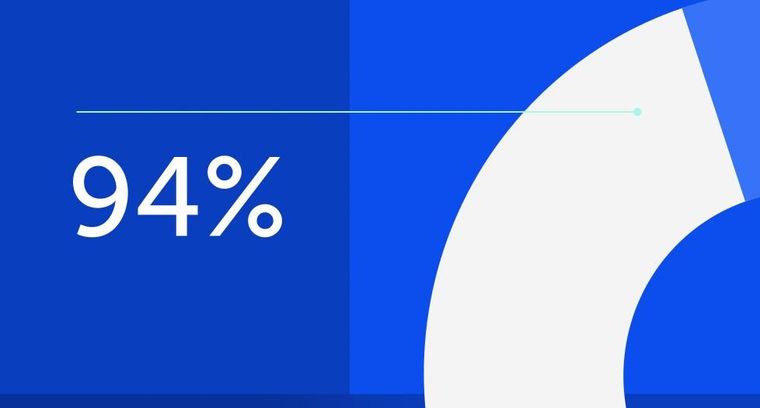
94% of researchers rate our articles as excellent or good
Learn more about the work of our research integrity team to safeguard the quality of each article we publish.
Find out more
ORIGINAL RESEARCH article
Front. Environ. Sci., 29 February 2024
Sec. Environmental Economics and Management
Volume 12 - 2024 | https://doi.org/10.3389/fenvs.2024.1355584
This article is part of the Research TopicEnergy-Environment Sustainability: Progresses and Impacts of Clean Energy System ConstructionView all 8 articles
Introduction: This paper presents a spatial effect model to examine the impact of industrial agglomeration and international R&D capital technology spillover on air pollution.
Methods: The study utilizes spatial panel data from 30 provinces and cities in China spanning the period 2008 to 2022, allowing for the decomposition of both direct and indirect spillover effects.
Results: Additionally, the research investigates the threshold effect of industrial agglomeration and international research and development capital and technology spillover on air pollution, considering independent research and development as the threshold variable. The findings reveal evident spatial autocorrelation and spatial dependence between industrial agglomeration, international R&D capital, and air pollution. Importantly, both international R&D capital technology spillover and industrial agglomeration exhibit a single threshold effect. This implies that surpassing the threshold value leads to a more pronounced positive impact of international R&D capital technology spillover on reducing air pollution, while the impact of industrial agglomeration on air pollution tends to be positive.
Discussion: The findings of this study have significant theoretical and practical implications for the promotion of sustainable economic development, particularly in China.
China’s development of a green economy and ecological civilization necessitates not only compliance with global emission reduction obligations but also adherence to the laws of nature to mitigate the adverse impacts of environmental pollution on human health (Cheng and You, 2019). After years of concerted efforts, China has achieved remarkable success in reducing CO2 emissions and currently holds the position of the world’s leading producer and consumer of biofuels. Additionally, in response to climate change, China has set a “double carbon target.” At the 2022 National Conference on Ecological and Environmental Protection, Li Gao, Director of the Department of Climate Change, announced that China will implement resolute new measures to achieve its carbon emission reduction goals for 2030, including strengthening the regulation of non-carbon dioxide greenhouse gas emissions. Urban areas, in particular, are significantly impacted by air pollution resulting from the release of pollutants such as SO2, particulate matter (PM), NOX, and volatile organic compounds (VOCs), which contribute to the frequent occurrence of haze (Zhang et al., 2021).
Technological advancements play a crucial role in mitigating air pollution, and they can be fostered through two primary channels: domestic research and development (R&D) efforts and international collaboration. Domestic R&D programs focus on exploring innovative solutions and developing cutting-edge technologies tailored to local environmental challenges. These initiatives involve government funding, academic institutions, and industry partnerships to drive scientific breakthroughs and practical applications. Simultaneously, international collaboration facilitates knowledge exchange, resource sharing, and joint efforts to tackle transboundary air pollution issues. By leveraging both domestic and international resources, countries can accelerate the development and deployment of effective technologies for combating air pollution on a global scale.
The research motivation and novelty of this paper are threefold. Firstly, it categorizes international R&D collaborations into two main types: horizontal collaborations and vertical collaborations. Horizontal collaborations involve partnerships between firms or organizations within the same industry or sector, aiming to share knowledge and resources to enhance technological advancements collectively. On the other hand, vertical collaborations refer to collaborations between firms or organizations across different stages of the value chain, facilitating the transfer of innovation and expertise along the production process; Secondly, this study investigates the determinants of international R&D collaborations, particularly focusing on economic factors such as market size, research capabilities, and intellectual property rights protection. By exploring these factors, the paper aims to provide insights into the drivers of successful international R&D collaborations and contribute to the existing literature on innovation and technology transfer; Lastly, this research examines the impact of international R&D collaborations on firm performance and competitiveness. It analyzes how collaboration affects various aspects of firm performance, including productivity, profitability, market share, and innovation outcomes. With this analysis, the study seeks to shed light on the potential benefits and challenges associated with international R&D collaborations and their implications for firms’ strategic decision-making and long-term sustainability.
Since the mid-20th century, environmental issues have grown increasingly severe, exerting a gradual influence on human life. Consequently, scholars worldwide have undertaken extensive research to analyze the various factors that can potentially impact the environment. Specifically, some scholars have concentrated on the effects of artificial intelligence (AI) on the environment (Wang et al., 2020; Xuan and Zhang, 2021; Lv et al., 2023; Wang et al., 2023), while others have examined the relationship between financial development and environmental pollution (Zheng et al., 2021; Yu and Fan, 2022; Wang et al., 2024). Additionally, certain researchers have explored the impact of environmental pollution from a geopolitical standpoint (Graham et al., 2022; Wang et al., 2023; Zhang and Yu, 2023), or investigated the effects of information and communication technology (ICT) on environmental pollution (Liu et al., 2018; Liu and Zhu, 2021; Wang et al., 2023). Moreover, there have been studies focusing on the links between corruption governance and environmental pollution (Zhang, 2016; Pei, 2022; Chen et al., 2023; Wang et al., 2023). The aim of this paper is to examine the impact of industrial agglomeration and international R&D cooperation on the environment, providing valuable insights into the complex relationship between these factors.
In the realm of contemporary research, both domestic and international scholars have advanced the study of industrial agglomeration and its correlate, environmental pollution. Despite these advancements, the discourse has yet to coalesce around a universal consensus, and debate persists, predominantly encapsulated within three primary schools of thought: ① there is the argument that industrial agglomeration mitigates pollution. Empirical analyses by Li et al. (2017) leveraging panel data vector autoregressive models illuminated a dynamic relationship between industrial agglomeration, technological innovation, and environmental pollution—evidencing that amplified degrees of industrial agglomeration appreciably curtail industrial pollution emissions and foster technological innovation. Xu and Zhang (2018) also substantiated that industrial agglomeration markedly enhances air quality, a robust finding when accounting for the heterogeneity of air quality assessments and upon the exclusion of cities with egregiously high pollution levels. Similarly, Han and Zhang (2023) and Fan and Xu (2023) respectively determined that industrial specialization agglomeration significantly lessens pollution intensity within industrial units, and that utilizing high-speed rail services to intensify factor agglomeration leads to improvements in green production efficiency within industrial clusters, thereby contributing to regional ecological advancement; ② an antithetical perspective posits that industrial agglomeration exacerbates environmental pollution. Ji and Zhu (2019), examining pollution emissions as an intermediary variable in the context of industrial agglomeration and resource misallocation, ascertained that while pollution emissions do not uniformly respond to differing degrees of agglomeration contingent upon misallocation conditions, an overall trend suggests an escalation in emissions correlating with increasing agglomeration. Likewise, He (2023) identified a single threshold effect of industrial agglomeration upon environmental efficiency within the Chengdu-Chongqing Economic Circle, revealing that initial agglomeration inhibits environmental efficiency enhancement, which may, however, be mitigated by technological innovation. This finding is congruent with Wang and Nie (2016), who noted that industrial agglomeration has the potential to obstruct environmental governance efforts in the short term, manifesting as spatially ‘concentrated emissions’ from polluting enterprises; ③ a faction of researchers emphasize the inherent uncertainty in the relationship between industrial agglomeration and environmental pollution. The work of Xu and Li (2023) discovered a pronounced inverted “U” relationship between dairy farming industry agglomeration and environmental efficiency, attributing initial efficiency improvements to a “scale effect” until a critical agglomeration turning point is reached, beyond which a “crowding effect” supersedes and suppresses environmental efficiency. He et al. (2022) posited an analogous inverted “U” relationship between the level of industrial agglomeration and pollution, suggesting most Chinese provinces currently reside on the positive externality side of the curve. Liu et al. (2018) identified a nonlinear spatial interrelation between industrial agglomeration and environmental pollution across varied Chinese regions. Kou (2021) and Sun et al. (2021) further nuanced the discourse by analyzing different types of industrial agglomerations and their disparate impacts on environmental pollution, with the latter uncovering an inverted “N” relationship where extreme agglomeration levels inversely affect environmental performance.
The relationship between international R&D capital technology spillovers and environmental pollution has been extensively studied. Gao (2016) conducted a comprehensive analysis using a system GMM model to examine the impact of global R&D investment on carbon emissions in China. The findings revealed that technological spillover resulting from foreign direct investment and import transactions effectively increased China’s carbon emissions. Similarly, Huang et al. (2018) utilized a CH-LP model to explore the linear and nonlinear effects of domestic R&D, foreign direct investment, and trade on carbon intensity. The study uncovered that the dissemination of domestic R&D and imported trade technologies contributed to increased carbon intensity, whereas foreign direct investment and export trade effectively reduced carbon intensity in China. In another investigation, Wang et al. (2023) employed a nonlinear panel threshold regression model and identified that when foreign direct investment exceeded a certain threshold, its impact on promoting carbon emissions initially increased, but then decreased. Furthermore, Gao and Nie (2019) highlighted that foreign direct investment and export trade not only fostered the enhancement of carbon productivity within a particular region but also indirectly improved carbon productivity in other regions. The introduction of foreign technology contracts had a direct effect on significantly augmenting carbon productivity in the region but resulted in a decline in carbon productivity in other regions. Examining the effects of technology spillovers on CO2 emissions, Jiao et al. (2018) incorporated variables such as direct and indirect technology spillover effects into an econometric model. The results indicated that the diffusion of foreign direct investment technology contributed to a reduction in CO2 emissions. In contrast, Wang and Zhu (2018) found that outward foreign direct investment substantially increased sulfur dioxide emissions in various provinces in China. Moreover, Ding and Zhang (2021) investigated the mediating effect of import trade technology spillover on the intensity of industrial atmospheric pollution. The study uncovered a “positive N-shaped” relationship, suggesting that both high and low levels of technology spillover can lead to environmental pollution. Lastly, Wang et al. (2023) explored the impact of trade on carbon emissions and discovered that the effect varied depending on the nature of trade. Trade openness was found to contribute to an increase in carbon emissions, whereas trade diversification was associated with a decrease in carbon emissions. Import diversification was identified as having the most substantial impact on reducing carbon emissions.
The extant body of literature has devoted substantial attention to examining the nexus between industrial agglomeration and air pollution, as well as the consequences of international R&D capital technological spillovers on carbon dioxide emissions. Despite these scholarly endeavors, there persists a divergence of conclusions within the research community. The existing scholarship manifests certain lacunae that warrant further scholarly inquiry: Firstly, there is a preponderance of research concentrating on the ramifications of international R&D capital technological spillovers for carbon emissions or carbon efficiency, while comparatively scant attention is paid to the multifaceted impact of such spillovers on sulfur dioxide emissions. Secondly, the spatial spillover effects of industrial agglomeration on environmental pollution, and their potential nonlinear interdependencies, require closer scrutiny. The compendium of literature focusing on the interrelation between industrial agglomeration and international R&D capital technology transfer remains incomplete.
To redress these deficiencies in the literature, the present study seeks to empirically explore the effects of industrial agglomeration and international R&D capital technological spillovers on air pollution. For this purpose, a spatial econometric model will be developed to ascertain both the direct and indirect influences exerted by these factors. An empirical investigation deploying spatial panel data, collected from 30 provinces and municipalities in China over the interval encompassing 2008 to 2022, will be undertaken. This research will disentangle the convoluted spillover effects, and it will probe into the potential threshold effects of industrial agglomeration and international R&D capital technological spillovers on air pollution quality, deploying independent R&D as a pivotal threshold variable. It is envisaged that the insights gleaned from this analysis will not only contribute to a more sophisticated theoretical framework surrounding industrial agglomeration but also furnish empirical evidence that can inform the formulation of policy interventions geared towards scientifically engineering a more congenial living environment.
Currently, the principal constituents of air pollution emissions in China are carbon dioxide (CO2) and sulfur dioxide (SO2). The focus of this study is on SO2 emissions for several reasons. Firstly, there is a lack of comprehensive statistical data on CO2 emissions, as well as a standardized calculation approach for assessing CO2 emissions. Additionally, the author’s prior research has extensively addressed CO2; thus, this study endeavors to substantiate the relevant theoretical framework by concentrating on SO2 emissions. Secondly, given that China is the world’s leading emitter of SO2, the data available from the National Bureau of Statistics is not only more granular but also holds a greater degree of veracity. Consequently, this study adopts the index of SO2 emissions intensity as a proxy for air pollution, operationalized as the ratio of total provincial and municipal SO2 emissions to the Gross Domestic Product (GDP)—denoted as pollution emission. The requisite data was procured from the official website of the National Bureau of Statistics of China.
The stock of foreign R&D capital obtained by Chinese provinces and cities through three spillover channels, namely import trade, export trade, and foreign direct investment, was measured by referring to Eqs 1-3 Lichtenberg et al.’s formulas, as follows:
where
Based on the availability of the data, the time range studied in this paper was from 2008 to 2022. The capital stock measurement method is used to calculate
There are many different criteria for measuring industrial agglomeration, such as the GINI coefficient, Hoover index, E-G index, and location entropy, each of which has its advantages and disadvantages. Here, we will draw lessons from the practices of Zhong and Wei (2019), and measure the industrial agglomeration level (IA) in all provinces and cities by location entropy. This paper uses industrial added value to measure the
The index of domestic independent R&D expenditure adopts the ratio of R&D internal expenditure to GDP, that is, the intensity of R&D investment. The more R&D investment, local enterprises can have a higher level of technology and use more advanced technology and equipment, to improve the efficiency of water resources utilization and reduce pollution emissions (Wang and Fan, 2021). R&D internal expenditure data are obtained from China Science and Technology Statistical Yearbook.
The choice of per capita GDP as the control variable can reflect the development degree of a region’s economy, and the level of economic development is an important variable affecting environmental pollution. To eliminate the price factors, the GDP index was used to reduce the per capita GDP in a period based on 2010. The total population adopts the resident population of each province at the end of the year. Per capita GDP is the total GDP of each province (city) divided by the resident population of each province (city) at the end of the year. The data on the total population and GDP are obtained from the website of the Chinese Bureau of Statistics.
The applicability of the spatial measurement model can be determined by using the spatial correlation test (Eq. 4). The “Moran index I” (Moran’sI) is the most common means of spatial correlation test in the current spatial measurement model. The formula is:
Where i = 1,2,…, n, j = 1,2,…, n;
Without considering spatial effects, traditional regression models may produce biased or even incorrect results (Eq. 5). Therefore, this paper uses a spatial panel econometric model based on the Cobb-Douglas production function (Douglas C. North, 1994; Coukmoe, 2000) to investigate spatial effects.
where
① If λ = 0, it is the Space Dubin Model (Spatial Dubin Model, SDM).
② If λ = 0 and θ = 0, it is the “spatial autoregressive model” (Spatial Autoregressive Model, SAR).
③ If ρ = 0 and θ = 0, then the Spatial Error Model (Spatial Error Model and SEM). The spatial Dubin model, the spatial autoregressive model, and the spatial error model are all set at τ = 0.
Although least squares estimation leads to inconsistency in the estimation of regression parameters, spatial parameters, and standard errors of models with spatial lag-dependent variables, the likelihood estimation is consistent (LEE et al., 2004), so the spatial panel econometric model is estimated by the likelihood method.
In selecting spatial weights, select the 0–1 spatial weight matrix, which is assigned according to the spatially geographic proximity, with geographically close regions assigned a value of “1” and other regions a value of “0.”
This paper draws on the method of Eq. 6 quoted in Hansen (1999) to construct the threshold measurement model of air pollution and examines the threshold effect of industrial agglomeration and international R&D capital technology overflow on air pollution when independent research and development is taken as the threshold variable. The threshold model is as follows:
where
Descriptive statistics for each variable are listed in Table 1.
To ascertain the appropriateness of incorporating a spatial effect into the analysis, this paper implements the Global Moran’s I index to assess the spatial autocorrelation of variables including international research and development (R&D) capital, sulfur dioxide (SO2) emission intensity, and the magnitude of industrial agglomeration. The outcomes, as delineated in Table 2, indicate that a majority of the examined variables, such as international R&D capital, SO2 emission intensity, and industrial agglomeration, achieve statistical significance, evidencing pronounced spatial dependence. Consequently, the adoption of a spatial panel econometric model is warranted to investigate the impact of international R&D capital and industrial agglomeration on air pollution meticulously.
TABLE 2. Moran’s I index values for the key variables for all provinces and cities of China in the period 2008–2022.
A local spatial autocorrelation test was performed on the SO2 emission intensity of 30 provinces and cities in China. Moran’s I scatter plot of sulfur dioxide emission intensity in 30 provinces in China (2022) as shown in Figure 1, most of the areas are located in the first and third quadrants, indicating that most areas of the sulfur dioxide intensity show similar spatial agglomeration characteristics with adjacent areas.
FIGURE 1. Moran’s I scatter plot of sulfur dioxide intensity for 30 provinces and cities of China in 2022.
At this juncture, it became essential to identify an appropriate spatial econometric model for the ensuing analysis. In pursuit of this objective, a battery of diagnostic tests was administered, comprising the Moran’s I test, LM (Robust) test, the Wald test, the likelihood ratio (LR) test, and the Hausman test. The Moran’s I test for spatial autocorrelation revealed significant values at the 5% significance threshold. Likewise, the LM (Robust) tests for both spatial error and spatial lag decisively rejected the null hypothesis, thereby substantiating the necessity for spatial econometric scrutiny. Subsequently, the Wald and LR tests were deployed to discern whether the Spatial Durbin Model (SDM) was reducible to a Spatial Autoregressive (SAR) or Spatial Error Model (SEM). The results of both tests unequivocally endorsed the application of the SDM model. The Hausman test outcomes indicated a proclivity towards a fixed-effects model. Lastly, through the application of the LR test, a differential determination was made between a time-fixed, area-fixed, and a two-way fixed effects model; the empirical evidence dictated the selection of a two-way fixed effects model. Thus, the elucidated analyses culminated in the adoption of the two-way fixed effects SDM model, the results of which are presented in Table 3.
The regression coefficients derived from Dubin’s spatial econometric model do not intrinsically encapsulate the complete marginal impacts of the explanatory variables. To elucidate the spatial dependencies, the effects must be fractionated into three distinct categories: indirect, direct, and cumulative effects. Within the spatial framework of Dubin’s model, the comprehensive influence exerted by foreign direct investment (FDI), import trade, export trade, and the degree of industrial agglomeration upon the emission intensity of sulfur dioxide can be dissected into direct and indirect components, as delineated in Table 4. The direct impact encapsulates the repercussions of FDI, import trade, export trade, and industrial agglomeration levels on sulfur dioxide emission intensity directly, within the immediate context. Conversely, the indirect impact, often referred to as the ‘spatial spillover effect,’ conveys the extent to which FDI, import trade, export trade, and industrial agglomeration influence sulfur dioxide emission intensity in an adjacent or co-located spatial context. The cumulative effect, derived from the aggregation of direct and indirect impacts, represents the total imputed effect of the variables under study on the emission intensity of sulfur dioxide.
TABLE 4. Decomposition of the total effect in the Spatial Durbin model into direct and indirect effects.
The decomposition of the spatial effect of industrial agglomeration and international R&D capital and technology spillover on air pollution reveals the following findings: ① the direct effect of foreign direct investment (FDI) technology spillover is negative, indicating that FDI can lead to a reduction in regional sulfur dioxide emission intensity through the demonstration effect and competition effect of technology spillover. Conversely, the indirect effect is significantly positive, suggesting that FDI increases air pollution in adjacent areas. This can be attributed to local FDI activities driving neighboring regions to actively seek FDI, leading to the rapid development of related industries and consequently, worsening environmental pollution. ② the direct, indirect, and total effects of technology spillover from import trade (IM) are all significantly negative. This implies that imported foreign advanced machinery, equipment, and intermediate goods facilitate the absorption and assimilation of advanced technology, resulting in a considerable reduction in sulfur dioxide emission intensity both locally and in neighboring areas. Import trade not only fosters the improvement of local advanced technology and advanced management practices but also influences the spatial spillover of technology to adjacent areas, thereby enhancing their advanced technology and management practices, thereby improving the overall environmental conditions. ③ The analysis of export trade (EX) reveals that while the direct technology spillover effect is negative, it does not achieve statistical significance. Contrastingly, the indirect effects are both positive and significant; however, the aggregate effect is positive but lacks statistical significance. Through the lens of direct effects, the analysis indicates that the export trade may exert a diminishing influence on air pollution to some degree, attributable to the competition effect, economies of scale, and the learning effects associated with export activities. From the standpoint of indirect effects, the phenomenon of developed eastern regions adhering to a green export trade paradigm, which involves the relocation of highly polluting industries to the less developed Midwest, contributes to interprovincial pollution. In a broader context, export trade appears to elevate the intensity of sulfur dioxide emissions in proximate regions. ④With regard to industrial agglomeration (IA), the direct effect on sulfur dioxide emission intensity is quantified at 0.0033, which does not reach statistical significance, suggesting that industrial agglomeration per se does not significantly exacerbate regional air pollution. However, the analysis of the indirect effect unveils a prominent increment (0.0136), surpassing the 1% threshold for significance, thereby implying that industrial agglomeration magnifies the severity of sulfur dioxide emissions in neighboring territories. In consideration of the total effect, which is measured at 0.0169 and passes the significance test, it can be concluded comprehensively that industrial agglomeration accentuates the emission intensity of sulfur dioxide.
Hence, it is posited that the impact of industrial agglomeration on diminishing the spatial spillover effect is more pronounced at the regional level than the interregional internal spillover effect. The primary impetus behind this observed phenomenon may be attributed to the diffusion of economic activity from local industrial clusters to adjacent areas. This diffusion catalyzes subsequent industrial agglomeration activities, culminating in augmented environmental pollution locally.
As shown in Table 5, the influence of industrial agglomeration and international R&D capital technology overflow on air pollution shows non-linear characteristics. With independent research and development (rd) as the threshold variable, the technology spillover effect of international research and development capital relying on foreign direct investment, import trade, and export trade channels is shown as a single threshold. Similarly, the impact of industrial agglomeration on air pollution also shows a threshold.
The threshold model estimates for core explanatory variables and other variables are shown in Table 6. Model (1)–(4) indicates the threshold effect of export trade (EX), import trade (IM), foreign direct investment (FDI), and industrial agglomeration (IA) on air pollution based on independent research and development (rd) threshold variables.
The results of the study indicate the following findings:
(1) When the main variable of interest is export trade (EX), the technology spillover effect from export trade demonstrates a single-threshold pattern, with autonomous R&D serving as the threshold variable. When independent R&D does not exceed the threshold (rd<7.84), there is a positive correlation between technology spillover from export trade and air pollution. Conversely, when the threshold is surpassed (rd≥7.84), the effect of technology spillover from export trade on air pollution becomes negative. This implies that a higher level of independent R&D is required to support the technology spillover effect from export trade, which in turn reduces air pollution.
(2) When the main variable of interest is foreign direct investment (FDI), there is a non-significant negative relationship between FDI technology spillover and air pollution when the intensity of R&D investment capital falls below the threshold (rd<7.84). However, once the threshold is exceeded (rd≥7.84), a significant negative effect of FDI technology spillover on air pollution is observed. When the intensity of R&D investment capital surpasses the threshold, the technology spillover effect of FDI can be better utilized, leading to the adoption of advanced technology for environmental purposes, thereby reducing sulfur dioxide emissions.
(3) When the main variable of interest is import trade (IM), its impact on air pollution aligns with the performance of export trade. In other words, when the threshold is not surpassed (rd<7.84), there exists a positive correlation between import trade technology spillover and air pollution. Conversely, once the threshold is surpassed (rd≥7.84), import trade technology spillover has a negative impact on air pollution. Beyond this threshold, the effect of import trade technology spillover can further motivate enterprises to upgrade their environmentally advanced technology and management practices, thus positively influencing air quality.
(4) When the main independent variable, namely industrial agglomeration (IA), is considered, it exhibits a singular threshold effect on air pollution. In cases where independent research and development (R&D) falls below the threshold value (rd < 7.20), a considerable positive impact of industrial agglomeration on air pollution is observed. Likewise, when independent R&D surpasses the initial threshold (rd ≥ 7.20), a significant positive effect of industrial agglomeration on air pollution persists, with the degree of impact increasing as reflected by the coefficient. These findings indicate that increased levels of independent R&D contribute to a rise in air pollution facilitated by industrial agglomeration. Consequently, heightened investment in R&D within areas characterized by industrial agglomeration leads to escalated resource and energy consumption, resulting in amplified local environmental pollution.
This study utilizes panel data collected from 30 provinces and regions in China spanning the period from 2008 to 2022. By employing a spatial panel model, it examines the intricate relationship between industrial agglomeration, international R&D capital technology spillover, and air pollution. Specifically, it investigates the direct, indirect, and overall effects of industrial agglomeration and international research and development capital on air pollution. Additionally, this study introduces the concept of independent research and development as a threshold variable, analyzing the threshold effect of industrial agglomeration and international research and development capital technology transfer on air pollution. The findings reveal the existence of evident spatial autocorrelation and spatial dependence among industrial agglomeration, international R&D capital, and air pollution. Notably, both international R&D capital technology spillover and industrial agglomeration exhibit a single threshold effect. When the threshold value is surpassed, international R&D capital technology spillover displays a more positive impact in reducing air pollution. Conversely, the impact of industrial agglomeration on air pollution tends to be positive.
Based on the above analysis, to improve the environmental pollution situation, this paper puts forward suggestions from the following aspects:
(1) In order to enhance the composition of import trade and facilitate the inflow of energy-efficient and environmentally friendly machinery, equipment, and intermediate products, it is imperative to adopt a strategy that involves emulating, assimilating, and incorporating advanced environmental protection technologies from developed nations. By assimilating these technologies into domestic production processes, the aim is to leverage the potential emission reduction effects resulting from the technology spillover associated with import trade (Wang et al., 2023);
(2) The government should enhance environmental standards for foreign capital inflow, limiting the establishment of highly polluting foreign-funded enterprises, while prioritizing the attraction of foreign direct investment (FDI) in industries characterized by advanced environmental protection technologies. Simultaneously, efforts should be made to harness the technology spillover effects associated with FDI, encouraging foreign enterprises to engage in technology upgrading and transformation. Domestic enterprises should also be incentivized to learn from the advanced environmental protection technologies and models employed by foreign-funded enterprises. In actively pursuing FDI, it is crucial to devise region-specific policy guidance that takes into account variations in environmental pollution levels and the degree of industrial agglomeration. This approach aims to minimize indirect effects resulting from FDI and achieve the dual objectives of attracting foreign investment and promoting environmental protection;
(3) Local governments at all levels should allocate substantial resources to comprehensively analyze the implications of industrial agglomeration on environmental pollution. With a systematic approach, they should develop sound strategies to optimize the arrangement of industrial agglomerations while implementing stringent environmental regulations to curtail excessive concentration and mitigate pollutant emissions. Moreover, it is imperative for both the government and enterprises to consistently augment their investments in low-carbon research and development (R&D). This can be achieved by formulating effective policies that incentivize R&D activities, enhancing the intensity and innovativeness of low-carbon technology enterprises, and progressively fostering mutually beneficial collaborations with industrial clusters and international R&D capital. These efforts are crucial in diminishing air pollution and establishing a sustainable living environment.
A primary limitation of this study lies in the establishment of panel data. The missing data on certain industries was not accounted for, leading to the exclusion of some data points and resulting in the use of provincial-level data in the analysis. This method restricts the study to a macro-level quantitative representation. Future research with higher data availability could refine and expand the sample by examining municipal or county-level data and incorporating other intermediary variables into the analysis framework. Such an approach could provide a deeper understanding of the internal action mechanisms of the variables under study and offer improved path selection.
The datasets presented in this study can be found in online repositories. The names of the repository/repositories and accession number(s) can be found in the article/supplementary material.
DG: Methodology, Software, Writing–review and editing. YD: Conceptualization, Funding acquisition, Methodology, Project administration, Resources, Writing–original draft, Writing–review and editing. ZC: Data curation, Investigation, Software, Writing–review and editing.
The author(s) declare financial support was received for the research, authorship, and/or publication of this article. This work was supported by the Henan Provincial Philosophy and Social Science Planning Project (2022BJJ109).
The authors declare that the research was conducted in the absence of any commercial or financial relationships that could be construed as a potential conflict of interest.
All claims expressed in this article are solely those of the authors and do not necessarily represent those of their affiliated organizations, or those of the publisher, the editors and the reviewers. Any product that may be evaluated in this article, or claim that may be made by its manufacturer, is not guaranteed or endorsed by the publisher.
Chen, H. Y., Yu, J. H., and Zhang, W. Z. (2023). The spatial agglomeration of China's heavy pollution industries and their environmental effects: characteristics and inspirations. J. Chin. Acad. Sci. 38 (12), 1939–1949. doi:10.16418/j.issn.1000-3045.20231006002
Chen, J., Shan, M., and Tan, J. (2023). How does the outgoing audit of leading cadres' natural resource assets affect the green innovation? Audit. study 2023 (03), 19–32.
Cheng, J. H., and You, Z. (2019). Scientific connotation and practical paths about the principle of taking mountains, rivers, forests, farmlands, lakes, and grasslands as a life community. China Popul. Resour. Environ. 29, 1–6. doi:10.12062/cpre.20181121
Deng, R. R., and Zhang, A. X. (2023). FDI technology Spillover,Absorption capacity and carbon emission intensity:empirical study based on the panel threshold model. Int. Bus. Res. 44, 1–13. doi:10.13680/j.cnki.ibr.2023.02.002
Ding, R. J., and Zhang, S. Y. (2021). Import trade, environmental effects of technology spillovers and industrial air pollution—analysis of mediating path based on provincial panel data. J. Beijing Inst. Technol. 23, 22–37. doi:10.15918/j.jbitss1009-3370.2021.4537
Fan, X., and Xu, Y. (2023). Opening of high-speed rail, industrial agglomeration and industrial pollution. J. Nanjing Univ. Finance Econ. 5, 24–35.
Fang, X., Tian, S., and Wang, X. (2019). Financial decentralization, industrial agglomeration and green economic efficiency — empirical analysis based on the panel data of 270 cities at the prefecture-level and above. Explor. Econ. Issues 2019 (11), 164–172.
Gao, D. (2016). Research on the impact of international RD capital and financial development on carbon productivity. Econ. Surv. 33, 150–154. doi:10.15931/j.cnki.1006-1096.2016.01.026
Gao, D., and Nie, H. (2019). Research on the impact of international R&D capital technology spillover on carbon productivity from the perspective of spatial effect. Ecol. Econ. 35 (6), 26–32.
Graham, M., Cao, H., and Guo, Y. (2022). Deadly zones: pandemic, geopolitical, and environmental crises. University of Journalism, 90–99.
Han, Q., and Zhang, X. (2023). Does industrial specialization agglomeration aggravate environmental pollution? empirical study based on industrial industry panel data. Shanghai Econ. Res. 6, 84–94. doi:10.19626/j.cnki.cn31-1163/f.2023.06.001
Hansen, B. E. (1999). Threshold effects in non-dynamic panels: estimation, testing, and inference. J. Econom. 93 (2), 345–368. doi:10.1016/S0304-4076(99)00025-1
He, S., and Lia, R. (2023). Study on the influence of industrial agglomeration on environmental efficiency and spatial difference in Chengdu-Chongqing Shuangcheng economic circle. Ecol. Econ. 39 (11), 87–93+127.
He, Z., Cao, C., and Wang, J. (2022). Research on environmental regulation, industrial agglomeration and environmental pollution. East China Econ. Manag. 36 (3), 12–23. doi:10.19629/j.cnki.34-1014/f.211031008
Huang, J., Liu, Q., Cai, X., Hao, Y., and Lei, H. (2018). The effect of technological factors on China's carbon intensity: new evidence from a panel threshold model. Energy Policy 115 (4), 32–42. doi:10.1016/j.enpol.2017.12.008
Ji, S., and Zhu, Y. (2019). Research on industrial agglomeration, environmental pollution and resource misallocation. Economist 6, 33–43. doi:10.16158/j.cnki.51-1312/f.2019.06.004
Jiang, B., and Raza, M. Y. (2023). Research on China's renewable energy policies under the dual carbon goals: a political discourse analysis. Energy Strategy Rev. 48, 101118. doi:10.1016/j.esr.2023.101118
Jiang, X. H., and Raza, M. Y. (2022). Delving into Pakistan's industrial economy and carbon mitigation: an effort toward sustainable development goals. Energy Strategy Revi 41, 100839. doi:10.1016/j.esr.2022.100839
Jiao, J., Jiang, G., and Yang, R. (2018). Impact of R&D technology spillovers on carbon emissions between China’s regions. Struct. Chang. Econ. Dyn. 47, 35–45. doi:10.1016/j.strueco.2018.07.002
Kou, D. (2021). Research on the relationship between industrial agglomeration mode and environmental pollution. Econ. Longit. 38 (4), 73–82. doi:10.15931/j.cnki.1006-1096.2021.04.004
Li, F., Sun, X. D., and Zhang, L. L. (2017). Industrial agglomeration, technological innovation and environmental pollution — empirical analysis of panel data based on China's industrial industry. Technol. Econ. 36 (3), 1–7. doi:10.3969/j.issn.1002-980X.2017.03.001
Li, R., Wang, Q., Li, L., and Hu, S. (2023). Do natural resource rent and corruption governance reshape the environmental Kuznets curve for ecological footprint? Evidence from 158 countries. Resour. Policy 85, 103890. doi:10.1016/j.resourpol.2023.103890
Lichtenberg, F. R., and De La Potterie, B. V. P. (1998). International R&D spillovers: a comment. Eur. Econ. Rev. 42 (8), 1483–1491. doi:10.1016/S0014-2921(97)00089-5
Liu, J. (2016). The dual role of information communication technology (ICT) in environmental protection — case comparison of US environmental movement (English). Int. press 38 (03), 151–172. doi:10.13495/j.cnki.cjjc.2016.03.010
Liu, X., and Zhu, F. (2021). Research on digital management mode of intelligent chemical Industry Park based on ICT technology — take Quzhou Green Industry Cluster area as an example. Surv. spatial Geogr. Inf. 44 (06), 132–134.
Liu, Y., Yuan, H., and Feng, Y. (2018). Spatial spillover and doorframe characteristics of the emission reduction effect of industrial agglomeration. Math. Statistics Manag. 2, 224–234.
Liu, Z. M., Xie, W. J., and Liu, H. J. (2023). Research on the impact of technological innovation and industrial agglomeration on energy ecological efficiency. Price Theory Pract. 5, 184–187+211. doi:10.19851/j.cnki.CN11-1010/F.2023.05.282
Lv, Y., Ma, M., and Chen, Y. (2023). Artificial intelligence enables green development. China's Population. Resour. Environ. 33 (10), 100–111.
Ma, Y. (2023). A study on the estimation of the desirable range of cross-border capital flows in emerging economies. Beijing.
Pei, J. (2022). Analysis of the utilization of natural resources and ecological and environmental protection. Resour. conservation Environ. Prot. 2022 (01), 34–37. doi:10.16317/j.cnki.12-1377/x.2022.01.010
Raza, M. Y., and Dongsheng, L. I. (2023). Analysis of energy-related CO2 emissions in Pakistan: carbon source and carbon damage decomposition analysis. Environ. Sci. Pollut. Res. 30 (49), 107598–107610. doi:10.1007/s11356-023-29824-8
Sun, Y., Zhang, P., and Chen, S. (2021). Research on the relationship between industrial agglomeration and environmental pollution control — analysis of the regulatory role based on environmental regulation and technological innovation. Price Theory Pract. 4, 161–164+171. doi:10.19851/j.cnki.cn11-1010/f.2021.04.223
Sun, Z. C., Wang, T., Guo, H. W., and Li, X. B. (2023). Technological innovation, industrial agglomeration, and economic development. Econ. Issues 7, 77–86. doi:10.16011/j.cnki.jjwt.2023.07.001
Verhoef, E. T., and Nijkamp, P. (2002). Externalities in urban sustainability: environmental versus localization-type agglomeration externalities in a general spatial equilibrium model of a single-sector monocentric industrial city. Ecol. Econ. 40 (2), 157–179. doi:10.1016/S0921-8009(01)00253-1
Wang, B., and Fan, Z. (2021). Study on the impact of high-tech industrial agglomeration on green water resources efficiency-Based on the spatial Durbin model. Resour. Ind. 1-13.
Wang, B., and Nie, X. (2016). Industrial agglomeration and environmental governance: power or resistance — evidence from the establishment of quasi-natural experiments in the development zone. Industrial Econ. China 12, 75–89. doi:10.19581/j.cnki.ciejournal.2016.12.006
Wang, H., and He, X. Y. (2022). Industrial agglomeration, environmental regulation, and green innovation efficiency. Statistics Decis. Mak. 38 (22), 184–188. doi:10.13546/j.cnki.tjyjc.2022.22.035
Wang, Q., Ge, Y., and Li, R. (2023). Does improving economic efficiency reduce ecological footprint? The role of financial development, renewable energy, and industrialization. Energy & Environ. 0 (0). doi:10.1177/0958305X231183914
Wang, Q., Hu, S., and Li, R. (2023). “Could information and communication technology (ICT) reduce carbon emissions?,” in The role of trade openness and financial development (Telecommunications Policy), 102699. doi:10.1016/j.telpol.2023.102699
Wang, Q., Ren, F., and Li, R. (2024). Exploring the impact of geopolitics on the environmental Kuznets curve research. Sustain. Dev., 1–23. doi:10.1002/sd.2743
Wang, Q., Sun, T., and Li, R. (2023). Does artificial intelligence promote green innovation? An assessment based on direct, indirect, spillover, and heterogeneity effects. Energy & Environ. 0 (0). doi:10.1177/0958305X231220520
Wang, Q., Wang, L., and Li, R. (2023). Trade openness helps move towards carbon neutrality—insight from 114 countries. Sustain. Dev., 1–15. doi:10.1002/sd.2720
Wang, Q., Zhang, F., and Li, R. (2023). Free trade and carbon emissions revisited: the asymmetric impacts of trade diversification and trade openness. Sustain. Dev., 1–26. doi:10.1002/sd.2703
Wang, R., and Zhu, D. (2018). Foreign direct investment in China's sulfur dioxide emissions. Econ. Surv. 35, 48–55. doi:10.15931/j.cnki.1006-1096.2018.02.004
Wang, X., Wang, Z., and Pan, Y. (2020). Problems and countermeasures of ai application in the field of water and environment in the 21st century. Proc. Chin. Acad. Sci. 35 (09), 1163–1176. doi:10.16418/j.issn.1000-3045.20200530001
Xu, J., and Li, C. (2023). Scale effect" or "crowding effect"? study on the nonlinear effects of dairy farming industry agglomeration on environmental efficiency. Agric. Tech. Econ. 10, 22–43. doi:10.13246/j.cnki.jae.2023.10.006
Xu, M., and Zhang, J. (2018). The impact of industrial agglomeration on air quality. Urban issues. 7, 55–62. doi:10.13239/j.bjsshkxy.cswt.180708
Xuan, Y., and Zhang, W. (2021). Smart city, economic agglomeration and green total factor productivity. Mod. Econ. Discuss. 2021 (09), 12–25. doi:10.13891/j.cnki.mer.2021.09.003
Yang, X. L., Chen, Y. Y., Ren, H., and Li, Y. L. (2023). The relationship between technological innovation, industrial agglomeration, and air pollution in the yellow river basin: a pvar model analysis based on 69 prefectural cities. Ecol. Econ. 39 (10), 191–199.
Yu, B., and Fan, c. (2022). Green finance, technological innovation and high-quality economic development. Soc. Sci. Nanjing 2022 (09), 31–43. doi:10.15937/j.cnki.issn1001-8263.2022.09.004
Yuan, H. X., Liu, Y. B., Hu, S. L., and Feng, Y. D. (2019). Does industrial agglomeration aggravate environmental pollution? -Based on the perspective of foreign direct investment. Yangtze River Basin Resour. Environ. 28, 794–804. doi:10.11870/cjlyzyyhj201904006
Zhang, H. (2016). Strategic interaction study of regional environmental regulation — explanation of the universality of incomplete implementation of environmental regulation. Industrial Econ. China 2016 (07), 74–90. doi:10.19581/j.cnki.ciejournal.2016.07.006
Zhang, R., and Yu, H. (2023). Arctic energy development in the context of carbon neutrality: progress, obstacles, and impact. Soft Sci. China 2023 (07), 1–13. + 22.
Zhang, R. L., and Liu, M. H. (2022). Industrial collaborative agglomeration, industrial coupling coordination and high quality economic development. Bus. Res., 13–22. doi:10.13902/j.cnki.syyj.2022.06.010
Zhang, Y. S., Chao, Q. C., Chen, Y., Zhang, J. Y., Wang, M., Zhang, Y., et al. (2021). China's carbon neutrality: leading the global climate governance and green transition. Int. Econ. Rev. 153, 9–26. doi:10.1142/s2345748121500196
Zheng, H., Fang, X. M., and Su, M. Y. (2023). Industrial agglomeration, environmental regulation, and efficiency of industrial green development: an empirical study based on the Chengdu chongqing dual city economic circle. Statistics Decis. 39 (8), 74–79. doi:10.13546/j.cnki.tjyjc.2023.08.013
Zheng, J., Zhao, Q., and Zhu, H. (2021). Financial Structure and Environmental Pollution: a preliminary theoretical study of environmental finance in the new structure. Explor. Econ. Issues 2021 (10), 165–172.
Keywords: international R&D capital, industrial agglomeration, spatial effect, air pollution, the threshold effect analysis
Citation: Gao D, Deng Y and Chen Z (2024) Industrial agglomeration, international R&D capital and air pollution-based on provincial spatial panel data. Front. Environ. Sci. 12:1355584. doi: 10.3389/fenvs.2024.1355584
Received: 14 December 2023; Accepted: 12 February 2024;
Published: 29 February 2024.
Edited by:
Jiaoyue Wang, Chinese Academy of Sciences (CAS), ChinaReviewed by:
Qiang Wang, China University of Petroleum, Beijing, ChinaCopyright © 2024 Gao, Deng and Chen. This is an open-access article distributed under the terms of the Creative Commons Attribution License (CC BY). The use, distribution or reproduction in other forums is permitted, provided the original author(s) and the copyright owner(s) are credited and that the original publication in this journal is cited, in accordance with accepted academic practice. No use, distribution or reproduction is permitted which does not comply with these terms.
*Correspondence: Yan Deng, ZGVuZ3lhbmVyMjAyM0AxNjMuY29t
†ORCID: Yan Deng, orcid.org/0000-0002-3524-1168
Disclaimer: All claims expressed in this article are solely those of the authors and do not necessarily represent those of their affiliated organizations, or those of the publisher, the editors and the reviewers. Any product that may be evaluated in this article or claim that may be made by its manufacturer is not guaranteed or endorsed by the publisher.
Research integrity at Frontiers
Learn more about the work of our research integrity team to safeguard the quality of each article we publish.