- 1Department of Geography and Planning, University of New England, Armidale, NSW, Australia
- 2Ministry of Environment, Battaramulla, Sri Lanka
- 3Alliance of Bioversity International and CIAT, Rome, Italy
- 4Department of Environmental Management, Rajarata University of Sri Lanka, Mihintale, Sri Lanka
- 5School of Environmental and Rural Science, University of New England, Armidale, NSW, Australia
- 6Faculty of Agriculture, Rajarata University of Sri Lanka, Mihintale, Sri Lanka
Social-ecological systems (SESs) possess a great diversity of land use and land cover (LULC) types with unique assemblages of biodiversity and ecosystem services. However, LULC changes due to landscape fragmentation are emerging as major threats to the system productivity of SESs around the world. This study examined changes to LULC extent and landscape patterns in the Village Tank Cascade Systems (VTCSs) of Sri Lanka using satellite imagery and GIS techniques between 1994 and 2021. Multispectral Landsat images (5 TM and 8 OLI/TIRS) obtained from Google Earth Engine were classified using machine learning algorithms. Overall accuracies obtained were 85.9% (1994) and 88.6% (2021). The LULC change matrix and spatial pattern metrics were used to examine LULC and landscape pattern change dynamics over the VTCS landscapes. LULC change matrix results revealed that forest, which is the dominant LULC class covering 73.7% of the total land area was reduced by 206,725 ha due to transformation into agricultural (70.43%) and scrub (24.33%) lands between 1994 and 2021. Over this time landscape pattern of the VTCS has gradually changed from forest to agricultural land-dominated landscape, with forest and agricultural land types showing a significant negative correlation (p < 0.001; R2 > 0.868), particularly in the southeastern region. Landscape patterns were analysed based on eight spatial metrics calculated at both the landscape and class levels using FRAGSTATS spatial pattern analysis software. At the landscape level, the structure became more dispersed and complex in shape. Heterogeneity was noted to have gradually increased with weakening connectivity, whereas the fragmentation process had gradually accelerated. At the class level, the dominance of forest patches decreased, fragmentation and isolation increased, and connectivity and shape complexity reduced leading to the loss of fragmented forest habitats. The number of patches within the agricultural class increased and became more aggregated and complex in shape. Landscape performance indicators show that VTCSs have experienced a gradual loss of environmental sustainability. Assessment of LULC along with fragmentation can help to monitor the spatial pattern impacts that determine ecological integrity. Thus, the study provides scientific guidance for ecological restoration in degraded VTCSs to effectively improve ecological productivity.
1 Introduction
Social-ecological systems (SESs) around the world are receiving increased recognition in scientific research as they are facing many challenges due to the dynamics of anthropogenic environmental changes [1-3]. Social-ecological system (SES) landscapes are recognised as landscapes managed by local people based on their traditional knowledge and long-term interaction with the surrounding natural environment and biocultural values [4]. In most cases, a mosaic of multifunctional land use and land cover (LULC) systems in these landscapes provide bundles of ecosystem services that contribute to resilience and sustainable food production [5]. However, over the last four decades, SESs have experienced profound socio-economic transformations leading to the degradation of ecological and cultural values embedded in the landscape structure and composition [3]. Many studies present LULC change as one of the leading drivers, alongside climate change, of the deterioration of SES landscape values [6-13].
SES landscapes, referred to as Village Tank Cascade Systems (VTCSs) in Sri Lanka have been designated as a Globally Important Agricultural Heritage System (GIAHS) in 2017 [14]. The GIAHS, initiated by the Food and Agriculture Organization of the United Nations (FAO) in 2002, aims to identify and safeguard agricultural heritage systems around the world. The significant feature of the GIAHS landscape is the remarkable mosaics of LULC systems. The landscape structure and pattern support a high level of agrobiodiversity and biocultural diversity that, in turn, sustain local food systems [4, 14]. However, the Green Revolution (during the 1960s), transformed land use patterns in GIAHSs, shifting focus to increasing economic and social productivity, while giving less attention to ecological productivity [15, 16]. As a result of this shift in traditional agricultural practices, which has resulted in ecological and cultural integrity and their interconnectivity to the sustenance of local food systems being ignored, GIAHSs in several parts of the world, including the VTCSs of Sri Lanka, have partially collapsed [17-19]. In addition, over the past couple of decades, VTCSs have encountered issues (e.g., encroachment of forest habitats and illegal fellings of forest trees) due to the absence of proper local land governance, resulting in these landscapes being more vulnerable to anthropogenic forces [20-23].
LULC changes in VTCSs, directly and indirectly, impact ecologically important habitats of VTCS landscapes that generate ecosystem functions and damage ecosystem services that are important for maintaining resilience and sustainable food production [24, 25]. Most LULC changes occurring in VTCSs are manifestations of long-term interactions between anthropogenic and environmental drivers [26-31]. LULC changes in VTCSs are characterised not only by changes to the extent of a given LULC type but also by changes in attributes, such as shape, connectivity, aggregation, dispersion and diversity that determine the composition and configuration of the landscape [32]. Given the strong link between LULC systems and ecological integrity, it is important to determine changes in land use and cover types as well as changes to the structure and pattern of the landscape, especially to core and edge habitats of particular land use and cover types [33]. Structural and pattern changes influence the ecology of tank cascade ecosystems by altering interactive relationships between biota and their abiotic environment in the VTCSs over time [29]. The literature review revealed that studies on the evolution of rural SES landscape patterns due to the LULC transformation are lacking, while many focused on urban landscapes [34, 35]. Thus, understanding the impact of landscape pattern changes on ecological integrity is key to ecological restoration and sustainable land management in the VTCSs [36, 37].
Remote sensing techniques are popular among researchers analysing LULC change because land cover data can be obtained through satellite imagery conveniently and cost-effectively without direct access to local areas [38]. Thus, many studies have used satellite imagery coupled with GIS and remote sensing techniques to assess and quantify LULC changes and landscape pattern dynamics in rural and urban landscape management more broadly [39]. Machine-leaning algorithms have made considerable contributions to the classification of the LULC in many regions [40-42]. Among these, support vector machine and random forest algorithms have been used to classify the remote sensing images in previous studies conducted in rural and urban landscapes in Sri Lanka [23, 43]. Landscape pattern dynamics can be quantified through spatial metrics (landscape metrics) which express and quantify the characteristics of landscape pattern change [44]. Moreover, spatial metrics are important indices for understanding how landscape fragmentation occurs and its implications for the ecological integrity of the landscape [45-48].
This study systematically examines long-term LULC changes in the North and North-central VTCS zone of Sri Lanka between 1994 and 2021. Studying LULC changes and their impacts in this area is significant because this zone is becoming a climate and LULC change hotspot for environmentally and social-ecologically sustainable food production [49-52] (see section 2.1). Therefore, the study attempts to: i) establish the characteristics of spatiotemporal transformations that occurred in LULC systems of the VTCS landscape between 1992 and 2021; ii) analyse the changes that occurred to the spatial pattern of the VTCS landscape due to the LULC transformations between 1992 and 2021; and iii) determine the major implications of the LULC transformation and landscape pattern dynamics in VTCSs.
Achieving these objectives will provide the quantitative data necessary to further examine the significance of LULC changes on the ecological productivity of VTCSs. Thus, the findings of this study support ecological restoration planning of degraded lands of this GIAHS which is in line with the implementation of the 2030 Sustainable Development Goals (SDGs) target 15 on ‘Life on Land’ (15.1, 15.2 and 15.3), and target 2 of the Kunming-Montreal Global Biodiversity Framework (GBF) [53-55].
2 Materials and methods
2.1 Study area
The study was confined to the VTCS zone located in the north and north-central parts of the dry zone of Sri Lanka (Figure 1). The selected landscape is the largest VTCS zone of Sri Lanka with 617 VTCSs (58.8% of total VTCSs) [56]. The sacred cultural city of Anuradhapura, which was declared a World Heritage cultural city by UNESCO in 1982, is located in the heart of this zone. The VTCSs started to evolve based on this hinterland of the kingdom Anuradhapura in the fourth century BCE [57]. The North and North-central VTCS zone occupies about one million hectares, which is approximately 30% of the dry zone extent of Sri Lanka, of which, about 20% is used for paddy farming [17, 56]. Further, this zone claims approximately 16% and 27% of the paddy farming areas of the total land extent of Sri Lanka and the low country dry zone of Sri Lanka, respectively [58]. The tanks and the minor irrigation network at this zone allow farmers to grow paddy during the wet and dry seasons, either as an irrigated or a rainfed crop. Paddy (Oryza sativa L.) is the staple food of the population in Sri Lanka.
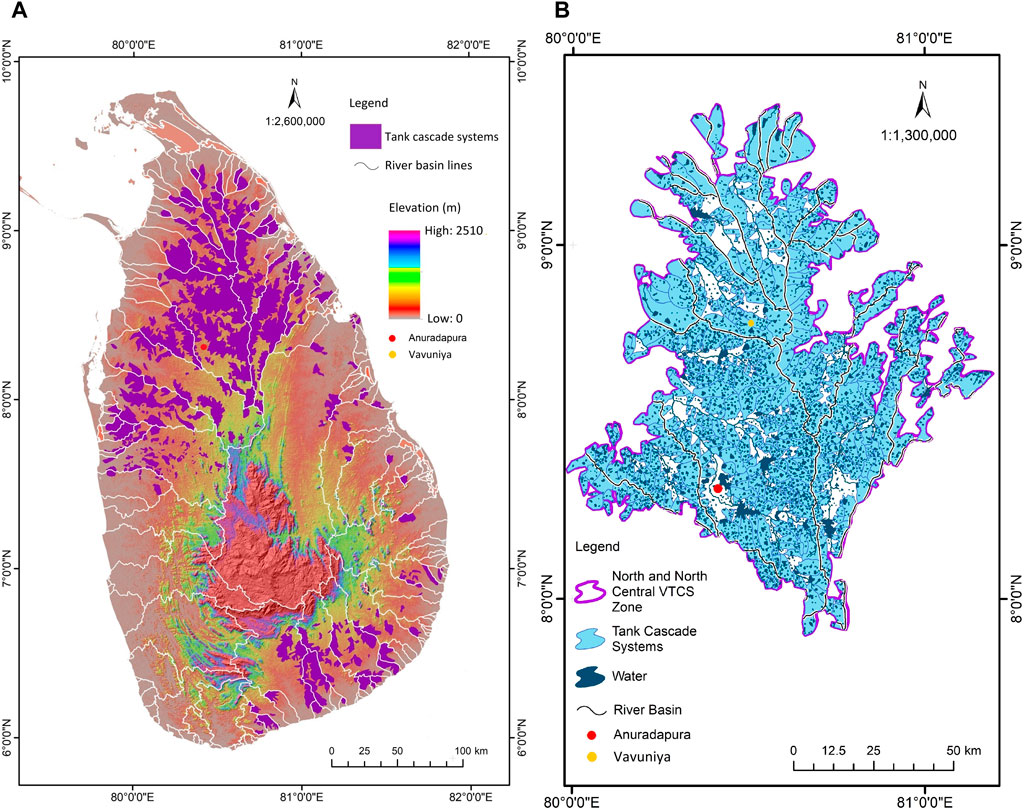
Figure 1. Location of the study area: (A) distribution of Village Tank Cascade Systems (VTCS) of Sri Lanka; (B) study area (North and North-central VTCS zone).
The entire VTCS zone is located within a single agroecological region (DL1) of Sri Lanka [59, 60]. The study area is characterised by a tropical monsoonal climate with a bi-modal rainfall pattern. The average annual rainfall in the study area is 1,320 mm and the average daily ambient temperature is 28°C according to the weather data of the Anuradhapura meteorological station [61]. The major soil groups prominent in this area are Reddish Brown Earths—Rhodustalfs (60%), Low Humic Gley—Tropaqualfs (30%) and alluvial (10%) [29]. There are undulating terrain features in this area with elevations ranging from 100 to 300 m amsl [29, 56]. The land use and cover systems of the VTCS zone, including features such as ecology, hydrology and vegetation, provide a favourable setting for the adoption of social-ecologically sustainable farming systems that are more resilient to the impacts of climate change [29, 59, 62, 63]. The bi-modal rainfall pattern allows farmers to cultivate two cultivation seasons: i) Yala (dry season) and ii) Maha (wet season). However, studies revealed that this zone is becoming vulnerable as a sustainable food production zone due to unpredictable climatic variations and reduction of environmental sustainability due to long-term changes to LULC systems caused by population growth, urbanisation and economic development [50, 63-67]. A significant forest cover loss was revealed in Anuradhapura district according to forest cover change assessment using satellite images during the last three decades (1992–2019) [23].
2.2 Preparation of satellite data
The study used Google Earth Engine (https://code.earthengine.google.com/; accessed on 10 December 2022) to acquire Landsat satellite images (5 TM) for 1994 and (8 OLI/TIRS) for 2021 with 30 m spatial resolution and multispectral bands based on the availability of high quality imagery for the study area during the evaluated period. The study extracted the annual median to produce cloud-free images with pixels of high quality for 1994 and 2021. In this study, we used 41 images for 1994 and 102 images for 2021 to obtain annual median pixels (Supplementary Table S1). The extracted images were then used for LULC classification in the study area described in Section 2.3. A flow chart of the study methodology is presented in Figure 2.
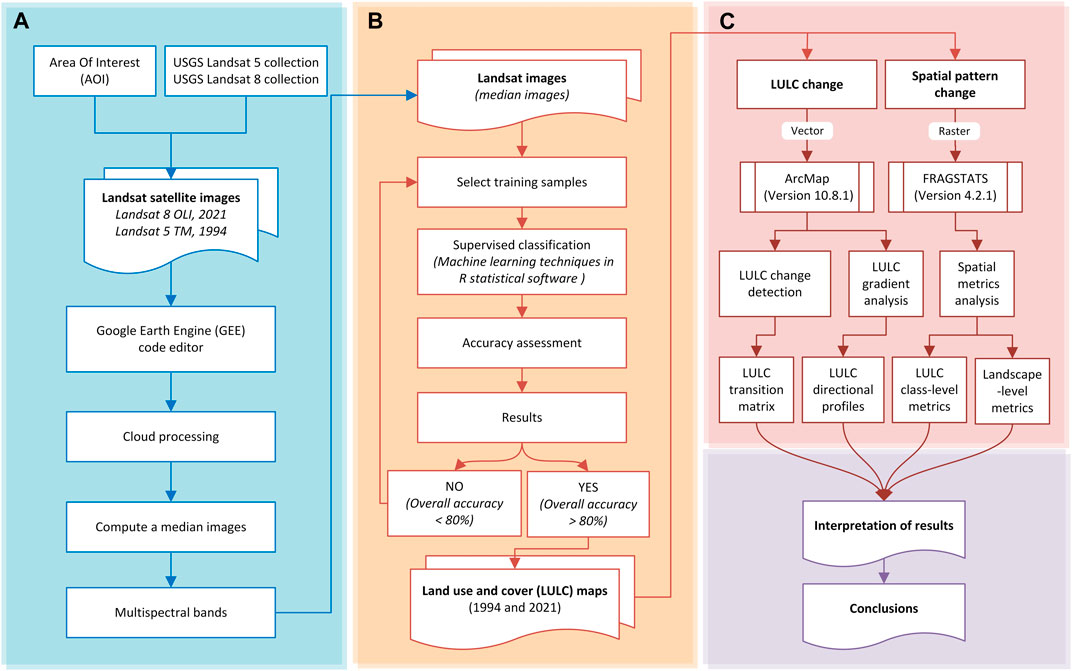
Figure 2. Workflow chart of the study methodology: (A) acquisition and pre-preparation of satellite data; (B) LULC classification and accuracy assessment; and (C) Analysis of LULC change and spatial metrics.
2.3 Land use land cover change detection
2.3.1 Defining LULC classification scheme
The study defined and adopted a LULC classification scheme encompassing five major socio-economically and ecologically important LULC systems of VTCSs, namely, agriculture, forest, waterbody, scrub land and other lands based on a 1:50,000 land use map of the Land Use Policy Planning Department of Sri Lanka. These LULC systems were a result of merging into broad groupings (Table 1). For example, LULC types of dense forest, open forest, micro forest and forest plantation were aggregated as forest LULC system as the study aims to investigate overall forest cover changes. Similarly, built-up land, barren land, commercial land and industrial land were put together as one group due to the intermixed nature of these LULC types. The study further aggregated paddy, shifting cultivation, seasonal crop and home garden horticultural lands as agricultural LULC system because they are small-scale farmlands and, in many cases, combined with hamlets because they are difficult to be distinguished from each other without fine scale image classifications. Further shrub land, grass land, and rock outcrop were categorised as scrub land LULC system due to their heterogeneity.
2.3.2 LULC classification and accuracy assessment
The study used more than 2000 training samples from the study area for the periods of 1994 and 2021 for supervised classification. The LULC classification, used machine learning algorithms such as Random Forest (RF), K-Nearest-Neighbor (KNN), Support Vector Machines (SVM), and Neural Network (NN) available in R statistical software [68]. The accuracy of the classification methods was determined by considering each year’s overall accuracy and kappa statistics. The LULC for 1994 and 2021 were classified using the SVM algorithm, and the RF method, respectively [69]. The study used ancillary data and visual interpretation to identify misclassified areas [70]. The hybrid classification and majority filter (8 by 8) methods were used to remove the misclassification errors. These methods are commonly used to remove the misclassification errors in Landsat data [71-73]. A random sampling method was used to generate 500 random points each year, and accuracy was checked using ground truthing with Google Earth. The data obtained from the comparison between the reference and actual points were compared, and finally, the user’s accuracy, producer’s accuracy, and overall accuracy were extracted for classified LULC maps of 1994 and 2021 using ArcMap (version 10.8.2) [74, 75].
2.3.3 LULC change gradient analysis
To analyse multidirectional LULC change dynamics, a gradient analysis was applied using GIS-based concentric rings surrounding the geometrical centre of the study area [76]. A 2 km buffer zone was created, overlaying with the LULC maps of 1994 and 2021. The concentric zones were divided into four quadrants (northwest, northeast, southwest and southeast) to extract multidirectional LULC to analyse LULC change between agricultural land and forest in the four directions in 1994 and 2021 from the centre of the study area (Supplementary Figure S1). After the four directional profiles were created, forest and agricultural land percentages were extracted for each buffer zone (buffer zone samples) and finally, the correlation was calculated using forest and agricultural land percentages.
2.4 Analysis of landscape pattern changes
The study used FRAGSTATS version 4.2.1 [77] (https://www.fragstats.org, accessed on 15 June 2023) for the computation of spatial metrics for forest and agricultural LULC types in the study area. FRAGSTATS is a free software (spatial pattern analysis program for quantifying the structure of landscapes or categorical maps) developed by Oregon State University [33, 78]. FRAGSTATS can measure spatial metrics at different spatial scales (landscape, class and patch), for landscape patterns (landscape composition and spatial configuration) analysis. Further, FRAGSTATS can compute several statistics that quantify landscape heterogeneity and provide first-order and second-order statistical summaries of the class-level and patch-level metrics for the entire landscape [78]. There is an increasing trend of using FRAGSTATS among ecologists to analyse, characterise and undertake evidence-based decision-making on the fragmentation of social-ecologically important landscapes [13].
Raster maps of agriculture and forest LULC types for 1994 and 2021 were processed in ArcMap software and used as input data for FRAGSTATS [77]. The study employed spatial metrics to measure and describe the spatial pattern change under agriculture and forest LULC types in the study area. Due to the high diversity and heterogeneity of the LULC system of VTCS landscapes, five levels of potential spatial metrics in different spatial scales can be identified, namely, micro patch-level, patch-level, class-level, micro catchment-level and landscape-level (Figure 3). The study employed spatial metrics at the landscape-level and class-level to measure and describe the spatial pattern change under agriculture and forest LULC types in the study area because agroecosystems and forest ecosystems are considered the most important social-ecological LULC systems of the productivity and sustainability of GIAHS [79, 80].
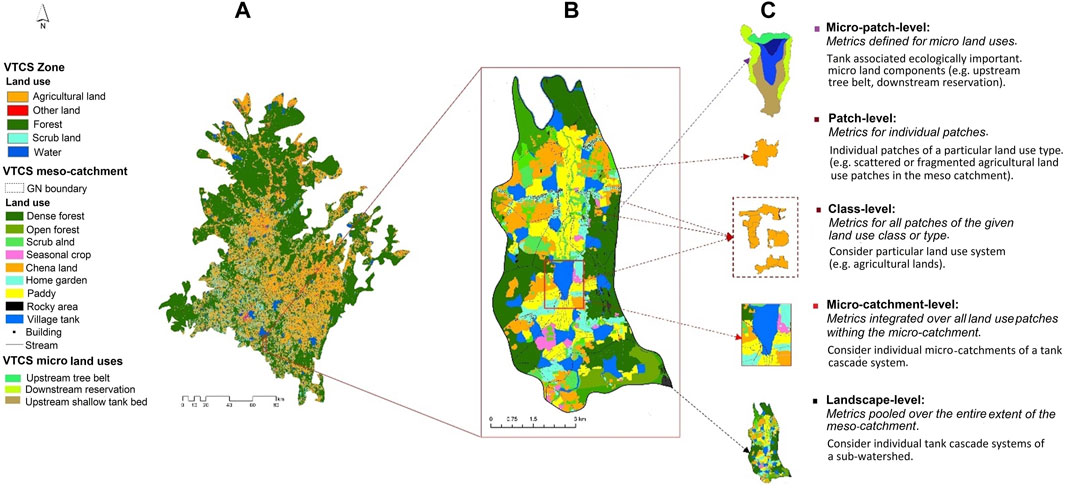
Figure 3. Graphical illustration of potential levels of spatial metrics identified in a Village Tank Cascade System (VTCS) landscape based on social-ecological land use types for analysing landscape pattern changes: (A) Land use and land cover (LULC) in North and North-central VTCS zone in 2021; (B) a VTCS landscape meso-catchment showing a mosaic of LULC types; and (C) potential levels of spatial metrics identified in the VTCS landscape.
The study analysed three metrics at the landscape level: i) landscape shape index (LSI); ii) contagion index (CONTAG); and iii) Shannon’s diversity index (SHDI); and five class-level metrics; i) a percentage of landscape (PLAND); ii) patch density (PD); iii) mean patch size (Area_MN); iv) area-weighted mean patch fractal dimension (Frac_AM); and v) mean Euclidean nearest neighbour distance (ENN_MN) to evaluate forest and agricultural LULC systems in 1994 and 2021 as described in Table 2. All the aforementioned metrics were computed using the FRAGSTATS eight-cell neighbour rule [13]. Metric selection was based on previous related studies of rural landscape patterns that have demonstrated which metrics provide the best measures of changes to ecological productivity and social-economic driving forces of VTCS landscapes [48, 81-86]. The selected spatial metrics reflect the measures of aggregation, dispersion, shape complexity, heterogeneity, composition, dominance, fragmentation, structural complexity, connectivity or/and isolation from the class and landscape levels to the study landscape. In addition to the FRAGSTATS metrics, the study used two more landscape-level performance indicators adopted and modified from the literature [84, 87] to assess the impact of LULC change on the sustainability and productivity potentials of the VTCS landscape: i) Sustainable Land Use Indicator [SLUI] defined as the ratio between forest lands and agricultural lands; and ii) Agricultural Land Use Intensity [ALUI] defined as the ratio between the total VTCS landscape area and the total agricultural lands.
3 Results
3.1 LULC classification and change detection
The classified LULC maps for the years 1994 and 2021 are presented in Figure 4. The results of spatial and temporal changes that have occurred on the LULC classes are presented in Table 3. The findings show that agricultural and forest LULC classes dominate to the greatest extent in the VTCS zone in both the 1994 and 2021 periods compared with other classes. Scrub lands occupied 660 ha in 1994 and increased to 76,474 ha in 2021, reflecting an increase of 8.5% over 27 years. Waterbodies and other land classes (see Table 1) remained more or less stable in extent over the period. The results show a considerable expansion of agricultural lands from 185,521 ha (20.9%) in 1994 to 280,613 ha (31.7%) in 2021. More agricultural land expansion can be seen in 2021 on the southeastern side of the study area, mostly encroaching forest lands. Meanwhile, the extent of forest cover decreased from 73.7% in 1994 to 54.6% in 2021. Generally, forest cover reduction occurs across the whole study area. However, forest cover is reduced at least at the periphery of the zone, especially in the North and North-west. Conversely, greater forest habitat loss occurred in the southeast of the zone. Ratios of the landscape performance indicators imply that the Agricultural Land Use Intensity (ALUI) value decreased from 3.5 to 1.7 and the Sustainable Land Use Indicator (SLUI) value decreased from 4.8 to 3.2 between 1994 and 2021. Decreasing values of both indicators suggest an increased impact of agricultural expansion and intensification on the environmental sustainability of the VTCS landscape.
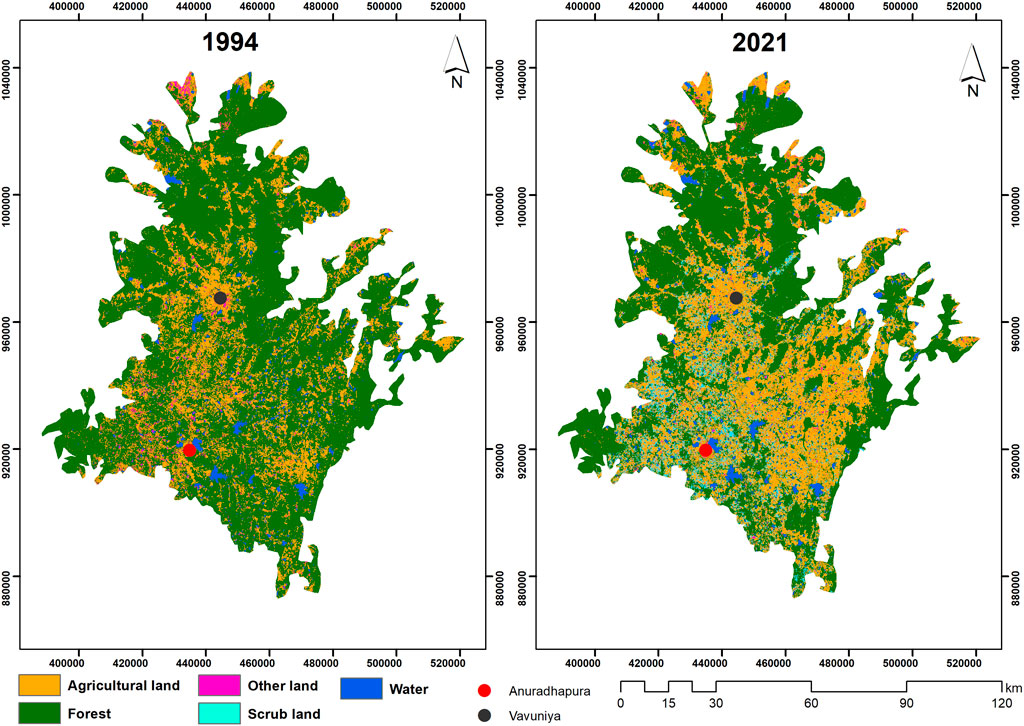
Figure 4. Land use and land cover classification for 1994 and 2021 in the North and North-central VTCS zone.
3.1.1 Accuracy assessment of LULC classification
The accuracy of the classification methods was evaluated by considering each year’s overall accuracy and kappa statistics as presented in Table 5. The overall classification accuracy of 85.9% (Kappa coefficient of 0.79) and 88.6% (Kappa coefficient of 0.84) were attained for 1994 and 2021, respectively (Table 4; Supplementary Table S2). The overall accuracy established above 85% with no LULC type less than 70% is a widely acceptable value in a diverse range of satellite image classification applications [90-92]. Thus, the accuracy values archived in this study indicate acceptable precision of LULC classification and is in agreement with the ground reality.
3.1.2 The dynamics of LULC types
The extent of LULC transformations, annual and net changes between LULC types from 1994 to 2021 are summarized in Table 5. The sum of row and column of each LULC system shows the total extent in 1994 and 2021. According to the LULC transformation statistics, a substantial forest area was converted to agricultural lands during the past 27 years. The net gain of agricultural lands was 95,092.7 ha (51.3%), being an annual increase of approximately 3,522 ha. During the past 27 years, forest cover reduced by −6,275.6 ha per year and the net forest cover lost was 169,441.9 ha (25.9%). The loss in forest cover was 206,725 ha, this transformed mainly into agricultural (70.43%) and scrub (24.33%) lands from 1994 to 2021. Substantial agricultural areas were also converted to scrub lands, resulting in a net gain of 75,814.7 ha from 1994 to 2021.
The LULC dynamics were examined using LULC transfer matrix (Figure 5A) and Sankey map (Figure 5B). The transfer matrix was used to calculate the area of new gains, losses, persistent and swaps between different LULC systems. The transfer matrix summarises the LULC transformations in which the diagonals of the matrix indicate the proportion of LULC systems that showed persistence while the non-diagonal entries comprise the area converted from one LULC system to the other system between 1994 and 2021. The Sanky map was used to visualise these relationships. The LULC change matrix indicates the percentage of transformation from one LULC class to another from 1994 to 2021. According to the results, 66% of the agricultural lands and 68% of the forest lands remained unchanged between 1994 and 2021. During the same period, 22.3% of the forest lands were converted into agricultural lands and 7.7% into scrub lands. In the VTCS zone, 60% of the waterbodies remained unchanged in 2021 and 28% were converted to forest and agricultural lands. Of scrub lands 24% remained unchanged in 2021 and 38% and 20% were converted into agricultural lands and forest lands, respectively. A further 56% of the other lands were converted into agricultural lands from 1994 to 2021. The Sankey diagram visualises the LULC transition flows from one LULC class to another from 1994 to 2021. The results indicate that within the VTCS zone 19.1% of forest cover was lost and agricultural lands increased by 10.8% between 1994 and 2021. Further, LULC transition flows show majority of newly gained agricultural lands (>90%) were converted from forest lands. Scrub lands increased by 7.8% from the total land in 2021 and, similar to agricultural land gain, the majority (>65%) was gained from forest lands. The most significant LULC transformation pattern of VTCS landscapes was observed between forest and agricultural lands which was indicated in the Sankey map. In addition, scrub lands show a significant gain. Results show that other LULC transformations between LULC types were not remarkable.
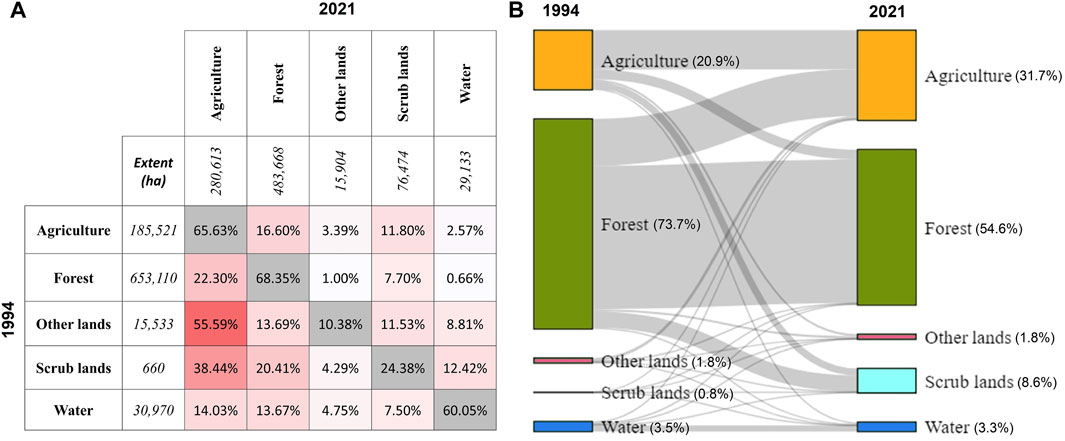
Figure 5. The land use and land cover (LULC) transformation from 1994 to 2021. (A) The LULC area transfer matrix shows the percentage of each LULC class area (ha) converted to other LULC classes from 1994 to 2021 compared with 1994 extent. The diagonal (shaded grey) corresponds to the area of each LULC class that stayed persistent. The darker the shade of red, the larger the percentage of change; and (B) The Sankey diagram showing the LULC transformation flows from 1994 to 2021 in the study area.
Figure 6 shows the loss and gain of LULC types from 1994 to 2021 in the study area. Accordingly, the southwestern and southeastern parts of the VTCS zone underwent substantial LULC changes. For example, most of the forest in the southwestern part of the study areas was converted to agricultural lands and scrub lands. Similarly, a substantial amount of forest was lost in the southeastern region due to the transformation into agricultural lands. Figure 7 shows the comparison of agricultural and forest land composition in 2021 and the gain from different LULC classes relative to the 1994 extent. In 2021, 43.4% of the agricultural lands remained from 1994 to 56.6% was gained from other LULC types, of which 91.7% was from forest lands. For forests, 92.3% of the 1994 forest land remained in 2021 and 7.7% was gained from other LULC types, of which the majority (82.6%) was from agricultural lands in 1994.
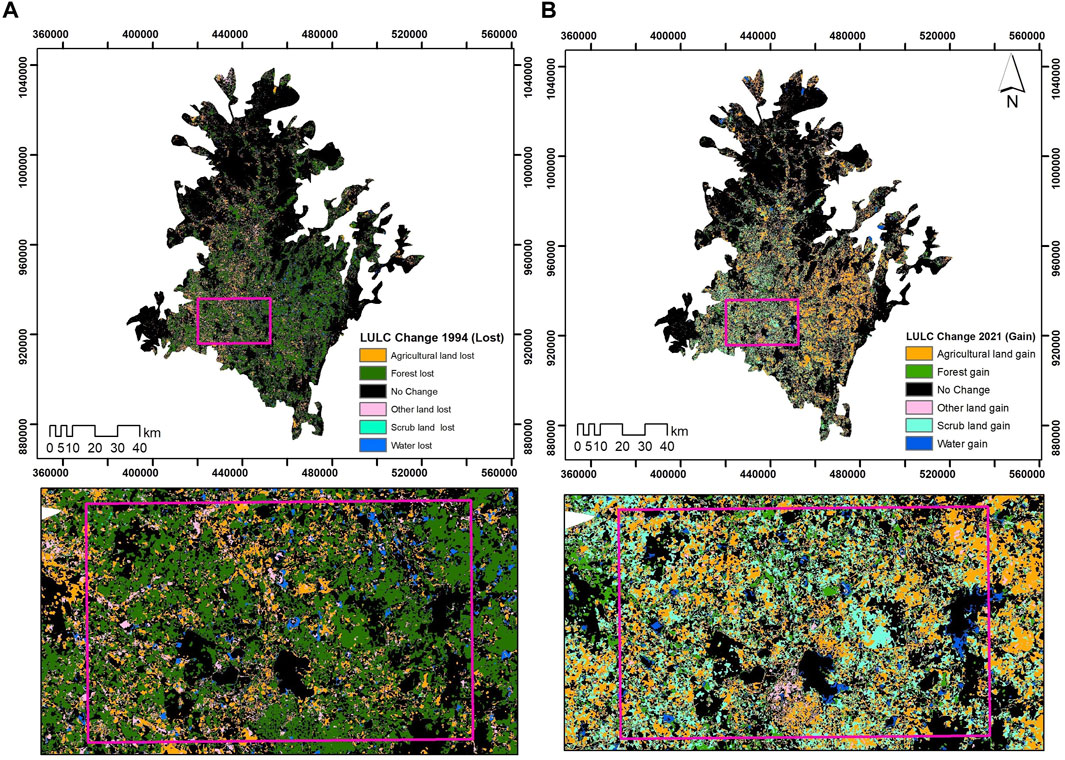
Figure 6. Spatiotemporal land use and land cover (LULC) changes in North and North-central VTCS zone: (A) LULC types lost in 1994, (B) LULC types gained in 2021.
3.1.3 Multidirectional spatiotemporal changes in LULC profiles
Figure 8 shows the multidirectional pattern changes in agricultural and forest LULC profiles, spreading from the centre of the study area to four directions (northeast, southeast, northwest and southwest) in 1994 and 2021. In 2021, the southeast direction, shows an expansion of agricultural lands (40%), from 2 km to 38 km from the centre of the study area. The northeast direction shows the expansion of agricultural lands by 85%, from 74 km to 78 km and a decrease in forest lands by an equal amount over similar distances in 1994 and 2021. In 1994 and 2021, there was a minimal increase in agricultural lands and a decrease in forest lands from 25 km to 74 km towards the northwest direction. Over this period there was a 35% increase in agricultural lands and a 30% decrease in forest lands occurring from 4 km to 18 km. Notable was also the expansion of agricultural lands by 55% and the decrease of forest lands by 70% happening approximately 75 km from the centre towards the coastal areas. Both northwest and northeast agricultural and forest lands LULC profiles showed similar changing patterns in 1994 and 2021.
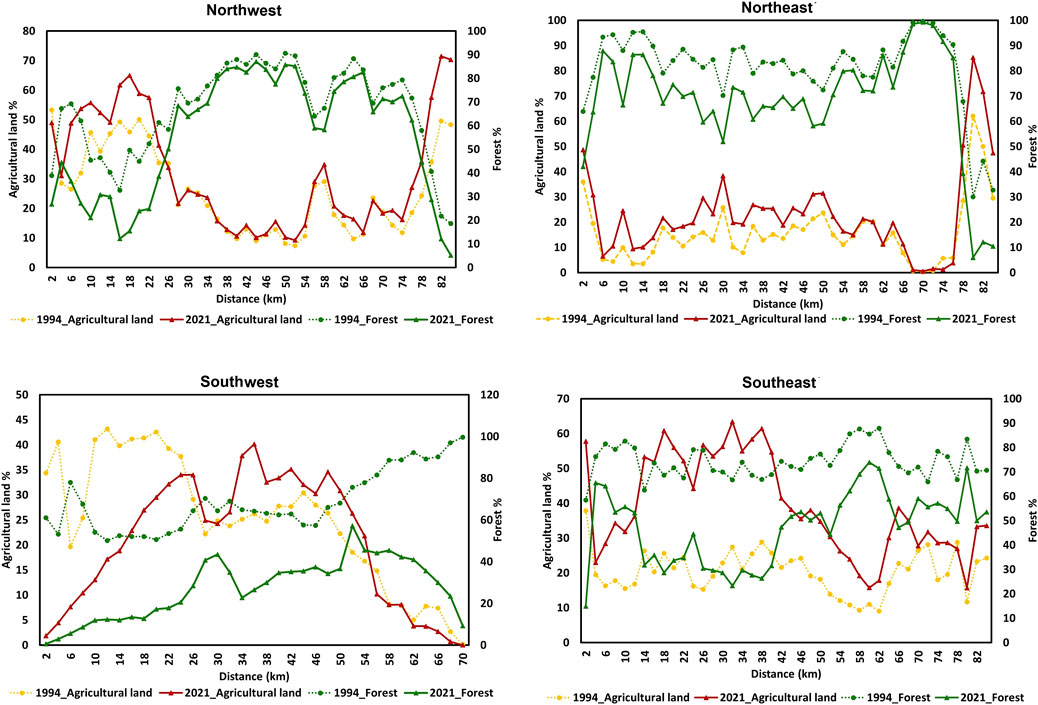
Figure 8. Multidirectional and multitemporal change of agricultural and forest land profiles from the centre of the study area in 1994 and 2021.
In 2021 agricultural land cover profile showed an increase up to 35 km from the centre of the study area towards the southwest and southeast directions and thereafter decreased towards the coastal area. In 1994 agricultural land cover profile of the southwest direction showed a decrease from the centre of the study area towards the coastal area. However, in 1994 the southeast direction, agricultural land cover profile did not show a large variation from the center of the study area towards the coastal area. In the southeast direction, the agricultural land cover profile shows high agricultural intensification in 2021 compared to 1994. In the southern part, the agricultural and forest land cover profiles, show a substantial difference between 1994 and 2021 indicating forest land loss or encroachment in 2021 compared to 1994. In the southwest direction, forest land increased up to 52 km from the centre of the study area towards the coastal areas, indicating less disturbance for forest lands compared to the southeast. Figure 9 plots the coverage of forest and agricultural land for each concentric zone within each quadrant in 1994 and 2021. It shows that percentages of agricultural land and forest cover had a significant negative correlation (p < 0.001; R2 > 0.868). However, in 2021 in the southwestern directions, it showed a weak significant positive correlation (p < 0.001; R2 > 0.05).
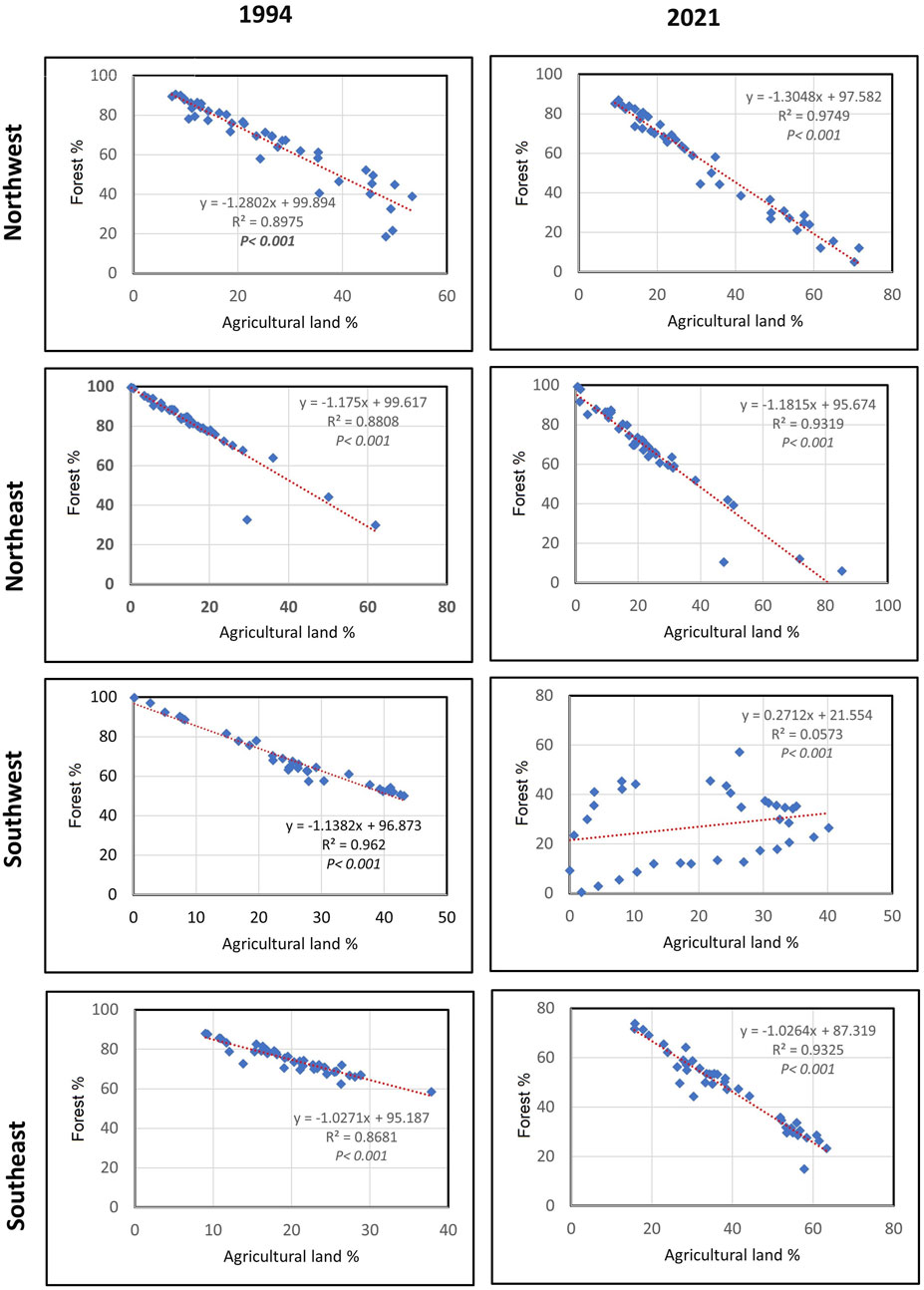
Figure 9. Scatter plots and statistical relationships between the agricultural lands and forest cover change in four directions in 1994 and 2021 from the centre of the study area.
3.2 Spatiotemporal changes in spatial metrics
Figure 10A shows the results for landscape-level metrics in the study area. It shows a decreasing CONTAG (contagion index) and increasing LSI (landscape shape index) and SHDI (Shannon’s diversity index) from 1994 to 2021. The CONTAG value decreased by approximately 20%, indicating that the degree of fragmentation in the North and North-central VTCS landscapes increased. The SHDI increased by approximately 0.35, implying an increase in the diversity or heterogeneity of the LULC patches making up the VTCS landscape. The increase in LSI from 90 to 170 indicates the degree of complexity in the shape of LULC patch types in the VTCS landscape increased in 2021.
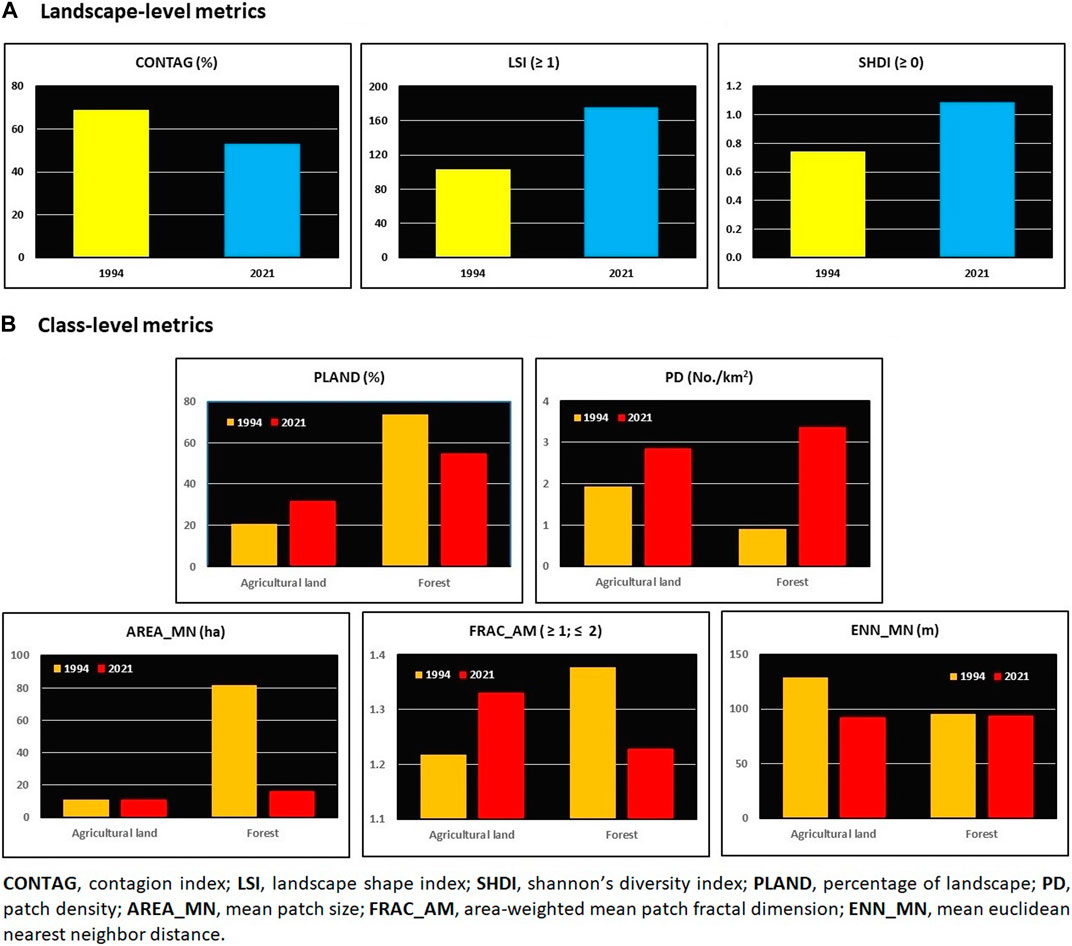
Figure 10. Spatial metrics analysis of agricultural and forest lands of the North and North-central VTCS zone in the years 1994 and 2021: (A) landscape-level metrics, and (B) class-level metrics for agricultural and forest lands.
Figure 10B shows changes in class-level metrics for forest and agricultural lands in the study area from 1994 to 2021. The result shows an increase in PLAND (percentage of agricultural land use class) by approximately 10% and PD (patch density) by approximately 1 in agricultural land use class and a decrease in PLAND (approximately 20%) and an increase in PD (approximately 2.5) in forest land use class. Increased PD and decreased AREA_MN (mean patch size) and ENN_MN (mean Euclidean nearest neighbour distance) values for forest indicate that forest became more fragmented and complex from 1994 to 2021 as larger patches were subdivided into smaller ones. The FRAC_AM (measure of the shape complexity of patches) of agricultural land use class increased in 2021, indicating that agricultural land use patches are complex in shape and do not tend to present simple perimeters in those patches. Further, a higher Frac_AM value indicates a greater proportion of the landscape is agricultural land. Conversely, the Frac_AM of forests decreased in 2021, likely due to deforestation. The ENN_MN for agricultural land use class declines from 1994 to 2021. A lower ENN_MN value in 2021 for patches of agricultural lands was probably due to patches within the class becoming more abundant and more clustered or aggregated in the study area. A slight decrease in ENN_MN for forests between 1994 and 2021, is probably due to the fragmentation of forest patches, resulting in a greater number of patches that are more interspersed in the landscape leading to isolation, reduced core-area and lowered connectivity.
4 Discussion
4.1 Land use and land cover change
This study examined the long-term changes to five macroscale LULC systems in the major VTCS zone of Sri Lanka between 1994 and 2021. The findings from this research revealed that the study area underwent considerable land use and cover changes between 1994 and 2021, the most dominant being the expansion of agricultural land resulting in shrinking and fragmentation of forest land. This change mostly occurred due to the expansion of agriculture into forested areas. According to the multidirectional change analysis the greatest expansion of agricultural lands and forest cover loss occurred in the southern part of the study area compared to the northern part. The increasing demand for new fertile land for food production due to various external and internal pressures might have contributed to the conversion of forest land into agriculture. The patterns revealed in this study are similar to LULC change patterns in similar landscapes in other parts of the world. For example, the expansion of new crop cultivation areas, population growth and urban sprawl have been observed and attributed to increasing pressure on forested lands observed in tropical Asian and African regions [3, 41, 93, 94]. In contrast, cultural landscapes in Europe are threatened by the abandonment of agricultural lands and urbanisation [95]. The loss and alteration of forest habitats affect the biological diversity in the VTCS landscapes [96]. This has consequences on the environmental sustainability and food production of the VTCS because of their reliance on ecological productivity of the landscape [63, 97, 98]. Agriculture expansion at the expense of forest degradation associated with loss of ecological productivity and environmental sustainability agrees with similar studies in tropical and sub-tropical regions [92, 99].
As noted, the observed increase in scrub lands was mainly due to transformation from agricultural lands. This could probably be due to the turn away from traditional shifting cultivation lands that are affected by climate and soil fertility issues [65]. The small-scale shifting cultivation practised by local people in the past did not result in large-scale environmental damage due to the use of traditional practices of soil management to maintain ecological productivity [61, 100, 101]. However, at present shifting cultivation is becoming commercialised and intensive [102] and has lost its sustainability (evidenced by declining soil health) and economic productivity (evidenced by decreased profits) with the influence of the aforementioned driving forces. Thus, new cultivations tend to be established by encroaching on forested areas of VTCSs, because these forest lands are rich in soil organic matter. This trend can be seen in other tropical Southeast Asian, South Asian and African regions where shifting cultivation is largely practised [94, 103]. Encroachment and expansion of agricultural land use into forest areas led to forest fragmentation—the creation of new LULC patches around the encroached forest areas of VTCSs, depending on the spatial or environmental characteristics of the forest landscape.
4.2 Spatial metrics analysis
The analysis of three landscape-level metrics (CONTAG, SHDI, LSI) confirmed that the VTCS landscape became more fragmented between 1994 and 2021, altering the landscape pattern over the period. A decrease in CONTAG values reflects an increase in dispersion and/or interspersion of individual patches indicating the increase of inter-patch distance within the landscape [78, 104]. Increased SHDI indicates increased LULC heterogeneity of land use patches (number of LULC patches per unit area) while increased LSI depicts a high degree of complexity in the shape of LULC patches (number of ecotone length per unit area) in the VTCS landscapes [105].
The analysis of five class-level metrics revealed that agricultural lands in the study area are becoming more dominant with a more dispersed mosaic pattern between 1994 and 2021. The overall increase in the PLAND and PD, as well as the FRAC_AM indicates the expansion of agricultural lands, with the creation of new patches explaining an increase in fragmentation as well as the complexity of patch shape between 1994 and 2021. The reduction of distance to the nearest neighbouring agricultural land use patch (ENN_MN) and more or less stable mean patch size (Area_MN) indicate agricultural land patches were gained from other LULC types during the process of LULC transformation between 1994 and 2021.
The forest class metrics show a decrease in the percentage of forest class area (PLAND) between 1994 and 2021, suggesting overall forest loss in the VTCS zone during the evaluated period. Moreover, the reduction in the mean patch size of forest patches (Area_MN) and increase in patch density (PD) suggest that forested areas of VTCSs have undergone the process of fragmentation of forest patches during the evaluated period [106]. From 1994 to 2021, the shape complexity (FRAC_AM) decreased and the distance between patches (ENN_MN) remained largely stable. This suggests the loss of small fragmented forest patches in the landscape, with a few remaining large forest patches of the VTCS having become more isolated and confined to one or two areas. Thus, analysis of class-level metrics provided more detailed information about the process of landscape fragmentation that can be useful for understanding the ecological impacts of fragmentation [32, 105].
4.3 The driving forces and major implications of LULC change
External drivers and internal mechanisms influence LULC changes in VTCSs. Key among these are socio-economic, demographic, political, environmental and climate factors [107]. In this study, it emerged that the main cause of the LULC transformations in VTCSs was the encroachment of forest lands for agricultural expansion. This could have resulted from the population growth and urbanisation pressure as a result of large development programs that occurred after the end of the thirty-year-long civil war in the year 2009 at the North and North-central regions of Sri Lanka [50, 64, 67, 108-111]. According to Toma et al., 2023 [112] and Parvin et al., 2024 [113] socio-demographic factors such as increasing population and changing livelihood patterns increase the demand for food production. Thus, intensification of agriculture was obvious to ensure food security in the VTCSs. There is ample literature that supports the fact that social, economic and demographic factors are the major causes of LULC change dynamics in SESs, a phenomenon similar to the VTCSs [50, 64, 114]. In addition, policy gaps in natural resource conservation and sustainable land management have also been highlighted to influence socio-economic drivers in the study area [113, 115, 116]. Political drivers may also influence the natural resource governance in the VTCS areas [117, 118].
The findings of spatial metrics assessment established that LULC transformation resulted in uneven fragmentation of agricultural and forest lands in VTCSs. Thus, LULC dynamics and fragmentation changes the landscape composition and configuration changed considerably between 1994 and 2021. This led to a decrease in landscape variability (diversity of LULC types) and landscape heterogeneity (number, size and arrangement of LULC patches) especially in the forest lands, altering the structural balance of VTCS landscape (spatial proportions among natural, semi-natural and cultivated areas) [84, 87, 105, 119]. Thus, the VTCS landscapes showed decreasing biotic diversity and abiotic heterogeneity required to support and regulate ecosystem services leading to declining ecological productivity, similar to other cultural landscapes in the region [120]. Though agricultural expansion and intensification create economic and social benefits, it causes detrimental problems to ecological and social productivities in the VTCSs as a result of soil erosion, increased levels of nutrient flows, stormwater runoff, increased pollution levels, thermal environment changes and habitat destruction [19].
Fragmentation and loss of forest vegetation lead to modification of atmospheric moisture circulation and evapotranspiration regime in the VTCS environments [121]. In addition, the reduction of forest canopy and soil cover result to increased splash soil erosion due to canopy drips with high kinetic energy and surface runoff leading to increased sedimentation of tanks [61]. This causes increased eutrophication and water pollution in village tanks leading to decreased water productivity and increased risks of floods. Further, tank water pollution increases the risk to human health and aquatic biotopes, and decreased social and ecological productivity of VTCSs [120, 121]. Furthermore, landscape fragmentation alters the structural balance of natural vegetation, water bodies and croplands and may lead to a change in the thermal environment regime in VTCS areas [122]. This may also cause an increase in temperature to levels that may affect both human and crop health [123]. The conversion of forests into agricultural use is acknowledged as one of the main drivers of human-induced climate change [124]. In addition, agriculture intensification may also lead to health implications for communities living in VTCS areas as a result of air and water quality decline. Land use decisions may have a significant impact on air quality in both urban and rural landscapes [125, 126]. Shifting cultivation through slash and burning of forest lands increases smaller carbon particles in the air due to emissions of smoke [107]. Further, overuse of agrochemicals and fertiliser increases pollution loads to downstream areas and may lead to serious health implications [127].
Moreover, fragmentation of the landscape resulted in the weakening of habitat connectivity and likely had impacts on food webs, especially foraging by wild animals living there [128]. Previous research has found that changes in foraging behaviour and patterns of wild animals have led to reduced economic and social productivity of VTCS landscapes (e.g., human-elephant conflict) [65, 129, 130]. Thus, landscape fragmentation could decrease adaptive capacity and increase sensitivity of species communities leading to increased vulnerability to global environmental changes such as climate change [131-134]. Further, fragmentation of ecologically important habitats could impact the probability of bird occurrence in VTCSs which is an important element that contributes to cultural ecosystem services [135]. Therefore, ecological impacts of landscape fragmentation are inevitable and could have major consequences for the sustainability and productivity of the VTCSs. Even though fragmentation resulted in negative consequences on the social-ecological system, increased heterogeneity of agricultural lands due to fragmentation could positively support the production of some ecosystem services such as crop production, carbon sequestration and soil organic carbon retention [136]. Irrational land use planning can also undermine the sustainability and productivity potentials of VTCSs.
So far, only one peer-reviewed research study has been conducted highlighting the process of LULC changes using past topographic maps (1910, 1979, 2002 and 2018) and GIS technology [50]. However, this study was limited to quantifying short-term LULC spatiotemporal transformations in one of the VTCSs. Further, that study did not analyse spatial pattern changes that occurred as a result of LULC transformations. The landscape pattern dynamics due to LULC conversions should be analysed and quantified, as well as the ecological impacts. Therefore, systematic assessment and quantification of LULC change are required to inform social-ecologically acceptable land use policies considering social-ecological interdependencies and interactions to achieve sustainable land management practices in the VTCSs. However, to evaluate the effectiveness of land use decisions whether better or worse outcomes needs proper assessments of the aforementioned drivers and implications. Figure 11 shows the conceptual framework indicating relationships between the drivers of LULC change and their implications on VTCS landscape services and society.
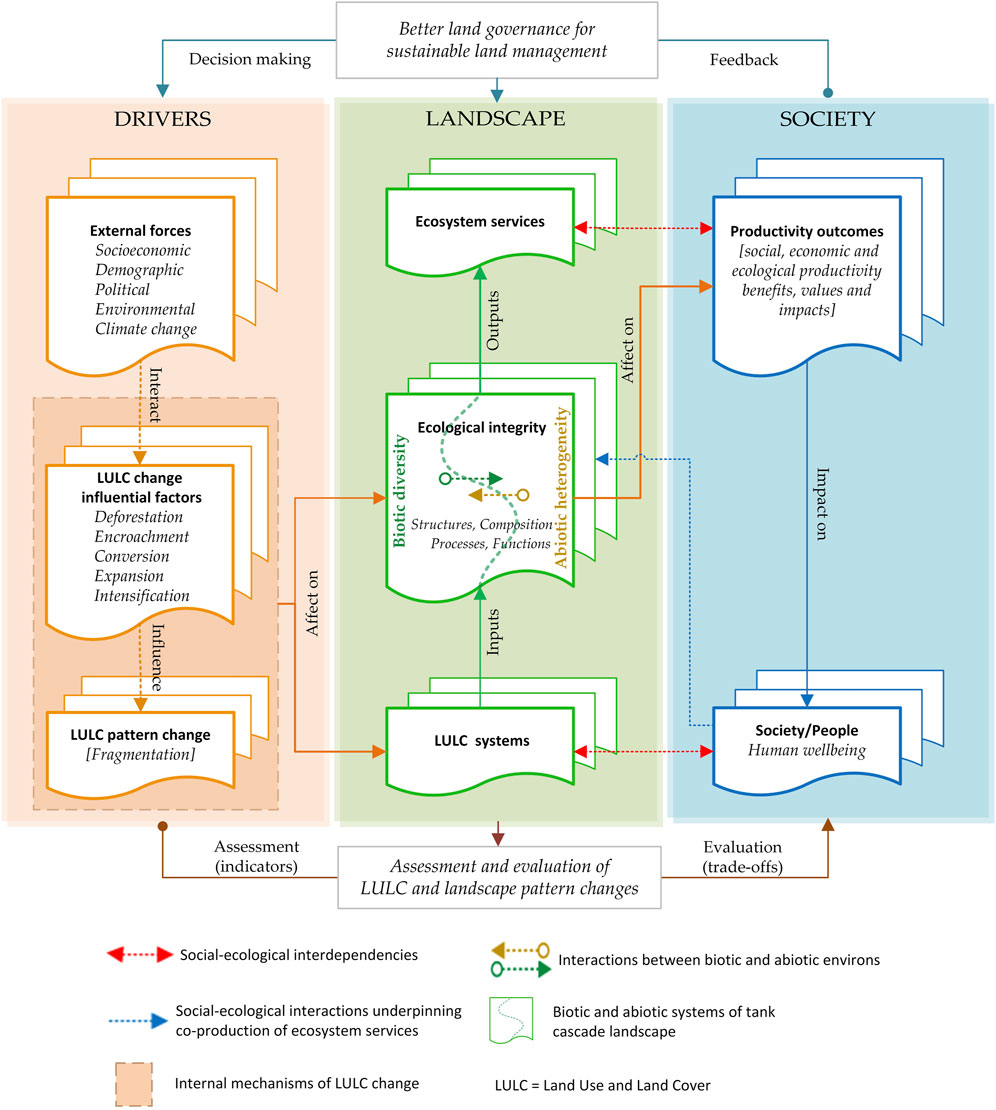
Figure 11. Conceptual framework indicating drivers and implications of LULC changes in village tank cascade systems (VTCSs). The left part of the framework represents the external and internal mechanisms that interact and influence LULC and landscape pattern changes in VTCSs. The right part of the framework represents the benefits/impacts that arise from landscape ecosystem services/disservices to the society means of socio-economic and ecological productivity benefits and impacts.
4.4 Limitations and future directions
This study used Landsat 5 (TM) and Landsat 8 (8OLI/TIRS) images with different precision. In addition, 30 m resolution satellite data were used based on the availability of Landsat images of the study area for the period 1994–2021. The spatial resolution, 30 m of Landsat images is often not enough for capturing all microscale characteristics of land use and cover types such as upstream tree belt and downstream reservation of VTCS [56]. Thus, microscale LULC types that were found difficult to separate due to the limitation of image resolution were merged and segregated into five groups in order to achieve the study objectives. According to Misana et al., 2012 [137], merging different LULC types prevents some important spatial characteristics from being visible and may impact the quality of the classification. However, the five macroscale LULC classification groups used in this study have also been used for related studies undertaken in tropical regions, including Sri Lanka and were adopted for ease of comparison of LULC dynamics within the region [32, 50, 86, 92, 107, 113]. To enhance the precision of land use change results, future research can consider the use of higher-resolution satellite data (e.g., 10-m resolution Sentinel-2 data) to improve the identification capabilities for small-scale features. In addition, the use of a multi-scale approach that combines data of different resolutions to optimise the accuracy and applicability of land cover classification, especially in ecologically sensitive and complex change areas can also be considered. This study did not also evaluate the LULC data in shorter timescales due to the nature of VTCS rural landscapes that do not show significant changes in shorter timescales like those in urban ecosystems in Sri Lanka. However, in future studies, more detailed time series analyses of LULC change data could be considered to model LULC variations under different land use scenarios and propose corresponding strategies [138].
The study used only eight spatial metrics to evaluate the changes in landscape patterns and their ecological significance. According to the literature, several other types of ecologically important spatial metrics could improve the metrics analysis such as edge density, total core and edge contrast index [45, 89, 139]. However, considering the diversity of available spatial metrics and the complexity of landscape fragmentation it is difficult to select the optimal group of metrics for a particular landscape [13]. Thus, selecting the best available metrics to describe the ecological impacts of landscape pattern changes needs deep knowledge and understanding of spatial metrics as well as ecological characteristics and driving forces of the study area based on past studies [47, 140]. For example, some measurements explained by spatial metrics’ equations such as ENN_MN (in this study the shortest distance between LULC patches) measure the structural distance between patches [141] that may not reflect real movement patterns (ecological distance or functional movements) of ecologically important species [47]. However, such ecological studies are limited in the study location.
The study did not take into account the anthropogenic, environmental and climate change driving forces that impact landscape pattern changes [31, 47, 142-144]. In addition, it is worth noting that this study did not take into consideration the impacts of new cultivations that result from shifting cultivation. Due to the scope of the study, in-depth analysis of the socio-economic and ecological impacts of LULC changes were not accounted. The potential impacts of environmental pollution parameters, such as water and air quality, due to agricultural land expansion were not considered. Accordingly, the landscape pattern changes influence ecosystem functions that generate ecosystem services were also not considered. These aspects could be considered in future VTCS research, including a comparison of the findings with similar GIAHS landscapes in the region.
5 Conclusion
This study examined the LULC landscapes of dry zone VTCSs in Sri Lanka by using satellite imagery and GIS techniques. The results show that between 1994 and 2021 agricultural and scrub lands consistently increased whereas forests decreased. The declining forest cover was mainly due to the expansion of agricultural areas within the region. The spatial pattern analysis revealed increased fragmentation of the LULC types, indicating the subdivision of the landscape into smaller patches. This change accelerated the fragmentation of forest habitats as the subdivided patches decreased in number, became more isolated and transformed into agricultural and scrub lands over time which weakened the connectivity of ecologically important habitats [141]. Further, fragmentation caused forest edges and core areas to become exposed to external threats, making them increasingly susceptible to anthropogenic disturbances and climate change impacts [145].
The substantial conversion of forests into agricultural lands indicates that future studies should consider a sustainable land management and agriculture strategy in the VTCSs. Robust changes to national land use policy and legislation are required, particularly in the VTCS landscapes integrating an ecological productivity approach. This approach is largely missing from current national land use policy and legislation as well as national research portfolios. Thus, considering the importance of these landscapes as a hotspot of global agricultural and cultural heritage, the study recommends a national strategy for land use and management for VTCSs in Sri Lanka.
The study acknowledges the importance of LULC change detection along with the spatial pattern analysis as useful techniques to estimate LULC change impacts over time to produce realistic results by employing GIS, remote sensing and Google Earth Engine tools that can be adopted for similar tropical SES landscapes. Therefore, this study has provided a detailed assessment of LULC transformations along with spatial metrics analysis and its implication in ecological productivity in the VTCS landscapes where land use issues are becoming a serious threat to the sustainability and productivity of the system. The approach used in this study could be applied as a tool for designing sustainable land management strategies to optimise the overall system productivity of SESs.
Data availability statement
The raw data supporting the conclusion of this article will be made available by the authors, without undue reservation.
Author contributions
SR: Conceptualization, Data curation, Formal Analysis, Methodology, Software, Validation, Writing–original draft, Writing–review and editing. MiR: Resources, Supervision, Writing–review and editing. NL: Supervision, Writing–review and editing. DH: Resources, Writing–review and editing. MaR: Data curation, Formal Analysis, Software, Writing–review and editing. BK: Writing–review and editing. PD: Writing–review and editing. CK: Data curation, Writing–review and editing.
Funding
The author(s) declare that financial support was received for the research, authorship, and/or publication of this article. This study was supported by the Australian Government Research Training Program (RTP) and Destination Australia Program (DAP) scholarships for the PhD study of first author. The authors acknowledge the support of the Global Environment Facility-funded Healthy Landscapes Project for the article publishing charges (APCs).
Conflict of interest
The authors declare that the research was conducted in the absence of any commercial or financial relationships that could be construed as a potential conflict of interest.
Publisher’s note
All claims expressed in this article are solely those of the authors and do not necessarily represent those of their affiliated organizations, or those of the publisher, the editors and the reviewers. Any product that may be evaluated in this article, or claim that may be made by its manufacturer, is not guaranteed or endorsed by the publisher.
Supplementary material
The Supplementary Material for this article can be found online at: https://www.frontiersin.org/articles/10.3389/fenvs.2024.1353459/full#supplementary-material
References
Abeysingha, N., Dassanayake, K., and Weerarathna, C. (2018). Will restoration of ecological functions of tank cascade system contribute to reduce CKDu in Sri Lanka? A review. Environ. Manag. Sustain. Dev. 7, 60–81. doi:10.5296/emsd.v7i3.13129
Allan, A., Soltani, A., Abdi, M. H., and Zarei, M. (2022). Driving forces behind land use and land cover change: a systematic and bibliometric review. Land 11 (8), 1222. doi:10.3390/land11081222
Antwi, E. K. (2009). “Integrating GIS and remote sensing for assessing the impact of disturbance on habitat diversity and land cover change in a post-mining landscape,” in Dept. Of general ecology (Germany: Brandenburg University of Technology in Cottbus: University in Cottbus), 179.
Anuradha, J., Fujimura, M., Inaoka, T., and Sakai, N. (2019). The role of agricultural land use pattern dynamics on elephant habitat depletion and human-elephant conflict in Sri Lanka. Sustainability 11 (10), 2818. doi:10.3390/su11102818
Ariyaratne, W. M. T. P. (2020). “Effect of climate change on rice production and mitigation, in adapting to climate change: a Sri Lankan perspective,” in Institute of biology: colombo, Sri Lanka Editors H. I. U. Caldera, and S. A. C. N. Perera, 85–97.
Arnés García, M., Yagüe, J. L., de Nicolás, V. L., and Díaz-Puente, J. M. (2020). Characterization of globally important agricultural heritage systems (GIAHS) in Europe. Sustainability 12 (4), 1611. doi:10.3390/su12041611
Baral, H., and Holmgren, P. (2015). A framework for measuring sustainability outcomes for landscape investments. Vol. 195. Bogor, Indonesia: CIFOR.
Bebermeier, W., Abeywardana, N., Susarina, M., and Schütt, B. (2023). Domestication of water: management of water resources in the dry zone of Sri Lanka as living cultural heritage. Wiley Interdiscip. Rev. Water 10, e1642. doi:10.1002/wat2.1642
Bebermeier, W., Meister, J., Withanachchi, C., Middelhaufe, I., and Schütt, B. (2017). Tank cascade systems as a sustainable measure of watershed management in South Asia. Water 9 (3), 231. doi:10.3390/w9030231
Berkes, F., Colding, J., and Folke, C. (2008). Navigating social-ecological systems: building resilience for complexity and change. Cambridge University Press.
Boateng, E. Y., Otoo, J., and Abaye, D. A. (2020). Basic tenets of classification algorithms K-nearest-neighbor, support vector machine, random forest and neural network: a review. J. Data Analysis Inf. Process. 8 (4), 341–357. doi:10.4236/jdaip.2020.84020
Borlaug, N. E., et al. (1969). A green revolution yields a golden harvest. Columbia J. World Bus. 4 (3), 9–19.
Brunori, E., Salvati, L., Mancinelli, R., Smiraglia, D., and Biasi, R. (2017). Multi-temporal land use and cover changing analysis: the environmental impact in Mediterranean area. Int. J. Sustain. Dev. World Ecol. 24 (3), 276–288. doi:10.1080/13504509.2016.1205156
Çakmakçı, R., Salık, M. A., and Çakmakçı, S. (2023). Assessment and principles of environmentally sustainable food and agriculture systems. Agriculture 13 (5), 1073. doi:10.3390/agriculture13051073
Chen, B., and Iannone, B. V. (2020). FRAGSTATS: a free tool for quantifying and evaluating spatial patterns. Electron. Data Inf. Syst. (EDIS) Univ. Fla. Inst. Food Agric. Sci. 2020 (6), 9. doi:10.32473/edis-fr431-2020
Chen, H., Yan, W., Li, Z., Wende, W., Xiao, S., Wan, S., et al. (2022). Spatial patterns of associations among ecosystem services across different spatial scales in metropolitan areas: a case study of Shanghai, China. Ecol. Indic. 136, 108682. doi:10.1016/j.ecolind.2022.108682
Chen, S., Olofsson, P., Saphangthong, T., and Woodcock, C. E. (2023b). Monitoring shifting cultivation in Laos with Landsat time series. Remote Sens. Environ. 288, 113507. doi:10.1016/j.rse.2023.113507
Chen, S., Woodcock, C. E., Saphangthong, T., and Olofsson, P. (2023a). Satellite data reveals a recent increase in shifting cultivation and associated carbon emissions in Laos. Environ. Res. Lett. 18 (11), 114012. doi:10.1088/1748-9326/acffdd
Csorba, P., and Szabó, S. (2012). “The application of landscape indices in landscape ecology,” in Perspectives on nature conservation-Patterns, pressures and prospects (Rijeka: InTech), 121–140.
Dale, V. H. (1997). The relationship between land-use change and climate change. Ecol. Appl. 7 (3), 753–769. doi:10.1890/1051-0761(1997)007[0753:trbluc]2.0.co;2
Dash, C. J., et al. (2021). “Quantifying forest cover changes in Koraput district, India using remote sensing and GIS,” in Forest resources resilience and conflicts (Elsevier), 185–191.
de la Riva, E. G., Ulrich, W., Batáry, P., Baudry, J., Beaumelle, L., Bucher, R., et al. (2023). From functional diversity to human well-being: a conceptual framework for agroecosystem sustainability. Agric. Syst. 208, 103659. doi:10.1016/j.agsy.2023.103659
Dharmasena, P. (1992). “Soil erodibility and rainfall erosivity in the dry zone of Sri Lanka: chapter 5- Soil erosion control measures for rain-fed farming in the dry zone of Sri Lanka,” in Department of geography (Sri Lanka: University of Peradeniya: Peradeniya), 279.
Dharmasena, P. (1994). Conservation farming practices for small reservoir watersheds: a case study from Sri Lanka. Agrofor. Syst. 28, 203–212. doi:10.1007/bf00704756
Dharmasena, P. (2004). “Small tank heritage and current problems,” in Proceedings of the Symposium on small tank Settlements in Sri Lanka (Colombo, Sri Lanka: Hectorkobbekaduwa Agrarian Research and Training Institute HARTI, Sri Lanka).
Dharmasena, P. (2010). “Evolution of hydraulic societies in the ancient Anuradhapura Kingdom of Sri Lanka,” in Landscapes and societies Editors I. P. Martini, and W. Chesworth (Dordrecht, The Netherlands: Springer Dordrecht), 341–352.
Dharmasena, P. (2020). “Cascaded tank-village system: present status and prospects,” in Agricultural research for sustainable food systems in Sri Lanka Editors B. Marambe, J. Weerahewa, and W. S. Dandeniya (Singapore: Springer), 63–75.
Dissanayake, D., Morimoto, T., Murayama, Y., Ranagalage, M., and Perera, E. (2020). Analysis of life quality in a tropical mountain city using a multi-criteria geospatial technique: a case study of Kandy City, Sri Lanka. Sustainability 12 (7), 2918. doi:10.3390/su12072918
Dissanayake, S. T., and Vidanage, S. (2023). Valuing ecosystem services from restoring ancient irrigation systems: an application comparing labor vs. monetary payments for choice experiments. Agric. Resour. Econ. Rev. 52 (2), 422–449. doi:10.1017/age.2023.24
Duelli, P. (1997). Biodiversity evaluation in agricultural landscapes: an approach at two different scales. Agric. Ecosyst. Environ. 62 (2-3), 81–91. doi:10.1016/s0167-8809(96)01143-7
Echeverría, C., Coomes, D., Salas, J., Rey-Benayas, J. M., Lara, A., and Newton, A. (2006). Rapid deforestation and fragmentation of Chilean temperate forests. Biol. Conserv. 130 (4), 481–494. doi:10.1016/j.biocon.2006.01.017
Elsen, P. R., Oakes, L. E., Cross, M. S., DeGemmis, A., Watson, J. E., Cooke, H. A., et al. (2023). Priorities for embedding ecological integrity in climate adaptation policy and practice. One Earth 6 (6), 632–644. doi:10.1016/j.oneear.2023.05.014
Estoque, R. C., and Murayama, Y. (2017). Monitoring surface urban heat island formation in a tropical mountain city using Landsat data (1987–2015). ISPRS J. Photogrammetry Remote Sens. 133, 18–29. doi:10.1016/j.isprsjprs.2017.09.008
Estrada-Carmona, N., Sánchez, A. C., Remans, R., and Jones, S. K. (2022). Complex agricultural landscapes host more biodiversity than simple ones: a global meta-analysis. Proc. Natl. Acad. Sci. 119 (38), e2203385119. doi:10.1073/pnas.2203385119
Ewane, E. B. (2021). Land use land cover change and the resilience of social-ecological systems in a sub-region in South west Cameroon. Environ. Monit. Assess. 193 (6), 338. doi:10.1007/s10661-021-09077-z
FAO (2021). Globally important agricultural heritage systems (GIAHS). Available at: http://www.fao.org/giahs/en/ (Accessed January 25, 2021).
Flowers, B., Huang, K.-T., and Aldana, G. O. (2020). Analysis of the habitat fragmentation of ecosystems in Belize using landscape metrics. Sustainability 12 (7), 3024. doi:10.3390/su12073024
Foody, G. M. (2008). Harshness in image classification accuracy assessment. Int. J. Remote Sens. 29 (11), 3137–3158. doi:10.1080/01431160701442120
Fu, G., Wang, W., Li, J., Xiao, N., and Qi, Y. (2021). Prediction and selection of appropriate landscape metrics and optimal scale ranges based on multi-scale interaction analysis. Land 10 (11), 1192. doi:10.3390/land10111192
Gong, Y., You, G., Chen, T., Wang, L., and Hu, Y. (2023). Rural landscape change: the driving forces of land use transformation from 1980 to 2020 in Southern Henan, China. Sustainability 15 (3), 2565. doi:10.3390/su15032565
Haines-Young, R., and Potschin, M., (2010). The links between biodiversity, ecosystem services and human well-being. Ecosystem Ecology: a new synthesis, 1, 110–139.
Hansen, A. J., Noble, B. P., Veneros, J., East, A., Goetz, S. J., Supples, C., et al. (2021). Toward monitoring forest ecosystem integrity within the post-2020 Global Biodiversity Framework. Conserv. Lett. 14 (4), e12822. doi:10.1111/conl.12822
Horodnic, V.-D., et al. (2019). Assessment of land use changes and landscape fragmentation in the tourism area of Cacica and Vatra Dornei. Georeview Sci. Ann. Stefan cel Mare Univ. Suceava. Geogr. Ser. 29 (1), 12–30.
Hou, L., Wu, F., and Xie, X. (2020). The spatial characteristics and relationships between landscape pattern and ecosystem service value along an urban-rural gradient in Xi’an city, China. Ecol. Indic. 108, 105720. doi:10.1016/j.ecolind.2019.105720
Ichikawa, K. (2012). Socio-ecological production landscapes in asia. Japan: Tokyo, Japan: United Nations University Institute of Advanced Studies UNU-IAS, 106.
Ickowitz, A. (2006). Shifting cultivation and deforestation in tropical Africa: critical reflections. Dev. change 37 (3), 599–626. doi:10.1111/j.0012-155x.2006.00492.x
IPBES (2018). “The IPBES regional assessment report on biodiversity and ecosystem services for asia and the pacific: summary for policymakers,” in Secretariat of the intergovernmental science-policy platform on biodiversity and ecosystem services: secretariat of the intergovernmental science-policy platform on biodiversity and ecosystem services (IPBES), bonn, Germany Editor M. Karki, 620.
IPCC (2019). “Summary for policymakers,” in Climate change and land: an IPCC special report on climate change, desertification, land degradation, sustainable land management, food security, and greenhouse gas fluxes in terrestrial ecosystems (Cambridge, UK and New York, NY, USA: Cambridge University Press), 3–36.
Kadupitiya, H. K., Madushan, R. N. D., Gunawardhane, D., Sirisena, D., Rathnayake, U., Dissanayaka, D., et al. (2022). Mapping productivity-related spatial characteristics in rice-based cropping systems in Sri Lanka. J. Geovisualization Spatial Analysis 6 (2), 26–10. doi:10.1007/s41651-022-00122-0
Karr, J. R. (1996). “Ecological integrity and ecological health are not the same,” in Engineering within ecological constraints Editor P. C. Schulz (Washington, DC: The National Academy Press), 97–109.
Kindu, M., Schneider, T., Döllerer, M., Teketay, D., and Knoke, T. (2018). Scenario modelling of land use/land cover changes in Munessa-Shashemene landscape of the Ethiopian highlands. Sci. Total Environ. 622, 534–546. doi:10.1016/j.scitotenv.2017.11.338
Kogo, B. K., Kumar, L., and Koech, R. (2021). Analysis of spatio-temporal dynamics of land use and cover changes in Western Kenya. Geocarto Int. 36 (4), 376–391. doi:10.1080/10106049.2019.1608594
Kuule, D. A., Ssentongo, B., Magaya, P. J., Mwesigwa, G. Y., Okurut, I. T., Nyombi, K., et al. (2022). Land use and land cover change dynamics and perceived drivers in rangeland areas in Central Uganda. Land 11 (9), 1402. doi:10.3390/land11091402
Lasanthika, K. A. G. A., and Vitharana, U. W. A. (2021). “Land use nad land cover change in the Kapriggama Tank Cascade System during 2010 to 2020,” in Proceedings of cascade ecology and management (Sri Lanka: University of Peradeniya Sri Lanka: Faculty of Agriculture, University of Peradeniya).
Leadley, P., Archer, E., Bendandi, B., Cavender-Bares, J., Davalos, L., DeClerck, F., et al. (2022). Setting ambitious international restoration objectives for terrestrial ecosystems for 2030 and beyond. PLOS Sustain. Transformation 1 (12), e0000039. doi:10.1371/journal.pstr.0000039
Legarreta-Miranda, C. K., Prieto-Amparán, J. A., Villarreal-Guerrero, F., Morales-Nieto, C. R., and Pinedo-Alvarez, A. (2021). Long-term land-use/land-cover change increased the landscape heterogeneity of a fragmented temperate forest in Mexico. Forests 12 (8), 1099. doi:10.3390/f12081099
Lei, D., Zhang, Y., Ran, Y., Gao, L., Li, J., Li, Z., et al. (2023). Assessment of ecosystem health based on landscape pattern in ecologically fragile regions at different spatial scales: a case study of Dianchi Lake basin, China. Front. Environ. Sci. 11, 1076344. doi:10.3389/fenvs.2023.1076344
Levers, C., Romero-Muñoz, A., Baumann, M., De Marzo, T., Fernández, P. D., Gasparri, N. I., et al. (2021). Agricultural expansion and the ecological marginalization of forest-dependent people. Proc. Natl. Acad. Sci. 118 (44), e2100436118. doi:10.1073/pnas.2100436118
Li, M., Li, X., Liu, S., Lyu, X., Dang, D., Dou, H., et al. (2022). Analysis of the spatiotemporal variation of landscape patterns and their driving factors in inner Mongolia from 2000 to 2015. Land 11 (9), 1410. doi:10.3390/land11091410
Liu, Z., Chen, J., Qi, J., John, R., Cheng, J., and Zhu, Z. (2016). The effect of landscape composition on the abundance of Laodelphax striatellus Fallén in fragmented agricultural landscapes. Land 5 (4), 36. doi:10.3390/land5040036
Lu, D., Mausel, P., Brondízio, E., and Moran, E. (2004). Change detection techniques. Int. J. remote Sens. 25 (12), 2365–2401. doi:10.1080/0143116031000139863
Lugoi, L. P., Bamutaze, Y., Martinsen, V., and Rossebø Almås, Å. (2023). Climate change hotpots and their implications on rain-fed cropping system in a tropical environment. Appl. Geogr. 154, 102953. doi:10.1016/j.apgeog.2023.102953
Lyu, R., Zhao, W., Tian, X., and Zhang, J. (2022). Non-linearity impacts of landscape pattern on ecosystem services and their trade-offs: a case study in the City Belt along the Yellow River in Ningxia, China. Ecol. Indic. 136, 108608. doi:10.1016/j.ecolind.2022.108608
Mancinelli, R., Di Felice, V., Karkalis, K., Bari, S., Radicetti, E., and Campiglia, E. (2018). Assessment of the state of agroecosystem sustainability using landscape indicators: a comparative study of three rural areas in Greece. Int. J. Sustain. Dev. World Ecol. 25 (1), 35–46. doi:10.1080/13504509.2016.1277377
McGarigal, K. (1995). FRAGSTATS: spatial pattern analysis program for quantifying landscape structure. Vol. 351. Portland, USA: US Department of Agriculture, Forest Service, Pacific Northwest Research Station.
McGarigal, K. (2017). “Landscape metrics for categorical map patterns,” in Lecture notes. Available at: http://po.licka.cz/_media/chapter9_metrics.pdf (Accessed June 22, 2023).
McGarigal, K., Cushman, S. A., and Ene, E. (2023). Spatial pattern analysis program for categorical maps. Computer software program produced by the authors. Available at: https://www.fragstats.org (Accessed June 15, 2023).
Mekuria, W., Diyasa, M., Tengberg, A., and Haileslassie, A. (2021). Effects of long-term land use and land cover changes on ecosystem service values: an example from the central rift valley, Ethiopia. Land 10 (12), 1373. doi:10.3390/land10121373
Misana, S. B., Sokoni, C., and Mbonile, M. J. (2012). Land-use/cover changes and their drivers on the slopes of Mount Kilimanjaro, Tanzania. J. Geogr. Regional Plan. 5 (8), 151. doi:10.5897/jgrp11.050
Mitchell, N. J., and Barrett, B. (2017). “Exploring agricultural heritage landscapes: a journey across" terra incognita,” in The george wright forum (JSTOR).
Murcia, C. (1995). Edge effects in fragmented forests: implications for conservation. Trends Ecol. Evol. 10 (2), 58–62. doi:10.1016/s0169-5347(00)88977-6
Nayakekorala, H. B. (2010). “Traditional practices of soil management in agriculture in Sri Lanka,” in Economic review (Colombo, Sri Lanka: People's Bank, Sri Lanka), 37–39.
Nianthi, R. (2011). “Drought risk reduction in the dry zone of Sri Lanka,” in Droughts in asian monsoon region Editors R. Shaw, and H. Nguyen (Bingley, UK: Emerald Group Publishing Limited), 97–120.
Nyatuame, M., Agodzo, S., Amekudzi, L. K., and Mensah-Brako, B. (2023). Assessment of past and future land use/cover change over Tordzie watershed in Ghana. Front. Environ. Sci. 11, 1139264. doi:10.3389/fenvs.2023.1139264
O’Donnell, A., et al. (2018). Shadows of conflict in northern and eastern Sri Lanka: socioeconomic challenges and a way forward. World Bank Publications.
Oliver, T. H., and Morecroft, M. D. (2014). Interactions between climate change and land use change on biodiversity: attribution problems, risks, and opportunities. Wiley Interdiscip. Rev. Clim. Change 5 (3), 317–335. doi:10.1002/wcc.271
Ostrom, E. (2009). A general framework for analyzing sustainability of social-ecological systems. Science 325 (5939), 419–422. doi:10.1126/science.1172133
Panabokke, C. R. (1996). Soils and agro-ecological Environments of Sri Lanka (natural resources series No.2). Colombo, Sri Lanka: Natural Resources, Energy and Science Authority of Sri Lanka NARESA, 220.
Panabokke, C. R., Sakthivadivel, R., and Weerasinghe, A. D. (2002). Evolution, present status and issues concerning small tank systems in Sri Lanka. Battaramulla, Sri Lanka: International Water Management Institute IWMI.
Paranage, K. (2018). Understanding the relationship between water infrastructure and socio-political configurations: a case study from Sri Lanka. Water 10 (10), 1402. doi:10.3390/w10101402
Parisi, M. D., Huber, P. R., and Greco, S. E. (2023). Assessing conservation outcomes and maximizing habitat connectivity for multiple species in systematic conservation plans: a case study in Yolo County, California. Landsc. Ecol. 38, 1621–1642. doi:10.1007/s10980-023-01664-4
Parresol, B. R., and Edwards, L. A. (2014). An entropy-based contagion index and its sampling properties for landscape analysis. Entropy 16 (4), 1842–1859. doi:10.3390/e16041842
Parvin, I., Islam, M. A., Perveen, M. F., Jamal, M. R., Faruque, M. J., Hossen, B., et al. (2024). Assessment of land use transition and crop intensification using geospatial technology in Bangladesh. Case Stud. Chem. Environ. Eng. 9, 100660. doi:10.1016/j.cscee.2024.100660
Paz, D. B., Henderson, K., and Loreau, M. (2020). Agricultural land use and the sustainability of social-ecological systems. Ecol. Model. 437, 109312. doi:10.1016/j.ecolmodel.2020.109312
Piras, F., and Santoro, A. (2023). Land use changes in globally important cultural forests. The case of two traditionally managed forests for non-wood forest products (NWFPs) in China and Japan. Biodivers. Conservation, 1–23. doi:10.1007/s10531-023-02690-z
Priyankara, P., Ranagalage, M., Dissanayake, D., Morimoto, T., and Murayama, Y. (2019). Spatial process of surface urban heat island in rapidly growing Seoul metropolitan area for sustainable urban planning using Landsat data (1996–2017). Climate 7 (9), 110. doi:10.3390/cli7090110
Punyawardena, B. V. R. (2008). Rainfall of Sri Lanka and agroecological zones. Peradeniaya, Sri Lanka: Department of Agriculture.
Qin, F., Fukamachi, K., and Shibata, S. (2022). Land-use/landscape pattern changes and related environmental driving forces in a Dong Ethnic Minority Village in Southwestern China. Land 11 (3), 349. doi:10.3390/land11030349
Ramalingam, B. (2013). From bali, with complexity. Aid Edge Chaos Rethink. Int. Coop. a Complex World, 154–196.
Ranagalage, M., Gunarathna, M. H. J. P., Surasinghe, T. D., Dissanayake, D., Simwanda, M., Murayama, Y., et al. (2020). Multi-decadal forest-cover dynamics in the tropical realm: past trends and policy insights for forest conservation in dry zone of Sri Lanka. Forests 11 (8), 836. doi:10.3390/f11080836
Ranagalage, M., Wang, R., Gunarathna, M. H. J. P., Dissanayake, D. M. S. L. B., Murayama, Y., and Simwanda, M. (2019). Spatial forecasting of the landscape in rapidly urbanizing hill stations of South Asia: a case study of Nuwara Eliya, Sri Lanka (1996–2037). Remote Sens. 11 (15), 1743. doi:10.3390/rs11151743
Rathnayake, C. W., Jones, S., and Soto-Berelov, M. (2020). Mapping land cover change over a 25-year period (1993–2018) in Sri Lanka using Landsat time-series. Land 9 (1), 27. doi:10.3390/land9010027
Ratnayake, S. S., Khan, A., Reid, M., Dharmasena, P. B., Hunter, D., Kumar, L., et al. (2022). Land use-based participatory assessment of ecosystem services for ecological restoration in village tank cascade systems of Sri Lanka. Sustainability 14 (16), 10180. doi:10.3390/su141610180
Ratnayake, S. S., Kumar, L., Dharmasena, P. B., Kadupitiya, H. K., Kariyawasam, C. S., and Hunter, D. (2021). Sustainability of village tank cascade systems of Sri Lanka: exploring cascade anatomy and socio-ecological nexus for ecological restoration planning. Challenges 12 (2), 24. doi:10.3390/challe12020024
Ratnayake, S. S., Reid, M., Larder, N., Kadupitiya, H. K., Hunter, D., Dharmasena, P. B., et al. (2023). Impact of climate change on paddy farming in the village tank cascade systems of Sri Lanka. Sustainability 15 (12), 9271. doi:10.3390/su15129271
Ratnayake, S. S., Reid, M., Larder, N., Kariyawasam, C. S., Hunter, C., Hunter, D., et al. (2024). Sustainability and productivity of village tank cascade systems: a bibliometric analysis and knowledge mapping. Sustainability 16 (8), 3360. doi:10.3390/su16083360
Redlands, C. E. S. R. I. (2011). ArcGIS desktop: release 10. Redlands, California, USA: Environmental Systems Research Institute.
Ren, J., Yang, J., Wu, F., Sun, W., Xiao, X., and Xia, J. C. (2023). Regional thermal environment changes: integration of satellite data and land use/land cover. Iscience 26 (2), 105820. doi:10.1016/j.isci.2022.105820
Reyers, B., Folke, C., Moore, M. L., Biggs, R., and Galaz, V. (2018). Social-ecological systems insights for navigating the dynamics of the Anthropocene. Annu. Rev. Environ. Resour. 43, 267–289. doi:10.1146/annurev-environ-110615-085349
Rogers, B. M., Mackey, B., Shestakova, T. A., Keith, H., Young, V., Kormos, C. F., et al. (2022). Using ecosystem integrity to maximize climate mitigation and minimize risk in international forest policy. Front. For. Glob. Change 5, 929281. doi:10.3389/ffgc.2022.929281
Ryser, R., Hirt, M. R., Häussler, J., Gravel, D., and Brose, U. (2021). Landscape heterogeneity buffers biodiversity of simulated meta-food-webs under global change through rescue and drainage effects. Nat. Commun. 12 (1), 4716. doi:10.1038/s41467-021-24877-0
Saito, O., et al. (2020). Managing socio-ecological production landscapes and seascapes for sustainable communities in asia: mapping and navigating stakeholders, policy and action. Springer Nature.
Santoro, A., Venturi, M., Bertani, R., and Agnoletti, M. (2020). A review of the role of forests and agroforestry systems in the FAO Globally Important Agricultural Heritage Systems (GIAHS) programme. Forests 11 (8), 860. doi:10.3390/f11080860
Sarif, M. O., Ranagalage, M., Gupta, R. D., and Murayama, Y. (2022). Monitoring urbanization induced surface urban cool island formation in a South Asian megacity: a case study of Bengaluru, India (1989–2019). Front. Ecol. Evol. 10, 901156. doi:10.3389/fevo.2022.901156
Sarvananthan, M. (2003). Economic revival in north and east Sri Lanka: what are the impediments? Econ. Political Wkly., 1844–1850.
Saunders, S. P., Meehan, T. D., Michel, N. L., Bateman, B. L., DeLuca, W., Deppe, J. L., et al. (2022). Unraveling a century of global change impacts on winter bird distributions in the eastern United States. Glob. change Biol. 28 (7), 2221–2235. doi:10.1111/gcb.16063
Schößer, B., Helming, K., and Wiggering, H. (2010). Assessing land use change impacts–a comparison of the SENSOR land use function approach with other frameworks. J. Land Use Sci. 5 (2), 159–178. doi:10.1080/1747423x.2010.485727
Schulp, C. J., Levers, C., Kuemmerle, T., Tieskens, K. F., and Verburg, P. H. (2019). Mapping and modelling past and future land use change in Europe’s cultural landscapes. Land use policy 80, 332–344. doi:10.1016/j.landusepol.2018.04.030
Schweiger, A. K., Schütz, M., Anderwald, P., Schaepman, M. E., Kneubühler, M., Haller, R., et al. (2015). Foraging ecology of three sympatric ungulate species–behavioural and resource maps indicate differences between chamois, ibex and red deer. Mov. Ecol. 3 (1), 6–12. doi:10.1186/s40462-015-0033-x
Shao, G., Iannone, B. V., and Fei, S. (2018). Enhanced forest interior estimations utilizing lidar-assisted 3D forest cover map. Ecol. Indic. 93, 1236–1243. doi:10.1016/j.ecolind.2018.06.037
Shiferaw, A., and Singh, K. (2011). Evaluating the land use and land cover dynamics in borena woreda South wollo highlands, Ethiopia. Ethiop. J. Bus. Econ. (The).).
Simwanda, M., and Murayama, Y. (2017). Integrating geospatial techniques for urban land use classification in the developing sub-Saharan African city of Lusaka, Zambia. ISPRS Int. J. Geo-Information 6 (4), 102. doi:10.3390/ijgi6040102
Singh, S. K., Srivastava, P. K., Szabó, S., Petropoulos, G. P., Gupta, M., and Islam, T. (2017). Landscape transform and spatial metrics for mapping spatiotemporal land cover dynamics using Earth Observation data-sets. Geocarto Int. 32 (2), 1–15. doi:10.1080/10106049.2015.1130084
Sirimanna, S., Kahathuduwa, K., and Prasada, D. (2022). Are cascade reservoir systems sustainable agroecosystems? A comparative assessment of efficiency, effectiveness and resource footprint in a Sri Lankan micro-cascade. Agric. Syst. 203, 103493. doi:10.1016/j.agsy.2022.103493
Smith, P., et al. (2014). “Agriculture, forestry and other land use (AFOLU),” in Climate change 2014: mitigation of climate change. Contribution of working group III to the fifth assessment report of the intergovernmental panel on climate change (Cambridge University Press), 811–922.
Tadese, M., Kumar, L., Koech, R., and Kogo, B. K. (2020). Mapping of land-use/land-cover changes and its dynamics in Awash River Basin using remote sensing and GIS. Remote Sens. Appl. Soc. Environ. 19, 100352. doi:10.1016/j.rsase.2020.100352
Team, R. D. C. R. (2011). A language and environment for statistical computing. Available at: https://www.r-project.org/ (Accessed July 7, 2023).
Thakrar, S. K., Johnson, J. A., and Polasky, S. (2023). Land-use decisions have substantial air quality health effects. Environ. Sci. Technol. 58 (1), 381–390. doi:10.1021/acs.est.3c02280
Tikuye, B. G., Rusnak, M., Manjunatha, B. R., and Jose, J. (2023). Land use and land cover change detection using the random forest approach: the case of the upper blue nile river basin, Ethiopia. Glob. Challenges 7 (10), 2300155. doi:10.1002/gch2.202300155
Tobias, J. A., Durant, S. M., and Pettorelli, N. (2021). Improving predictions of climate change–land use change interactions. Trends Ecol. Evol. 36 (1), 29–38. doi:10.1016/j.tree.2020.08.019
Tolessa, T., Senbeta, F., and Kidane, M. (2016). Landscape composition and configuration in the central highlands of Ethiopia. Ecol. Evol. 6 (20), 7409–7421. doi:10.1002/ece3.2477
Toma, M. B., Belete, M. D., and Ulsido, M. D. (2023). Historical and future dynamics of land use land cover and its drivers in Ajora-Woybo watershed, Omo-Gibe basin, Ethiopia. Nat. Resour. Model. 36 (1), e12353. doi:10.1111/nrm.12353
Tsarouchi, G., and Buytaert, W. (2018). Land-use change may exacerbate climate change impacts on water resources in the Ganges basin. Hydrology Earth Syst. Sci. 22 (2), 1411–1435. doi:10.5194/hess-22-1411-2018
Ul Din, S., and Mak, H. W. L. (2021). Retrieval of land-use/land cover change (LUCC) maps and urban expansion dynamics of Hyderabad, Pakistan via Landsat datasets and support vector machine framework. Remote Sens. 13 (16), 3337. doi:10.3390/rs13163337
Vidanage, S. P., Kotagama, H. B., and Dunusinghe, P. M. (2022). “Sri Lanka’s small tank cascade systems: building agricultural resilience in the dry zone,” in Climate change and community resilience (Singapore: Springer), 225–235.
Walker, B., and Meyers, J. A. (2004). Thresholds in ecological and Social&#8211;Ecological systems: a developing database. Ecol. Soc. 9 (2), art3. doi:10.5751/es-00664-090203
WallisDeVries, M. F. (1996). Effects of resource distribution patterns on ungulate foraging behaviour: a modelling approach. For. Ecol. Manag. 88 (1-2), 167–177. doi:10.1016/s0378-1127(96)03822-4
Wang, Q., Xiong, K., Zhou, J., Xiao, H., and Song, S. (2023b). Impact of land use and land cover change on the landscape pattern and service value of the village ecosystem in the karst desertification control. Front. Environ. Sci. 11, 1020331. doi:10.3389/fenvs.2023.1020331
Wang, S., Zuo, Q., Zhou, K., Wang, J., and Wang, W. (2023a). Predictions of land use/land cover change and landscape pattern analysis in the lower reaches of the tarim river, China. Land 12 (5), 1093. doi:10.3390/land12051093
Wang, X., Blanchet, F. G., and Koper, N. (2014). Measuring habitat fragmentation: an evaluation of landscape pattern metrics. Methods Ecol. Evol. 5 (7), 634–646. doi:10.1111/2041-210x.12198
Wickramasinghe, M. R. C. P., et al. (2021). “Impact of human interventions on the ancient village tank cascade systems: a case study of mahakanumulla cascade system in Sri Lanka,” in Proceedings of the cascade ecology & management (CEM) conference (Sri Lanka: University of Peradeniya Sri Lanka: Faculty of Agriculture, University of Peradeniya).
Wickramasinghe, M. R. C. P., et al. (2023). A study on external pressures of an ancient irrigation cascade system in Sri Lanka. Agric. Syst., 205.
World Bank and CIAT (2015). Climate-smart agriculture in Sri Lanka. Csa country profiles for africa, asia, and Latin America and the caribbean series. Washington DC, USA: The World Bank Group, 16.
Yanying, B., Xueping, S., Mi, T., and Fuller, A. M. (2014). Typical water-land utilization GIAHS in low-lying areas: the Xinghua duotian agrosystem example in China. J. Resour. Ecol. 5 (4), 320–327. doi:10.5814/j.issn.1674-764x.2014.04.006
Yesuph, A. Y., and Dagnew, A. B. (2019). Land use/cover spatiotemporal dynamics, driving forces and implications at the Beshillo catchment of the Blue Nile Basin, North Eastern Highlands of Ethiopia. Environ. Syst. Res. 8 (1), 21–30. doi:10.1186/s40068-019-0148-y
Yu, H., Verburg, P. H., Liu, L., and Eitelberg, D. A. (2016). Spatial analysis of cultural heritage landscapes in rural China: land use change and its risks for conservation. Environ. Manag. 57, 1304–1318. doi:10.1007/s00267-016-0683-5
Yuh, Y. G., Tracz, W., Matthews, H. D., and Turner, S. E. (2023). Application of machine learning approaches for land cover monitoring in northern Cameroon. Ecol. Inf. 74, 101955. doi:10.1016/j.ecoinf.2022.101955
Zhan, J. (2023). The sustainable management of land systems. Front. Sustain. Resour. Manag. 2, 1240771. doi:10.3389/fsrma.2023.1240771
Zhang, D., Zhou, C., and He, B.-J. (2022). Spatial and temporal heterogeneity of urban land area and PM2. 5 concentration in China. Urban Clim. 45, 101268. doi:10.1016/j.uclim.2022.101268
Zhang, X., Dong, X., Liu, F., Lv, T., Wu, Z., and Ranagalage, M. (2023b). Spatiotemporal dynamics of ecological security in a typical conservation region of southern China based on catastrophe theory and GIS. Environ. Monit. Assess. 195 (1), 90. doi:10.1007/s10661-022-10669-6
Zhang, X., Dong, X., Xu, C., Wang, R., Lv, T., and Ranagalage, M. (2023a). Landscape ecological risk assessment in the Dongjiangyuan region, China, from 1985 to 2020 using geospatial techniques. Geomatics, Nat. Hazards Risk 14 (1), 2173662. doi:10.1080/19475705.2023.2173662
Keywords: land use and cover change, landscape fragmentation, landscape composition and configuration, ecological integrity, sustainable land management, social-ecological systems
Citation: Ratnayake SS, Reid M, Larder N, Hunter D, Ranagalage M, Kogo B, Dharmasena PB and Kariyawasam CS (2024) Knowing the lay of the land: changes to land use and cover and landscape pattern in village tank cascade systems of Sri Lanka. Front. Environ. Sci. 12:1353459. doi: 10.3389/fenvs.2024.1353459
Received: 10 December 2023; Accepted: 13 May 2024;
Published: 24 June 2024.
Edited by:
Hugo Wai Leung MAK, Hong Kong University of Science and Technology, Hong Kong SAR, ChinaReviewed by:
Antonio Santoro, University of Florence, ItalyHuiqing Han, Guizhou Institute of Technology, China
Copyright © 2024 Ratnayake, Reid, Larder, Hunter, Ranagalage, Kogo, Dharmasena and Kariyawasam. This is an open-access article distributed under the terms of the Creative Commons Attribution License (CC BY). The use, distribution or reproduction in other forums is permitted, provided the original author(s) and the copyright owner(s) are credited and that the original publication in this journal is cited, in accordance with accepted academic practice. No use, distribution or reproduction is permitted which does not comply with these terms.
*Correspondence: Sujith S. Ratnayake, rratnay2@myune.edu.au