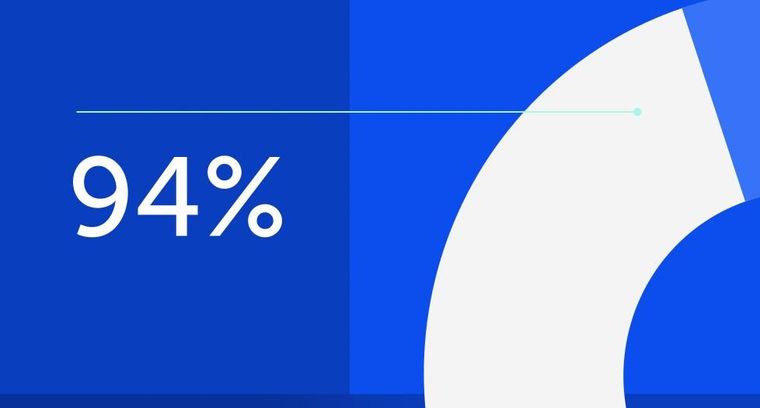
94% of researchers rate our articles as excellent or good
Learn more about the work of our research integrity team to safeguard the quality of each article we publish.
Find out more
ORIGINAL RESEARCH article
Front. Environ. Sci., 06 June 2024
Sec. Environmental Economics and Management
Volume 12 - 2024 | https://doi.org/10.3389/fenvs.2024.1342321
This article is part of the Research TopicLow-Carbon Economy and Sustainable Development: Driving Force, Synergistic Mechanism, and Implementation PathView all 21 articles
The accountability audit of natural resources (AANR) is a major institutional arrangement for advancing the construction of an ecological civilization in China. Based on the panel data of 271 cities in China from 2005 to 2017, this paper investigates the relationship between the AANR and carbon dioxide (CO2) emissions using a multi-period difference-in-differences (DID) model. The results show that AANR significantly increases the CO2 emission reduction rate by 0.009 units at the 5% significance level. The results still hold after a series of robustness tests. Given all else being equal, this significant effect is 0.001. Further analyses show that AANR improves pilot cities’ CO2 emission reduction rates mainly by enhancing their green innovation capability. The mediating effect of cities’ green technology innovation capability accounts for 96.00%, while the AANR’s direct effect only accounts for 4.00%. The AANR has significantly positive effects of 0.017% and 0.029% for western cities and cities with high fiscal pressure at the 5% and 1% significance levels, respectively. Therefore, strengthening AANR implementation by enhancing the mediating efficiency of cities’ green technology innovations and implementing dynamically differentiated AANR policies in Chinese meso-cities will contribute to the achievement of China’s carbon peaking and carbon neutrality targets.
Although high-carbon enterprises stagnated worldwide to varying degrees in 2020 due to the COVID-19 pandemic, China’s CO2 emissions were as high as 9.899 billion tons, which is a level far higher than those observed in other regions. According to the World Energy Statistical Yearbook (70th Edition), carbon emissions in the Asia–Pacific region accounted for 52% of total global emissions in 2020, with China accounting for as much as 30.70% of those emissions. Excessive CO2 emissions destroy the ozone layer, cause global warming, and thus pose a great threat to human security. As the world’s largest carbon emitter, China has undertaken many policy measures and actions to reduce carbon emissions. In 2020, the General Secretary of the Communist Party of China (CPC), Jinping Xi, proposed the goal of “achieving a carbon peak by 2030 and carbon neutrality by 2060” (i.e., the “dual carbon” goals). In 2021, the “Opinions on the Complete and Accurate Implementation of the New Development Concept to Achieve Carbon Peak and Carbon Neutrality” and the “Carbon Peaking Action Plan by 2030” were issued. In 2022, the CPC’s 20th National Congress report proposed measures to engage in carbon emission and pollution reduction while actively and steadily moving toward carbon neutrality. Although China has made some achievements in reducing carbon emissions since then, there is still much progress to be made to achieve the “dual carbon” goals.
In China’s existing environmental governance system, the central government is mainly responsible for formulating policies and providing normative guidance to local governments and enterprises. Local governments, as the intermediary between the central government and enterprises, enable information transmission between those parties. However, local officials are influenced by the traditional idea of “GDP championship,” so their long-held practice of sacrificing the environment for GDP growth is difficult to change. Economic development has traditionally been pursued at the expense of environmental resources, and environmental protection has been either verbally guaranteed or not guaranteed at all. In this context, the accountability audit of natural resources (AANR) came into being to force local officials to realize the importance of environmental protection. In 2013, the Third Plenary Session of the 18th CPC Central Committee first proposed the AANR. In 2014, the National Audit Office organized Hubei, Shandong, Inner Mongolia, and other local audit organs to carry out the AANR. China continued to carry out pilot audits in stages from 2015 to 2017. In 2018, the AANR was fully implemented in China when it entered a normalized phase. The auditing authorities evaluate the environmental performance of leading actors using a scientific index system, the results of which serve as an important basis for the assessment, appointment, dismissal, compensation, and punishment of local officials. Specifically, in terms of carbon emissions, the auditing authorities focus on local governments’ implementation of major initiatives aimed at achieving carbon peaking and carbon neutrality and scrutinize their formulation and implementation of low-carbon policies, their management and use of low-carbon funds, and the success of low-carbon engineering projects. However, the AANR, which is still in its incipient stages, still faces problems such as unclear delineations of responsibility, difficulty in data extraction, and underdeveloped assessment and evaluation systems (Zhu and Xu, 2022). These make the AANR suboptimal in its current state.
As an institutional innovation in China, the literature on the AANR has mainly focused on its theoretical basis and policy implementation effects. From a theoretical perspective, the existing literature mainly conducts normative research in the context of audit theory (Li and Yin, 2016), audit elements (Liu and Sun, 2016), and audit evaluation indices (Xiong et al., 2022). In terms of policy implementation effects, the existing literature is mostly empirical in nature and examines such factors as economic surplus management (Liu and Xie, 2018), green technology innovation (He and Feng, 2021), environmental information disclosure quality (Zhang and Li, 2021), and environmental investment at the firm level (Huang and Xie, 2022). At the macro level, existing literature investigates the effects of the AANR on urban innovation capability (Fu, 2022), regional environmental governance (Wu et al., 2020), and government officials (Zhu et al., 2022). These research studies affirm the AANR’s positive effects. However, Yan et al. (2022) found that the AANR has a significant negative impact on corporate ESG. In addition, Wu et al. (2020) found that the implementation of the AANR has an insignificant impact on exhaust and smog emission improvement. Current interpretations of AANR’s potential economic consequences have also not covered the mechanism of AANR’s impact on carbon emission reduction efficiency.
There are lots of researchers who study the impact of auditing on carbon emissions. In the case of energy audits, researchers found that they can reduce energy use (Kontokosta et al., 2020) and carbon dioxide emissions from paper mills (Kong et al., 2013). Energy audits even achieve carbon emission reduction by improving energy use efficiency in industrial firms (Thollander et al., 2013; Kluczek and Olszewski, 2017). In terms of environmental auditing, the AANR reduces carbon emissions by utilizing more industrial capacity (Li and Guo, 2022). It also promotes agricultural technological progress to reduce agricultural carbon emission intensity (Liu et al., 2023). Zhao et al. (2022), on the other hand, found that air pollution audits curb carbon emissions but have no effect on carbon emission intensity. In summary, these scholars explore special audits’ impacts on carbon emissions. They emphasize that auditing improves energy use efficiency to achieve carbon reduction. Research in the field of economics suggests that R&D for endogenous technological changes improves the effectiveness of the power sector’s decarbonization (Jiang et al., 2023). However, there are fewer studies related to the AANR impact on carbon reduction efficiency based on a meso-city perspective.
As such, it remains unclear whether the AANR can reduce China’s overall emission reduction rates and, if so, through which mechanisms it can exert its effect. Furthermore, whether and the degree to which the AANR effect varies across cities must also be investigated. This paper systematically assesses the AANR impact on the CO2 emission reduction rate in pilot cities in China by adopting a multi-period difference in differences (DID) model with CO2 emissions as the observation object during 2005–2017. The significant advantage of the multi-period DID model is that it treats the AANR as a policy dummy variable to quantify the changing effect of CO2 reduction rates before and after the AANR implementation in the pilot cities. This can directly induce the potential CO2 governance effects of the AANR implementation with which this paper is concerned.
This research aims to quantitatively analyze the AANR’s externality effects on carbon emission reduction in order to reveal the key mechanisms underlying China’s AANR implementation on carbon emission reduction in meso-cities so as to provide a scientific theoretical basis and practical policy support for effectively reducing CO2 emissions. Our work’s innovations are summarized as follows: (1) existing research studies have mainly investigated the AANR’s economic consequences at the enterprise level. Research studies on the AANR impact on carbon emissions have mainly focused on improving energy use efficiency to reduce carbon emissions. However, there is limited literature that analyzes in depth the AANR impact on carbon emission reduction efficiency in meso-cities in China. This paper expands the scope of research in this area. (2) We investigate whether the AANR impact on cities’ carbon emission reduction efficiency varies significantly depending on the superimposed impact of central environmental protection inspections, geographical differences between east-central and western cities, the level of cities’ green innovation capability, and the magnitude of financial pressure. It supplements the heterogeneity research on the AANR impact on carbon emission reduction efficiency. (3) This paper further examines the mechanism of the AANR impact on cities’ carbon emission reduction efficiency under the mediation effect of cities’ green innovation capability. It enriches the research on the influencing factors of carbon emission reduction efficiency and improves the theoretical basis of this paper’s conclusions and policy recommendations. The main contributions of this study include the following two aspects: on the one hand, against the background of the “dual carbon” goals and AANR, this paper investigates the AANR’s impact on carbon emissions by constructing a multi-period DID model; on the other hand, it differentiates and reveals the influencing mechanisms in the difference between what the local government “says” and what it “does” with regard to carbon emissions, as represented by cities’ green technology innovation capability. This study therefore not only supplements the existing studies but also helps local Chinese governments understand and implement the carbon emission reduction function of the AANR and provides meso-city level ideas and empirical evidence for achieving the “dual carbon” goals in China.
CO2 emissions have negative economic externalities that cannot be regulated by the market mechanism. Thus, the Chinese government has to intervene using its “visible hand.” However, there are still problems with the awareness and attitude of Chinese local officials toward CO2 emissions, thereby resulting in insufficient and inefficient policy implementation (Huang and Xie, 2022). After unveiling the AANR pilot project, local governments’ appraisal systems have incorporated environmental protection as a mandatory indicator for evaluating Chinese local officials’ performance. Based on the “rational man” assumption, local officials will seek to maximize their performance to enhance their own value (Chen, 2023). Local officials realize that their performance is no longer only judged on GDP growth and must now pursue a balance between GDP growth and environmental protection (Huang and Xie, 2022). To this end, local officials will strive to outperform on indicators with clear metrics such as energy conservation and emissions reduction, which are often the focus of auditing authorities. In particular, when local officials’ carbon reduction efforts receive positive feedback from the central government, they will be motivated to further reduce carbon emissions and explore new models and pathways to achieving the “dual carbon” goals.
The AANR comprises multiple functions and exerts strong pressure on local governments’ carbon emission reduction efforts (Zhu and Li, 2022). First, as an environmental regulation tool, the AANR comprehensively supervises the environmental performance of local officials and holds them accountable while urging the rectification and improvement of lingering problems. By scrutinizing low-carbon policy formulation and implementation, auditing authorities can determine whether local governments and relevant departments have upheld the emission targets. With the help of carbon fund auditing, they can decide whether local governments have misappropriated funds or retained idle carbon. They can also identify whether such projects have effective planning, delayed construction progress, or falsified data by checking the management of low-carbon projects (Li and Guo, 2022). Second, the AANR is characterized by lifelong accountability for ecological and environmental damage. Even if local officials achieve rapid carbon emission reductions in their jurisdictions during their tenure through campaign-style governance, the auditing authorities will still monitor the subsequent air quality after their departure. This increases government officials’ environmental responsibility. Local officials will therefore take effective measures to improve carbon emission governance to reduce their risk of being held accountable. Finally, the AANR reveals the shortcomings of local officials’ ecological and environmental governance. In response to the problems identified by the audit, relevant departments can hold local officials accountable and urge them to rectify all aspects of their ecological and environmental governance. Therefore, the AANR ensures the achievement of scheduled CO2 emission reduction targets.
Based on the above analysis, this paper proposes the following hypothesis:
H1:. The implementation of the AANR improves the CO2 emission reduction rate.
The previous section discusses the AANR impact on the CO2 emission reduction rate. Next, we examine the AANR’s transmission mechanism, which affects the CO2 emission reduction rate from the perspectives of government’s concern for environment and cities’ green technology innovation capability.
First, we examine the potential transmission mechanism of the government’s concern for the environment. Environmental governance will be focused on areas where the government’s attention is directed (Shen et al., 2020). In recent years, China has attached great importance to ecological and environmental governance. The implementation of the AANR suggests that local officials’ assessments will incorporate ecological and environmental protection, whether for the sake of personal promotion or exemption from accountability. In the context of carbon peaking and carbon neutrality, carbon reduction has become the key factor in China’s ecological development, which will greatly improve the government’s attention to environmental considerations. The government’s concern for the environment (Wang et al., 2014) provides guidance for local carbon emission reduction efforts to a certain extent. On the one hand, this concern alleviates the possibility of contradictory policy implementations. Local officials often take the initiative to coordinate various departments in finding ways to reduce carbon emissions and improve carbon policy implementation. On the other hand, it sends a signal of the importance of carbon emission reduction and the urgency of attracting significant human, financial, and technological resources to the field of environmental management. As such, it provides an important impetus for carbon emission reduction.
Second, we examine the potential transmission mechanism of cities’ green technology innovation capability. Local governments have a significant impact on innovation capability at the regional level (Zhu et al., 2022). After the implementation of the AANR, local governments realized that existing measures to meet environmental regulations, such as shutting down heavily polluting and high-emission enterprises, were undesirable. They also realized that they should encourage and incentivize enterprises in their jurisdictions to engage in green technology innovation (He and Feng, 2021). Local governments generally force enterprises to engage in green technology innovation by increasing environmental penalties or providing relevant policy support and financial subsidies. Improving cities’ green technology innovation can reduce CO2 emissions (Wang et al., 2019). It reduces regional carbon emissions through improved green production methods, specialized division of labor, and cost savings. It also eliminates high-emission enterprises, improves energy efficiency, and reduces fossil energy consumption through industrial reconstruction and other means, thereby reducing carbon emissions (Gu et al., 2022).
Therefore, this paper proposes the following hypothesis:
H2:. The AANR improves the CO2 emission reduction rate by increasing the government’s concern for the environment as well as cities’ green technology innovation capability.
This paper uses the panel data of 271 prefecture-level cities across China from 2005 to 2017 as the research object. Because of the lack of a control group in 2018, when all cities implemented the AANR, it does not contain data from 2018 and beyond. We manually collected and verified pilot cities’ data through official web pages, media reports, and the China Audit Yearbook. Twenty-six cities began implementing the AANR in 2014. Thus, we chose 2014 as the AANR reference year. We also calculated the CO2 emission reduction rate based on China’s county-level carbon emission data in China’s Carbon Accounting Database (CEAD) from 2005 to 2017. The texts of the government’s work reports are taken from the official website of each city. China’s Urban Statistical Yearbook and provincial statistical yearbooks offer the number of cities’ green invention data. Data for control variables are from the CSMAR database. The EPS DATA database and the Chinese Research Data Services Platform (CNRDS) provide supplementary information.
This paper excludes regions with serious missing variable data and fills in some missing data by forward or backward interpolation. Finally, we obtained a total of 3,523 observations from 2005 to 2017, including 305 in the treatment group and 3,218 in the control group. To avoid the influence of extreme values on the results, we apply winsorization at the 1% and 99% levels on all continuous variables. In addition, we adjust the standard errors of all regression models for clustering at the city level.
The impact of the implementation of the AANR on cities’ CO2 emission reduction rates may originate from policy- or time-generated effects. Accordingly, we must correctly measure the AANR’s net policy effect on cities’ CO2 emission reduction rates. Here, we divide China’s 271 prefecture-level cities into treatment and control groups according to whether they have implemented the AANR. The multi-period DID model is set as
where
(1) Explanatory variable. The CO2 emission reduction rate (Y) is the opposite of the CO2 emission growth rate, which is given by
where
(2) Explanatory variable. Audit is the key explanatory variable, which represents the interaction between the pilot city and time dummy variables. It takes the value of one when the pilot city starts to implement the AANR and thereafter; otherwise, it takes the value of zero.
(3) Mediating variables. The government’s work reports reflect the importance of government in environmental management. Hence, the total word count of “emission reduction,” “low carbon,” “CO2,” “energy consumption,” “air,” and “ecology” in the local government’s work reports indicates their concern for the environment (Gov). The cities’ green technology innovation capability (Inno) is the logarithm of the number of green inventions obtained in year +1.
(4) Control variables. To ensure the accuracy of empirical results, we introduce other control variables that may affect CO2 emissions into the multi-period DID model by referring to the existing literature (Xue et al., 2022). These variables include fiscal status (Finance), which is expressed as the ratio of general revenues to general expenditures; population density (Lnpopd), which is obtained by dividing the household population by the land area of cities’ administrative region; economic development (Lnpgdp), as expressed by the logarithm of GDP per capita; the level of foreign investment (Lnfdi), which is the logarithm of the amount of actual foreign direct investment utilized at the end of the year; and industrial structure indicator (Indust), which is the ratio of secondary industry GDP to total GDP. The variable descriptions are summarized in Table 1.
Table 1. Variable information. All variables are extracted and transformed according to their descriptions.
Table 2 shows the descriptive statistics of the variables. The minimum and maximum values of the CO2 emission reduction rate (Y) are −29.50% and 10.20%, respectively, with a mean value of −6.10% and a standard deviation of 7.20%. This infers that there is a large gap in the CO2 emission reduction rate among cities. The mean of Audit is 0.087, which indicates that 8.70% of the cities are affected by the AANR. The descriptive statistics of other variables also illustrate the large differences among cities.
The mean of the carbon reduction rate before the implementation of the AANR (i.e., prior to 2014) was −0.065, which is much smaller than the mean value (−0.015) after the implementation of the AANR (i.e., 2014 and beyond). A variety of factors affect carbon reduction rates, one of which may increase the rate while another may decrease it. It is not possible to directly quantify from Figure 1 that the implementation of the AANR has enhanced the carbon reduction rate. Nevertheless, this does not affect our rough inference that hypothesis H1 is valid from the observations before and after the implementation of the AANR.
Figure 1. Trends in carbon reduction rates. The black scatters indicate the carbon reduction rate for cities where the AANR is not implemented. Red color scatters are the carbon reduction rates of cities that have implemented the AANR.
The variable correlations are shown in Table 3. The correlation coefficients of most variable pairs are below 0.50. The correlation between Audit and Y is 0.194 and is statistically significant. This preliminarily verifies Hypothesis H1. In addition, we perform a variance inflation factor (VIF) test on the variables. The average VIF is 2.15, and the maximum is 3.50, which is much less than 10. The test result indicates that there is no multicollinearity among variables.
Table 4 illustrates the AANR effect on the CO2 emission reduction rate by fitting the DID model (1). Model (1) in Table 4 shows the regression results for the key explanatory variable Audit only. The estimated coefficient of Audit is significantly positive at the 5% significance level, thus indicating that the AANR significantly improves the CO2 emission reduction rate in the pilot cities. It verifies Hypothesis H1. Models (2) to (6) are the regression results after gradually adding control variables to Model (1). The estimated coefficients of Audit are still significantly positive at the 5% significance level, which further illustrates that the AANR dramatically improves the CO2 emission reduction rate in the AANR pilot cities.
The previous results suggest that the AANR improves the CO2 emission reduction rate, but an important prerequisite for the DID model’s results to hold is that the cities in the treatment and control groups must have the same trend in CO2 emission reduction before AANR implementation. To verify this, this paper adopts the event analysis method to conduct a parallel trend test. The regression model of the parallel trend test is set as follows:
where pren and postn are year dummy variables that denote the nth year before or after AANR implementation and take the value of 1 for pilot cities; otherwise, they are 0. Then, they are multiplied by quota, whose value is 1 for AANR pilot cities and 0 for non-AANR pilot cities.
In regression model (3), we choose the first period before the AANR implementation as the base period in the DID model. We also truncate the number of periods before AANR implementation as necessary. None of the estimated coefficients on
Figure 2. Coefficient plot of the parallel trend test in regression model (3). The whiskers represent the 95% confidence intervals of estimated coefficients.
The sample contingency or other factors may also lead to a significant effect on the AANR. To verify whether the effect of the AANR is real, this paper utilizes Stata 16.0 software to randomly sample the treatment and experimental groups for a placebo test. We repeat this process 5,000 times. Figure 3 implies that the distribution of the treatment effect estimates based on random sampling is approximately normally distributed with a mean value of 0. The estimated AANR coefficients are dramatically different from those of the basic regression (approximately 0.009), as shown in Table 4. Therefore, the placebo test holds that the effect of the AANR on the CO2 emission reduction rate is not significantly influenced by potential unobserved random factors. Thus, the reliability of the fitting results in Table 4 is further verified.
Figure 3. Placebo test results. The density of the treatment effect estimates with randomly sampled control and treatment sample groups.
We advance the AANR occurrence timing by 5, 4, and 3 years and rerun the regressions. The results are Models (1), (2), and (3) in Table 6. The estimated coefficients of F5.Audit, F4.Audit, and F3.Audit are statistically nonsignificant, indicating no effects from other unobservable variables. Thus, the benchmark results in Table 4 are robust. In China, the AANR is implemented on a yearly basis. The AANR exerts the greatest deterrent pressure on local officials in the observing year of censorship. The results in Table 6 are consistent with this fact.
Table 6. Robustness test results. Models (1), (2), and (3) are the result of placebo test II. Models (4) and (5) describe the effects of the AANR when excluding the influence of extreme values and interference from other factors, respectively.
We continue to re-estimate the multi-period DID model with upper and lower 5% tailing for all continuous variables. The results are shown in Model (4) of Table 6. The AANR coefficient estimates are still markedly positive at the 5% significance level, and the magnitude is comparable to the basic regression coefficient estimates in Table 4. This somewhat verifies the reliability of the benchmark results.
Since 2012, China has attached great importance to its ecological development and introduced a number of environmental governance policies in pursuit of this goal. Rationally, it must be determined whether the CO2 emission reduction rate will be affected by related policies during the AANR pilot period. From 2016 to 2017, China continually carried out environmental protection inspections in 31 provinces and cities, whose implementation targets overlapped with those of the AANR to some extent (Huang and Xie, 2022). To exclude the interference of the same type of policy on the CO2 emission reduction rate, this paper includes the environmental protection inspection variable (Inspect) in the multi-period DID regression model. If environmental protection inspection is conducted in the observing year, Inspect takes the value of 1 thereafter; otherwise; it takes the value of 0. Model (5) in Table 6 shows the fitting results. The estimated coefficient on the AANR pilot (Audit) is still significantly positive. Therefore, the fitting results of basic regression (1) in Table 4 remain robust.
The basic regressions (Table 4) and a series of robustness tests (Tables 5, 6; Figures 2, 3) suggest that the implementation of the AANR increases the cities’ CO2 emission reduction rate. However, we are yet to determine through which mechanism the AANR improves the cities’ CO2 emission reduction rate. We scrutinize the impact of the AANR pilot on the CO2 emission reduction rate from two perspectives: the government’s concern for the environment and cities’ green technology innovation capability. Drawing upon the existing literature (Wen and Ye, 2014), we test these potential mechanisms by applying the mediating effect, as shown in Eq. 1 and Eqs 4, 5:
Table 7 shows the regression results of the mediating effect model. Models (1) and (3) show that the coefficient estimates of the AANR (Audit) are significantly positive at the 5% and 1% levels. This indicates that the AANR significantly increases the government’s concern for the environment and enhances cities’ green technology innovation capability. The coefficient estimate of Gov in Model (2) of Table 7 is statistically nonsignificant. Furthermore, the Sobel test results show that the government’s concern for the environment as a mediating variable is not significant. It implies no mediating significance of the local government’s concern for the environment on the CO2 emission reduction rate. The possible reason for this finding lies in the AANR, as a newly introduced policy, being more likely to convey a new value tendency. However, due to limited cognition, a clear action roadmap has not yet been developed (Yang, 2016). Model (4) in Table 7 shows that the coefficient estimate on Inno is significantly positive at the 1% significance level. This verifies the mediating effect of cities’ green technology innovation capability on the CO2 emission reduction rate. In other words, when facing various environmental performance assessment pressures or promotion incentives brought about by the AANR, local officials will strengthen the CO2 emission reduction rate by engaging in green technology innovations. We conclude that the affecting mechanism of the government’s concern for the environment in H2 does not hold, while that of cities’ green technology innovation capability holds.
Table 7. Mechanism test results. Models (1) and (2) scrutinize the mediating effect of the government’s concern for the environment (Gov), while models (3) and (4) show the mediating effect of the cities’ green technology innovations (Inno).
The full sample is divided into east–central and western cities’ subsamples by geographical location. From models (1) and (2) in Table 8, we find that in the western cities, the implementation of the AANR significantly increases the CO2 emission reduction rate in the pilot cities. In contrast, in the east–central cities, the positive effect of the AANR on the CO2 emission reduction rate is not significant. In particular, the western cities show a 1.70% increase in the CO2 emission reduction rate compared to the pre-AANR pilot. The reason for this finding may be that the level of development in the east–central cities is much higher than that in the western cities. This also means that carbon abatement costs are higher in the east–central cities (Jiang et al., 2023). Economic growth in the east–central cities also brings about problems associated with resource consumption and carbon emissions. The governments in the east-central cities may have also realized the importance of environmental protection before those in the western cities. Before the implementation of the AANR, officials in the east-central cities may have already started to deal with pollution problems arising from carbon emissions; thus, the impact of the AANR on the CO2 emission reduction rate is not significant. In contrast to the east-central cities, the western cities have been slower to develop, and local officials may still be developing the economy at the expense of the environment. However, the implementation of the AANR has sounded an alarm for officials in the western cities. Local governments are forced by strong administrative pressure to increase environmental controls to reduce the CO2 emission growth rate. In a word, the AANR has a significant effect on the CO2 emission reduction rate in the western pilot cities.
Table 8. Results of the heterogeneity analyses. Models (1) and (2) depict cities’ geographical heterogeneity. Models (3) and (4) represent the heterogeneity effects of cities’ fiscal pressure.
The difference between local fiscal expenditures and local fiscal revenues is divided by local fiscal revenues, which represents fiscal pressure. We define the sample below the median of the fiscal pressure as low fiscal pressure; otherwise, it is high fiscal pressure. Then, the full sample is divided into high- and low-fiscal pressure subsamples. In the pilot cities with high fiscal pressure, the AANR significantly increases the CO2 emission reduction rate by 2.90% compared to the pre-AANR pilot (Model (3) in Table 8). In contrast, the effect of the AANR on the CO2 emission reduction rate is not significant in the pilot cities with low fiscal pressure (Model (4) in Table 8). One possible reason for this may be that officials in cities with high fiscal pressure focus on developing the regional economy, while cities with low fiscal pressure had sufficient financial resources to support carbon emission management activities before the AANR pilot. When implementing the AANR pilot, officials in the regions with high fiscal pressure started focusing on ecological management due to the incentive mechanisms and inspection pressures induced by the AANR, thus reducing CO2 emissions.
This paper analyzed the panel data of China’s 271 cities from 2005 to 2017 to explore the effect of the AANR on the CO2 emission reduction rate. The AANR is used as a quasi-natural experiment to apply the multi-period DID model to empirically scrutinize the AANR’s CO2 emission reduction effect. Furthermore, a mediation model is adopted to verify the mechanism by which AANR affects CO2 emissions. The main findings are as follows: first, the AANR significantly increases the CO2 emission reduction rate. This result still holds after a series of robustness tests. The AANR is a top-down national ecological and environmental governance policy in China. Although it does not point to decarbonization alone, the empirical results confirm the AANR’s positive externality on CO2 reduction. Second, the mechanism analysis shows that improving cities’ green technology innovation capability is the main mediating mechanism through which the AANR improves the CO2 emission reduction rate in pilot cities. The AANR has limited positive externality that directly contributes to CO2 emission reduction in cities, and the wind vane effect of the AANR policy guides cities to innovate green technologies. High-carbon emitting segments will gradually complete the “trade-in,” thus giving rise to the cities’ CO2 emission reduction. There is no sufficient source of evidence to suggest that the AANR increases the CO2 emission reduction rate through the government’s concern for the environment. Third, the heterogeneity analysis suggests that the AANR plays a more vital role in China’s western cities and cities with high fiscal pressure. The economies of cities in east-central China are more scalable and more costly in terms of carbon emission reduction. Therefore, the AANR’s policy effect has stronger inertia. In addition, cities with high financial pressure tend to do well in AANR policy performance to relieve such pressure. This will send a positive signal to society and help obtain financial support.
The following policy implications emerge from this research: first, the AANR improves the CO2 emission reduction rate and implies that in the context of carbon peaking and carbon neutrality, all Chinese government departments should pursue both central and local carbon emission reduction strategies; establish a scientific carbon governance, analysis, and evaluation system; widen the scope of the AANR; and enhance local officials’ awareness of their carbon emission-related responsibilities. Second, AANR’s CO2 emission reduction effect mainly comes from the mediating effect of cities’ green technology innovations and indicates that local governments should increase financial subsidies, technology subsidies, and tax preferences to mobilize enterprises to engage in green technology innovations while accelerating the innovation platform construction, actively cultivating innovative talent, and promoting the transfer and transformation of innovative technologies to enhance cities’ low-carbon soft power. With the goals of reducing trust and financing costs at both ends of the green technology innovation capital and advancing the efficiency of meso-cities’ green technology innovation mediation, China will gradually build more low-carbon cities through AANR implementation. Third, for cities in east-central and western China and cities with different fiscal pressures in applying the AANR for carbon emission reduction, local governments should implement dynamic and differentiated AANR policies, taking into account the cities’ developmental characteristics and local conditions in a coordinated manner. It is due to the fact that most enterprises in a city have not adopted sustainable business models and do not prioritize endogenous green technological changes driven by research and development (R&D), thus leading to inefficient carbon emission management. Chinese cities should also develop appropriate audit content and standards so that the effect of the AANR is enhanced.
Currently, there are many studies on policy evaluation. However, few studies have evaluated the effect of the AANR on CO2 emissions reduction. The most important contribution of this study is its quantitative evaluation of the effect of the AANR on CO2 emission reduction in China using a multi-period difference-in-differences model. One limitation is that only 271 cities were selected for the research sample due to data limitations. Although limited by the availability of data, the results of this paper provide a quantitative complement for the AANR to assist China in reaching its carbon peaking and carbon neutrality targets. Future research should use a broader sample to deepen our understanding of the effectiveness of the AANR. In addition, this study can be regarded as the first step in analyzing the AANR policy impact on carbon emission reduction. Future research can also investigate the mechanisms in the implementation of the AANR on corporate social responsibility, green innovation, and even business model innovations.
The raw data supporting the conclusion of this article will be made available by the authors, without undue reservation.
LX and YS: conceptualization, data curation, formal analysis, investigation, methodology, software, validation, visualization, and writing–original draft. XL: conceptualization, data curation, funding acquisition, investigation, methodology, project administration, validation, and writing–review and editing.
The author(s) declare that financial support was received for the research, authorship, and/or publication of this article. This research was funded by the Key Scientific Research Project of Hunan Provincial Department of Education, “Research on the Measurement, Impact, and Enhancement Path of Corporate Digital Intelligence Business Model Resilience”, grant number 2023A0320; the General Project of Hunan Provincial Social Science Achievement Review Committee, “Research on Business Model Resilience of Modernized New Hunan Enterprise Construction Led by Chinese Style Modernization,” grant number XSP24YBC190; and University of South China’s School-level Research Project Foundation, “the innovative framework study of corporate investment value,” grant number 220XQD095.
The authors are very grateful to the editors and reviewers for their insightful comments and suggestions.
The authors declare that the research was conducted in the absence of any commercial or financial relationships that could be construed as a potential conflict of interest.
All claims expressed in this article are solely those of the authors and do not necessarily represent those of their affiliated organizations, or those of the publisher, the editors, and the reviewers. Any product that may be evaluated in this article, or claim that may be made by its manufacturer, is not guaranteed or endorsed by the publisher.
Chen, Z. (2023). The impact of natural resource asset separation audit on carbon emissions of industrial enterprises—an empirical study based on 13 prefecture-level cities in Jiangsu Province. Financ. Manage. Res. 40 (1), 60–64.
Fu, Y. (2022). Can natural resource exit audit enhance urban innovation capability? J. Guizhou Univ. Financ. Econ. 219 (4), 52–60.
Gu, H. D., Yang, W. G., and Chen, W. J. (2022). Research on the effect of green technology innovation on urban carbon emissions reduction. Acad. Exp. 268 (3), 120–132.
He, B. C., and Feng, Y. Q. (2021). How does natural resource asset exit audit affect corporate green technology innovation? Ecol. Econ. 37 (5), 192–198.
Hu, J. K., and Wang, Y. M. (2022). Local government competition paradigm shift and carbon emissions performance—empirical evidence from prefecture-level municipal government work reports. Econ 282 (6), 78–87.
Huang, R. B., and Xie, X. J. (2022). Leading cadres’ natural resources assets discharge audit and corporate environmental protection investment. Bus. Econ. Manage. 371 (9), 54–71.
Jiang, H. D., Dong, K. Y., Qing, J., and Teng, Q. (2023). The role of technical change in low-carbon transformation and crises in the electricity market: a CGE analysis with R&D investment. Energ. Econ. 125 (11), 106897. doi:10.1016/j.eneco.2023.106897
Jiang, H. D., Purohit, P., Liang, Q. M., Liu, L. J., and Zhang, Y. F. (2023). Improving the regional deployment of carbon mitigation efforts by incorporating air-quality co-benefits: a multi-provincial analysis of China. Ecol. Econ. 204 (11), 107675. doi:10.1016/j.ecolecon.2022.107675
Kluczek, A., and Olszewski, P. (2017). Energy audits in industrial processes. J. Clean. Prod. 142 (4), 3437–3453. doi:10.1016/j.jclepro.2016.10.123
Kong, L., Price, L., Hasanbeigi, A., Liu, H., and Li, J. (2013). Potential for reducing paper mill energy use and carbon dioxide emissions through plant-wide energy audits: a case study in China. Appl. Energy. 102 (2), 1334–1342. doi:10.1016/j.apenergy.2012.07.013
Kontokosta, Ce., Spiegel-Feld, D., and Papadopoulos, S. (2020). The impact of mandatory energy audits on building energy use. Nat. Energy. 5 (4), 309–316. doi:10.1038/s41560-020-0589-6
Li, B. Y., and Yin, H. T. (2016). The theoretical basis and methods of leading cadres’ natural resources assets out-of-office audit. Audit. Res. 193 (5), 32–37.
Li, Z. D., and Guo, L. (2022). Can leading cadres’ natural resource assets discharge audits promote energy conservation and emissions reduction? J. Nanjing Audit. Univ. 19 (3), 31–39.
Liu, M. H., and Sun, J. P. (2016). Research on the elements of leading cadres’ natural resources assets out-of-office audit. Audit. Econ. Res. 31 (4), 12–20.
Liu, W. J., and Xie, B. S. (2018). Do leading cadres’ natural resources assets exit audits affect corporate surplus management? J. Zhongnan Univ. Econ. Law. 226 (1), 13–23+158.
Liu, Y., Ye, D., Liu, S., and Lan, H. (2023). The effect of China’s leading officials’ accountability audit of natural resources policy on provincial agricultural carbon intensities: the mediating role of technological progress. Environ. Sci. Pollut. Res. 30 (3), 5634–5661. doi:10.1007/s11356-022-22465-3
Shen, W. N., Chai, Z. Y., and Zhang, H. M. (2020). Heterogeneous ecological attention and environmental governance performance—based on the perspective of Beijing-Tianjin-Hebei “Government Work Report”. Soft Sci. 34 (9), 65–71.
Thollander, P., Backlund, S., Trianni, A., and Cagno, E. (2013). Beyond barriers—a case study on driving forces for improved energy efficiency in the foundry industries in Finland, France, Germany, Italy,Poland, Spain, and Sweden. Appl. Energy. 111 (11), 636–643. doi:10.1016/j.apenergy.2013.05.036
Wang, S., Ma, J. H., and Li, Z. H. (2014). The influence of government environmental concern on the efficiency of urban land resource allocation. Econ. Geogr. 42 (12), 186–193.
Wang, X. J., Cheng, Y., Ding, L., and Wang, J. S. (2019). Research on the mechanism of the influence of science and technology innovation on carbon emissions efficiency in countries along the “Belt and Road.”. Soft Sci. 33 (6), 72–78.
Wen, Z. L., and Ye, B. J. (2014). Mediation effect analysis: methodology and model development. Adv. Psychol. Sci. 5, 731–745.
Wu, X., Cao, Q., Tan, X., and Li, L. (2020). The effect of audit of outgoing leading officials’ natural resource accountability on environmental governance: evidence from China. Manage. Audit. J. 35 (9), 1213–1241. doi:10.1108/maj-08-2019-2378
Xiong, H., Li, Y., and He, J. (2022). Construction and application of the evaluation system of natural resources asset accountability audit of officials: a case study of Jiangxi, China. Sustainability 14 (1), 528. doi:10.3390/su14010528
Xue, F., Zhou, M. L., and Liu, J. Q. (2022). Study on the effect of digital infrastructure on reducing carbon emissions: a quasi-natural experiment based on the “broadband China” strategy. South Econ. J. (8), 19–36.
Yan, Y. Z., Cheng, Q. W., Huang, M. G., Lin, Q. H., and Lin, W. H. (2022). Government environmental regulation and corporate ESG performance: evidence from natural resource accountability audits in China. Int. J. Environ. Res. Public Health. 20 (1), 447. doi:10.3390/ijerph20010447
Yang, H. S. (2016). Context and model: the action logic of policy implementation in China. Xuehai (3), 12–17.
Zhang, T. J., and Li, Q. (2021). Discharge audit of natural resource assets and the quality of corporate environmental information disclosure. Financ. Acc. Mon. 902 (10), 115–123.
Zhao, C., Zhu, J. X., Xu, Z. Y., Wang, Y. X., Liu, B., Yuan, L., et al. (2022). The effect of air pollution control auditing on reducing carbon emissions: evidence from China. Int. J. Environ. Res. Public Health. 19 (24), 17019. doi:10.3390/ijerph192417019
Zhu, F. N., and Xu, W. S. (2022). Exploring the issue of leading cadres’ natural resource assets’ discharge audit—a statistical analysis based on the audit reports from 2018–2021. Financ. Account. Commun. 903 (19), 113–120.
Zhu, J. Y., and Li, Y. Y. (2022). Does national audit have a governance role for carbon emissions? Contemp. Financ. Econ. 454 (9), 136–148.
Zhu, J. Y., Li, Y. Y., and Long, J. (2022). Do national audits affect regional innovation capability? an empirical study based on local government innovation preferences. J. Nanjing Audit. Univ. 19 (3), 10–19.
Keywords: accountability audit of natural resources, carbon dioxide emission reduction rate, government concern for the environment, green technology innovation capability, multi-period difference-in-differences model
Citation: Xiong L, Shu Y and Li X (2024) Evaluating the effect of the accountability audit of natural resources on carbon emissions reduction in China. Front. Environ. Sci. 12:1342321. doi: 10.3389/fenvs.2024.1342321
Received: 21 November 2023; Accepted: 18 April 2024;
Published: 06 June 2024.
Edited by:
Faik Bilgili, Erciyes University, TürkiyeReviewed by:
Hong-Dian Jiang, China University of Geosciences, ChinaCopyright © 2024 Xiong, Shu and Li. This is an open-access article distributed under the terms of the Creative Commons Attribution License (CC BY). The use, distribution or reproduction in other forums is permitted, provided the original author(s) and the copyright owner(s) are credited and that the original publication in this journal is cited, in accordance with accepted academic practice. No use, distribution or reproduction is permitted which does not comply with these terms.
*Correspondence: Xuefeng Li, aGVuc2hlcmxAc2luYS5jb20=
Disclaimer: All claims expressed in this article are solely those of the authors and do not necessarily represent those of their affiliated organizations, or those of the publisher, the editors and the reviewers. Any product that may be evaluated in this article or claim that may be made by its manufacturer is not guaranteed or endorsed by the publisher.
Research integrity at Frontiers
Learn more about the work of our research integrity team to safeguard the quality of each article we publish.