. Additionally, Roosjen et al. (Roosjen et al., 2018) estimated LAI and leaf chlorophyll content of potatoes using UAV imagery, noting the impact of multi-angular angles and zenith angle on LAI estimation accuracy. Figure 3 provides a schematic overview of the key steps involved in estimating plant height and LAI using UAV imagery.
Figure 3. Schematic illustration of the three main steps involved in estimation of canopy height and LAI from UAV images (Li et al., 2022).
Identifying plant diseases using RGB images or visual inspection is often only feasible once visible symptoms manifest, often too late for effective intervention by farmers. For instance, Ganoderma disease, a significant threat to oil palm plantations, typically presents noticeable symptoms like foliar chlorosis, frond breakage, decayed tissues at the palm base, and fruiting body production at an advanced stage. This disease, causing both basal and upper stem rot, remains a severe issue in Southeast Asia, leading to stand loss, reduced yields, and the need for premature replanting. Young palms exhibiting symptoms may perish within 6–24 months, while mature palms can survive up to 3 years, although basal stem rot can destroy up to 80% of the total standing palms. Studies suggest a strong correlation between oil palm yields and nutrient levels. Hyperspectral analysis of images in agriculture offers promising opportunities for early Ganoderma disease detection in oil palms, with preliminary data indicating distinct spectral characteristics of infected leaves. Developing a rapid and effective field-level detection and mapping method for Ganoderma would aid growers in disease management and potentially enhance financial outcomes.
The methodology outlined in Figure 4 proposes a customizable solution, adaptable and scalable with various multi-spectral and hyperspectral cameras for disease detection. The procedure involves systematic steps involving (i) analyzing disease spectral characteristics in controlled lab settings, (ii) developing a classification method to differentiate the disease from other stresses and similar diseases, (iii) exploring the use of low-cost spectral radiometers for rapid screening, (iv) creating an instrumented platform for hyperspectral image collection and georeferencing on farms, and (v) conducting field trials to assess hyperspectral imagery effectiveness in diverse conditions. Adapting a UAV remote sensing platform for early disease detection entails addressing key questions: (i) the disease’s detectability at different infection stages, (ii) unique spectral characteristics of Ganoderma reflectance data, (iii) optimal statistical or mathematical methods for analyzing Ganoderma spectral data, and (iv) the effectiveness of low-cost multiband radiometers in aiding scouting crews to identify suspiciously infected trees.
Figure 4. Feasibility of using autonomous UAV-based hyperspectral imaging for the detection of Ganoderma Boninense disease in oil palms, showing (A) scanning the palm, (B) hyperspectral data collected at different wavelenghts, and (C) sample of one image generated at a specific wavelength (Shamshiri et al., 2018c).
Computer vision is a rapidly growing field within digital agriculture that aims to automate the process of phenotyping, which is the measurement and analysis of the physical characteristics of plants. This technology uses cameras and image processing algorithms to capture and analyze data on plant growth, development, and health. One of the main advantages of using computer vision for phenotyping is that it allows for the rapid and efficient collection of large amounts of data. Traditional methods of phenotyping, such as manual measurement and visual inspection, can be time-consuming and labor-intensive. With computer vision, data can be collected at a much faster rate, allowing for more frequent and detailed monitoring of plant growth and development. In addition, computer vision can provide more accurate and consistent data than traditional methods. Human error and subjectivity can affect the accuracy and consistency of manual measurements and visual inspections. Computer vision algorithms, on the other hand, are able to provide a more objective and consistent assessment of plant characteristics, providing a cutting-edge solution to analyze plant stress and disease identification. This is done by capturing images of the plant and then using image processing algorithms to analyze the images for signs of stress or infection. Various studies have highlighted the contributions of computer vision to improving yields and reducing costs. The technology has been also used to automate the process of seedling counting and selection, using image processing algorithms to accurately count and identify seedlings, which can help to improve the efficiency and accuracy of seedling selection. The following sub-sections provide summary reports on some of the projects in digital agriculture that incorporated computer vision.
Hyperspectral imaging and line scanning are two advanced non-destructive and non-invasive techniques that are being used in digital agriculture to collect data on the crop, even during the growing season and without affecting crop yields, with the objective of improving crop monitoring and management. Hyperspectral imaging captures images of crop plants and leaves using a wide range of wavelengths of light, from the visible to the infrared, and uses these images to identify different plant species, detect signs of stress or disease, or measure the amount of moisture, chlorophyll, and other important plant characteristics. This technology can provide farmers with detailed information about the health and growth of their crops, and provide knowledge-based decisions about irrigation, fertilization, and pest control. In recent years, hyperspectral imaging has gathered a large amount of interest in the field of non-destructive techniques. Originally developed for remote sensing applications, hyperspectral imaging is now being widely used in a multitude of fields including the food and agricultural sector. In the food industry, the commonly used standard methods are destructive and invasive in nature. Thus, they are not only time-consuming but also resource and energy intensive. With varying quality parameters across different products, the food industry continuously seeks in/on-line processing techniques that meet the quality demands as well as provide rapid, accurate, and reliable results. This approach combines the salient features of machine vision and near-infrared spectroscopy (Yu et al., 2020). Through the spectral and spatial information obtained from hyperspectral imaging, detailed information on the product has now become possible. Of the different acquisition techniques, line scanning is one of the most commonly used methods within the food industry (Ma et al., 2019). A typical hyperspectral imaging line scanner is shown in Figure 5.
Line scanning is a technique that captures images of crops by scanning a line of light across the field. This allows farmers to rapidly and efficiently collect detailed information about the crop canopy, including the height, width, and density of the plants. This information can be used to optimize planting density and monitor crop growth. Moreover, line scanning allows for continuous scanning of the product line-by-line, thus acquiring extensive spectral information on the product. This technique is being applied to predict moisture content and the distribution within fruits and vegetables such as apples, and purple-speckled cocoyam (Crichton et al., 2018; Ndisya et al., 2021). In addition, moisture content hyperspectral imaging has also shown the ability to predict several quality parameters such as total phenols and antioxidants properties in cocoa beans (Caporaso et al., 2018), chromaticity in apples’ slices (Crichton et al., 2017), and total carotenoids content in carrots (Md Saleh et al., 2022). Crichton et al., 2017 also implemented HSI to classify the freshness in beef. The results from this investigation present successful classification between the different storage conditions (i.e., fresh, matured, fresh-frozen thawed and matured-frozen thawed) through the varying color changes among the beef slices. With the view of moving towards in-line monitoring using hyperspectral imaging, Sturm et al., 2020 (Sturm et al., 2020) integrated a Vis-NIR camera within a pilot-scale hop dryer to investigate the dynamic changes within the hop cones. The study shows a proof of concept of integration of method within semi-industrial scale drying systems to the dynamic changes occurring within the product. In conjunction with this study (Sturm et al., 2020; von Gersdorff et al., 2021; Shrestha et al., 2020), also compared hyperspectral imaging and standard laboratory methods to assess its applicability for continuous monitoring. Through the application of methods comparison, these studies proved the feasibility of hyperspectral imaging for replacing standard laboratory methods and thus paving the way for real-time monitoring and quality assessment within the product. It should be noted that hyperspectral imaging and line scanning can be integrated with other technologies such as drones and robots, enabling farmers to scan large areas in a short time and collect a large amount of data, which can be used to optimize crop yields.
Implementation of digital agriculture requires wireless communication between sensors and controllers for remote monitoring and sending warning messages in open-field and closed-field farming via a flexible and modular automation solution that is compact in size, cost-effective, and easy to install and maintain. Studies show that smart irrigation and fertilization management systems (Giannoccaro et al., 2020; Lin et al., 2020) are capable of maintaining optimum level of pH and nutrient contents for plants with minimum inputs. The success of such an optimization relies on the integration and adaptation of the sensors and controllers with wireless communication and the IoT concepts for incorporating real-time data transfer and live monitoring. Wireless sensor network (WSN) was adopted in agriculture in the early 2000s, and has served as the backbone of IoT-driven automation systems, comprising various sensor nodes, repeaters, and receivers interconnected and meshed across fields to sustain DA. This network aids in comprehending the interplay among soil, crop, and weather, thereby enhancing productivity and profitability. In a wireless monitoring setup, data storage occurs locally on the receiver node, limiting accessibility. Conversely, in IoT-based monitoring systems, data from the receiver node are uploaded to a cloud web server, enabling access from any internet-connected client device (Shamshiri and Weltzien, 2021; Shamshiri and Hameed, 2021). In large-scale farming operations, data gathered from multiple wireless sensors are integrated into conventional decision support systems, AI algorithms, or crop growth models via internet connections and cloud-based streaming platforms, aiming to optimize production efficiency and financial returns. An example of such application is the works of (Sanjeevi et al., 2020; Popescu et al., 2020) that involved a scalable and collaborative UAV-WSN architecture for IoT monitoring and controlling in remote areas. IoT devices have been shown to be effective solutions to improve agricultural resource management and contribute to the sustainability of production. Farmers need mobile applications offering real-time data monitoring and dynamic decision support systems that leverage wireless automation and control. This technology significantly reduces wiring costs and maintenance efforts, especially in remote agricultural regions. However, studies have highlighted challenges in radio wave propagation inside dense plant populations (Shamshiri and Hameed, 2021), and therefore necessitating the use of low-powered long-range wireless communication protocols. In large-scale farming operations, factors like sensor node quantity, repeater placement, power usage, operating frequencies, and transmitter-receiver distances require careful consideration for seamless data collection.
In recent years, LoRa technology has emerged as a solution, enabling long-range communication between sensor nodes and receivers for field parameter monitoring. LoRaWAN, its networking protocol layer, is a leading LPWAN technology renowned for ultra-long-range wireless data transmission with minimal power consumption, ideal for digital agriculture applications (Shamshiri and Weltzien, 2021). LoRa bridges the gap between power efficiency and transmission range in remote areas lacking mobile coverage, utilizing reserved ISM radio bands like 433 MHz (Asia), 868 MHz (Europe), and 915 MHz (Australia and North America). Depending on network architecture and repeater node density, LoRa can cover distances of 2–10 km in rural areas, extendable to 100 km with repeaters. Figure 6 illustrates the primary components of this technology, comprising a smart sender node, communication module, and IoT platform.
Figure 6. Main components of an IoT monitoring system in agriculture using LoRa transmitters showing (A) data flow from the sensor node to the cloud, (B) elements of the smart sensor node with LoRa and CANBUS communication for in-field measurements, and (C) back-end software for storing sensor measurements in the database. (Source: Adaptive AgroTech) (Shamshiri and Hameed, 2021).
This setup allows live data viewing and sharing with unlimited users and applications from any location. The design or selection of compact sender nodes (Figure 6B) with efficient battery management and durability in harsh indoor environments is crucial. These sensing modules typically feature a programmable microcontroller interfaced with diverse sensor probes, onboard memory storage, a LoRa module (e.g., SX1276 or E220-900M22S LoRa breakout board), and battery management units with solar charging circuits. For control actuator nodes (e.g., smart fertigation or model-predictive microclimate control), sensor data is received by a LoRa controller node via peer-to-peer communication or first transmitted to secure cloud-based applications using a LoRaWAN gateway before reaching IoT-based controllers. The architecture presented in Figure 6C encompasses multiple hardware and software layers connected either via wires or wirelessly through standard communication protocols like WiFi and CANBUS. Previous studies have highlighted numerous successful applications of this technology for real-time monitoring and control in both closed-field and open-field crop production, particularly in remote areas lacking mobile network coverage. These applications have been extensively discussed in (Shamshiri and Weltzien, 2021; Shamshiri and Hameed, 2021; Singh et al., 2020; Shamshiri et al., 2020). It should be noted that while LoRa is the main physical layer of the LoRaWAN network, but the LoRaWAN protocol can also use other physical layer protocols such as GFSK in specific bands. In addition, LoRa can be used as the physical layer for other networking technologies. LoRaWAN topology is star, or star-to-star, which is capable of increasing communication range and maintaining low battery consumption. This is demonstrated in Figure 7 where each sensor node can transmits data to multiple gateways, and the network sends messages to a central server by means of these gateways. Sensor data that are successfully forwarded to the web-server are checked for redundancy and security. The LoRaWAN gateway typically comprises three primary components: a concentrator board linked to an antenna, a Raspberry Pi onboard computer facilitating connections between the concentrator and the LoRaWAN backend, and custom-written C++ codes managing the entire process. This gateway utilizes the existing LAN or WiFi network within the farm office to establish a connection with the web server. For deployment in farming applications, all devices must be waterproof, housed in IP68-rated cases, and equipped with appropriate connectors. The LoRa sensor node depicted in Figure 7 can be connected to various plug-and-sense probes, including the DS1820 for soil temperature sensing, BlueDot BME280 + TSL2591 for microclimate and light condition monitoring, ADP-LWS2020 for leaf wetness, and SKU capacitive sensor for soil moisture measurement.
Figure 7. Schematic demonstration of remote monitoring and control in digital agriculture using LoRaWAN technology. (Source: Adaptive AgroTech).
By tracking of agricultural machinery using LoRa GPS tracker it is possible to determine their timeliness in large scale operations. This is of interest for growers from a management perspective, providing them with an overview of the efficient time that the machine has spent on the field, and the number of hours that has been spent on stops and row-end turning. For this purpose, information such as time, latitude, and longitude from standard NMEA GPS strings are stored and transmitted using one or multiple LoRaWAN GPS tracker modules. The messages are received by one or more LoRaWAN gateways that can be located up to 10 km or more from the machine. The gateways might benefit from preprocessing software before uploading the data to a cloud-based mobile management app, for live monitoring of the total operation time, total stops and row-end turning time (ineffective operation time), total covered area, and average travel speed. An overview of the steps involved in data collection and processing of this approach together with sample results are shown in Figure 8. The outputs of the software is directly used to calculate field efficiency and machine index (Shamshiri et al., 2013). One of the main difficulties in processing raw GPS data is that they usually contain empty lines or broken strings. The application software that was used to produce results demonstrated in Figure 8 has built-in features that can detect different interruptions and outliers before processing the data via a simple user interface. For offline data processing, the entire calculation is carried out via three simple steps: “Open data”, “extract data”, and “process data”. As a result, the software generates an output table in Excel containing detailed information about the operation time and location of the machine in the field.
Figure 8. Overview of a tracking software for determining field machine index of agricultural machinery (Shamshiri et al., 2013).
Conventional data loggers that have been integrated with wireless modules and IoT patches have demonstrated to be a promising solution for improving the reliability of data collection for digital agriculture applications. These redundant devices minimize the disruptive effect of outdoor environment on field monitoring. A multi-channel hybrid data logger, illustrated in Figure 9A, features an IP66 enclosure, WiFi and LoRa antennas, an external power supply, and aviation plug connectors specifically designed for seamless integration with various sensor probes in both closed-field and open-field crop production systems. Each node is equipped with two separate circuit boards: one for transmitting sensor and GPS data via LoRa 868 MHz (Figure 9B) and another for LoRa/WiFi communication and data storage on an SD card (Figure 9C). This design facilitates the addition of new sensing capabilities to existing wireless networks and allows for easy replacement of defective sensor probes, minimizing network maintenance costs. The three connectivity boards demonstrated in Figure 9 include all necessary electronics and sockets for connecting typical sensors used in wireless monitoring of the indoor environment. These sensors include the BME280 (for air temperature, humidity, and atmospheric pressure), DS18B20 (for soil temperature), LDR Photoresistor (for light sensing), SX239 (for soil moisture), and NEO-7 GNSS modules. To ensure robust and efficient processing, the sensor node utilizes powerful ESP32 and Atmega328P microcontrollers integrated with customized codes for high efficiency and ultra-low power consumption. For example, the logger board shown in Figure 9C supports Bluetooth and WiFi communication and can save data on an onboard SD card via SPI data transfer. This board can also be interfaced with other microcontrollers using the onboard CANBUS modules. All sensor boards have been optimized for low-power consumption (deep sleep mode) and utilize MOSFET transistors in switch mode for sensor probes and memory cards in a way that when the board wakes up from a deep sleep mode, its controller triggers the MOSFET transistor to activate all power lines. The sensor node has a DS1337 IC for real-time logging clock and can access dates and times from an available world clock server in the presence of a WiFi network. The final log file is saved on a cloud server or the onboard SD card with GPS and time stamps and may include hundreds or thousands of data lines, depending on the data collection frequency and growing season. Several sensor nodes has been deployed and tested successfully in multiple farming applications and has measured, recorded, and transferred data without interruptions.
Figure 9. Components of a hybrid data logger including (A) an IP66 enclosure with an LCD, (B) a multi-channel sensor controller with Ethernet ports, and (C) a LoRa/WiFi wireless connectivity board with an onboard clock and a memory card, (D, E) desktop software for downloading and monitoring sensor data from data logger and cloud storage (Shamshiri and Weltzien, 2021).
The hybrid data logger system presented in Figure 9C is used for dynamic assessment of controlled environments, particularly regarding microclimate parameters and soil temperature (ST) set-points prior to cultivation. Understanding the reference values for air temperature, relative humidity (RH), vapor pressure deficit (VPD), and ST across various growth stages of fodder production (Ahamed et al., 2023), allows for real-time visualization of collected data on a mobile app, offering insights into deviations from ideal conditions. This approach is vital for decision-making in large-scale productions, where a controlled environment model is initially constructed and tested. To facilitate the monitoring and download of data from multiple sensors and cloud storage, the two desktop software applications shown in Figures 9D, E were developed. These applications can interface with sensor controllers via multiple serial COM ports, allowing users to execute commands and configure custom settings. Additionally, the software enables users to download log files containing sensor performance data (e.g., battery status, clock status, and historical parameters) and upload stored data to a cloud server. Users can also assign labels to each node for simultaneous reading and writing of log files from multiple devices, storing the data on local memory cards. These applications were created using the C# programming language and Microsoft.Net Core technology, ensuring compatibility with Microsoft Windows, Apple macOS, and Linux operating systems. It should be noted that Microsoft.Net Core is free, open-source software supporting various programming languages such as C#, C++, and VB.NET. These features offer a cost-effective and adaptable solution for future enhancements of the Port Logger and the IoT monitoring software. To optimize performance, both applications utilize multi-threading technology to execute parallel routines, enabling simultaneous listening to multiple ports and channels, and concurrent execution of multiple tasks. Each thread defines a unique flow of control, automatically setting different execution paths for complex and time-consuming parallel operations.
The workflow of an IoT-based monitoring system that has been realized by means of distributed nodes and modular hardware in a digital agriculture project for berry orchards (Shamshiri and Weltzien, 2021) is shown in Figure 10. In this scheme, each platform is custom-designed for specific applications in open-field cultivations based on a powerful microcontroller (32-bit, dual-core, 240 MHz) with LoRa modulation at 868 MHz. The nodes’ controllers were installed on long wood supports at an average height of 2 m from ground to overcome the issues with signal connectivity near high-density bushes and plants. For large-scale farms, the number of the sensor nodes, locations of the repeaters, power consumption, operating frequencies, and the distance between transmitters and receivers should be considered for continuous data collection. The set of hardware that is used in IoT-based automation of farming processes includes controllers that collect data from sensor probes (or send command signals to control actuators), connectivity nodes (ex., LoRaWAN), gateways, and protocol servers. Some of the radio protocols that are most widely used in IoT applications include WiFi, BLE, LoRaWAN, SigFox, NB-IoT, and LTE. It is sometimes necessary to have protocol conversion in the network. Various studies have discussed the limitations of radio wave propagation in the presence of high-density plants (Shamshiri and Hameed, 2021; Rezvani et al., 2020) and therefore suggested redundant nodes with onboard memory to ensure data transmission. Experimenting with different combinations of hardware and software setup in real farming conditions have shown that a typical challenge with IoT deployment is that most of the gateways use single-board computers with ARM processors, while many software applications are designed for x86 processors, causing compatibility issues and strange behaviors. Other challenges include battery failures due to low or high air temperature, solar-panel failure due to lightning, high winds, and birds droppings, and sensor probes failure due to corrosion.
Figure 10. Workflow of an IoT-based distribution automation system with solar-powered modular hardware for farms located in remote, hard-to-access areas.
Figure 11 presents plots generated from a dataset obtained using the hybrid data logger system in an Agricube prototype model, which includes an electrical heater and thermoelectric cooling device (utilizing Peltier elements and fans) to manipulate the environment. The experiment aimed to investigate heat exchange between air and soil bed, hypothesizing a potential linear correlation between air and soil temperature during substantial environmental temperature fluctuations. Further details of this experiment can be found in Shamshiri et al. (2021) (Shrestha et al., 2020). The proposed data acquisition system, along with sensor probes and modular battery packs, was deployed inside the cube for a 7-day period. Data were collected at 10-minute intervals and stored on a private cloud via WiFi connection for IoT monitoring. Simultaneously, the same data were logged on an onboard SD card and could be retrieved using a standard USB port as needed. The plots displayed in Figure 11 validate the reliability and resilience of the low-cost data logger system in operating on battery power under varying temperatures, crucial for long-term evaluations of controlled environment crop production systems, particularly in remote regions. This monitoring system offers seamless integration into modular shipping containers, ensuring efficient and precise operations for controlled environment fodder production facilities.
Figure 11. Testing connectivity and robustness of an IoT data logger in evaluating an experimental Agricube with large temperature gradients.
Traditional methods of plant disease identification, such as visual inspections and manual measurements, are time-consuming and labor-intensive. Plant disease symptoms, weed plants, or pest insects are normally tiny constituents in a plant canopy and are usually hard to detect with conventional remote sensing applications specially in the early stages of the outbreak or growth of the pest. The plant disease yellow rust (Puccinia striiformis West. F. sp. tritici), for example, develops small but distinctive features as symptoms that resemble long and narrow yellow to orange stripes. They usually occur on the plant leaves between the veins and consist of Urediniospores pustules with a dimension of 0.4–0.7 mm accompanied by chlorosis and necrosis (Chen et al., 2015). An expert that assesses crop diseases in the field can easily distinguish yellow rust from other crop diseases and score its severity at that location. This is possible because the visual symptoms of most diseases have unique features that are quite different from each other. This is true for many weed plants and other pests as well. A monitoring system for crop protection that can exploit this information in a timely, site-specific and selective manner would help to improve control strategies for crop protection and reduce pesticides by applying measures more precisely and sustainably in the field. However, those systems would be in dire need of very high-resolution data about the crop canopy. The unique and decisive features cannot be found at the canopy or field scale but rather at the plant or leaf scale. Thus, even drones operating at altitudes 20–100 m typically used for photogrammetric orthophoto production cannot resolve the features accurately enough to detect and distinguish pests in the field successfully. It is therefore required to have a closer canopy view, not more than 2 m away, which is the area where proximal sensing is operating and sensor systems are installed on platforms that are very close or even in contact with the object of interest (Adamchuk et al., 2018; Viscarra Rossel et al., 2010). In proximal sensing, even low-cost imaging systems, such as RGB cameras, would deliver highly detailed data that includes vital information for crop protection. The problem, however, is the sheer amount of unstructured image data that needs to be evaluated to extract the important crop information.
With the advent of more sophisticated neural network architectures including hundreds of layers and millions of adaptable weights and the possibility of training them with the currently available computing infrastructure, specialized deep learning models for image classification and image object detection (Convolution neural networks) became practically available (He et al., 2016). Deep learning is a type of machine learning that uses neural networks to analyze data and make predictions. With deep learning, the neural network can learn from thousands of images of different plant species, allowing for accurate and efficient identification. The main advantage of this technique is its ability to identify and classify plants based on subtle differences in their characteristics. This can be particularly useful for identifying different varieties or cultivars of the same species. Deep learning is a powerful tool for digital agriculture in solving object detection problems as an alternative to traditional vision methods in which feature descriptors (such as a scale-invariant feature transform (SIFT)) are used for recognizing objects. In the SIFT approach, all hand-crafted key features are used to form a definition for an interested object class from a set of reference images and these definitions are searched in new images to detect objects (OMahony et al., 2020). The major drawback of these feature descriptors is that they require a priori expert knowledge, time-consuming hand-tuning and poor reproducibility (Lu and Young, 2020). On the other hand, the deep learning method uses a series of hidden layers to discover the most descriptive and salient features of an object class on a given dataset of images, annotated with interested classes of objects, during model training. A typical deep convolutional neural network (CNN) architecture such as the one shown in Figure 12 consists of a multilayer stack of simple modules that learn to map a fixed-size input (an image of dimensions 224 × 224 × 3) to a fixed-size output (probability for each of predicted classes 1 × 1 × 60). Within a convolutional layer, a collection of adaptable filters (or kernels) traverses the width and height of the input volume, generating feature maps through the dot product computation between the filters and the input region where they overlap. The outcomes of this locally weighted summation subsequently undergo a non-linear transformation, often employing functions like the rectified linear unit (ReLU). The Pooling layer facilitates feature map down-sampling by consolidating semantically similar features. Finally, a fully connected layer is employed to flatten the preceding layer volume into a feature vector, followed by the application of a regression function such as Softmax to normalize the feature vector into a probability distribution representing predicted classes (OMahony et al., 2020; LeCun et al., 2015). Initial findings suggest significant promise in utilizing this approach for grasslands monitoring, offering advantages over conventional monitoring techniques (Basavegowda et al., 2022).
Today, convolutional neural networks outperform humans in the accuracy of detecting the information of interest in images (Zhou et al., 2021). One important leap forward with CNNs is that the filters that recognize interesting features for the model are now trained within the network itself and not deterministically chosen such as for example in the case of the bag of visual words classifiers (Pflanz et al., 2018). This makes CNNs much more versatile and adaptable for automatic image evaluation. In Schirrmann et al. (2021) (Schirrmann et al., 2021), a deep learning model was trained to detect yellow rust from very high-resolution RGB images at different stages of the disease outbreak. A deep residual neural network (ResNet-18) was used as deep learning architecture. ResNets are CNNs that include shortcut connections in the network architecture based on residual functions that enable skipping specific layers in the network, which increases the training performance of the deeper layers (He et al., 2016). Input for training and for testing included thousands of images taken at 2 m in nadir perspective from an RGB camera. Image acquisition was performed unselectively to mimic sensor data collection from a moving platform. For supervised training, snippets of images with and without yellow rust symptoms were used, which had been annotated earlier by experts on the screen. Although images could resolve sub-leaf features easily, there were cases in which even an expert was unable to identify the symptoms of stripe rust conclusively. Reasons were manifold and included occlusion of symptoms by other leaves, contrast issues, or highly similar features to yellow rust such as symptoms due to sunburn, water deficits, or damages caused by feeding. For example, the cereal leaf beetle produced symptoms on the leaves with a remarkable resemblance to yellow rust because they feed between the leaf veins. One distinguishing feature of the damages is their rough edges, whereas stripe rust symptoms show a smoother transition from invested to healthy leaf areas (Figure 13). Thus, it is not an easy task for the model to decide if yellow rust infection occurs, especially at the early infection stages.
Figure 13. Winter wheat canopy with and without yellow rust (YR) symptoms (A). Deep learning architecture used for classifying yellow rust symptoms in the images (B). Receiver operating characteristics (ROC) curves for the classification of images taken at different days after inoculation (DAI) (Schirrmann et al., 2021).
The trained ResNet model showed high accuracy for estimating the yellow rust symptoms after the disease has spread into the canopy to about 2%–4%, which was after 40 days of inoculation (DAI). During this time, symptoms showed the orange uredospore pustules aligned along stripes on the leaves. For these images, the model had a high area under the curve in the corresponding ROC plot (Figure 13) and the estimation accuracy was greater than 80%. With drone imagery from 20 m above the canopy, the disease spreading was still not recognizable because no apparent disease loci had developed. One week before, however, when symptoms were sparser and underdeveloped, estimation accuracy was worse with an overall accuracy of about 57%. These first symptoms were mostly chlorotic patches with a stripe-like appearance on the leaves that occurred randomly in the lower leaf layers and only in a few areas of the infected plots. These first symptoms were quite hard to detect by the model.
Weed detection is one of the most important aspects of digital agriculture that has received significant attention in recent years, with the goal of applying computer vision and machine learning algorithms to analyze images of crops in real time for rapid identification and removal of weeds. Some companies have developed sensor-based weed detection systems, which use a combination of sensors, such as cameras, infrared, and LiDAR, to detect weeds. These systems can be mounted on UAVs, field robots, tractors, or other ground vehicles to scan a field while the vehicle is in motion. In addition, some studies have reported on the development of weed detection systems that can scan a large area in a short time and are trained to recognize specific weeds by analyzing large amounts of image data in order to improve the accuracy and efficiency of weed classification based on their characteristics. An example includes the work of de Camargo et al. (2021) (de Camargo et al., 2021), in which the optimization of a ResNet-18 model for the classification of weed and crop plants in UAV imagery was considered. This study is part of a larger project that aims to develop an intelligent real-time monitoring and mapping system for the detection of weed distribution in cereal crops. The idea is to capture low-altitude imagery from UAVs with sufficient details to differentiate individual weed plants and evaluate the images directly on the drone using optimized onboard AI image recognition during flying. The planned system will not only differentiate between crop plants and weeds but will also enable the identification of learned plant species in a cultivated area. Based on this, better application maps for site-specific and selective herbicide management can be derived, which increases environmental and consumer protection. In the referenced study, the prediction pipeline of the ResNet-18 model underwent optimization to eliminate redundant computations within a classification model applied to overlapping tiles in a larger input image, such as a full camera image captured from the UAV, as described in Figure 14.
Figure 14. An intelligent online mapping system for monitoring and mapping weed plants with UAVs in crop fields based on optimized deep learning models that can be implemented in embedded systems for fast, automatic image evaluation (de Camargo et al., 2021).
Convolution and max pooling layers, along with residual blocks, span a broader spatial dimension due to the enlarged input size resulting from the full camera image. To circumvent redundant pooling operations across overlapping tiles, a custom cumulative local average pooling layer was integrated into the network. Annotated tiles of the UAV images were used for training the ResNet model, which depicted weed, wheat, or soil background. The optimized model was implemented on an NVIDIA Jetson AGX Xavier embedded system with TensorRT (NVIDIA CORPORATE, Santa Clara, CA, United States). In 16-bit mode, a full-image evaluation with the optimized model was about 2.2 frames per second. No memory issues occurred during training and testing. Using images from a test field, the image classifier had an overall accuracy of 94%. Even in more challenging parts of the images where plants overlapped, the model quite accurately identified the weed species. Both exemplary research studies show that combining low-cost imaging technologies, e.g., RGB imaging, with artificial intelligence enables the extraction of more specific field information for crop protection. This would allow autonomous monitoring of crops using imaging platforms in near proximity to the plants, e.g., with low-flying drones or tractors passing through the field because the images can be evaluated by specialized deep learning models automatically while the sensor platform operates over the field.
The integration of robotics and wireless connectivity that are integrated with virtual reality, digital twin concepts, and IoT platforms, is often denoted as the Internet of Robotic Things (IoRT) (Vermesan et al., 2020) and has emerged in the last few years for collaborative control and teleoperation (Su, 2020) to optimize the use of autonomous agricultural machinery in unstructured farms. The main justifications for the deployment of IoRT infrastructure in agriculture can be summarized as (i) to provide real-time monitoring and control of the robot’s states and functionality (i.e., location, orientation, speed, distance to obstacles, and battery status), (ii) to feed these data to simulation models, digital shadows, and cloud-based decision support systems, and (iii) to send instant responses to the robot for assisting the autonomous navigation. An effective IoRT-based solution should incorporate the use of long-range wireless communication, simulation environment, and web-based applications to constantly monitor the robot in the field, and transmit human-in-the-loop control commands for robot teleoperation. A conceptual illustration of the proposed IoRT solution using a local LoRa network for exchanging messages between the actual mobile robot in the field and the digital shadow of that robot inside a virtual environment is shown in Figure 15. This approach assists the navigation of the robot in complex situations without the need for high-end network infrastructure.
Figure 15. Overview of the IoRT concept for exchanging float32 messages with a field robot and assisting autonomous navigation in complex situations using LoRa transceivers without the need for high-end network infrastructure, highlighting the architecture of the LoRa connectivity for exchanging messages between the actual robot and its digital shadow.
An overview of the message exchange between the actual robot and its digital shadow is presented in Figure 15. The input of the digital shadow inside the simulation is a new target position (x, y), and the outputs are a ROS topic called SimIrus, and a CSV file that includes the corresponding latitude and longitude coordinates of the simulated robot. The LoRa transceiver board that was interfaced with the digital shadow of the robot using ROS serial subscribes to this SimIrus topic and extracts the latitude and longitude coordinates. This node also receives LoRa messages that include the current position of the actual robot. When the digital shadow receives a new target position, it autonomously drives to this position using the Pure-Pursuit controller. The current positions of the digital shadow (
In precision agriculture, variable rate applications such as spraying or fertilizing were either realized by means of georeferenced prescription maps that were usually generated based on satellite remote sensing techniques, or by using on-the-go sensors. To this aim, tractors and other large machinery were required, and the availability of accurate GPS signals was crucial for the success of the operation. In digital agriculture however, drones (Shamshiri et al., 2018c) and swarms of small-scale robots that benefit from sensor fusion can operate in GPS denial environments and can deliver more precise VR applications by targeting individual plants (Shamshiri et al., 2018a). This is possible due to the availability of low-cost sensors, high-performance microcontrollers, and onboard computers that can process big data, support complex models, and simulate parallel decision-making scenarios for converting precise data into actions, which in return provides farmers with local-specific information on-the-go. Figure 16 showcases a novel design of a variable rate liquid fertilizer applicator, featuring a distinctive flow control and spray system capable of administering NPK (Nitrogen, Phosphorus, and Potassium) simultaneously at variable rates around oil palm trees in a single pass. This system, developed following the spot application method, is capable of evaluating the NPK status of a 25 m2 soil area and applying N, P, and K nutrients at different variable rates using aqueous solutions of straight fertilizers (Yamin et al., 2020a; Yamin et al., 2020b). Based on simulation analysis, six 8006 flat fan nozzles were meticulously chosen to ensure optimal swath coverage of fertilizer spray. Nozzles 1–3 were affixed vertically on the horizontal boom to apply spray on the machine side of oil palm trees, while nozzles 4–6 were positioned at −22°, −21°, and −20° angles to the horizontal plane on a 45° inclined boom to administer spray across the tree, employing the trajectory approach as depicted in Figure 16. Employing this approach, an average simulated liquid velocity of 14.05 m/s per nozzle was determined, allowing for spraying at a distance of 2.5 m across the oil palm tree. Consequently, this technique achieves enhanced fertilizer distribution around the tree within a 25 m2 region encompassing the most effective roots. Finite element analysis was utilized to devise the mechanical structure of the applicator, responsible for housing all equipment and fertilizer tanks. The mechanical structure and fertilizer tank assembly exhibited minimum safety factors of 3.13 and 11.34, respectively, ensuring their ability to withstand the requisite weights during field operation.
Figure 16. An automatic variable-rate liquid fertilizer with adjustable spray coverage, developed for practicing digital agriculture in oil palm plantations (Yamin et al., 2022).
Agro-food robotics represents a fast advancing domain that is transforming farm production capacities, leveraging the advantages of robots over human labor, including heightened accuracy and efficiency, enhanced consistency and reliability, and reduced operational costs. In digital agriculture, farmers are eager to identify deficiencies and variations in large-scale cultivations, employing precise technology and accurate management solutions to address them effectively. Furthermore, optimizing input utilization is a promising approach to boost farm profitability. Comprehensive research and development in agricultural robotics have been documented in a wide range of review papers (Shamshiri et al., 2018a; Bergerman et al., 2016; Duong et al., 2020; Kootstra et al., 2020; Oliveira et al., 2021a) covering specific tasks such as phenotyping (Atefi et al., 2021; Yao et al., 2021; Xu and Li, 2022), arable farming (Emmi and Gonzalez-de-Santos, 2017), livestock farming (Ren et al., 2020), greenhouse horticulture (Barth et al., 2016), orchard management (Zhang et al., 2019), forestry (Oliveira et al., 2021b), and food processing (Duong et al., 2020). Review papers also cover specific technologies used in agricultural robotics, such as computer vision (Lu and Young, 2020; Tian et al., 2020; Fountas et al., 2022; Wang et al., 2022), active perception (Magalhães et al., 2022), path planning (Santos et al., 2020), and grasping and soft grasping (Elfferich et al., 2022; Navas et al., 2024). The majority of these studies have emphasized that in order for agricultural robots to operate efficiently in harsh and unpredictable environments (i.e., including extreme weather conditions), they must be equipped with redundant sensing solutions to effectively perceive their surroundings and be able to communicate and interact seamlessly with other robots and machinery in the field. Some studied have proposed robotic platforms with flexible designs that can be integrated with custom-built attachments to perform specific tasks such as mowing (Verne, 2020), weeding (Gerhards et al., 2022), and spraying (Meshram et al., 2022). Robots that are equipped with several data acquisition devices such as multi-spectral (Karpyshev et al., 2021), hyperspectral (Zhang et al., 2012), NDVI (Tiozzo Fasiolo et al., 2022), thermal (da Silva et al., 2021), or NIR cameras (Milella et al., 2019) provide a great opportunity for field scouting (Yamasaki et al., 2022), early disease detection (Mishra et al., 2020), and yield estimation (Kurtser et al., 2020; Massah et al., 2021). Employing robots for these tasks have a high potential for saving costs, however this is justified if additional cultivation steps or a higher repetition rate leads to a qualitative or quantitative increase in yield. A recent study on the acceptance level of agricultural robots in Germany clearly indicates that the majority of the farmers surveyed are keen to immediately use this technology on their farms specially for tasks such as weeding due to the potential benefits of saving labor and practicing more sustainable farming methods (Rübcke von Veltheim and Heise, 2021). A survey in the published studies also shows that small robots are particularly of interest for small and irregularly shaped fields where large machinery are unable to operate efficiently (Shamshiri et al., 2018a). The scalability of field robots and suitability for small field sizes besides their lower ownership costs create opportunities for smaller farms to become economically viable.
The development of robust collision avoidance systems for mobile robots that operate inside unstructured agricultural fields proposes serious challenges due to the extreme variations in high-density bushes and disturbances of the outdoor environment. Data fusion and multiple perception solutions are usually employed to assist the existing GPS-based navigation and to improve the reliability of the operation. Figure 17 shows the hardware layer of a control system that benefits from a set of ROS-based multi-channel infrared sensors for providing feedback, and a Jetson Nano onboard computer for performing the computation. The system is expected to maintain an agricultural tractor between the plants’ rows with an accuracy of 5–10 cm from the side with an ideal speed of 5–8 km/h (Weltzien and Shamshiri, 2019). In the software layer, different controllers including PID, machine learning, and fuzzy knowledge-based algorithms (Shamshiri et al., 2024) can be implemented and compared. However successful development of such systems requires a proof-of-concept via extensive validation tests with the digital representation of the sensors, a dynamic model of the robot platform, and a virtual replica of the orchard. The effectiveness and throughput of agricultural mobile robots are propelled by the utilization of machine learning (ML) and deep learning (DL) techniques, which empower robots to learn from and analyze data autonomously, without explicit programming. ML/DL has emerged concurrently with the discipline of Big Data, facilitating the detection of relationships, analysis of patterns, and generation of predictions within farming activities. An illustrative example of the application of supervised machine learning algorithms, coupled with multiple distance detection sensors, is demonstrated by the SunBot project, as depicted in Figure 17, which proposes the use of a field agent robot for autonomous navigation within berry orchards, conducting health assessments and gathering data to support digital agriculture initiatives. Given the limitations of traditional farming approaches in enhancing productivity, modern farms increasingly rely on IoT systems for data collection, alongside ML/DL techniques for data analysis and decision-making. This integration enables farms to automate partially or fully, thereby optimizing operations and driving productivity growth.
Figure 17. A proposed control system for collision avoidance in a four-wheel steering field robot agent for berry orchards, utilizing multiple programmable distance sensors to implement machine learning and knowledge-based algorithms for assisted navigation (Shamshiri et al., 2024).
To verify the effectiveness of the proposed collision avoidance system depicted in Figure 17, initial field visits were conducted to collect preliminary data using high-precision RTK GPS. These data served as the foundation for creating a virtual orchard within CoppeliaSim (Shamshiri et al., 2018b), which was interfaced with the ROS (Quigley et al., 2009). This setup facilitated the testing of different sensors, hardware in the loop, and control algorithms on a full-scale simulated tractor and orchard model, as depicted in Figure 18. The simulation methodology involved translating raw data streams from various sensor inputs (such as GNSS, LiDAR, laser, radar, and RGB camera) into actionable information within the command and control system. This allowed for experimentation with autonomous navigation, enabling the tractor to avoid both moving and stationary obstacles within the orchard environment. Through this simulation-based approach, the proposed collision avoidance system could be thoroughly evaluated and refined before implementation in real-world settings. The result provided a safe, fast, and low-cost experiment platform for the development, testing, and validating of the sensing and control strategies with different algorithms. The simulation scene shown in Figure 18A enabled human-aware navigation by finding the best positions for each sensor on different tractors and provided a flexible solution for attaching other implements and determining the optimum row-end turning patterns in presence of random obstacles. It also accelerated complicated analysis with the weight distribution of the attached implements and to understand the behavior of the tractor on uneven terrains. The main elements of the simulation scenes in this project were (i) mesh files representing plants, tractors, and obstacles, (ii) API and codes that created interfaces between different software environments, and (iii) algorithms and dynamic models including image processing for human detection, inverse kinematics for the hydraulic arms, minimum distance calculation, steering system, path following, and obstacle avoidance algorithms. A prototype of the final proposed solution that benefits from various sensors for autonomous navigation, obstacle avoidance, and safety is shown in Figure 18B. It should be noted that although electrical tractors and mobile robots are contributing to the digital transformation of agriculture by replacing drivers and human operators with artificial intelligence, they are still functioning in experimental phases and require supervision, which makes them far from being deployed on commercial and large operational scales.
Figure 18. Demonstration of a proof-of-concept for assisted autonomous navigation showing (A) a dynamic simulation for experimenting with multiple sensing solutions, and (B) an articulated steering electric tractor manufactured by Weidemann, equipped with a custom-built electric mower (courtesy of the SunBot project).
Maintaining precise control of environmental variables within both open-field and closed-field production systems has significant potential for enhancing operational sustainability. By minimizing water, chemical, and energy demands while simultaneously mitigating disease spread, and increasing yield, such control measures can result higher profits. In controlled environments like aeroponic or hydroponic indoor farming, automation systems encounter various uncertainties and disturbances that elude complete modeling or implementation via conventional control algorithms. Consequently, adaptive solutions are necessary to effectively limit production costs and enhance efficiency. To achieve this, data collected from multiple wireless sensors distributed across the growth environment should be leveraged in conjunction with knowledge-based software and dynamic models. For instance, the IoT-based fertigation control system, shown in Figure 19, can monitor various aspects of a hydroponic production, including flow rate, electrical conductivity (EC), and pH of the fertigation solution, alongside external variables such as solar radiation and climatic conditions. Utilizing the collected data, the system integrates them into models, rule-based algorithms, or adaptive control laws to ensure that specific control commands, such as triggering particular pumps or initiating other processes, are executed at the right moments to effect environmental adjustments. This approach optimizes resource utilization, and enhance sustainability and overall system performance.
Figure 19. Realization of IoT-based control for multiple actuators using LoRa sensors and a WiFi receiver for precision irrigation, showing (A) the main components and connections between modules, (B) a wireless sensor node with onboard GPS, and (C) a wireless controller with onboard relays. (Source: Adaptive AgroTech).
Since control of some actuators require separate driver boards that can only receive specific type of messages, a separate custom-designed IoT-based controller was designed that communicates with wireless sensor nodes, end-users, and actuators drivers, and can send and receive command signals via CANBUS as shown in Figure 20. This controller board benefits from a STM32 32-bit ARM processor, and an ESP8266 microcontroller, an onboard RTC clock, two CANBUS ports for industrial communication, and an SD card for data logging. The board can also be interfaced simultaneously with multiple controller driver boards such as relay modules via wired communication ports such as I2C, USART, and SPI, or by means of WiFi wireless signals. The control commands can be generated by the crop growth models that have been implemented in the processor as codes or Simulink blocks. Furthermore, the controller is capable of receiving command signals from cloud-based applications. Concurrently, environmental sensors are attached to collect measurements, storing data on an SD card, and transmitting data either directly to a web server or through wireless communication to a gateway utilizing LoRa modulation. An in-depth elucidation of this framework pertaining to greenhouse tomatoes is provided in (Shamshiri et al., 2020; Rezvani et al., 2020). The board presented in Figure 20 was utilized to collect air temperature data within an experimental Agricube. Programmed to read and transmit measurements every 10 s, the board conveyed this information to an open-source secure cloud database via WiFi connection. These data points, each assigned a unique ID representing the collection time and location, were stored on a private cloud database accessible via a secured API key address. Subsequently, they were utilized by the IoT controller as feedback for the control algorithm.
Figure 20. Realization of IoT-based control for multiple actuators with separate drivers via WiFi, showing the connectivity board that functions as the receiver and main controller, and sample data collected from an experimental Agricube, demonstrating the closed-loop response of a simple ON/OFF control system for a heater to maintain the air temperature between 25°C and 27°C with a feedback frequency of 0.16 Hz (Source: Adaptive AgroTech).
To ensure the reliability of IoT control, numerous data collection samples were conducted and analyzed. The results indicated that no data points were lost during the tests as long as the WiFi network remained available. Enhancing the system’s reliability can be achieved by augmenting the number of WiFi access points, enabling the controller to seamlessly switch between networks. The response of the controller, as depicted in Figure 20, illustrates the robust performance of IoT-based automation, characterized by high spatiotemporal resolution and excellent stability in data transfer, with 10 readings per minute achievable within a 1.0 km distance from the wireless controller. This level of performance underscores the reliability of this approach in adjusting growth parameters for controlled environment crop production systems.
The majority of agricultural fields are situated in remote regions with restricted mobile coverage and network accessibility. Consequently, it is commonplace to utilize wireless transmitters and WiFi repeaters to extend coverage over broader areas. However, the energy consumption of these devices, coupled with their reliance on limited energy sources, poses a significant challenge that necessitates design of power management boards. Figure 21 illustrates multiple solar-powered LoRaWAN sensors deployed across various berry orchards in the state of Brandenburg, Germany (Shamshiri and Weltzien, 2021). These sensors are employed for IoT monitoring of various agricultural parameters, including air and soil temperature, relative humidity, soil moisture, leaf wetness, light conditions, and dew-point temperature. Using solar power, these sensors offer a sustainable solution for remote monitoring, ensuring battery charging for continuous data collection and transmission without relying on frequently battery replacement. Some of the main difficulties experienced with the implementation and use of these IoT sensors in orchards can be mentioned as: lack of infrastructure for mobile network coverage, data management and concerns regarding security and privacy, issues related to cost and maintenance, scalability limitations, tolerance to faults, and the need for skilled professionals to implement and manage the system. In addition, inflexibility of the available IoT solutions to operate in harsh environmental conditions, as well as regulatory compliance and standards adherence can be mentioned as the main factors that prevents farmers from adopting these devices. Although LPWAN, point-to-point LoRa, and LoRaWAN sensors are low-energy solutions with long communication range, but they can also face connectivity limitations such as signal interruption and wireless signal quality. For example, in remote areas characterized by geographical constraints and diverse land topologies, wireless signals may encounter attenuation issues due to environmental obstacles or electromagnetic interference from other devices. Such challenges can delay the propagation of wireless signals, impacting the reliability of communication networks. To address these issues, various solutions such as installing signal repeaters to amplify and extend the reach of wireless signals can be used. Additionally, designing more efficient network topologies, such as mesh networks, can help optimize signal propagation by establishing multiple communication pathways and enabling data to circumvent obstacles more effectively. These measures contribute to improving the robustness and reliability of wireless communication systems in remote and challenging environments.
Figure 21. Implementation of an IoT-based monitoring system in a berry orchard to overcome uncertainties and connectivity issues in remote locations, showing (A) multiple solar-powered WiFi/LoRa sensors with modular accessories, (B,C) wireless data logger and transmitter for wireless monitoring of microclimate parameters, and (D) connectivity boards with WiFi/LoRa modules (Source: SunBot.de).
In small-scale fields, the costs associated with maintenance and ownership may not be justifiable for farmers, particularly when concerns arise regarding the potential sharing of sensitive field information and the associated risks to their production reputation due to inadequate IoT security protocols. The differences between hardware and software from different manufacturers imply heterogeneity in wireless communication protocols and connectivity standards, making it difficult to integrate and standardize the IoT automation process. Additionally, there is currently a lack of standardization and regulation in the IoT industry, which can lead to confusion and complexity when implementing IoT devices in agriculture. Therefore implementation and maintenance of IoT in commercial farms can be expensive and require significant investment in hardware, software, and network infrastructure. Moreover, the reliability of IoT-based automation systems in agriculture is significantly influenced by the harsh environmental conditions and varying climatic characteristics, such as high temperatures, wind speeds, heavy rain, and dusty environments, which can damage sensors or disrupt their performance. Consequently, selecting robust hardware setups capable of withstanding these conditions is paramount. An illustrative example of such a robust hardware setup is presented in Figure 21, showcasing a modular IoT solution featuring multiple LoRaWAN sensors and gateways custom-built for live field monitoring projects (Shamshiri and Weltzien, 2021; Weltzien and Shamshiri, 2019). These devices are designed to withstand harsh field conditions and address challenges associated with WiFi instability. Each sensor benefits from multiple transmitters to mitigate the risk of signal loss, while multiple gateways ensure data uploads to private clouds, enhancing system reliability and resilience.
The introduction of the fifth generation mobile network (5G) is reshaping and redefining digital agriculture, initiating new possibilities for farming mechanization. A noticeable trend in this context involves the deployment of distributed automation systems, including collaborative robots and swarms of small-scale unmanned machinery. These systems can autonomously perform a range of site-specific operations such as weeding and spraying, leveraging IoT-based cloud computing services. Although similar solutions have been piloted or implemented on a commercial scale, ensuring stable and secure connections between nodes remains a persistent concern. The dynamic nature of agricultural environments, coupled with the reliance on wireless communication, underscores the importance of addressing connectivity stability and security to maximize the effectiveness of these automation systems. Efforts to enhance connection stability and security between nodes are essential for realizing the full potential of distributed automation systems in digital agriculture. The 5G network will provide a reliable and secure communication infrastructure with low latency capabilities for the realization of automated farms (Ma et al., 2017; Khanna and Kaur, 2019; Valecce et al., 2019; Tang et al., 2021) with AI-robotics. Compared with 4G networks, 5G has a faster information transmission rate with higher quality of dissemination, which can effectively be used in developing smart systems with high-speed data transfer, up to 20 Gbps, and can connect more devices per square kilometer (Li and Li, 2020; Said Mohamed et al., 2021). This is crucial to enable robotization and digital agriculture processes. Simultaneous use of local mesh and cellular networks can effectively address the problems with poor communications, allowing growers to have uninterrupted stream of data (Franchi et al., 2021), including crop yield, soil, fertilization, smart monitoring, irrigation management, pesticide applications, disease management, autonomous navigation, fruits harvesting (Navas et al., 2024), and supply chain management (Khujamatov et al., 2021; Friha et al., 2021). An example lies in the work of Xue et al. (2021) (Xue et al., 2022), in which a frame structure for a drip irrigation remote control system (DIRCS) utilizing 5G-IoT technology alongside a mobile application was introduced. Additionally, Tang et al. (2021) (Tang et al., 2021) demonstrated significant benefits achieved through the implementation of IoT, including a 20% reduction in labor force, a corresponding 20% decrease in pesticide usage, and optimized utilization of water resources and fertilizers (Yu et al., 2021). Figure 22 visually depicts various applications of the 5G network in digital agriculture, illustrating the connectivity links between different sections. These applications showcase the potential of 5G technology to revolutionize agricultural practices, enabling efficient remote control and monitoring systems that enhance productivity while promoting sustainable resource management.
Figure 22. Selected applications of 5G technology in digital agriculture for improving crop production and increasing efficiency.
The deployment of the 5G mobile network is currently underway in some developed countries, including the United States, the United Kingdom, Germany, South Korea, Japan, and China. However, the initiation of 5G network deployment in many least developed countries is anticipated to require a significantly longer timeframe (Rahman et al., 2021). While the 5G network offers advantages in wireless communication, ensuring uninterrupted connectivity, there remain substantial challenges such as reducing interference, minimizing latency, optimizing power consumption, and enhancing data rates (Sah et al., 2022). Despite assurances from 5G service providers regarding data integrity, confidentiality, and availability, security remains a critical concern that necessitates attention (Humayun et al., 2021). Moreover, the limited battery capacity of sensor nodes poses a challenge for achieving sustainable digital agriculture within a 5G framework. When sensor nodes exhaust their energy reserves, the data center becomes unable to capture environmental information, leading to potential disruptions in decision-making and action implementation (Chien et al., 2022). However, nevertheless these challenges, IoT-enabled precision smallholder farming holds significant promise for enhancing livelihoods and expediting the journey to self-reliance for low- and middle-income countries (Antony et al., 2020). By leveraging advancements in connectivity and data analytics, digital agriculture powered by 5G technology has the potential to revolutionize existing farming practices, and contributing to a more sustainable agriculture.
Digital twin (DT) is one of the trending solutions toward real-time evaluation, optimization, and predictive control of complex systemic process, which has been successfully implemented in various industrial fields including manufacturing (Kritzinger et al., 2018), construction (Korenhof et al., 2021), automotive (Vachálek et al., 2017), energy (Howard et al., 2020). Originated back in 2003 by Michael Grieves (Jones et al., 2020), digital twin is commonly described as consisting of real-world entity (i.e., a physical product, a process, or a machine component) that is interfaced with a virtual replication of that entity (i.e., a simulation model) via bi-directional data connections for feeding data and exchanging information between the two (Grieves and Vickers, 2017). In this concept, the physical system interacts with the digital counterpart within a centralized or cloud-based architecture in order to optimize the process, update control parameters, and generate predictive solutions for what-if scenarios. It should be noted that a system without a connection from the virtual object to the physical object is different from digital twin, and is called digital shadow (Elahi et al., 2022).
Digital Twins have been used successfully in agriculture for developing autonomous farming robots (Foldager et al., 2020), identification of plant pests and diseases in crop production (Pylianidis et al., 2021), stock monitoring of feed silos of livestock farms (Raba et al., 2021), and energy management in commercial greenhouses (Ashraf et al., 2021; Chaux et al., 2021; Howard et al., 2021). Compared to the industrial application, the agricultural use of DT is still limited, but has a high potential to be expanded in the near future. The main use cases of digital twin in agriculture are focused on predictive analytics, remote monitoring, resource optimization, and risk mitigation (Purcell et al., 2023). Examples includes studies on predictive models that simulate crop growths and soil conditions in order to improve fertilizing and irrigation (Skobelev et al., 2021), or IoT monitoring of plants health and environmental conditions and simulate difference scenarios such as disease outbreaks to mitigate potential losses (Tekinerdogan and Verdouw, 2020). Recently, digital twins have been adopted and employed as a framework in the automation process of commercial greenhouses and hydroponic farms with the objective of reducing energy cost and improving sustainability of the production. To run the digital twin, a connection between the virtual and the physical object is necessary, which includes a various range of sensors at and around the physical object, so the digital twin can realise and react in real-time to all internal and external impacts that have an effect on the behaviour of the physical object. These sensor data are then processed by the digital twin and compared with the physical object from the real world, often carried out with artificial intelligence and machine learning. In this way, the digital twin is always learning from its physical counterpart. Using this approach, it is possible to have the DT trained in a way that it can predict the physical reactions solely from the external impacts, which result in great opportunities such as perceiving failures before they occur or simulating different scenarios to optimize processes without any physical effort. The connections between the physical and virtual part can also exist the other way round, so the gathered data and the resulting predictions from the virtual part can be used to control and correct the actions of the physical part. An overview of the data architecture and concepts of applying DT for greenhouse crop production is shown in Figure 23 that involves three main tasks as energy control, microclimate control, and production changes. The goal is to monitor optimality degrees of microclimate parameters (Shamshiri, 2017), reduce energy inputs (Ahamed et al., 2019; Jain and Tiwari, 2002), and enhance all processes from the start of the incoming plants until the delivery.
Figure 23. Workflow and architecture of a digital twin approach for optimizing environmental control in greenhouse crop production under tropical lowland climate conditions (Source: Adaptive AgroTech).
Modern commercial indoor growing systems have embraced predictive models and model-reference adaptive controllers within their automation systems, leveraging feedback from wireless sensing or IoT monitoring platforms to overcome the challenges posed by conventional timer-based control methods. Through the utilization of digital twin concepts, real-time monitoring of parameters facilitates the training of AI-based algorithms such as machine learning controllers or the development of self-optimizing dynamic models (Shamshiri and Hameed, 2021; Shamshiri et al., 2020; Ashraf et al., 2021; Shamshiri H. C. M. et al., 2017; Shamshiri R. et al., 2017; Asfahan et al., 2021; Rezvani, 2021; Sultan et al., 2021), aimed at minimizing energy inputs while maximizing profitability. Figure 23 also illustrates the architecture of a digital twin designed for optimizing greenhouse environmental control, that continuously updates parameters based on various objective functions, including maximizing yield, profit, waste reduction, and minimizing energy consumption. In this framework, the control objective revolves around maintaining internal parameters close to predefined set-points by minimizing a cost function that drives the error between reference values and model values to zero. For microclimate parameters, the controller engages ventilation fans, shading covers, heating, or cooling systems to achieve optimal air temperature and relative humidity, corresponding to an optimal vapor pressure deficit. It should be noted that the dynamics of such systems are highly nonlinear, subject to variations in solar radiation, crop growth stages, covering materials, external conditions, and other disturbances, necessitating the self-tuning of control parameters to mitigate nonlinearities. To address these challenges, multiple wireless sensor nodes are deployed both inside and outside the greenhouse, transmitting measurements to a receiver board. A control algorithm is then employed to adjust the growth environment by activating pumps and other actuators, thereby ensuring optimal conditions for plant growth and productivity while minimizing resource consumption and waste.
Blockchain is an emerging digital technology that has the potential to revolutionize the way farming and food production is conducted by creating a decentralized and secure network, contributing to better transparency, traceability, and efficiency in the agricultural supply chain. Blockchain can be used to create a digital ledger that records all of the data generated by sensors and controllers. This data can then be used to make more informed decisions about planting, fertilizing, and harvesting crops. For example, growers can use blockchain-based smart contracts to automatically adjust the amount of fertilizer used in their fields by taking into account the soil’s nutrient content in order to reduce the amount needed and minimize environmental impact. A key application of this technology in digital agriculture is supply chain traceability, which means creating a digital ledger that records the entire history of a product, from farm to consumer. This can help to improve food safety, reduce the risk of fraud by tracking the origin of products, and ensure that they meet certain quality standards. Such information are required to improve the efficiency of supply chains, as it allows for better tracking of inventory and logistics. Additionally, by providing consumers with a transparent view of the entire supply chain, Blockchain leads to higher consumers’ trust in the products they are buying. In terms of farmers’ compensation, Blockchain can be used to create decentralized platforms where farmers can sell their products directly to consumers, bypassing intermediaries and increasing their profit margins. This also helps to ensure that farmers are paid a fair price for their products, rather than relying on intermediaries who may take a significant cut of the profits. For example, from farmers and seed seed to consumers’ shelf, a blockchain-based supply chain solution can track each process with unique identifiers as shown in Figure 24 and create a digitally traceable end-to-end journey.
Another potential application of blockchain technology in agricultural robotics is the use of autonomous drones and other robots. Blockchain can be used to create a secure and decentralized network that allows drones and robots to communicate and share data in real-time. This can help to improve efficiency, reduce costs, and minimize human error in the agricultural supply chain. For example, drones can be used to survey crops and identify areas that require attention, while robots can be used to perform tasks such as planting, harvesting, and maintaining equipment. In addition to these applications, blockchain technology can be used to improve the way that agricultural land is managed. For example, it can be used to create an immutable record of land ownership, reducing disputes and increasing transparency in land transactions. Additionally, blockchain can be used to create a digital record of land use, making it easier for farmers to access government subsidies and other benefits. In addition, it can help to reduce environmental impact, optimize crop yields, and increase revenue potential for farmers. This is particularly important in countries where land ownership records are often poorly maintained or subject to corruption. It should be noted that while blockchain is considered a promising technology towards a transparent supply chain of food, there are still many barriers and challenges that hinder its wider acceptance among farmers and producers. These challenges are mainly due to technical aspects and limitations in the existing infrastructure, as well as education, policies, and regulations. Nevertheless, it is widely discussed that when successfully implemented, blockchain has the potential to create a more efficient, transparent, and secure supply chain. This reduces environmental impact and optimize crop yields, making agriculture more sustainable and profitable for farmers.
While the highlighted technological solutions play a significant role in the digitalization of agriculture, there exists several limitations and barriers such as high costs that farmers, especially those operating on tighter budgets, must address to ensure broad acceptance, adoption, and utilization. For example, farmers should consider the return on investment (ROI) (Griffin et al., 2018) associated with deploying expensive 5G infrastructure (van Hilten and Wolfert, 2022), autonomous electric tractors and robots (Rose et al., 2021), and IoT devices (Liu and Wu, 2021), alongside exploring potential subsidies or financial support mechanisms. For ROI calculations, factors such as reduced labor costs, optimized resource utilization (such as water and fertilizers) (Sandor et al., 2022), minimized waste, and enhanced decision-making should be taken into account. Robotics, wireless automation, and live monitoring systems can provide excellent insights into crop health to prevent losses, as well as targeted application of inputs for cost savings and yield improvements. Therefore calculating the ROI should involve evaluating not only the initial investment but also the long-term savings and increased productivity they offer. In addition, challenges related to the reliability and scalability of current technologies pose significant concerns to their widespread adoption.
Looking to the future, potential breakthroughs in digital agriculture involve advancements in AI and machine learning algorithms for predictive modeling and decision support (Aworka et al., 2022), the integration of Blockchain technology for transparent and traceable supply chains (Kamilaris et al., 2019), and the development of biotechnology solutions for crop improvement and pest management (Steinwand and Ronald, 2020). Additionally, the continued expansion of rural connectivity and the adoption of 5G technology is expected to further accelerate the digital transformation, enabling real-time data exchange even in remote areas. Although this transition to highly digitalized farming practices, particularly for those in developing countries, is still a significant challenge, but it can be facilitated by the availability of affordable hardware and infrastructure, customized solutions, training centers, and feedback mechanisms. It should be noted that this transition carries social and cultural implications, especially for rural communities deeply rooted in traditional methods, leading to a restructuring of their economies, potentially changing existing employment patterns. For instance, as fieldworks become more automated and data-driven, there may be a shift away from labor-intensive tasks, hence impacting the roles of seasonal workforce and their integration within local communities. This results in both opportunities and challenges, such as the creation of new skilled jobs in technology-related fields, as well as the displacement of workers who lack the necessary digital literacy or access to training opportunities. While some farmers may benefit from the increased productivity and efficiency enabled by digital tools, others struggle to afford or access the necessary technology and training. This can divide and widen socioeconomic inequalities within rural communities, reinforcing disparities between large commercial farms and small-scale or subsistence farmers. Preserving and honoring these cultural legacies while simultaneously embracing innovation pose a delicate balancing act for rural communities undergoing digital transformation. To this aim, developing robots, sensors, mobile apps, and software that are compatible with low-resource settings and support multiple languages, or organizing community-based hands-on workshops, peer-to-peer learning networks, and collaboration between research institutions for enhancing digital literacy will accelerate the accessibility of technology to a broader range of farmers irrespective of their geographic location or socioeconomic status.
The digitalization of agriculture is revolutionizing the way crops are produced and food is secured. The use of cutting-edge technologies such as robotics, computer vision, IoT, 5G, digital twin, and blockchain has allowed farmers to make more informed decisions, optimize crop yields, and reduce costs. This has led to more sustainable and efficient agriculture, which is crucial for ensuring food security in an increasingly populated world. The use of robotics in agriculture has increased efficiency and reduced labor costs, while computer vision and IoT have allowed for real-time monitoring and data collection. Whether it is through the use of drones for crop scouting, autonomous tractors for tilling and planting, or robot manipulators for harvesting, agricultural robots are changing the way farming activities have been conducted for decades. The integration of 5G networks has improved connectivity and data transfer speeds, making it easier for farmers to access information and make decisions. Future trends in this field shows that new concepts such as digital twin allows for virtual testing and simulations, providing a cost-effective way for farmers to make informed decisions. In addition, blockchain technology has the potential to improve traceability and food safety by providing a secure and transparent way to track the movement of crops from the farm to the consumer. However, the widespread adoption of these technologies in agriculture is not without its challenges and limitations. Network coverage and connectivity, data management and storage, security and privacy, cost, interoperability and integration, and regulation and standards are just some of the challenges that were highlighted in this paper that need to be overcome. To address these challenges and promote the acceptance of digital technologies in agriculture, it is important for all stakeholders, including governments, industry, and the research community, to collaborate and work together. Governments can play a key role by providing funding and support for the development and implementation of these technologies. Industry can help by investing in research and development and providing solutions to the challenges faced by farmers. The research community can contribute by conducting studies to better understand the limitations and challenges of these technologies and exploring new and innovative solutions. In conclusion, with the right support and investments, digital agriculture has the potential to make a significant contribution to transform crop production into a more sustainable and efficient system that can ensure food security for generations to come. Future studies may involve analyzing of the socio-economic impacts of digital technologies in agriculture, such as the impacts of digitalization on farmers and rural communities, the accessibility and affordability of the existing solutions, and the policies and regulations that support or hinder the adoption of future developments.
RS: Conceptualization, Data curation, Formal Analysis, Funding acquisition, Investigation, Methodology, Project administration, Resources, Software, Supervision, Validation, Visualization, Writing–original draft, Writing–review and editing. BS: Conceptualization, Supervision, Validation, Writing–review and editing. CW: Conceptualization, Funding acquisition, Supervision, Writing–review and editing. JF: Conceptualization, Supervision, Validation, Writing–review and editing. RK: Conceptualization, Methodology, Supervision, Writing–review and editing. MS: Conceptualization, Writing–review and editing. SR: Writing–review and editing. DB: Writing–review and editing. MY: Conceptualization, Writing–review and editing. IH: Conceptualization, Supervision, Writing–review and editing.
The author(s) declare that financial support was received for the research, authorship, and/or publication of this article. This study is based on research funded by: the European Innovation Partnership Program for Agricultural Productivity and Sustainability (EIP-AGRI); European Agricultural Fund for Rural Development (EFRE); Project SunBot; GAN/FKZ: 204018000001/80173118 the Federal Ministry for Digital and Traffic (BMDV), Federal Agency for Administrative Services (BAV); Funding measure: 5G implementation funding as part of the 5G innovation program; Project foodChain; GAN/FKZ: 45FGU119_E the Federal Ministry of Food and Agriculture (BMEL); Federal Agency for Agriculture and Food (BLE); Funding program Big Data in agriculture - Innovation funding; Project FungiDetect; GAN/FKZ 2815705615 the Federal Ministry of Education and Research (BMBF); Funding agency Project Management Jülich (PtJ); Funding framework Agricultural systems for the future; Project DAKIS; GAN/ FKZ 031B0513A.
The technical support from Maryam Behjati and the laboratory facilities from Adaptive AgroTech are duly acknowledged.
The authors declare that the research was conducted in the absence of any commercial or financial relationships that could be construed as a potential conflict of interest.
All claims expressed in this article are solely those of the authors and do not necessarily represent those of their affiliated organizations, or those of the publisher, the editors and the reviewers. Any product that may be evaluated in this article, or claim that may be made by its manufacturer, is not guaranteed or endorsed by the publisher.
Abbas, A., Waseem, M., Ahmad, R., khan, K. A., Zhao, C., and Zhu, J. (2022a). Sensitivity analysis of greenhouse gas emissions at farm level: case study of grain and cash crops. Environ. Sci. Pollut. Res. 29 (54), 82559–82573. doi:10.1007/s11356-022-21560-9
Abbas, A., Zhao, C., Waseem, M., Ahmed khan, K., and Ahmad, R. (2022b). Analysis of energy input–output of farms and assessment of greenhouse gas emissions: a case study of cotton growers. Front. Environ. Sci. 9. doi:10.3389/fenvs.2021.826838
Adamchuk, V., Ji, W., Rossel, R. V., Gebbers, R., and Tremblay, N. (2018). “Proximal soil and plant sensing,” in Precision agriculture basics, 119–140.
Ahamed, M. S., Guo, H., and Tanino, K. (2019). Energy saving techniques for reducing the heating cost of conventional greenhouses. Biosyst. Eng. 178, 9–33. doi:10.1016/j.biosystemseng.2018.10.017
Ahamed, M. S., Sultan, M., Shamshiri, R. R., Rahman, M. M., Aleem, M., and Balasundram, S. K. (2023). Present status and challenges of fodder production in controlled environments: a review. Smart Agric. Technol. 3, 100080. doi:10.1016/j.atech.2022.100080
Antony, A. P., Leith, K., Jolley, C., Lu, J., and Sweeney, D. J. (2020). A review of practice and implementation of the internet of Things (IoT) for smallholder agriculture. Sustainability 12 (9), 3750. doi:10.3390/su12093750
Asfahan, H. M., Sajjad, U., Sultan, M., Hussain, I., Hamid, K., Ali, M., et al. (2021). Artificial intelligence for the prediction of the thermal performance of evaporative cooling systems. Energies 14 (13), 3946. doi:10.3390/en14133946
Ashraf, H., Sultan, M., Shamshiri, R. R., Abbas, F., Farooq, M., Sajjad, U., et al. (2021). Dynamic evaluation of desiccant dehumidification evaporative cooling options for greenhouse air-conditioning application in multan (Pakistan). Energies 14 (4), 1097. doi:10.3390/en14041097
Atefi, A., Ge, Y., Pitla, S., and Schnable, J. (2021). Robotic technologies for high-throughput plant phenotyping: contemporary reviews and future perspectives. Front. Plant Sci. 12, 611940. doi:10.3389/fpls.2021.611940
Aworka, R., Cedric, L. S., Adoni, W. Y. H., Zoueu, J. T., Mutombo, F. K., Kimpolo, C. L. M., et al. (2022). Agricultural decision system based on advanced machine learning models for yield prediction: case of East African countries. Smart Agric. Technol. 2, 100048. doi:10.1016/j.atech.2022.100048
Balasundram, S. K., Shamshiri, R. R., Sridhara, S., and Rizan, N. (2023). The role of digital agriculture in mitigating climate change and ensuring food security: an overview. Sustainability 15 (6), 5325. doi:10.3390/su15065325
Barth, R., Hemming, J., and van Henten, E. J. (2016). Design of an eye-in-hand sensing and servo control framework for harvesting robotics in dense vegetation. Biosyst. Eng. 146, 71–84. doi:10.1016/j.biosystemseng.2015.12.001
Basavegowda, D. H., Mosebach, P., Schleip, I., and Weltzien, C. (2022). “Indicator plant species detection in grassland using EfficientDet object detector,” in 42. GIL-Jahrestagung, Künstliche Intelligenz in der Agrar-und Ernährungswirtschaft.
Basso, B., and Antle, J. (2020). Digital agriculture to design sustainable agricultural systems. Nat. Sustain. 3 (4), 254–256. doi:10.1038/s41893-020-0510-0
Bates, J. S., Montzka, C., Schmidt, M., and Jonard, F. (2021). Estimating canopy density parameters time-series for winter wheat using UAS mounted LiDAR. Remote Sens. 13 (4), 710. doi:10.3390/rs13040710
Bergerman, M., Billingsley, J., Reid, J., and van Henten, E. (2016). in Robotics in agriculture and forestry BT - springer handbook of robotics. Editors B. Siciliano, and O. Khatib (Cham: Springer International Publishing), 1463–1492.
Caporaso, N., Whitworth, M. B., Fowler, M. S., and Fisk, I. D. (2018). Hyperspectral imaging for non-destructive prediction of fermentation index, polyphenol content and antioxidant activity in single cocoa beans. Food Chem. 258, 343–351. doi:10.1016/j.foodchem.2018.03.039
Chaux, J. D., Sanchez-Londono, D., and Barbieri, G. (2021). A digital twin architecture to optimize productivity within controlled environment agriculture. Appl. Sci. 11 (19), 8875. doi:10.3390/app11198875
Chen, S., Wang, J., Zhang, T., Hu, Z., and Zhou, G. (2021). Warming and straw application increased soil respiration during the different growing seasons by changing crop biomass and leaf area index in a winter wheat-soybean rotation cropland. Geoderma 391, 114985. doi:10.1016/j.geoderma.2021.114985
Chen, Y.-E., Cui, J.-M., Su, Y.-Q., Yuan, S., Yuan, M., and Zhang, H.-Y. (2015). Influence of stripe rust infection on the photosynthetic characteristics and antioxidant system of susceptible and resistant wheat cultivars at the adult plant stage. Front. plant Sci. 6, 779. doi:10.3389/fpls.2015.00779
Chien, W.-C., Hassan, M. M., Alsanad, A., and Fortino, G. (2022). UAV-assist joint wireless power transfer and data collection mechanism for sustainable precision agriculture in 5G. IEEE Micro 42, 25–32. doi:10.1109/MM.2021.3122553
Cisternas, I., Velásquez, I., Caro, A., and Rodríguez, A. (2020). Systematic literature review of implementations of precision agriculture. Comput. Electron. Agric. 176, 105626. doi:10.1016/j.compag.2020.105626
Comba, L., Biglia, A., Ricauda Aimonino, D., Tortia, C., Mania, E., Guidoni, S., et al. (2020). Leaf Area Index evaluation in vineyards using 3D point clouds from UAV imagery. Precis. Agric. 21 (4), 881–896. doi:10.1007/s11119-019-09699-x
Córcoles, J. I., Ortega, J. F., Hernández, D., and Moreno, M. A. (2013). Estimation of leaf area index in onion (Allium cepa L.) using an unmanned aerial vehicle. Biosyst. Eng. 115 (1), 31–42. doi:10.1016/j.biosystemseng.2013.02.002
Crichton, S., Shrestha, L., Hurlbert, A., and Sturm, B. (2018). Use of hyperspectral imaging for the prediction of moisture content and chromaticity of raw and pretreated apple slices during convection drying. Dry. Technol. 36 (7), 804–816. doi:10.1080/07373937.2017.1356847
Crichton, S. O. J., Kirchner, S. M., Porley, V., Retz, S., von Gersdorff, G., Hensel, O., et al. (2017). Classification of organic beef freshness using VNIR hyperspectral imaging. Meat Sci. 129, 20–27. doi:10.1016/j.meatsci.2017.02.005
da Silva, D. Q., Dos Santos, F. N., Sousa, A. J., and Filipe, V. (2021). Visible and thermal image-based trunk detection with deep learning for forestry mobile robotics. J. Imaging 7 (9), 176. doi:10.3390/jimaging7090176
de Camargo, T., Schirrmann, M., Landwehr, N., Dammer, K.-H., and Pflanz, M. (2021). Optimized deep learning model as a basis for fast UAV mapping of weed species in winter wheat crops. Remote Sens. 13 (9), 1704. doi:10.3390/rs13091704
Duong, L. N. K., Al-Fadhli, M., Jagtap, S., Bader, F., Martindale, W., Swainson, M., et al. (2020). A review of robotics and autonomous systems in the food industry: from the supply chains perspective. Trends Food Sci. Technol. 106, 355–364. doi:10.1016/j.tifs.2020.10.028
Elahi, E., Li, G., Han, X., Zhu, W., Liu, Y., Cheng, A., et al. (2024). Decoupling livestock and poultry pollution emissions from industrial development: a step towards reducing environmental emissions. J. Environ. Manag. 350, 119654. doi:10.1016/j.jenvman.2023.119654
Elahi, E., Khalid, Z., and Zhang, Z. (2022). Understanding farmers’ intention and willingness to install renewable energy technology: a solution to reduce the environmental emissions of agriculture. Appl. Energy 309, 118459. doi:10.1016/j.apenergy.2021.118459
Elfferich, J. F., Dodou, D., and Santina, C. D. (2022). Soft robotic grippers for crop handling or harvesting: a review. IEEE Access 10, 75428–75443. doi:10.1109/access.2022.3190863
Emmi, L., and Gonzalez-de-Santos, P. (2017). “Mobile robotics in arable lands: current state and future trends,” in 2017 European conference on mobile robots (ECMR), 1–6.
Fielke, S., Taylor, B., and Jakku, E. (2020). Digitalisation of agricultural knowledge and advice networks: a state-of-the-art review. Agric. Syst. 180, 102763. doi:10.1016/j.agsy.2019.102763
Foldager, F. F., Thule, C., Balling, O., and Larsen, P. (2020). “Towards a digital twin-modelling an agricultural vehicle,” in International symposium on leveraging applications of formal methods, 109–123.
Fountas, S., Malounas, I., Athanasakos, L., Avgoustakis, I., and Espejo-Garcia, B. (2022). AI-assisted vision for agricultural robots. AgriEngineering 4 (3), 674–694. doi:10.3390/agriengineering4030043
Franchi, N., Fettweis, G. P., and Herlitzius, T. (2021). The significance of the Tactile Internet and 5G for digital agriculture. A. T. - Autom. 69 (4), 281–286. doi:10.1515/auto-2020-0130
Friha, O., Ferrag, M. A., Shu, L., Maglaras, L., and Wang, X. (2021). Internet of Things for the future of smart agriculture: a comprehensive survey of emerging technologies. IEEE/CAA J. Automatica Sinica 8 (4), 718–752. doi:10.1109/jas.2021.1003925
Garcerá, C., Doruchowski, G., and Chueca, P. (2021). Harmonization of plant protection products dose expression and dose adjustment for high growing 3D crops: a review. Crop Prot. 140, 105417. doi:10.1016/j.cropro.2020.105417
Gené-Mola, J., Gregorio, E., Auat Cheein, F., Guevara, J., Llorens, J., Sanz-Cortiella, R., et al. (2020). Fruit detection, yield prediction and canopy geometric characterization using LiDAR with forced air flow. Comput. Electron. Agric. 168, 105121. doi:10.1016/j.compag.2019.105121
Gerhards, R., Andújar Sanchez, D., Hamouz, P., Peteinatos, G. G., Christensen, S., and Fernandez-Quintanilla, C. (2022). Advances in site-specific weed management in agriculture—a review. Weed Res. 62 (2), 123–133. doi:10.1111/wre.12526
Giannoccaro, N. I., Persico, G., Strazzella, S., Lay-Ekuakille, A., and Visconti, P. (2020). A system for optimizing fertilizer dosing in innovative smart fertigation pipelines: modeling, construction, testing and control. Int. J. Precis. Eng. Manuf. 21 (8), 1581–1596. doi:10.1007/s12541-020-00349-1
Grieves, M., and Vickers, J. (2017). “Digital twin: mitigating unpredictable, undesirable emergent behavior in complex systems,” in Transdisciplinary perspectives on complex systems (Springer), 85–113.
Griffin, T. W., Shockley, J. M., and Mark, T. B. (2018). “Economics of precision farming,” in Precision agriculture basics, 221–230.
Guo, W., Carroll, M. E., Singh, A., Swetnam, T. L., Merchant, N., Sarkar, S., et al. (2021a). UAS-based plant phenotyping for research and breeding applications. Plant Phenomics 2021, 9840192. doi:10.34133/2021/9840192
Guo, Y., Chen, S., Wu, Z., Wang, S., Robin Bryant, C., Senthilnath, J., et al. (2021b). Integrating spectral and textural information for monitoring the growth of pear trees using optical images from the UAV platform. Remote Sens. 13 (9), 1795. doi:10.3390/rs13091795
Han, L., Yang, G., Yang, H., Xu, B., Li, Z., and Yang, X. (2018). Clustering field-based maize phenotyping of plant-height growth and canopy spectral dynamics using a UAV remote-sensing approach. Front. plant Sci. 9, 1638. doi:10.3389/fpls.2018.01638
Hardin, P. J., and Jensen, R. R. (2011). Small-scale unmanned aerial vehicles in environmental remote sensing: challenges and opportunities. GIScience Remote Sens. 48 (1), 99–111. doi:10.2747/1548-1603.48.1.99
He, K., Zhang, X., Ren, S., and Sun, J. (2016). “Deep residual learning for image recognition,” in Proceedings of the IEEE conference on computer vision and pattern recognition, 770–778.
Herrero-Huerta, M., González-Aguilera, D., Rodriguez-Gonzalvez, P., and Hernández-López, D. (2015). Vineyard yield estimation by automatic 3D bunch modelling in field conditions. Comput. Electron. Agric. 110, 17–26. doi:10.1016/j.compag.2014.10.003
Hobart, M., Pflanz, M., Weltzien, C., and Schirrmann, M. (2020). Growth height determination of tree walls for precise monitoring in apple fruit production using UAV photogrammetry. Remote Sens. 12 (10), 1656. doi:10.3390/rs12101656
Howard, D. A., Ma, Z., and Jørgensen, B. N. (2020). “Digital twin framework for energy efficient greenhouse industry 4.0,” in International symposium on ambient intelligence, 293–297.
Howard, D. A., Ma, Z., Veje, C., Clausen, A., Aaslyng, J. M., and Jørgensen, B. N. (2021). Greenhouse industry 4.0–digital twin technology for commercial greenhouses. Energy Inf. 4 (2), 37–13. doi:10.1186/s42162-021-00161-9
Humayun, M., Hamid, B., Jhanjhi, N. Z., Suseendran, G., and Talib, M. N. (2021). 5G network security issues, challenges, opportunities and future directions: a survey. J. Phys. Conf. Ser. 1979 (1), 012037. doi:10.1088/1742-6596/1979/1/012037
Jain, D., and Tiwari, G. N. (2002). Modeling and optimal design of evaporative cooling system in controlled environment greenhouse. Energy Convers. Manag. 43 (16), 2235–2250. doi:10.1016/s0196-8904(01)00151-0
Jiménez-Brenes, F. M., López-Granados, F., de Castro, A. I., Torres-Sánchez, J., Serrano, N., and Peña, J. M. (2017). Quantifying pruning impacts on olive tree architecture and annual canopy growth by using UAV-based 3D modelling. Plant Methods 13 (1), 55. doi:10.1186/s13007-017-0205-3
Jones, D., Snider, C., Nassehi, A., Yon, J., and Hicks, B. (2020). Characterising the Digital Twin: a systematic literature review. CIRP J. Manuf. Sci. Technol. 29, 36–52. doi:10.1016/j.cirpj.2020.02.002
Jurado, J. M., Ortega, L., Cubillas, J. J., and Feito, F. R. (2020). Multispectral mapping on 3D models and multi-temporal monitoring for individual characterization of olive trees. Remote Sens. 12 (7), 1106. doi:10.3390/rs12071106
Kamilaris, A., Fonts, A., and Prenafeta-Boldύ, F. X. (2019). The rise of blockchain technology in agriculture and food supply chains. Trends Food Sci. Technol. 91, 640–652. doi:10.1016/j.tifs.2019.07.034
Karpyshev, P., Ilin, V., Kalinov, I., Petrovsky, A., and Tsetserukou, D. (2021). “Autonomous mobile robot for apple plant disease detection based on cnn and multi-spectral vision system,” in 2021 IEEE/SICE international symposium on system integration (SII), 157–162.
Khanna, A., and Kaur, S. (2019). Evolution of internet of Things (IoT) and its significant impact in the field of precision agriculture. Comput. Electron. Agric. 157, 218–231. doi:10.1016/j.compag.2018.12.039
Khujamatov, K. E., Toshtemirov, T. K., Lazarev, A. P., and Raximjonov, Q. T. (2021). “IoT and 5G technology in agriculture,” in 2021 international conference on information science and communications technologies (ICISCT), 1–6.
Klaina, H., Guembe, I. P., Lopez-Iturri, P., Campo-Bescós, M. Á., Azpilicueta, L., Aghzout, O., et al. (2022). Analysis of low power wide area network wireless technologies in smart agriculture for large-scale farm monitoring and tractor communications. Measurement 187, 110231. doi:10.1016/j.measurement.2021.110231
Knoth, C., Klein, B., Prinz, T., and Kleinebecker, T. (2013). Unmanned aerial vehicles as innovative remote sensing platforms for high-resolution infrared imagery to support restoration monitoring in cut-over bogs. Appl. Veg. Sci. 16 (3), 509–517. doi:10.1111/avsc.12024
Kootstra, G., Bender, A., Perez, T., and van Henten, E. J. (2020). in Robotics in agriculture BT - encyclopedia of robotics. Editors M. H. Ang, O. Khatib, and B. Siciliano (Berlin, Heidelberg: Springer Berlin Heidelberg), 1–19.
Korenhof, P., Blok, V., and Kloppenburg, S. (2021). Steering representations—towards a critical understanding of digital twins. Philosophy Technol. 34 (4), 1751–1773. doi:10.1007/s13347-021-00484-1
Kritzinger, W., Karner, M., Traar, G., Henjes, J., and Sihn, W. (2018). Digital Twin in manufacturing: a categorical literature review and classification. IFAC-PapersOnLine 51 (11), 1016–1022. doi:10.1016/j.ifacol.2018.08.474
Kurtser, P., Ringdahl, O., Rotstein, N., Berenstein, R., and Edan, Y. (2020). In-field grape cluster size assessment for vine yield estimation using a mobile robot and a consumer level RGB-D camera. IEEE Robotics Automation Lett. 5 (2), 2031–2038. doi:10.1109/lra.2020.2970654
Lajoie-OMalley, A., Bronson, K., van der Burg, S., and Klerkx, L. (2020). The future (s) of digital agriculture and sustainable food systems: an analysis of high-level policy documents. Ecosyst. Serv. 45, 101183. doi:10.1016/j.ecoser.2020.101183
LeCun, Y., Bengio, Y., and Hinton, G. (2015). Deep learning. nature 521 (7553), 436–444. doi:10.1038/nature14539
Lei, L., Qiu, C., Li, Z., Han, D., Han, L., Zhu, Y., et al. (2019). Effect of leaf occlusion on leaf area index inversion of maize using UAV–LiDAR data. Remote Sens. 11 (9), 1067. doi:10.3390/rs11091067
Lendzioch, T., Langhammer, J., and Jenicek, M. (2019). Estimating snow depth and leaf area index based on UAV digital photogrammetry. Sensors Switz. 19 (5), 1027. doi:10.3390/s19051027
Li, M., Shamshiri, R. R., Schirrmann, M., and Weltzien, C. (2021). Impact of camera viewing angle for estimating leaf parameters of wheat plants from 3D point clouds. Agriculture 11 (6), 563. doi:10.3390/agriculture11060563
Li, M., Shamshiri, R. R., Schirrmann, M., Weltzien, C., Shafian, S., and Laursen, M. S. (2022). UAV oblique imagery with an adaptive micro-terrain model for estimation of leaf area index and height of maize canopy from 3D point clouds. Remote Sens. 14 (3), 585. doi:10.3390/rs14030585
Li, T., and Li, D. (2020). “Prospects for the application of 5G technology in agriculture and rural areas,” in 2020 5th international conference on mechanical, control and computer engineering (ICMCCE), 2176–2179.
Lin, N., Wang, X., Zhang, Y., Hu, X., and Ruan, J. (2020). Fertigation management for sustainable precision agriculture based on Internet of Things. J. Clean. Prod. 277, 124119. doi:10.1016/j.jclepro.2020.124119
Linchant, J., Lisein, J., Semeki, J., Lejeune, P., and Vermeulen, C. (2015). Are unmanned aircraft systems (UASs) the future of wildlife monitoring? A review of accomplishments and challenges. Mammal. Rev. 45 (4), 239–252. doi:10.1111/mam.12046
Liu, S., and Wu, Y. (2021). [Retracted] economic benefit evaluation and analysis based on intelligent agriculture internet of Things. J. Math. 2021, 1. doi:10.1155/2024/9894065
Lu, Y., and Young, S. (2020). A survey of public datasets for computer vision tasks in precision agriculture. Comput. Electron. Agric. 178, 105760. doi:10.1016/j.compag.2020.105760
Ma, J., Sun, D.-W., Pu, H., Cheng, J.-H., and Wei, Q. (2019). Advanced techniques for hyperspectral imaging in the food industry: principles and recent applications. Annu. Rev. food Sci. Technol. 10, 197–220. doi:10.1146/annurev-food-032818-121155
Ma, R., Teo, K. H., Shinjo, S., Yamanaka, K., and Asbeck, P. M. (2017). A GaN PA for 4G LTE-advanced and 5G: meeting the telecommunication needs of various vertical sectors including automobiles, robotics, health care, factory automation, agriculture, education, and more. IEEE Microw. Mag. 18 (7), 77–85. doi:10.1109/mmm.2017.2738498
Magalhães, S. A., Moreira, A. P., dos Santos, F. N., and Dias, J. (2022). Active perception fruit harvesting robots — a systematic review. J. Intelligent Robotic Syst. 105 (1), 14. doi:10.1007/s10846-022-01595-3
Massah, J., Vakilian, K. A., Shabanian, M., and Shariatmadari, S. M. (2021). Design, development, and performance evaluation of a robot for yield estimation of kiwifruit. Comput. Electron. Agric. 185, 106132. doi:10.1016/j.compag.2021.106132
Mathews, A., and Jensen, J. (2013). Visualizing and quantifying vineyard canopy LAI using an unmanned aerial vehicle (UAV) collected high density structure from motion point cloud. Remote Sens. 5 (5), 2164–2183. doi:10.3390/rs5052164
Md Saleh, R., Kulig, B., Arefi, A., Hensel, O., and Sturm, B. (2022). Prediction of total carotenoids, color, and moisture content of carrot slices during hot air drying using non-invasive hyperspectral imaging technique. J. Food Process. Preserv. 46 (9), e16460. doi:10.1111/jfpp.16460
Meshram, A. T., V Vanalkar, A., Kalambe, K. B., and Badar, A. M. (2022). Pesticide spraying robot for precision agriculture: a categorical literature review and future trends. J. Field Robotics 39 (2), 153–171. doi:10.1002/rob.22043
Mier, G., Valente, J., and de Bruin, S. (2023). Fields2Cover: an open-source coverage path planning library for unmanned agricultural vehicles. IEEE Robotics Automation Lett. 8 (4), 2166–2172. doi:10.1109/lra.2023.3248439
Milella, A., Reina, G., and Nielsen, M. (2019). A multi-sensor robotic platform for ground mapping and estimation beyond the visible spectrum. Precis. Agric. 20 (2), 423–444. doi:10.1007/s11119-018-9605-2
Mishra, P., Polder, G., and Vilfan, N. (2020). Close range spectral imaging for disease detection in plants using autonomous platforms: a review on recent studies. Curr. Robot. Rep. 1 (2), 43–48. doi:10.1007/s43154-020-00004-7
Mourad, R., Jaafar, H., Anderson, M., and Gao, F. (2020). Assessment of leaf area index models using harmonized landsat and sentinel-2 surface reflectance data over a semi-arid irrigated landscape. Remote Sens. 12 (19), 3121. doi:10.3390/rs12193121
Navas, E., Shamshiri, R. R., Dworak, V., Weltzien, C., and Fernández, R. (2024). Soft gripper for small fruits harvesting and pick and place operations. Front. Robotics AI 10, 1330496. doi:10.3389/frobt.2023.1330496
Ndisya, J., Gitau, A., Mbuge, D., Arefi, A., Bădulescu, L., Pawelzik, E., et al. (2021). Vis-nir hyperspectral imaging for online quality evaluation during food processing: a case study of hot air drying of purple-speckled cocoyam (colocasia esculenta (l.) schott). Processes 9 (10), 1804. doi:10.3390/pr9101804
Oliveira, L. F. P., Moreira, A. P., and Silva, M. F. (2021a). Advances in agriculture robotics: a state-of-the-art review and challenges ahead. Robotics 10 (2), 52. doi:10.3390/robotics10020052
Oliveira, L. F. P., Moreira, A. P., and Silva, M. F. (2021b). Advances in forest robotics: a state-of-the-art survey. Robotics 10 (2), 53. doi:10.3390/robotics10020053
OMahony, N., Campbell, S., Carvalho, A., Harapanahalli, S., Hernandez, G. V., Krpalkova, L., et al. (2020). Deep learning vs. traditional computer vision. Adv. Comput. Vis. Proc. 2019 Comput. Vis. Conf. (CVC) 1 (1), 128–144. doi:10.1007/978-3-030-17795-9_10
Pathak, H. S., Brown, P., and Best, T. (2019). A systematic literature review of the factors affecting the precision agriculture adoption process. Precis. Agric. 20 (6), 1292–1316. doi:10.1007/s11119-019-09653-x
Pflanz, M., Nordmeyer, H., and Schirrmann, M. (2018). Weed mapping with UAS imagery and a bag of visual words based image classifier. Remote Sens. 10 (10), 1530. doi:10.3390/rs10101530
Popescu, D., Stoican, F., Stamatescu, G., Ichim, L., and Dragana, C. (2020). Advanced UAV–WSN system for intelligent monitoring in precision agriculture. Sensors 20 (3), 817. doi:10.3390/s20030817
Purcell, W., Neubauer, T., and Mallinger, K. (2023). Digital Twins in agriculture: challenges and opportunities for environmental sustainability. Curr. Opin. Environ. Sustain. 61, 101252. doi:10.1016/j.cosust.2022.101252
Pylianidis, C., Osinga, S., and Athanasiadis, I. N. (2021). Introducing digital twins to agriculture. Comput. Electron. Agric. 184, 105942. doi:10.1016/j.compag.2020.105942
Quigley, M., Conley, K., Gerkey, B., Faust, J., Foote, T., Leibs, J., et al. (2009). ROS: an open-source robot operating system. ICRA workshop on open source software 3.
Raba, D., Tordecilla, R. D., Copado, P., Juan, A. A., and Mount, D. (2021). A digital twin for decision making on livestock feeding. Inf. J. Appl. Anal. 52, 267–282. doi:10.1287/inte.2021.1110
Rahman, A., Arabi, S., and Rab, R. (2021). Feasibility and challenges of 5G network deployment in least developed countries (LDC). Wirel. Sens. Netw. 13 (1), 1–16. doi:10.4236/wsn.2021.131001
Ren, G., Lin, T., Ying, Y., Chowdhary, G., and Ting, K. C. (2020). Agricultural robotics research applicable to poultry production: a review. Comput. Electron. Agric. 169, 105216. doi:10.1016/j.compag.2020.105216
Rezvani, S. M., Abyaneh, H. Z., Shamshiri, R. R., Balasundram, S. K., Dworak, V., Goodarzi, M., et al. (2020). IoT-based sensor data fusion for determining optimality degrees of microclimate parameters in commercial greenhouse production of tomato. Sensors 20 (22), 6474. doi:10.3390/s20226474
Rezvani, S. M.-E.-D. (2021). “Greenhouse crop simulation models and microclimate control systems, A review,” in Next-generation greenhouses for food security (IntechOpen).
Rodrigo-Comino, J. (2018). Five decades of soil erosion research in ‘terroir’. The State-of-the-Art. Earth-Science Rev. 179, 436–447. doi:10.1016/j.earscirev.2018.02.014
Roosjen, P. P. J., Brede, B., Suomalainen, J. M., Bartholomeus, H. M., Kooistra, L., and Clevers, J. G. P. W. (2018). Improved estimation of leaf area index and leaf chlorophyll content of a potato crop using multi-angle spectral data – potential of unmanned aerial vehicle imagery. Int. J. Appl. Earth Observation Geoinformation 66, 14–26. doi:10.1016/j.jag.2017.10.012
Rose, D. C., Lyon, J., de Boon, A., Hanheide, M., and Pearson, S. (2021). Responsible development of autonomous robotics in agriculture. Nat. Food 2 (5), 306–309. doi:10.1038/s43016-021-00287-9
Rübcke von Veltheim, F., and Heise, H. (2021). German farmers’ attitudes on adopting autonomous field robots: an empirical survey. Agriculture 11 (3), 216. doi:10.3390/agriculture11030216
Sah, M. B., Bindle, A., and Gulati, T. (2022). Issues and challenges in the implementation of 5G technology BT - computer networks and inventive communication technologies, 385–398.
Said Mohamed, E., Belal, A. A., Kotb Abd-Elmabod, S., El-Shirbeny, M. A., Gad, A., and Zahran, M. B. (2021). Smart farming for improving agricultural management. Egypt. J. Remote Sens. Space Sci. 24 (3), 971–981. doi:10.1016/j.ejrs.2021.08.007
Sandor, D., Karcher, D., and Richardson, M. (2022). Return on investment and water savings of add-on irrigation sensors for bermudagrass lawn irrigation in Northwest Arkansas. Crop, Forage & Turfgrass Manag. 8 (2), e20181. doi:10.1002/cft2.20181
Sanjeevi, P., Prasanna, S., Siva Kumar, B., Gunasekaran, G., Alagiri, I., and Vijay Anand, R. (2020). Precision agriculture and farming using Internet of Things based on wireless sensor network. Trans. Emerg. Telecommun. Technol. 31 (12), e3978. doi:10.1002/ett.3978
Santos, L. C., Santos, F. N., Pires, E. J. S., Valente, A., Costa, P., and Magalhães, S. (2020). “Path Planning for ground robots in agriculture: a short review,” in 2020 IEEE international conference on autonomous robot systems and competitions (ICARSC), 61–66.
Schirrmann, M., Landwehr, N., Giebel, A., Garz, A., and Dammer, K.-H. (2021). Early detection of stripe rust in winter wheat using deep residual neural networks. Front. Plant Sci. 12, 469689. doi:10.3389/fpls.2021.469689
Sha, Z., Wang, Y., Bai, Y., Zhao, Y., Jin, H., Na, Y., et al. (2018). Comparison of leaf area index inversion for grassland vegetation through remotely sensed spectra by unmanned aerial vehicle and field-based spectroradiometer. J. Plant Ecol. 12 (3), 395–408. doi:10.1093/jpe/rty036
Shahbazi, M., Théau, J., and Ménard, P. (2014). Recent applications of unmanned aerial imagery in natural resource management. GIScience Remote Sens. 51 (4), 339–365. doi:10.1080/15481603.2014.926650
Shamshiri, H. C. M., Redmond, R., Razif Mahadi, M., Thorp, K. R., and Wan Ismail, W. I. (2017a). Adaptive management framework for evaluating and adjusting microclimate parameters in tropical greenhouse crop production systems. Plant Eng.
Shamshiri, R. (2017). Measuring optimality degrees of microclimate parameters in protected cultivation of tomato under tropical climate condition. Meas. J. Int. Meas. Confed. 106, 236–244. doi:10.1016/j.measurement.2017.02.028
Shamshiri, R., Ehsani, R., Maja, J. M., and Roka, F. M. (2013). Determining machine efficiency parameters for a citrus canopy shaker using yield monitor data. Appl. Eng. Agric. 29 (1), 33–41. doi:10.13031/2013.42526
Shamshiri, R., and Ismail, W. I. W. (2013). Exploring GPS data for operational analysis of farm machinery. Res. J. Appl. Sci. Eng. Technol. 5 (12), 3281–3286. doi:10.19026/rjaset.5.4568
Shamshiri, R., van Beveren, P., Che Man, H., and Zakaria, A. J. (2017b). Dynamic assessment of air temperature for tomato (Lycopersicon esculentum mill) cultivation in a naturally ventilated net-screen greenhouse under tropical lowlands climate. J. Agric. Sci. Technol. 19 (1).
Shamshiri, R. R., Hameed, I. A., Balasundram, S. K., Ahmad, D., Weltzien, C., and Yamin, M. (2018c). “Fundamental research on unmanned aerial vehicles to support precision agriculture in oil palm plantations”, in Agricultural robots-fundamentals and application (London, United Kingdom: Rijeka: IntechOpen), 91–116.
Shamshiri, R. R. (2021). in Greenhouse automation using wireless sensors and IoT instruments integrated with artificial intelligence. Editor I. A. Hameed (Rijeka: IntechOpen). Ch. 1.
Shamshiri, R. R., A. Hameed, I., Pitonakova, L., Weltzien, C., K. Balasundram, S., J. Yule, I., et al. (2018b). Simulation software and virtual environments for acceleration of agricultural robotics: features highlights and performance comparison. Int. J. Agric. Biol. Eng. 11 (4), 12–20. doi:10.25165/j.ijabe.20181103.4032
Shamshiri, R. R., Bojic, I., van Henten, E., Balasundram, S. K., Dworak, V., Sultan, M., et al. (2020). Model-based evaluation of greenhouse microclimate using IoT-Sensor data fusion for energy efficient crop production. J. Clean. Prod. 263, 121303. doi:10.1016/j.jclepro.2020.121303
Shamshiri, R. R., Navas, E., Dworak, V., Auat Cheein, F. A., and Weltzien, C. (2024). A modular sensing system with CANBUS communication for assisted navigation of an agricultural mobile robot. Comput. Electron. Agric. 223, 109112. doi:10.1016/j.compag.2024.109112
Shamshiri, R. R., and Weltzien, C. (2021). “Development and field evaluation of a multichannel LoRa sensor for IoT monitoring in berry orchards,” in 41. GIL-jahrestagung, informations-und kommunikationstechnologie in kritischen zeiten.
Shamshiri, R. R., Weltzien, C., A. Hameed, I., J. Yule, I., E. Grift, T., K. Balasundram, S., et al. (2018a). Research and development in agricultural robotics: a perspective of digital farming. Int. J. Agric. Biol. Eng. 11 (4), 1–11. doi:10.25165/j.ijabe.20181103.4278
Sharma, A., Jain, A., Gupta, P., and Chowdary, V. (2021). Machine learning applications for precision agriculture: a comprehensive review. IEEE Access 9, 4843–4873. doi:10.1109/access.2020.3048415
Sharma, R., Kamble, S. S., Gunasekaran, A., Kumar, V., and Kumar, A. (2020). A systematic literature review on machine learning applications for sustainable agriculture supply chain performance. Comput. Operations Res. 119, 104926. doi:10.1016/j.cor.2020.104926
Shrestha, L., Kulig, B., Moscetti, R., Massantini, R., Pawelzik, E., Hensel, O., et al. (2020). Comparison between hyperspectral imaging and chemical analysis of polyphenol oxidase activity on fresh-cut apple slices. J. Spectrosc. 2020, 1–10. doi:10.1155/2020/7012525
Singh, R. K., Aernouts, M., De Meyer, M., Weyn, M., and Berkvens, R. (2020). Leveraging LoRaWAN technology for precision agriculture in greenhouses. Sensors 20 (7), 1827. doi:10.3390/s20071827
Skobelev, P., Tabachinskiy, A., Simonova, E., Lee, T. R., Zhilyaev, A., and Laryukhin, V. (2021) “Digital twin of rice as a decision-making service for precise farming, based on environmental datasets from the fields,” in Proceedings of ITNT 2021 - 7th IEEE international conference on information technology and nanotechnology, 3–4.
Sparrow, R., and Howard, M. (2021). Robots in agriculture: prospects, impacts, ethics, and policy. Precis. Agric. 22 (3), 818–833. doi:10.1007/s11119-020-09757-9
Steinwand, M. A., and Ronald, P. C. (2020). Crop biotechnology and the future of food. Nat. Food 1 (5), 273–283. doi:10.1038/s43016-020-0072-3
Sturm, B., Raut, S., Kulig, B., Münsterer, J., Kammhuber, K., Hensel, O., et al. (2020). In-process investigation of the dynamics in drying behavior and quality development of hops using visual and environmental sensors combined with chemometrics. Comput. Electron. Agric. 175, 105547. doi:10.1016/j.compag.2020.105547
Su, H. (2020). “Internet of Things (IoT)-based collaborative control of a redundant manipulator for teleoperated minimally invasive surgeries,” in 2020 IEEE international conference on robotics and automation (ICRA), 9737–9742.
Sultan, M., Ashraf, H., Miyazaki, T., Shamshiri, R. R., and Hameed, I. A. (2021) “Temperature and humidity control for the next generation greenhouses: overview of desiccant and evaporative cooling systems,” in Next-generation greenhouses for food security.
Tang, Y., Dananjayan, S., Hou, C., Guo, Q., Luo, S., and He, Y. (2021). A survey on the 5G network and its impact on agriculture: challenges and opportunities. Comput. Electron. Agric. 180, 105895. doi:10.1016/j.compag.2020.105895
Tee, Y. K., Balasundram, S. K., Shamshiri, R. R., Shariff, A. R. M., and Ding, P. (2023). Yield potential of site-specific integrated pest and soil nutrient management at different harvest intervals under two commercial cocoa planting systems in Malaysia. Precis. Agric. 24 (3), 1132–1153. doi:10.1007/s11119-023-10003-1
Tekinerdogan, B., and Verdouw, C. (2020). Systems architecture design pattern catalog for developing digital twins. Sensors Switz. 20 (18), 5103–5120. doi:10.3390/s20185103
Tian, H., Wang, T., Liu, Y., Qiao, X., and Li, Y. (2020). Computer vision technology in agricultural automation —a review. Inf. Process. Agric. 7 (1), 1–19. doi:10.1016/j.inpa.2019.09.006
Tiozzo Fasiolo, D., Scalera, L., Maset, E., and Gasparetto, A. (2022). “Recent trends in mobile robotics for 3D mapping in agriculture,” in International conference on robotics in alpe-adria danube region, 428–435.
Torres-Sánchez, J., de Castro, A. I., Peña, J. M., Jiménez-Brenes, F. M., Arquero, O., Lovera, M., et al. (2018). Mapping the 3D structure of almond trees using UAV acquired photogrammetric point clouds and object-based image analysis. Biosyst. Eng. 176, 172–184. doi:10.1016/j.biosystemseng.2018.10.018
Vachálek, J., Bartalský, L., Rovný, O., Šišmišová, D., Morháč, M., and Lokšík, M. (2017). “The digital twin of an industrial production line within the industry 4.0 concept,” in 2017 21st international conference on process control (PC), 258–262.
Valecce, G., Strazzella, S., and Grieco, L. A. (2019). On the interplay between 5G, mobile edge computing and robotics in smart agriculture scenarios BT - ad-hoc, mobile. Wirel. Netw., 549–559.
van Hilten, M., and Wolfert, S. (2022). 5G in agri-food - a review on current status, opportunities and challenges. Comput. Electron. Agric. 201, 107291. doi:10.1016/j.compag.2022.107291
Vergara-Díaz, O., Zaman-Allah, M. A., Masuka, B., Hornero, A., Zarco-Tejada, P., Prasanna, B. M., et al. (2016). A novel remote sensing approach for prediction of maize yield under different conditions of nitrogen fertilization. Front. Plant Sci. 7, 666. doi:10.3389/fpls.2016.00666
Vermesan, O., Bahr, R., Ottella, M., Serrano, M., Karlsen, T., Wahlstrøm, T., et al. (2020). Internet of robotic Things intelligent connectivity and platforms. Front. Robotics AI 7, 104. doi:10.3389/frobt.2020.00104
Verne, G. B. (2020). “Adapting to a robot: adapting gardening and the garden to fit a robot lawn mower,” in Companion of the 2020 ACM/IEEE international conference on human-robot interaction, 34–42.
von Gersdorff, G. J. E., Kulig, B., Hensel, O., and Sturm, B. (2021). Method comparison between real-time spectral and laboratory based measurements of moisture content and CIELAB color pattern during dehydration of beef slices. J. Food Eng. 294, 110419. doi:10.1016/j.jfoodeng.2020.110419
Wallace, L., Lucieer, A., Watson, C., and Turner, D. (2012). Development of a UAV-LiDAR system with application to forest inventory. Remote Sens. 4 (6), 1519–1543. doi:10.3390/rs4061519
Wang, T., Chen, B., Zhang, Z., Li, H., and Zhang, M. (2022). Applications of machine vision in agricultural robot navigation: a review. Comput. Electron. Agric. 198, 107085. doi:10.1016/j.compag.2022.107085
Weltzien, C., and Shamshiri, R. R. (2019). SunBot: autonomous nursing assistant for emission-free berry production, general concepts and framework. LAND.TECHNIK AgEng, 463–470. doi:10.51202/9783181023617-463
Whitehead, K., Hugenholtz, C. H., Myshak, S., Brown, O., LeClair, A., Tamminga, A., et al. (2014). Remote sensing of the environment with small unmanned aircraft systems (UASs), part 2: scientific and commercial applications. J. Unmanned Veh. Syst. 02 (03), 86–102. doi:10.1139/juvs-2014-0007
Xu, R., and Li, C. (2022). A review of high-throughput field phenotyping systems: focusing on ground robots. Plant Phenomics 2022, 9760269. doi:10.34133/2022/9760269
Xue, C., Feng, Y., Bai, F., and Liu, T. (2022). A drip irrigation remote control system using 5G-IoT technology BT - broadband communications, networks, and. Systems, 182–192.
Yamasaki, Y., Morie, M., and Noguchi, N. (2022). Development of a high-accuracy autonomous sensing system for a field scouting robot. Comput. Electron. Agric. 193, 106630. doi:10.1016/j.compag.2021.106630
Yamin, M., bin Wan Ismail, W. I., Abd Aziz, S., bin Mohd Kassim, M. S., Akbar, F. N., and Ibrahim, M. (2022). Design considerations of variable rate liquid fertilizer applicator for mature oil palm trees. Precis. Agric. 23 (4), 1413–1448. doi:10.1007/s11119-022-09892-5
Yamin, M., Wan Ismail, W. I., Mohd Kassim, M. S, Abd Aziz, S., Shamshiri, R., Akbar, F., et al. (2020a). Development and calibration of or sensor for the estimation of macronutrients in the soil of oil palm plantation. Pak. J. Agric. Sci. 57, 1363–1369. doi:10.21162/PAKJAS/20.9946
Yamin, M., Ishak bin Wan Ismail, W., Saufi bin Mohd Kassim, M., Binti Abd Aziz, S., Naz Akbar, F., R. Shamshiri, R., et al. (2020b). Modification of colorimetric method based digital soil test kit for determination of macronutrients in oil palm plantation. Int. J. Agric. Biol. Eng. 13 (4), 188–197. doi:10.25165/j.ijabe.20201304.5694
Yao, L., van de Zedde, R., and Kowalchuk, G. (2021). Recent developments and potential of robotics in plant eco-phenotyping. Emerg. Top. Life Sci. 5 (2), 289–300. doi:10.1042/etls20200275
Yu, L., Ren, Y., Tao, S., Gao, W., Song, X., Zhang, X., et al. (2021). “Eco-climate intelligent monitoring system of an agricultural science-and-technology park based on internet of Things,” in 2021 IEEE international conference on artificial intelligence and computer applications (ICAICA), Dalian, China, 708–715. doi:10.1109/ICAICA52286.2021.9498218
Yu, P., Huang, M., Zhang, M., Zhu, Q., and Qin, J. (2020). Rapid detection of moisture content and shrinkage ratio of dried carrot slices by using a multispectral imaging system. Infrared Phys. Technol. 108, 103361. doi:10.1016/j.infrared.2020.103361
Zhang, Q., Karkee, M., and Tabb, A. (2019). “The use of agricultural robots in orchard management,” in Robotics and automation for improving agriculture, burleigh dodds science publishing, 187–214.
Zhang, X., Ren, Y., Yin, Z. Y., Lin, Z., and Zheng, D. (2009). Spatial and temporal variation patterns of reference evapotranspiration across the Qinghai-Tibetan Plateau during 1971-2004. J. Geophys. Res. Atmos. 114 (15). doi:10.1029/2009jd011753
Zhang, Y., Staab, E. S., Slaughter, D. C., Giles, D. K., and Downey, D. (2012). Automated weed control in organic row crops using hyperspectral species identification and thermal micro-dosing. Crop Prot. 41, 96–105. doi:10.1016/j.cropro.2012.05.007
Keywords: digital agriculture, artificial intelligence, robotics, digital twins, internet of things, wireless, 5G, block chain
Citation: Shamshiri RR, Sturm B, Weltzien C, Fulton J, Khosla R, Schirrmann M, Raut S, Basavegowda DH, Yamin M and Hameed IA (2024) Digitalization of agriculture for sustainable crop production: a use-case review. Front. Environ. Sci. 12:1375193. doi: 10.3389/fenvs.2024.1375193
Received: 23 January 2024; Accepted: 02 July 2024;
Published: 25 July 2024.
Edited by:
Sushant K Singh, CAIES Foundation, IndiaReviewed by:
Adnan Abbas, Nanjing University of Information Science and Technology, ChinaCopyright © 2024 Shamshiri, Sturm, Weltzien, Fulton, Khosla, Schirrmann, Raut, Basavegowda, Yamin and Hameed. This is an open-access article distributed under the terms of the Creative Commons Attribution License (CC BY). The use, distribution or reproduction in other forums is permitted, provided the original author(s) and the copyright owner(s) are credited and that the original publication in this journal is cited, in accordance with accepted academic practice. No use, distribution or reproduction is permitted which does not comply with these terms.
*Correspondence: Redmond R. Shamshiri, cnNoYW1zaGlyaUBhdGItcG90c2RhbS5kZQ==
Disclaimer: All claims expressed in this article are solely those of the authors and do not necessarily represent those of their affiliated organizations, or those of the publisher, the editors and the reviewers. Any product that may be evaluated in this article or claim that may be made by its manufacturer is not guaranteed or endorsed by the publisher.
Research integrity at Frontiers
Learn more about the work of our research integrity team to safeguard the quality of each article we publish.