- 1Business School, Hohai University, Nanjing, China
- 2International Economic & Technical Cooperation and Exchange Center, Ministry of Water Resources, Beijing, China
- 3College of Geography and Environment, Henan University, Zhengzhou, China
- 4Institute of Environment and Sustainable Development in Agriculture, Chinese Academy of Agricultural Sciences, Beijing, China
- 5Shanghai Institute of Satellite Engineering, Shanghai, China
This study addresses the critical problem of understanding the changing dynamics of glacier meltwater in Lake Mertzbakher, a challenge heightened by ongoing global climate change. Employing the innovative method of the Google Earth Engine (GEE) platform, this research meticulously extracted surface water data at 60 time points during the years 2000, 2005, 2010, 2015, and 2021. This approach represents a significant advancement over previous methods by offering more frequent and precise data analysis. We incorporated meteorological factors such as temperature and precipitation to assess their influence on the monthly changes in the glacier lake area. Our findings indicate a pronounced outburst in July, leading to a substantial decrease in the lake’s area, which reaches its lowest in September. Through detailed partial regression analysis, we established a hierarchy of meteorological influences on the lake’s area, identifying minimum temperature (r = 0.245), mean temperature (r = −0.239), precipitation (r = 0.228), radiation (r = 0.154), and maximum temperature (r = 0.128) as key factors. Additionally, our use of a structural equation model unveiled the most impactful elements, with mean temperature (r = −3.320), minimum temperature (r = 2.870), radiation (r = 0.480), and precipitation (r = 0.470) leading the effects. These insights mark a substantial contribution to our understanding of glacier lake dynamics, offering crucial data for predicting and managing glacier lake floods. This study’s novel methodology and comprehensive analysis underscore its significance in enhancing disaster prevention and preparedness strategies amidst the challenges of global climate change.
1 Introduction
Lake Mertzbakher, located in Kyrgyzstan near the upper reaches of the Kunmalike River, a tributary of the Aksu River in China, is situated approximately 100 km from the Sino-Kyrgyz border. Between 1932 and 2011, Lake Mertzbakher has experienced 65 floods, demonstrating a frequency exceeding 92.5% (Pekel J. et al., 2016; Donchyts et al., 2016; Zheng et al., 2019; Araujo et al., 2021; Chen et al., 2021). The escalation in global temperatures is anticipated to further increase the frequency of extreme climate events, consequently intensifying the glacier meltwater recharge. This increase in meltwater influx is poised to augment the water volume of the glacier lake, thereby reducing its stability (Pekel J. et al., 2016; Donchyts et al., 2016; Zheng et al., 2019; Araujo et al., 2021; Chen et al., 2021). The understanding of glacier meltwater dynamics in Lake Mertzbakher is of paramount importance, given its susceptibility to the impacts of global climate change. The accelerated melting of glaciers in this region due to rising temperatures leads to a substantial increase in meltwater flow into the lake, potentially altering the lake’s water level, stability, and frequency of outburst floods. These outburst floods from Lake Mertzbakher pose a significant threat to the flood control safety and the security of life and property in the Aksu region in China. The Aksu River, accounting for approximately 73% of the total runoff of the Tarim River, is crucial for the economic, social development, and ecological protection of the Tarim River Basin in China (Zhou et al., 2021; Wu et al., 2022). Understanding the dynamics of Lake Mertzbakher’s meltwater outbursts is therefore critical for both China and Kyrgyzstan. Changes in the glacier meltwater dynamics directly influence the water resources of the Aksu-Kashgar River system and the Tarim River, which are vital for the region’s development and environmental conservation (Zhou et al., 2021; Wu et al., 2022).
Remote sensing technology presents a viable solution to observe and monitor the area changes of Lake Mertzbakher, particularly given its remote location and difficult accessibility. Presently, there are two main water body extraction algorithms based on remote sensing data: the traditional algorithm, including the water index and threshold method, and the machine learning classification algorithm (Chen et al., 2019; Li et al., 2019; Zhou and Dong, 2019). The accuracy of these algorithms in extracting water body information varies, also influenced by the data used. Consequently, combining different algorithms and data results in water body extraction methods with varying degrees of accuracy (Palmer et al., 2015; Li et al., 2020a; Khandelwal et al., 2022; Zhang et al., 2022; Guo et al., 2023). Scholars like Yan Zhou, Jinwei Dong, et al. (Zhou and Dong, 2019) have provided a systematic and comprehensive analysis and comparative discussion of these methods, classifying current machine learning algorithms into three types: Support Vector Machine (SVM), Random Forest (RF), and Deep Learning (DL). Researchers such as Shuhui Zhao et al. (Palmer et al., 2015) and Xufeng Wei et al. (Zhao et al., 2018) have applied these methods to extract water bodies from various lakes, demonstrating the applicability and effectiveness of these techniques. In the era of big data, the study of surface water changes has shifted from regional-scale studies based on local computing to global-scale studies utilizing high-performance cloud computing platforms, such as Google Earth Engine (GEE). This platform offers several advantages, including low manual participation, high automation, high classification accuracy, and efficiency, making it highly suitable for remote sensing applications (Hansen et al., 2013; Pekel J. F. et al., 2016; Zou et al., 2017; Zou et al., 2018; Zhou et al., 2019; Wang et al., 2020; Wei et al., 2020; Zhou et al., 2022). Notable research efforts using GEE include global forest change maps by Hansen et al. (Wang et al., 2020) and global surface water coverage data sets by Pekel et al. (Hansen et al., 2013), exemplifying the platform’s utility in environmental monitoring. Besides, glacier lake outburst floods, caused by rapid drainage of glacier lakes or dam collapses, are a focus of ongoing research. Scholars have begun analyzing changes in glacier lake areas, with studies like those by Xin Wang et al. (Qi et al.) and Changxian Qi et al. (Pekel J. F. et al., 2016) highlighting the influence of glacier recession and increased meltwater due to warming as significant factors contributing to the expansion of glacier lake areas. The Mount Tianshan region, in particular, has seen a notable expansion in glacier lake areas due to rising temperatures, necessitating further research and analysis to understand these changes and their implications (Wang et al., 2013; Li, 2019). However, remote sensing technology has proven to be a viable solution for observing and monitoring area changes in remote and inaccessible locations like Lake Mertzbakher, previous studies have shown certain limitations. For example, the accuracy of traditional water body extraction algorithms, such as the water index and threshold method, and machine learning classification algorithms (including SVM, RF, and DL) varies and is influenced by the data used (Chen et al., 2019; Li et al., 2019; Zhou and Dong, 2019). This variance has led to inconsistencies in the results obtained from different algorithms and data combinations (Palmer et al., 2015; Li et al., 2020a; Khandelwal et al., 2022; Zhang et al., 2022; Guo et al., 2023). Additionally, while the shift to global-scale studies using high-performance cloud computing platforms like GEE has brought several benefits, including low manual participation and high classification accuracy, there remains a gap in the comprehensive utilization of these platforms for specific applications such as glacier lake monitoring (Hansen et al., 2013; Pekel J. F. et al., 2016; Zou et al., 2017; Zou et al., 2018; Zhou et al., 2019; Wang et al., 2020; Wei et al., 2020; Zhou et al., 2022). Moreover, despite existing research on glacier lake outburst floods and their causes, such as glacier recession and increased meltwater due to warming, there is still a need for more detailed and region-specific studies (Qi et al. 2023; Wang et al., 2013; Li, 2019).
This study addresses the challenge of understanding the changing dynamics of glacier meltwater in Lake Mertzbakher under global climate change. Innovatively, it leverages the GEE cloud computing platform to efficiently analyze the lake’s area changes from 2000 to 2021 using extensive Landsat data (Landsat 5 TM, Landsat 7 ETM+ and Landsat 8 OLI Collection 1 Tier 1 surface reflectance data). This approach is particularly novel as satellite data is crucial for studying remote glacier lakes, which have been largely overlooked in previous research due to their inaccessibility and lack of observational data. Additionally, the study offers a comprehensive analysis of the impact of temperature changes on the lake, laying a groundwork for future predictions of glacier lake floods and contributing to effective disaster prevention strategies. Despite some limitations due to weather-related data accuracy issues, this research marks a significant step forward in predicting and managing the effects of climate change on glacier lake floods.
2 Materials and methods
2.1 Study area
The Lake Mertzbakher is situated in Kyrgyzstan, specifically at the upper reaches of the Kunmalike River. This river is a tributary of the Aksu River. The lake is located at the confluence point of the north and south branches of the Inilchek Glacier, precisely at coordinates 42°13′N, 79°52′E. The lake is positioned at an elevation of approximately 3300 m (as shown in Figure 1). Since 1998, the Lake Mertzbakher has existed as two separate lakes. The first one is known as the “lower lake,” which is formed by the South Inilchek Glacier creating a dammed lake. The second one is called the “upper lake” and is linked to the terminus of the North Inilchek Glacier. A narrow waterway connects the upper and lower lakes. Additionally, there are small lakes scattered sporadically between these two main lakes. The upstream lake consistently remains filled with water throughout the year, maintaining a stable area. On the other hand, the downstream lake only contains water during the water storage period. After outbursts, it often becomes empty, resulting in significant inter-annual changes. This fluctuation in water levels has a more substantial impact on the lower reaches of the river. Consequently, the primary focus of this study is the downstream lake for lake area extraction and analysis purposes.
2.2 Data
2.2.1 Landsat remote sensing data
This study utilized all available surface reflectance data from Landsat 5 TM, Landsat 7 ETM+, and Landsat 8 OLI Collection 1 Tier 1 sensors, covering the study area from 2000 to 2021. The data was obtained from the United States Geological Survey (USGS). To ensure accuracy, all Landsat data underwent geometric and atmospheric calibration and cross-calibration between different sensors. To access and process the Landsat data, we utilized the GEE remote sensing cloud computing platform, which includes Landsat data in its data archives. Initially, for each Landsat 5 TM, Landsat 7 ETM+, and Landsat 8 OLI image within the study area, we employed the quality control layer provided by the GEE platform to identify and filter out invalid observations. Additionally, we detected and removed terrain shadows using the solar zenith angle information contained in the images and the SRTM digital elevation model (DEM). Following the filtering steps mentioned above, the remaining Landsat image elements represented valid observations suitable for monitoring changes in surface water bodies. To synthesize valid observation data on a monthly time scale, we used the median synthesis method through the GEE cloud platform. This process involved calculating various remote sensing spectral indexes (such as mNDWI and EVI) and generating long-term series remote sensing spectral index datasets. Statistical analyses revealed that, on average, more than 99.9% of pixels contained good-quality Landsat observations.
2.2.2 Climatic data
The climatic data used in this study was sourced from ERA5-land, which is a reanalysis dataset produced through the ECMWF ERA5 reanalysis model. The spatial resolution of the data is 0.1° × 0.1°, equivalent to approximately a 9 km × 9 km grid. This dataset accurately represents the climatic conditions of Lake Mertzbakher. Monthly meteorological factors selected for analysis include maximum temperature, mean temperature, minimum temperature, radiation, and precipitation.
2.3 Methodology
2.3.1 Remote sensing extraction of surface water
This study employed the relationship between water body indexes and vegetation indexes for the extraction of surface water bodies. Building on previous researches (Wei et al., 2020; Zou et al., 2017; Hong et al., 2019; Gu et al. 2023) that analyzed changes in surface water bodies, we utilized a time series of Landsat data and a water index and threshold algorithm. The water body and vegetation indexes utilized in this study include the modified Normal Difference Water Index (mNDWI) (Wei et al., 2020), the Enhanced Vegetation Index (EVI), and the Normal Difference Water Index (NDVI). Cloud-free and snow-free Landsat TM, ETM+, and OLI surface reflectance data were used to calculate these indexes using Eqs 1–3 as follows:
Where “ρ,” “Blue,” “ρ”, “Green,” “ρ”, “Red,” “ρ,” “NIR,” “ρ,” and “SWIR1” represent the surface reflectance values in the blue light band (0.45–0.52 μm), green light band (0.52–0.60 μm), red light band (0.63–0.69 μm), near-infrared band (0.77–0.90 μm), and short-wave infrared band (1.55–1.75 μm) respectively. The mNDWI > EVI or mNDWI > NDVI condition was utilized to identify image elements with a stronger water signal than the vegetation signal. Additionally, EVI<0.1 was used to remove vegetation or mixtures of vegetation and water bodies. Therefore, image elements satisfying the logical relations [(mNDWI > EVI or mNDWI > NDVI) and (EVI<0.1)] were classified as water bodies, while the remaining elements were classified as non-water bodies. Figure 2 illustrates an example of water body extraction using this method, as reported in previous studies (McFeeters, 1996; Chen et al., 2017; Zou et al., 2017; Zou et al., 2018).
2.3.2 Meteorology for lake area changes
Attribution studies encompass climate change attribution, impact attribution, and identification of weather sensitivity. Previous reports demonstrate that climate change has had a widespread and profound impact on global terrestrial and marine ecosystems (Ray et al., 2015; Hope et al., 2016; Huggel et al., 2016; Su et al., 2022a). In this study, we investigated the relationship between monthly glacier lake area and meteorological factors in Lake Mertzbakher from 2000 to 2021, aiming to determine the attribution of climate change to the lake’s area. Drawing on previous researches (Yang et al., 2006; Du et al., 2007; Farsi and Mahjouri, 2019; Sun et al., 2019; Su et al., 2022b; Shi et al., 2023; Zhang et al., 2023), five meteorological factors were selected: maximum temperature, mean temperature, minimum temperature, radiation, and precipitation. Due to the limitation of the available Landsat observations, it was not feasible to generate monthly maps of Lake Mertzbakher for all the years from 2000 to 2021. Year 2000, 2005, 2010, 2015, and 2021 were chosen as the typical years.
The focal techniques employed in this study include linear regression, partial regression analysis, and a structural equation model (SEM). SEM is a system of simultaneous equations comprising two or more structural equations that represent the interrelationships between variables. The origins of modern SEM can be traced back to Wright’s work on path analysis (Wright, 1918; Wright, 1920; Wright, 1921). By obtaining path coefficients through path analysis, researchers can deconstruct various relationships within the system and uncover the underlying mechanisms driving these relationships. SEM enables exploration of the direct and indirect effects of different factors on the same process, as well as determination of the relative importance of these factors. It is a statistical method that analyzes the relationship between variables based on the covariance matrix of variables. SEM extends general linear models, incorporates factor models and structural models, representing the perfect fusion of traditional path analysis and factor analysis (Muthen, 1984; Sanchez et al., 2005; Wang and Chu, 2011). Modern SEM encompasses regression, factor analysis, statistical modeling, model evaluation, and related software (Chen et al., 2004; Xin et al., 2021; Luo, 2022; Chen et al., 2023; Yuan et al., 2023). Figure 3 shows the whole methodology in this study.
3 Results
3.1 Glacier lake area
The results of glacier lake area extraction at 60 time points across 12 months for the years 2000, 2005, 2010, 2015, and 2021 are presented in Figure 4. Except for 2021, the area of Lake Mertzbakher consistently peaked in July and then experienced a sharp decline, indicating glacier lake outbursts and rapid downstream water flow. The minimum area was observed in September for the years 2000, 2005, 2010, and 2021, and in August for the year 2015. Additionally, the area exhibited a secondary small peak in September and October for these 4 years, signifying small-scale outbursts. Except for 2021, the annual changes were consistent across the remaining 4 years.
In terms of the glacier lake area in July, the maximum value was 2.70754 km2 in 2000, while the minimum value was 2.4406 km2 in 2015. The area data for 2021 exhibited three patterns. Firstly, the area throughout the year was relatively small, with the maximum value occurring in June (1.5135 km2) and the minimum value in September (0.63327 km2). Secondly, the month with the maximum value was 1 month earlier compared to other years. Lastly, the area change was gradual, particularly with minimal difference between June and July, indicating a lower volume of outburst water and the absence of a secondary small peak. We chose year 2005 to show the changes in 12 months as shown in Figure 5. The typical process for the year is that, water level increased slowly from January to May due to the warmer weather, and then increased sharply from May to June with high temperature and some precipitation. The glacier lake collapsed in August and had the smallest amount of water in September. After the outburst, glacier lake began to store more water again. For some of the years, like 2005, there could be a second but smaller outburst after September.
3.2 Relationship between meteorological factors and lake area
3.2.1 Linear regression analysis
The findings of the linear regression analysis are presented in Figure 6. The results indicated a positive regression between the area of Lake Mertzbakher and the meteorological factors, although none of them reached a significant level. The minimum explanatory degree for the relationship between the meteorological factors and the glacierlake area change was observed for the maximum temperature (16.6%), whereas the maximum explanatory degree was observed for precipitation (26.4%).
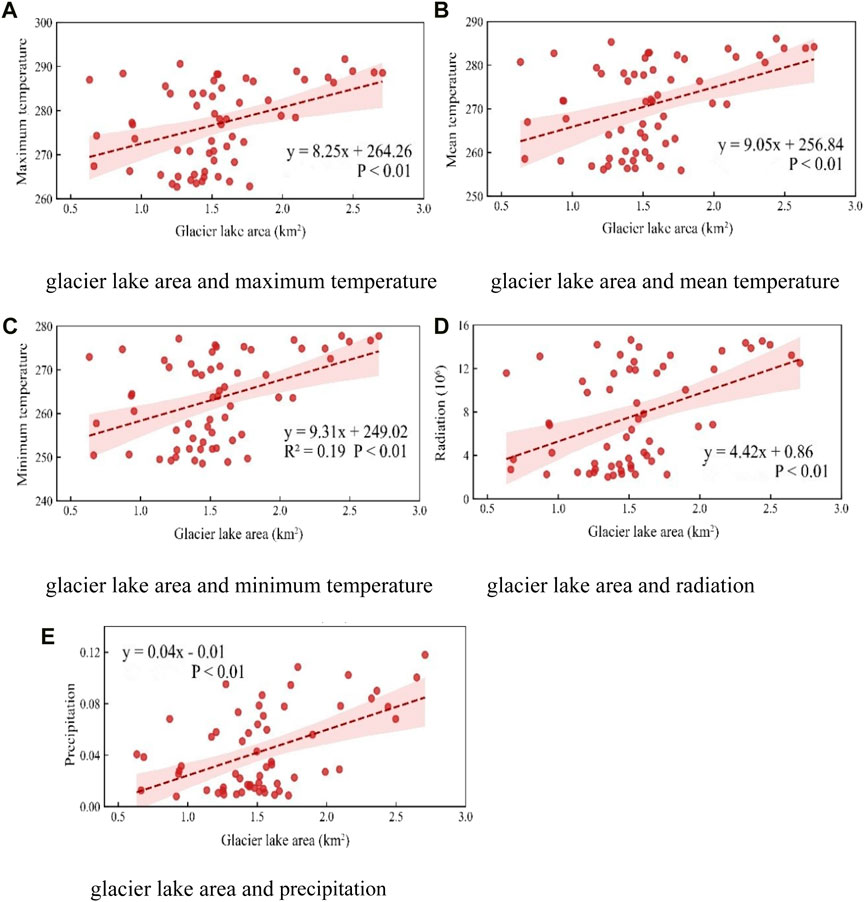
FIGURE 6. Linear regression results between the area of Lake Mertzbakher and the meteorological factors (A) glacier lake area and maximum temperature (B) glacier lake area and mean temperature (C) glacier lake area and minimum temperature (D) glacier lake area and radiation (E) glacier lake area and precipitation.
3.2.2 Partial regression analysis
The results of the partial regression analysis, as shown in Table 1, indicate that after controlling for other meteorological factors, there was a negative correlation between the area of Lake Mertzbakher and the mean temperature. The order of meteorological factors influencing the lake area from high to low were the minimum temperature (0.245), mean temperature (−0.239), precipitation (0.228), radiation (0.154), and maximum temperature (0.128). Considering the limited sample data, a significance level of 0.1 was used in this study, indicating a significant correlation between the glacier lake area and the meteorological factors.
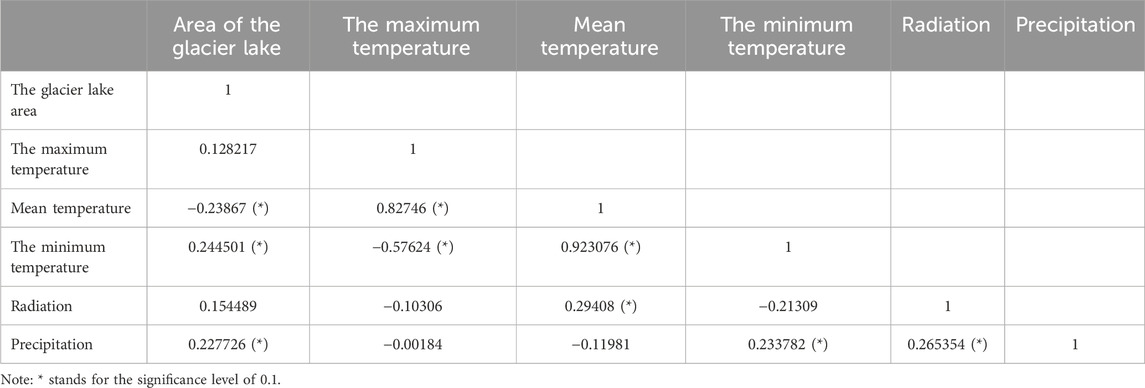
TABLE 1. Partial regression coefficients between the area of the Lake Mertzbakher and the meteorological factors.
3.2.3 Structural equation model
To further investigate the driving factors and influence pathways of the area change in Lake Mertzbakher under the interactions of meteorological factors, we constructed a structural equation model (SEM). In Figure 7, the p-value of the path analysis model was 0.323, indicating that the model fit was acceptable. The results of the path analysis were consistent with those of the partial regression analysis, suggesting a negative contribution of mean temperature to the area change of Lake Mertzbakher and positive contributions of minimum temperature, radiation, and precipitation to the area change. The overall explanatory power of the model for the area change in Lake Mertzbakher was 31%. The order of factors influencing the lake area from highest to lowest was mean temperature (−3.32), minimum temperature (2.87), radiation (0.48), and precipitation (0.47). These findings indicate that the mean temperature has the strongest negative impact on the area change of Lake Mertzbakher, followed by the minimum temperature, radiation, and precipitation.
Overall, the structural equation model provides insights into the driving factors and relationships among meteorological factors in influencing the area change of Lake Mertzbakher. It demonstrates the significant role of temperature, radiation, and precipitation in shaping the variations in lake area.
4 Discussion
4.1 Glacier Lake area dynamics
Lake Mertzbakher typically undergoes thawing from March to May annually, reaching peak storage in May and June. Consistent with previous findings, outbursts generally occur in the “lower lake” during late July and early August, aligning with the maximum lake area observed in our study in July (Zhou et al., 2019; Zhou et al., 2022). However, 2021 deviated from this pattern, exhibiting a significantly smaller area with the peak in June, likely due to extreme weather conditions. In 2021, many regions, including China with its highest recorded average surface temperature since 1901 (0.97°C above normal), and Kyrgyzstan, experienced unusual climatic conditions, adversely affecting crop production and potentially contributing to the observed decrease in glacier lake area and reduced outburst intensity (Apaer, 2014; Yu et al., 2021).
4.2 Attribution analysis of glacier lake area
Our linear regression analysis indicated a modest explanatory power (31%) for the area change of Lake Mertzbakher. This limited efficacy could stem from the complex interplay among various influencing factors, which linear regression may not fully capture. Further research suggests the timing of glacier lake outbursts, closely linked to mean temperature, significantly impacts area changes. Temperature increases, as shown in other studies, can escalate glacier meltwater, expand glacier lake areas and affect dam stability (Rouse et al., 1974; Jiang et al., 2014; Xin et al., 2019; Li et al., 2020b; Liu et al., 2020; Niu et al., 2022; Wang et al., 2022; Huang Z. et al., 2023). Despite a low regression coefficient, mean temperature remains a pivotal factor in Lake Mertzbakher’s area changes, underscoring the need for more intricate analyses to unravel the multifaceted impacts of mean temperature on the lake area.
4.3 Enlightenment for flood control and disaster reduction
To mitigate flood risks in Aksu and surrounding regions and safeguard economic and social stability, several strategies are proposed. Enhancing international collaboration for monitoring glacier lakes in Kyrgyzstan’s upper reaches and integrating efforts with Aksu’s water conservancy projects can significantly reduce flood damages. Accelerating the development and utilization of water resources in the Aksu River Basin, coupled with comprehensive basin-wide project planning, can strike a balance between disaster prevention and water resource utilization. Further investigation into glacier lake outburst flood mechanisms is crucial for improving flood warning and forecasting systems, thereby bolstering flood control, disaster reduction, and socio-economic progress in Xinjiang.
4.4 Limitations and prospects
This study faces limitations, notably the reliance on mean monthly area data due to weather constraints, potentially introducing analytical errors and impacting regression results. Future research should prioritize acquiring high-quality, frequent imagery data for more precise analyses. Additionally, employing machine learning and deep learning techniques for water body extraction could enhance natural disaster monitoring and warning, particularly for glacier lake outburst floods, improving accuracy and efficacy in disaster prevention efforts (Guo et al., 2021).
5 Conclusion
In addressing the critical challenge of deciphering the changing dynamics of glacier meltwater in Lake Mertzbakher, particularly under the escalating influence of climate change, this study has made significant strides. Utilizing the advanced capabilities of the GEE platform, we conducted a comprehensive analysis of the lake’s surface water body, tracking its area changes at numerous time points. This innovative approach not only enabled a more precise and frequent assessment compared to previous methods but also facilitated a detailed exploration of the correlation between lake area and various meteorological factors. Our findings reveal a pronounced outburst in Lake Mertzbakher occurring in July, culminating in a notable decrease in the lake’s area by September. The study meticulously quantifies the impact of meteorological factors on the lake area, identifying minimum temperature as having the most substantial influence, followed by mean temperature, precipitation, radiation, and maximum temperature. This nuanced understanding of the relationship between these factors and the lake area is a testament to the study’s methodological rigor and analytical depth. The significance of this research extends beyond the academic realm, offering crucial insights for the prediction and management of glacier lake floods. By establishing a clear connection between climate change-related factors and glacier lake dynamics, the study makes a substantial contribution to the field of disaster prevention and preparedness. In an era of increasing climate variability, these findings are invaluable for developing effective strategies to mitigate the impacts of climate change on vulnerable glacier lakes like Mertzbakher (Sun et al., 2013; Li et al., 2022; Huang S. et al., 2023).
Data availability statement
The original contributions presented in the study are included in the article/Supplementary material, further inquiries can be directed to the corresponding author.
Author contributions
XZ: Conceptualization, Investigation, Methodology, Writing–original draft. ZT: Conceptualization, Supervision, Validation, Writing–original draft. YZ: Resources, Software, Validation, Writing–review and editing. JZ: Software, Writing–review and editing. GS: Data curation, Validation, Visualization, Writing–review and editing. CH: Investigation, Writing–review and editing.
Funding
The author(s) declare financial support was received for the research, authorship, and/or publication of this article. This research was funded by the National Natural Science Foundation of China (Grant Number 42007185, 42001416, 42101343, and 71974053).
Conflict of interest
The authors declare that the research was conducted in the absence of any commercial or financial relationships that could be construed as a potential conflict of interest.
The reviewer NX declared a shared affiliation with the author ZT to the handling editor at the time of review.
Publisher’s note
All claims expressed in this article are solely those of the authors and do not necessarily represent those of their affiliated organizations, or those of the publisher, the editors and the reviewers. Any product that may be evaluated in this article, or claim that may be made by its manufacturer, is not guaranteed or endorsed by the publisher.
References
Apaer, (2014). Changes of major climatic elements of Mount Tianshan in Xinjiang in recent 50 years. J. Shihezi Univ. Nat. Sci. 32 (1), 86–92.
Araujo, R., Ponte, M. X., and Eslamian, S. (2021). Effects of land cover change on hydrological responses of a Large-Scale Basin in Brazilian Amazon. Int. J. Hydrology Sci. Technol. 12 (1), 39–58. doi:10.1504/ijhst.2021.116246
Chen, F., Zhang, M., Tian, B. S., and Li, Z. (2017). Extraction of glacial lake outlines in tibet plateau using Landsat 8 imagery and Google Earth engine. Ieee J-Stars 10, 4002–4009. doi:10.1109/Jstars.2017.2705718
Chen, Q., Lei, X., and Zhang, G. (2023). Runoff changes and attribution identification in the upper reaches of minjiang River in recent 59 years. Water Resour. Power 41 (3), 10–13. doi:10.20040/j.cnki.1000-7709.2023.20221672
Chen, Q., Liang, W., and Meng, Q. (2004). Structural equation modeling (SEM) in medical practice:introduction and application. Chin. J. Health Statistics 21 (2), 70–74. doi:10.3969/j.issn.1002-3674.2004.02.002
Chen, Q., Zheng, L., and Li, X. (2019). Study on water body extraction model with high-resolution remote sensing images based on deep learning. Geogr. Geo-Information Sci. 35 (4), 43–49.
Chen, T., Song, C., Ke, L., Wang, J., Liu, K., and Wu, Q. (2021). Estimating seasonal water budgets in global lakes by using multi-source remote sensing measurements. J. Hydrol. 593, 125781. doi:10.1016/j.jhydrol.2020.125781
Donchyts, G., Baart, F., Winsemius, H., Gorelick, N., Kwadijk, J., and van de Giesen, N. (2016). Earth’s surface water change over the past 30 years. Nat. Clim. Change 6, 810–813. doi:10.1038/nclimate3111
Du, Y., Xie, Z., Zeng, Y., Shi, Y., and Wu, J. (2007). Impact of urban expansion on regional temperature change in the Yangtze River Delta. J. Geogr. 17 (4), 387–398. doi:10.1007/s11442-007-0387-0
Farsi, N., and Mahjouri, N. (2019). Evaluating the contribution of the climate change and human activities to runoff change under uncertainty. J. Geogr. 574, 872–891. doi:10.1016/j.jhydrol.2019.04.028
Gu, C., Liu, M., and Hu, K. (2023). Satellite Remote sensing monitoring of glacierglacier lake outburst in alpine areas of Asia: a case study of monitoring of the Lake Mertzbakher. Disaster Reduct. China 25, 16–19.
Guo, J., Wang, X., Liu, B., Liu, K., Zhang, Y., and Wang, C. (2023). Remote-sensing extraction of small water bodies on the loess plateau. Water 15, 866. doi:10.3390/w15050866
Guo, Y. H., Fu, Y. S., Hao, F. H., Zhang, X., Wu, W. X., Jin, L. X., et al. (2021). Integrated phenology and climate in rice yields prediction using machine learning methods. Ecol. Indic. 1 (10), 106935. doi:10.1016/j.ecolind.2020.106935
Hansen, M., Potapov, P., Moore, R., Hancher, M., Turubanova, S. A., Tyukavina, A., et al. (2013). High-resolution global maps of 21st-century forest cover change. Science 342 (6160), 850–853. doi:10.1126/science.1244693
Hong, Y., Deng, W., and Zhang, X. (2019). Study on sequential changes of the Sarez Lake and driving factors in recent 50 years. J. Nat. Disasters 32 (2), 125–132.
Hope, P., Lim, E. P., Hendon, H., and Wang, G. (2016). The effect of increasing CO2 on the extreme September 2016 rainfall across southeastern Australia. Bull. Amer Meteor Soc. 99 (1), S133–S138. doi:10.1175/bams-d-17-0094.1
Huang, S., Chen, X., Ma, X., Fang, H., Liu, T., Kurban, A., et al. (2023a). Monitoring surface water area changes in the aral sea basin using the Google Earth engine cloud platform. Water 15, 1729. doi:10.3390/w15091729
Huang, Z., Xu, J., and Zheng, L. (2023b). Long-term change of Lake water storage and its response to climate change for typical lakes in arid Xinjiang, China. Water 15, 1444. doi:10.3390/w15081444
Huggel, C., Wallimann-Helmer, I., Stone, D., and Cramer, W. (2016). Reconciling justice and attribution research to advance climate policy. Nat. Clim. Change 6 (10), 901–908. doi:10.1038/nclimate3104
Jiang, H., Feng, M., Zhu, Y. Q., Lu, N., Huang, J. X., and Xiao, T. (2014). An automated method for extracting rivers and lakes from Landsat imagery. Remote Sens. 6, 5067–5089. doi:10.3390/rs6065067
Khandelwal, A., Karpatne, A., Ravirathinam, P., Ghosh, R., Wei, Z., Dugan, H. A., et al. (2022). ReaLSAT, a global dataset of reservoir and lake surface area variations. Sci. Data 9, 356. doi:10.1038/s41597-022-01449-5
Li, D. (2019). Study on area changes of the Lake mertzbakher on Mount Tianshan from 1998 to 2017. J. Glaciol. Geocryol. 12 (42), 1126–1134.
Li, D., Wu, B., and Chen, B. (2019). Study advance and prospects of water body information extraction based on satellite remote sensing. J. Tsinghua Univ. 59 (22), 1–25.
Li, Y., Niu, Z., Xu, Z., and Yan, X. (2020a). Construction of high spatial-temporal water body dataset in China based on sentinel-1 archives and GEE. Remote Sens. 12, 2413. doi:10.3390/rs12152413
Li, Y., Niu, Z. G., Xu, Z. Y., and Yan, X. (2020b). Construction of high spatial-temporal water body dataset in China based on Sentinel-1 archives and GEE. Remote Sens. 12, 2413. doi:10.3390/rs12152413
Li, Y. S., Dang, B., Zhang, Y. J., and Du, Z. H. (2022). Water body classification from high-resolution optical remote sensing imagery: achievements and perspectives. ISPRS J. Photogramm. Remote Sens. 187, 306–327. doi:10.1016/j.isprsjprs.2022.03.013
Liu, Q. H., Huang, C., Shi, Z. L., and Zhang, S. Q. (2020). Probabilistic River water mapping from Landsat-8 using the support vector machine method. Remote Sens. 12, 1374. doi:10.3390/rs12091374
Luo, Y. (2022). Academic contribution of klaus hasselmann, winner of the 2021 nobel prize in physics to climate change attribution. Physics 51 (1), 24–28. doi:10.7693/wl20220104
McFeeters, S. K. (1996). The use of the normalized difference water index (NDWI) in the delineation of open water features. Inter. J.Remote Sens. 17, 1425–1432. doi:10.1080/01431169608948714
Muthen, B. (1984). A general structural equation model with dichotomous, ordered categorical, and continuous latent variable indicators. Psychometrika 49 (1), 115–132. doi:10.1007/bf02294210
Niu, P., Zhang, E., Feng, Y., and Peng, P. (2022). Spatial-Temporal pattern analysis of land use and water yield in water source region of middle route of south-to-north water transfer project based on Google Earth engine. Water 14, 2535. doi:10.3390/w14162535
Palmer, S. C. J., Kutser, T., and Hunter, P. D. (2015). Remote sensing of inland waters: challenges, progress and future directions. Remote Sens. Environ. 157, 1–8. doi:10.1016/j.rse.2014.09.021
Pekel, J., Cottam, A., Gorelick, N., and Belward, A. S. (2016a). High-resolution mapping of global surface water and its long-term changes. Nature 540, 418–422. doi:10.1038/nature20584
Pekel, J. F., Cottam, A., Gorelick, N., and Belward, A. S. (2016b). High-resolution mapping of global surface water and its long-term changes. Nature 540 (7633), 418–422. doi:10.1038/nature20584
Qi, C., Ren, Y., and Peng, H. 2023 Study on lake area extraction and dynamic change in the source of three rivers based on GEE cloud platform [J/OL]. Qinghai, China: Journal of Yangtze River Scientific Research Institute.
Ray, D. K., Gerber, J., MacDonald, G. K., and West, P. C. (2015). Climate variation explains a third of global crop yield variability. Nat. Commun. 6, 5989. doi:10.1038/ncomms6989
Rouse, J. W., Haas, R. H., Schell, J. A., and Deering, D. W. (1974). “Third Earth resources technology satellite-1 symposium: the proceedings of a symposium held by goddard space flight center at Washington, DC on 10–14 december 1973,” in Prepared at goddard space flight center; scientific and technical information office (Washington, DC, USA: National Aeronautics and Space Administration).
Sanchez, B., Budtz-jorgensen, E., Ryan, L., and Hu, H. (2005). Structural equation models: a review with applications to environmental epidemiology. J. Am. Stat. Assoc. 100 (472), 1443–1455. doi:10.1198/016214505000001005
Shi, W., He, Y., and Shao, Y. (2023). Attribution analysis of runoff change and its influencing factors in the upper reaches of ialing river. J. Soil Water Conservation 37 (3), 124–131. doi:10.13870/j.cnki.stbcxb.2023.03.017
Su, B., Chen, Z., and Huang, J. (2022b). Impact attribution of climate change:the new cognition from IPCC AR6. WGⅡTrans Atmos. Sci. 45 (4), 512–519.
Su, B., Chen, Z., Huang, J., and Jiang, T. (2022a). Impact attribution of climate change:the new cognition from IPCC AR6 WGⅡ. Trans. Atmos. Sci. 45 (4), 512–519. doi:10.13878/j.cnki.dqkxxb.20220425006
Sun, S., Bao, C., and Tang, Z. (2019). Tele-connecting water consumption in Tibet:patterns and socio-economic driving factors for virtual water trades. J. Clean. Prod. 2019 233, 1250–1258. doi:10.1016/j.jclepro.2019.06.141
Sun, Y., Yin, H., Tian, Q., Hu, T., Shi, Y., Liu, H., et al. (2013). Recent progress in studies of climate change detection and attribution in the globe and China in the past 50 years. Clim. Change Res. 9 (4), 235–245. doi:10.3969/j.issn.1673-1719.2013.04.001
Wang, H., Qi, Y., Lian, X., Zhang, J., Yang, R., and Zhang, M. (2022). Effects of climate change and land use/cover change on the volume of the Qinghai Lake in China. J. Arid. Land 14, 245–261. doi:10.1007/s40333-022-0062-4
Wang, X., Chen, Y., Li, Z., Fang, G., Wang, F., and Liu, H. (2020). The impact of climate change and human activities on the Aral Sea Basin over the past 50 years. Atmos. Res. 245, 105125. doi:10.1016/j.atmosres.2020.105125
Wang, X., Ding, Y., and Liu, S. (2013). Changes of glacierglacier lakes and implications in Tian Shan, central Asia, based on remote sensing data from 1990 to 2010. Environ. Res. Lett. 8 (4), 575–591.
Wang, Y., and Chu, C. (2011). A brief introduction of structural equation model and its application in ecology. Chin. J. Plant Ecol. 35 (3), 337–344. doi:10.3724/SP.J.1258.2011.00337
Wei, X., Xu, W., Bao, K., Hou, W., Su, J., Li, H., et al. (2020). A water body extraction methods comparison based on FengYun satellite data: a case study of poyang lake region, China. Remote Sens. 12 (23), 3875. doi:10.3390/rs12233875
Wright, S. (1920). The relative importance of heredity and environment in determining the piebald pattern of Guinea pigs. P Natl. Acad. Sci. U. S. A. 6, 320–332. doi:10.1073/pnas.6.6.320
Wu, L., Wang, S., Ma, Y., Yang, R., Guan, Y., Hai, K., et al. (2022). Response of vegetation to climate change in Central Asia with remote sensing and meteorological data. J. Remote Sens. 26 (11), 2248–2267. doi:10.11834/jrs.20209216
Xin, L., Liu, X., and Zhang, B. (2021). Land surface temperature retrieval and geothermal resources prediction by remote sensing image: a case study in the Shijiazhuang area, Hebei province. Int. J. Geomech. 27 (1), 40–51.
Xin, Z., Li, Y., Zhang, L., Ding, W., Ye, L., Wu, J., et al. (2019). Quantifying the relative contribution of climate and human impacts on seasonal streamflow. J. Hydrol. 574, 936–945. doi:10.1016/j.jhydrol.2019.04.095
Yang, D., Sun, F., Liu, Z., Cong, Z., and Lei, Z. (2006). Interpreting the complementary relationship in non-humid environments based on the Budyko and Penman hypotheses. Geophys Res. Lett. 33 (18), 122–140. doi:10.1029/2006gl027657
Yu, B., Cui, B., Zang, Y., Wu, C., Zhao, Z., and Wang, Y. (2021). Long-term dynamics of different surface water body types and their possible driving factors in China. Remote Sens. 13, 1154. doi:10.3390/rs13061154
Yuan, Y., Xiao, W., and Yu, Y. (2023). Criteria of structural equation modeling: comparisons and enlightenments of CB-SEM and PLS-SEM. J. Inf. Resour. Manag. 13 (3), 6–22. doi:10.13365/j.jirm.2023.03.006
Zhang, G., Huang, L., Wang, J., Jiang, Z., Lu, X., and Luo, X. (2023). Variation characteristics of meteorological and hydrological factors and attribution analysis of runoff variation in Qinhuai River Basin. J. Arid Meteorology 41 (1), 54–63. doi:10.11755/j.issn.1006-7639(2023)-01-0054
Zhang, Y., Wang, N., Yang, X., and Mao, Z. (2022). The dynamic changes of Lake issyk-kul from 1958 to 2020 based on multi-source satellite data. Remote Sens. 14, 1575. doi:10.3390/rs14071575
Zhao, S., Duan, H., and Gao, S. (2018). Study on water body classification with MODIS remote sensing images based on random forest. J. Shandong Normal Univ. 33 (1), 19–25.
Zheng, G., Bao, A., Li, J., Zhang, G., Xie, H., Guo, H., et al. (2019). Sustained growth of high mountain lakes in the headwaters of the syr darya river, central asia. Glob. Planet Change 176, 84–99. doi:10.1016/j.gloplacha.2019.03.004
Zhou, Q., Li, J., and Cui, A. (2021). The response of terrestrial water resources to climate change in central asia aridzone. J. China Hydrology 41 (2), 8–13. doi:10.19797/j.cnki.1000-0852.20201222
Zhou, Y., and Dong, J. (2019). Study advance of land surface water body monitoring by remote sensing. J. Geo-Information Sci. 21 (11), 1768–1778.
Zhou, Y., Dong, J., Cui, Y., Zhou, S., Wang, X., Zou, Z., et al. (2022). Rapid surface water expansion due to increasing artificial reservoirs and aquaculture ponds in North China Plain. J. Hydrology 2022, 127637. doi:10.1016/j.jhydrol.2022.127637
Zhou, Y., Dong, J., Xiao, X., Liu, R., Zou, Z., Zhao, G., et al. (2019). Continuous monitoring of lake dynamics on the Mongolian Plateau using all available Landsat imagery and Google Earth Engine. Sci. Total Environ. 689, 366–380. doi:10.1016/j.scitotenv.2019.06.341
Zou, Z., Dong, J., Menarguez, M. A., Xiao, X., Qin, Y., Doughty, R. B., et al. (2017). Continued decrease of open surface water body area in Oklahoma during 1984-2015. Sci. Total Environ. 595, 451–460. doi:10.1016/j.scitotenv.2017.03.259
Keywords: glacier lake, climate change, flood disaster, Google Earth Engine platform, attribution analysis
Citation: Zhang X, Tang Z, Zhou Y, Zhu J, Sun G and Huang C (2024) Beyond the ice: decoding Lake Mertzbakher’s response to global climate shifts. Front. Environ. Sci. 12:1351872. doi: 10.3389/fenvs.2024.1351872
Received: 07 December 2023; Accepted: 06 February 2024;
Published: 29 February 2024.
Edited by:
Fan Mo, Ministry of Natural Resources of the People’s Republic of China, ChinaReviewed by:
Yahui Guo, Central China Normal University, ChinaZhichao Li, Institute of Geographic Sciences and Natural Resources Research (CAS), China
Nan Xu, Hohai University, China
Copyright © 2024 Zhang, Tang, Zhou, Zhu, Sun and Huang. This is an open-access article distributed under the terms of the Creative Commons Attribution License (CC BY). The use, distribution or reproduction in other forums is permitted, provided the original author(s) and the copyright owner(s) are credited and that the original publication in this journal is cited, in accordance with accepted academic practice. No use, distribution or reproduction is permitted which does not comply with these terms.
*Correspondence: Jie Zhu, zhujie03@caas.cn