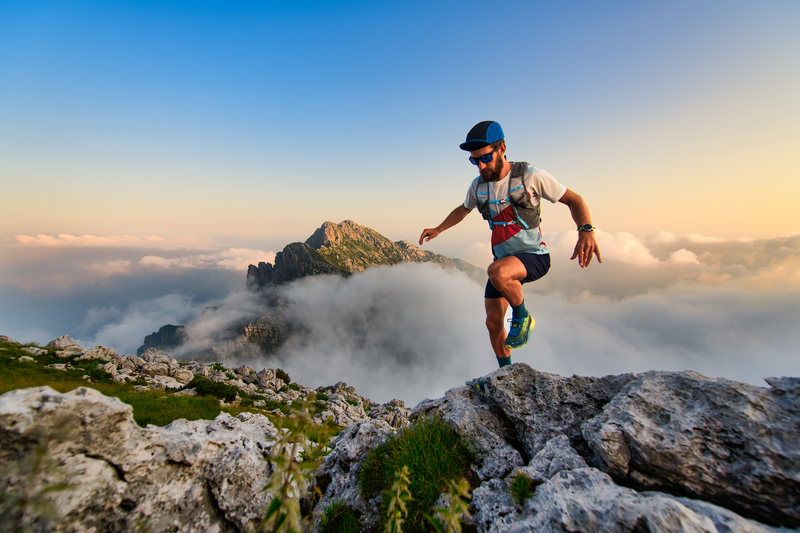
94% of researchers rate our articles as excellent or good
Learn more about the work of our research integrity team to safeguard the quality of each article we publish.
Find out more
ORIGINAL RESEARCH article
Front. Environ. Sci. , 22 March 2024
Sec. Environmental Economics and Management
Volume 12 - 2024 | https://doi.org/10.3389/fenvs.2024.1336803
This article is part of the Research Topic Low-Carbon Economy and Sustainable Development: Driving Force, Synergistic Mechanism, and Implementation Path View all 21 articles
In this paper, panel data from Chinese cities from 2000 to 2020 were used, and the intellectual property pilot was used as a quasi-natural experiment. The double difference model mainly tests the underlying mechanism of the impact of intellectual property rights pilots on urban carbon emissions. This study revealed that IPR pilots play an important role in reducing urban carbon emissions. Heterogeneity experiments reveal that IPR pilots are conducive to reducing carbon emissions in coastal as well as high greening cities and that the underlying mechanism through which IPR pilots affect urban carbon emissions mainly occurs at the level of innovation and the optimization of the industrial structure. In addition, this paper also validates the smoothness of the model by replacing the explanatory variables and performing a placebo test, which further strengthens the reliability of the paper’s conclusions.
In recent years, global climate problems caused by excessive carbon emissions have had negative impacts on human living environments and socioeconomic development, and controlling carbon dioxide emissions has become the consensus of major countries worldwide (Duan and Zhou, 2017). Reducing carbon emissions requires the synergy of technology, environmental awareness and social responsibility, which means that carbon emissions need to be regulated. However, currently, most of the regulation of carbon emissions occurs in the private sector (Reinhard et al., 2023), which is far less efficient and fair than state regulation. Therefore, it is necessary for the State as the main body to formulate effective policies to reduce carbon emissions. According to the World Energy Statistics Yearbook 2021, China’s carbon emissions will increase from 8.83 billion tons in 2011 to 9.90 billion tons in 2021, accounting for a larger proportion of the world’s total carbon emissions; therefore, the conclusions drawn from selecting China’s policies as a quasi-natural experiment can be somewhat representative (Yu et al., 2023).
Curbing carbon emissions is one of the hot topics of current research, and many scholars have focused on the impact of different policies on carbon emissions. Based on the carbon emission trading pilot (CETS) study conducted by selecting firm-level panel data from 2010 to 2018, several scholars have suggested that total factor productivity improvement can be promoted through government policy formulation to achieve the effect of reducing carbon emissions (Pan et al., 2022). Moreover, according to a study on environmental taxes utilizing data from G7 countries from 1994 to 2004, it has been suggested that carbon emissions can be reduced by increasing the cost of corporate emissions through government policies (Doğan et al., 2022). However, another group of scholars has argued that the effect of influencing carbon emissions through policy formulation is limited. For example, carbon tax policies do not necessarily lead to the generation of technological innovations (Fu et al., 2023), and studies based on a large dataset of 32 OECD countries for the period 1997–2018 have shown that the impact of government policies on carbon emissions is unstable at different stages and that there is a rebound effect (Yıldırım et al., 2022).
In addition, studies have shown that the increase in the number of patent applications to prevent climate change is inversely related to carbon dioxide emissions (Yu et al., 2021). It is worth noting that China’s IPR pilot policy was initially implemented in 2012 with the aim of strengthening the economic, cultural and social orientation of IPRs through improved laws and regulations and a sound judicial protection system (Zheng et al., 2023). Since the implementation of the policy, there have been six batches of IPR pilot projects in 77 cities, including 14 subprovincial cities, 54 prefectural-level cities and 9 county-level cities. Therefore, what type of policy effect has the implementation of the IPR pilot program had on carbon emissions? Through what transmission of effects has such an effect been achieved? These questions need to be answered.
The possible marginal contributions of this paper are threefold: 1) Unlike the literature that mainly studies the carbon emission suppression effect of pilot policies on the enterprise or national level, the data in this paper are selected at the city level, integrating the innovation effect and industrial structure optimization effect brought by the pilot policies and exploring their impacts on the city’s carbon emissions. 2) Unlike established studies that conclude that pilot policies produce a single promotion or inhibition, this paper argues that IPR pilot policies have different effects on cities with different levels of greening and different geographic locations, and it develops a heterogeneous study to address these two points. 3) The literature mainly focuses on the innovation effect and industrial structure upgrading effect of the IPR pilot policy (Qian et al., 2023; Song et al., 2023), and few articles directly discuss and study the relationship between the pilot policy and carbon emissions. Therefore, this paper explains the role of the IPR pilot policy in carbon emission reduction on this basis.
The remainder of the study is structured as follows: Section 2 presents the theoretical foundations and research hypotheses; Section 3 presents the research design; Section 4 presents the empirical results and discussion; Section 5 provides further analysis; and Section 6 presents the research conclusions and countermeasure recommendations.
In previous research on the impact of government support on carbon emissions management, scholars have not reached a unified opinion on whether government support can effectively reduce carbon emissions; this paper adopts the perspective of evolutionary economics to explore this issue.
According to the basic idea of evolutionary economics, socioeconomic systems are complex systems that evolve continuously from lower to higher levels (Capellán-Pérez et al., 2014). By summarizing the three industrial revolutions that have taken place, it can be found that the evolution of the socioeconomic system is accompanied by the improvement of the efficiency of resource utilization, and green economy technology, which is mainly embodied by the reduction of carbon emissions, can improve the situation of energy consumption in production and increase the efficiency of energy utilization (Miao et al., 2018). Therefore, the reduction in carbon emissions is one of the important symbols of the evolution of the economic system to an advanced stage. In addition, evolutionary economics also emphasizes that the system is an important force driving economic evolution; institutional innovation plays a key role in this process, and the pilot policy on intellectual property is a typical institutional innovation that reflects the evolution of the property rights system. This will contribute to an increase in patent applications (Lemley and Shapiro, 2005), which in turn will effectively contribute to a reduction in carbon emissions.
From the perspective of evolutionary economics, this article hypothesizes that the IPR pilot policy can have a positive inhibitory effect on carbon emissions through government support, which may be achieved mainly by increasing the emission costs of polluting industries and encouraging the development of green industries. Therefore, Hypothesis 1 is proposed.
H1. Pilot IPR policies can significantly reduce carbon emissions.
The established literature suggests that clearly defining the attribution of intellectual property is the first step in guaranteeing a rational allocation of resources (Restuccia and Rogerson, 2017), affirming that pilot IPR policies have an impact on the level of innovation in cities; however, scholars are divided as to whether IPR protection promotes the development of innovation levels. One group of scholars argues that IPR pilot policies guarantee monopoly gains for inventors (Parra, 2019), make firms more inclined to innovate (Neves et al., 2021), and incentivize firms to invest in R&D (Branstetter et al., 2006), which in turn promotes innovation. On the other hand, another group of scholars argues that IPR pilot policies increase the costs of technology introduction and imitation of innovations (Hurmelinna-Laukkanen and Puumalainen, 2007), thus inhibiting the development of innovation. Although there is some disagreement among scholars on this issue, more scholars still believe that IPR pilots can promote innovation. Moreover, several studies have shown that an increase in innovation level can inhibit carbon emissions (Gerlagh, 2007; Zhou et al., 2010; Wang et al., 2020).
Specifically, for intellectual property rights, the relevant laws and regulations are used to protect the rights of patent inventors. In turn, this policy encourages enterprises and institutions to innovate, and more patented inventions often mean that through the progress of green technology, enterprises can optimize their own production methods, for example, through online queuing, network enrollment, online certificates and other ways to promote “paperless” offices to reduce the level of carbon emissions, and so on, Hypothesis 2 is proposed.
H2. Pilot IPR policies significantly reduce carbon emissions by increasing the level of innovation.
In addition, existing research suggests that IPR pilot policies can improve export trade structures by facilitating the acquisition of competitive advantages by knowledge-intensive firms, thereby contributing to the upgrading of the domestic industrial structure (Markusen et al., 2001). At the same time, academics currently believe that industrial structure upgrading inhibits carbon emissions from energy consumption, technological progress and other paths, and existing studies have shown that industrial structure upgrading inhibits carbon emissions by affecting changes in the structure and efficiency of energy consumption (Brannlund and Persson, 2012).
The specific performance of this mechanism is that the pilot policy of intellectual property rights through the relevant laws and regulations of the clean energy industry to provide financial subsidies to increase the cost of emissions of high energy-consuming industries, which makes the green industry more competitive, to replace the traditional industry by the green industry and to optimize the industrial structure. On the other hand, according to the economic hypothesis, when a green industry shows strong competitiveness and high profits, traditional industry will also be driven by an interest in optimizing its own production methods in the direction of green development to obtain high profits and then upgrading its own industry, which promotes the optimization of the industrial structure. In a city, a higher proportion of green industries represents lower CO2 emissions; thus, Hypothesis 3 is proposed.
H3. Pilot IPR policies significantly reduce carbon emissions by improving the industrial structure.
The underlying mechanism through which the IPR pilot policy reduces regional carbon emissions is illustrated in Figure 1.
This paper uses data from China’s prefecture-level cities from 2000 to 2020 as the research sample. Carbon emission data were obtained from the Carbon Emission Accounts & Datasets (CEADs) database, data on IPR pilots were collated from policy texts, all other data were compiled from the China Urban Statistical Yearbook. Due to missing data for some cities, 14 prefecture-level cities, including Sansha and Danzhou in Hainan Province, Bijie and Tongren in Guizhou Province, Suihua city in Heilongjiang Province, all prefecture-level cities in the Tibet Autonomous Region, Haidong in Qinghai Province, and Turpan and Hami in the Xinjiang Uygur Autonomous Region, were excluded from the study. For some missing value data, interpolation and geometric mean were used to fill in the blanks. Finally, 285 cities with 5985 entries were obtained as data samples.
Explained variable: carbon dioxide emissions (co2): According to Merz et al. (2021), we used the particle swarm optimization-backpropagation (PSO-BP) algorithm for two sets of nighttime lighting data inversion through the nonlinear modeling method so that the carbon emission inversion data and the energy accounting data fit the superiority data is significantly improved to more accurately estimate the carbon emissions of the cities and, ultimately, the carbon dioxide emissions of the various prefectures (logarithmic) to measure the level of carbon emissions in the city.
Explanatory variable: intellectual property pilot (did). According to the relevant policies and data disclosed by the State Intellectual Property Office of China, the IPR pilot variable is a dummy variable, i.e., for pilot cities, it is set to 1 for the year the policy was implemented and subsequent years and 0 for the remaining years.
Innovation level: In this paper, the total number of patents granted in the city is selected to measure the innovation level of the city by taking the logarithm (patent). There are many indicators for measuring the level of innovation, including R&D capital investment, the output value of new products, trademarks and patents. However, it is difficult for R&D capital investment to reflect the actual innovation effectiveness of a region; moreover, there are differences in the statistical standards of the output value of new products, and the data for these indicators are difficult to obtain at the city level. In addition, to improve their core competitiveness, enterprises often obtain patents through technological innovation before producing products and then registering trademarks. Therefore, compared with trademarks, invention patents can better reflect the nature of technological innovation and measure the accumulation of knowledge in a region; therefore, this paper adopts the “total number of patents in logarithmic terms” to reflect the innovation level of a city (Nie et al., 2021).
Industrial structure upgrading: In this paper, the share of tertiary industry in GDP (gdp3) is selected to measure industrial structure upgrading. The optimization and upgrading of the industrial structure reflects the evolution of the regional industrial level from a low level to a high level, which is mainly reflected in the optimization and upgrading of the nonagricultural industrial structure.
Control variables: With reference to the literature, this paper selects the following variables, mainly including fiscal dependence (fin), a logarithmic measure of local general public budget revenue; human capital (student), measured as the number of college students enrolled at the end of the year (taking a logarithmic value); per capita income level (pgdp), defined as the per capita income level taking a logarithmic value, foreign direct investment taking a logarithmic value (fdi); financial institutions’ loan balances taking a logarithmic value (loans); and the logarithm of the total population (people) and wage income (wage) (Hu et al., 2020; Chen et al., 2021).
Table 1 shows the descriptive statistics of the main variables, which shows the mean value of carbon emissions (co2) is 5.719, and the standard deviation is 1.304, which indicates that there is a large difference in carbon emissions between the sample cities. The maximum value of the proportion of tertiary industry (gdp3) is 0.839, and the minimum value is 0.0858, which indicates that there is a large difference in the proportion of tertiary industry between the sample cities. The mean of the total number of patents taken in logarithm (pantent) is 5.998, and the standard deviation is 3.804, indicating that there are significant differences in the level of innovation among the sample cities. The statistical results for the other variables also indicate that there are significant differences between all the variables.
This paper adopts the double difference method to test the impact of the IPR pilot policy on reducing carbon emissions. From the sample of this paper, during the period 2000–2020, a total of 77 cities out of 285 cities were successively approved to become IPR pilot cities, which provides a good quasi-natural experiment for the study of this paper. The 77 cities that were affected by the policy will then become the treatment group, and the remaining cities that have not been approved constitute the control group of the study. Referring to Beck et al. (2010), this paper constructs Model (1).
In Eq. 1, the subscript i denotes the city, t denotes time,
To further verify the mechanism of action, this paper constructs Model (2) and Model (3) on the basis of Model (1).
In Eq. 2 to Eq. 3,
The condition that the treatment group and the control group satisfy the parallel trend in time is an important prerequisite for policy assessment using the double difference method. The double difference model with the same time point of policy implementation is used in the parallel trend test. In the present study, there is inconsistency in the year of implementation of the intellectual property pilot policy, which belongs to the multiperiod double difference model; therefore, the parallel trend test of the traditional double difference model cannot be applied. To solve this problem, this paper refers to the research of Beck et al. (2010) to test the multiperiod double-difference parallel trend. In combination with the sample time horizon, a window period of 10 years is set, i.e., 5 years before the approval of the IPR pilot policy and 5 years after the approval. Based on these data, the dynamic effect coefficients of the policy are analyzed to determine whether the year of the policy shock to the cities as well as the carbon emissions prior to that year satisfy the condition of the parallel trend, and the results are shown in Figure 2.
As shown in Figure 2, before the implementation of the pilot policy on intellectual property rights, the change in the dynamic effect coefficient of the policy basically shows a horizontal trend, indicating that before the implementation of the policy, the trend in carbon emissions is not obvious, and in the year of the implementation of the policy and the following 5 years, the dynamic effect coefficient of the policy shows a rapid downward trend. Therefore, the double-difference model in this paper satisfies the parallel trend condition, which tentatively suggests that the IPR pilot policy may contribute to the reduction in carbon emissions; however, whether this effect is statistically and mathematically significant still requires further testing. In addition, this article finds that the effect on carbon emission suppression is significantly stronger in the first 2 years of the IPR pilot policy, probably due to the increasing returns to scale in the early years of the implementation of the policy and the greater marginal gains for the pilot cities. Although the policy effects in the following years are smaller than those in the first 2 years after the start of implementation, which seems to be in line with the findings of Yıldırım’s (2022) study, the overall trend of the policy effects in the following years shows a gradual increase. This phenomenon may be attributed to the fact that the policy effects of gradual improvement in IPR protection policies continue to increase over time and that the IPR pilot policies can produce lasting benefits.
This paper uses the method of correlation analysis to test whether there is a correlation between the explanatory variables. If there is a failure to pass the test of significance between the variables, this means that there is autocorrelation in the model, and it is necessary to adjust the explanatory variables; the results of the correlation analysis are shown in Table 2. As shown in Table 2, all the explanatory variables pass the test of significance at the 10% level, and the vast majority of the variables pass the test of significance at the 1% level, indicating that there is no significant correlation among the explanatory variables.
On the basis of controlling for both temporal and individual effects, this paper conducts a double difference test of Hypothesis 1 to estimate the direct impact of IPR pilot policies on carbon emissions. Table 3 below shows the main regression results of this paper, in which regression (1) is the regression controlling for only year and individual effect fixed, regression (2) is the regression adding control variables, and its regression coefficient is significantly negative at the 1% level, indicating that the IPR pilot policy can significantly inhibit carbon emissions; thus, Hypothesis H1 is verified. In addition, the coefficients of financial dependence, human capital, per capita income level, the loan balance of financial institutions, the total population at the end of the year, and wage income are all significantly positive, suggesting that city development and an increase in the population may lead to an increase in carbon dioxide emissions (Lei et al., 2011). The coefficient of foreign direct investment is significantly negative, indicating that the utilization of foreign investment can also, to some extent, contribute to the enhancement of local innovation levels through the improvement of the quality of a city’s economic development, and more green technologies are put into use, which in turn reduces carbon dioxide emissions; these findings are the same as those of Mutafoglu’s (2012) study of Turkey.
Among the theoretical analyses and hypotheses, this paper concludes that IPR pilot policies significantly contribute to the reduction of carbon emissions by increasing the level of innovation and that the level of innovation plays a mediating role in the process through which IPR pilot policies affect carbon emissions. In accordance with Models (2) to (3) established in this paper, the mediating effect of the level of innovation will be tested empirically, and the results obtained are shown in Table 4.
As seen from Table 4, in regression (1), the impact of pilot intellectual property policies on carbon emissions is significantly negative at the 1% level, in regression (2), the impact of pilot intellectual property policies on patent inventions is significantly positive at the 5% level, and in regression (3), the regression coefficients of the impact of pilot intellectual property policies and patent inventions on carbon emissions are significantly negative at the 1% level, which proves that the level of innovation plays an intermediary role in the process of the impact of pilot intellectual property policies on the reduction of carbon emissions, and Hypothesis 2 has been verified. This may be because IPR pilot policies can further promote green technological innovation by firms and organizations through the mechanism of protecting patent applications, and encouraging patent innovation is an effective measure for reducing carbon emissions (Wahab et al., 2021), which in turn reduces carbon emissions.
According to our theoretical analysis and hypothesis, this paper proposes that the pilot policy of intellectual property rights significantly contributes to the reduction in carbon emissions by improving the industrial structure and that the industrial structure has an intermediary effect on the process through which the pilot policy of intellectual property rights affects carbon emissions. According to Model (2) to Model (3) established in this paper, the intermediary effect of the industrial structure will be empirically examined, and the results obtained are shown in Table 5.
As seen from Table 5, in regression (1), the regression coefficient of the impact of intellectual property pilot policy on carbon emissions is significantly negative at 1% level, in regression (2), the regression coefficient of the impact of intellectual property pilot policy on the proportion of the tertiary industry is significantly positive at 5% level, and in regression (3), the regression coefficients of the impact of intellectual property pilot policy and the proportion of the tertiary industry on carbon emissions are significantly negative at 1% level, which shows that the industrial structure of the pilot policy on intellectual property rights plays an intermediary effect on the process of the reduction of carbon emissions, and Hypothesis 3 has been verified. This phenomenon may be due to the pilot policy of intellectual property rights through the protection of patent rights to encourage enterprises and institutions to carry out technological innovation. On the one hand, the progress of green technological innovation has initiated the emergence of green emerging industries with lower carbon emissions. Compared with traditional industries, emerging industries with low costs and high profits that replace the original industry to optimize the industrial structure, encourage more green industries which means fewer carbon emissions are generated (Zhang et al., 2014). On the other hand, green technological innovation also helps traditional enterprises optimize their own production methods, leading them to upgrade their industries and change from their original offline production and sales to online e-commerce and other forms, which in turn reduces carbon dioxide emissions (Zhou et al., 2013).
This paper adopts the method of replacing explanatory variables to carry out the robustness test, and the results after re-regression are shown in Table 6. These results show that the empirical results do not largely violate the original conclusions and that the intellectual property pilot policy (did) variable passes the test of significance at the 5% level under the premise of fixed time effects and individual effects, regardless of whether or not the control variables are added. This finding proves that the findings of the present research are robust.
In this paper, we conduct a placebo test by changing the time point of policy implementation, randomly selecting 3 years in advance of policy implementation as the dummy policy time point for the placebo test, generating a new interaction term, did_2, and then re-regressing. The specific regression results are shown in Table 7. The regression coefficient of did_2 on co2 is −0.018, and the t value is −0.746, which fails the significance test. This finding indicates that the policy time point is nonrandom, the policy effect of the IPR model city is significant only for the given time and for the given city, and the above conclusion again verifies the reliability of the benchmark regression results.
Considering that the development degree and trade and export conditions of the inland cities in the sample are relatively backward compared with those of coastal cities, which may lead to different effects of the IPR pilot policy on carbon emissions, this paper divides the sample into a coastal city group and an inland city group according to the geographic attributes of the cities. The heterogeneity of the effect of IPR pilot policies on reducing carbon emissions is further analyzed across subsamples. As shown in Table 8, for the coastal cities, the regression coefficient of the IPR pilot policy on carbon emissions is −0.075, which is significantly negative at the 5% level; however, for the inland cities, the regression coefficient of the IPR pilot policy on carbon emissions is −0.027, which does not reach the level of significance. These results show that the inhibiting effect of IPR pilot cities on carbon emissions is more obvious. These results indicate that the inhibiting effect of IPR pilot cities on carbon emissions is more obvious in the coastal city group.
In addition, this article demonstrates that the coefficient of the fiscal dependence indicator is significant in both coastal and inland cities; however, in coastal cities, higher fiscal dependence suppresses carbon emissions, while in inland cities, high fiscal dependence corresponds to high carbon emissions. This may be because the high fiscal dependence in coastal cities is due to the low proportion of primary industries and the high proportion of emerging industries, resource-based industries and high value-added industries. A greater share of the above industries can inhibit the production of carbon dioxide, which negatively affects local fiscal dependence and carbon emissions. The high financial dependence of inland cities may be due to their lower level of local development, greater dependence on fiscal revenue to maintain GDP, and greater financial dependence, which means that more energy-consuming enterprises discharge emissions; thus, the financial dependence and carbon dioxide emissions in inland cities are positively related. Compared to inland cities, China’s coastal cities develop faster, so the implementation of IPR pilot policies in such areas has an inherent policy advantage as well as the impetus for industrial structure upgrading, while inland cities are relatively backward in terms of development, and the effect of the implementation of the policy is hampered by the development of the policy, which produces regional policy variability (Qian et al., 2018).
Considering that plants consume carbon dioxide during photosynthesis and that areas with higher levels of greening are rich in vegetation and may absorb more carbon dioxide to reduce carbon emissions, this paper divides the samples into a high greening level group and a low greening level group according to the greening level of the city. As shown in Table 9, the regression coefficient of the pilot policy on intellectual property rights on carbon emissions in the group with a high greening level is −0.247, which is significant and negative at the 1% level, while the regression coefficient of the pilot policy on intellectual property rights on carbon emissions in the group with a low greening level is −0.068, which is not significant at the 1% and 5% levels and is significant and negative at the 10% level. The results showed that the inhibitory effect of the pilot policy on intellectual property rights on carbon emissions was more significant in the group with a high greening level. In addition, this article finds that human capital, the loan balance of financial institutions, and population size have significantly more positive impacts on carbon emissions in cities with a low level of greening than in cities with a high level of greening. This phenomenon may occur because cities with a high level of greening have more complete green infrastructure and government policy support, and the capital for urban development is more invested in green technology upgrading and other construction; thus, the relationship between urban development and carbon emissions is not significant. Cities with a low level of greening may represent a city development strategy that focuses more on economic development than on sustainable development, and more funds are invested in energy-consuming enterprises, which aggravates carbon emissions in the development process. Compared with those of cities with low greening levels, the green infrastructure of cities with high greening levels provides a basic guarantee for the carbon reduction effect of the IPR pilot policy, and the tilting of government policy promotes the innovation of green technology and the optimization of the industrial structure, which in turn has a better carbon reduction effect.
Through theoretical analysis and empirical tests, this paper proves the inhibitory effect of the IPR-related pilot policy on carbon emissions and its underlying mechanism; specifically, the IPR-related pilot policy has an obvious inhibitory effect on urban carbon emissions, and furthermore, the carbon reduction effect of the IPR-related pilot policy mainly comes from the improvement of innovation levels and the optimization and upgrading of industrial structures. Moreover, the carbon reduction effect of the IPR pilot policy is significant in coastal cities and cities with a high level of greening but not in inland cities or cities with a low level of greening, which is characterized by obvious heterogeneity.
Based on the above research, the policy implications of this paper are as follows:
First, carbon emissions management can be further enhanced by introducing external investment. This paper finds that as local carbon emission restrictions are more stringent due to carbon emission management policies such as intellectual property rights pilots, external investment enhances the dissemination of green technologies while also being restricted from investing in highly polluting industries, thus facilitating the management of carbon emissions.
Second, carbon emission governance needs to adopt policy coordination to enhance effectiveness. For knowledge-intensive pilot policies, simultaneous implementation of policies encouraging innovation, entrepreneurship, and industrial upgrading can further promote the reduction of carbon emissions. Additionally, the level of greenery significantly influences the effectiveness of carbon reduction policies. Encouraging cities to engage in green infrastructure construction and upgrade green technology alongside the implementation of carbon reduction policies can provide robust support, further enhancing policy effectiveness and strengthening the inhibitory effect on carbon dioxide.
Third, cities in different geographical locations should adopt different strategies for carbon emission governance. Our research indicates that due to the higher quality and greater level of development in coastal cities in China, the effects of implementing carbon reduction policies are more pronounced in these areas. Therefore, for cities with good development quality and a high level of development, implementing carbon reduction policies is more suitable. Leveraging policy spillover effects and prioritizing these cities during pilot projects can influence the coordinated development of less developed regions. For cities with low development quality and a lack of development, prioritizing industrial structure optimization and relevant policy support should precede policy pilot projects for carbon reduction to maximize policy effectiveness.
This study has some limitations, and future research in this area is anticipated to address the following:
First, due to missing data, city data were used in this study. Additionally, because of the lack of data, some cities chose to exclude these data, and some of the data used for interpolation and other methods to compensate for the empirical results were affected to some extent. Second, because this paper discusses China’s relevant policies, compared to scholars in other countries, Chinese scholars in this aspect of the number of studies, in this paper as far as possible to select the research of scholars in different countries still appeared in the research literature of various countries in the imbalance between the proportion of the selection of the problem, We hope that in the future more scholars in other countries will research this policy from different perspectives.
The original contributions presented in the study are included in the article/supplementary material, further inquiries can be directed to the corresponding author.
HH: Conceptualization, Data curation, Formal Analysis, Funding acquisition, Investigation, Methodology, Project administration, Resources, Software, Supervision, Validation, Visualization, Writing–original draft.
The author(s) declare that no financial support was received for the research, authorship, and/or publication of this article.
The author declares that the research was conducted in the absence of any commercial or financial relationships that could be construed as a potential conflict of interest.
All claims expressed in this article are solely those of the authors and do not necessarily represent those of their affiliated organizations, or those of the publisher, the editors and the reviewers. Any product that may be evaluated in this article, or claim that may be made by its manufacturer, is not guaranteed or endorsed by the publisher.
Andreoni, J., and Levinson, A. (2001). The simple analytics of the environmental Kuznets curve. J. public Econ. 80 (2), 269–286. doi:10.1016/s0047-2727(00)00110-9
Beck, T., Levine, R., and Levkov, A. (2010). Big bad banks? The winners and losers from bank deregulation in the United States. J. finance 65 (5), 1637–1667. doi:10.1111/j.1540-6261.2010.01589.x
Brannlund, R., and Persson, L. (2012). To tax, or not to tax: preferences for climate policy attributes. Clim. Policy 12 (6), 704–721. doi:10.1080/14693062.2012.675732
Branstetter, L. G., Fisman, R., and Foley, C. F. (2006). Do stronger intellectual property rights increase international technology transfer? Empirical evidence from US firm-level panel data. Q. J. Econ. 121 (1), 321–349. doi:10.1093/qje/121.1.321
Capellán-Pérez, I., Mediavilla, M., de Castro, C., Carpintero, Ó., and Miguel, L. J. (2014). Fossil fuel depletion and socio-economic scenarios: an integrated approach. Energy 77, 641–666. doi:10.1016/j.energy.2014.09.063
Chen, Z., Zhang, X., and Chen, F. (2021). Do carbon emission trading schemes stimulate green innovation in enterprises? Evidence from China. Technol. Forecast. Soc. Change 168, 120744. doi:10.1016/j.techfore.2021.120744
Doğan, B., Chu, L. K., Ghosh, S., Truong, H. H. D., and Balsalobre-Lorente, D. (2022). How environmental taxes and carbon emissions are related in the G7 economies? Renew. Energy 187, 645–656. doi:10.1016/j.renene.2022.01.077
Duan, M., and Zhou, L. (2017). Key issues in designing China’s national carbon emissions trading system. Econ. Energy & Environ. Policy 6 (2), 55–72. doi:10.5547/2160-5890.6.2.mdua
Fu, K., Li, Y., Mao, H., and Miao, Z. (2023). Firms’ production and green technology strategies: the role of emission asymmetry and carbon taxes. Eur. J. Operational Res. 305 (3), 1100–1112. doi:10.1016/j.ejor.2022.06.024
Gerlagh, R. (2007). Measuring the value of induced technological change. Energy policy 35 (11), 5287–5297. doi:10.1016/j.enpol.2006.01.034
Hu, Y., Ren, S., Wang, Y., and Chen, X. (2020). Can carbon emission trading scheme achieve energy conservation and emission reduction? Evidence from the industrial sector in China. Energy Econ. 85, 104590. doi:10.1016/j.eneco.2019.104590
Hurmelinna-Laukkanen, P., and Puumalainen, K. (2007). Nature and dynamics of appropriability: strategies for appropriating returns on innovation. R&d Manag. 37 (2), 95–112. doi:10.1111/j.1467-9310.2007.00460.x
Lemley, M. A., and Shapiro, C. (2005). Probabilistic patents. J. Econ. Perspect. 19 (2), 75–98. doi:10.1257/0895330054048650
Markusen, J. R. (2001). Contracts, intellectual property rights, and multinational investment in developing countries. J. Int. Econ. 53 (1), 189–204. doi:10.1016/s0022-1996(00)00058-1
Meng, L., Guo, J. E., Chai, J., and Zhang, Z. (2011). China’s regional CO2 emissions: characteristics, inter-regional transfer and emission reduction policies. Energy Policy 39 (10), 6136–6144. doi:10.1016/j.enpol.2011.07.013
Merz, B., Blöschl, G., Vorogushyn, S., Dottori, F., Aerts, J. C., Bates, P., et al. (2021). Causes, impacts and patterns of disastrous river floods. Nat. Rev. Earth Environ. 2 (9), 592–609. doi:10.1038/s43017-021-00195-3
Miao, C., Fang, D., Sun, L., Luo, Q., and Yu, Q. (2018). Driving effect of technology innovation on energy utilization efficiency in strategic emerging industries. J. Clean. Prod. 170, 1177–1184. doi:10.1016/j.jclepro.2017.09.225
Mutafoglu, T. H. (2012). Foreign direct investment, pollution, and economic growth: evidence from Turkey. J. Develo** Societies 28 (3), 281–297. doi:10.1177/0169796x12453780
Neves, P. C., Afonso, O., Silva, D., and Sochirca, E. (2021). The link between intellectual property rights, innovation, and growth: a meta-analysis. Economic Modelling 97, 196–209. doi:10.1016/j.econmod.2021.01.019
Nie, Y., Wan, K., Wu, F., Zou, W., and Chang, T. (2022). Local government competition, development zones and urban green innovation: an empirical study of Chinese cities. Applied Economics Letters 29 (16), 1509–1514. doi:10.1080/13504851.2021.1942426
Pan, A., Zhang, W., Shi, X., and Dai, L. (2022). Climate policy and low-carbon innovation: evidence from low-carbon city pilots in China. Energy Economics 112, 106129. doi:10.1016/j.eneco.2022.106129
Parra, A. (2019). Sequential innovation, patent policy, and the dynamics of the replacement effect. The RAND Journal of Economics 50 (3), 568–590. doi:10.1111/1756-2171.12287
Qian, L., Zhou, Y., Sun, Y., Zhou, Q., and Zhang, M. (2023). Carbon emission reduction effects of intellectual property institution construction in China. Environmental Science and Pollution Research 30 (27), 70569–70591. doi:10.1007/s11356-023-27347-w
Reinhard, C. T., Planavsky, N. J., and Khan, A. (2023). Aligning incentives for carbon dioxide removal. Environmental Research Letters 18 (10), 101001. doi:10.1088/1748-9326/acf591
Restuccia, D., and Rogerson, R. (2017). The causes and costs of misallocation. Journal of Economic Perspectives 31 (3), 151–174. doi:10.1257/jep.31.3.151
Song, Q., Zhong, Q., and Zeng, S. (2023). Intellectual property protection, financial innovation and corporate innovation: evidence from a quasi-natural experiment in China. Emerging Markets Finance and Trade 80.
Wahab, S. (2021). Does technological innovation limit trade-adjusted carbon emissions? Environmental Science and Pollution Research 28 (28), 38043–38053. doi:10.1007/s11356-021-13345-3
Wang, R., Mirza, N., Vasbieva, D. G., Abbas, Q., and Xiong, D. (2020). The nexus of carbon emissions, financial development, renewable energy consumption, and technological innovation: what should be the priorities in light of COP 21 Agreements? Journal of Environmental Management 271, 111027. doi:10.1016/j.jenvman.2020.111027
Xu, Q., Dong, Y. X., and Yang, R. (2018). Urbanization impact on carbon emissions in the Pearl River Delta region: kuznets curve relationships. Journal of Cleaner Production 180, 514–523. doi:10.1016/j.jclepro.2018.01.194
Yıldırım, D. Ç., Esen, Ö., and Yıldırım, S. (2022). The nonlinear effects of environmental innovation on energy sector-based carbon dioxide emissions in OECD countries. Technological Forecasting and Social Change 182, 121800. doi:10.1016/j.techfore.2022.121800
Yu, X., Wan, K., and Du, Q. (2023). Can carbon market policies achieve a “point-to-surface” effect? quasi-experimental evidence from China. Energy Policy 183, 113803. doi:10.1016/j.enpol.2023.113803
Yu, Y., and Zhang, N. (2021). Low-carbon city pilot and carbon emission efficiency: quasi-experimental evidence from China. Energy Economics 96, 105125. doi:10.1016/j.eneco.2021.105125
Zhang, Y. J., Liu, Z., Zhang, H., and Tan, T. D. (2014). The impact of economic growth, industrial structure and urbanization on carbon emission intensity in China. Natural hazards 73, 579–595. doi:10.1007/s11069-014-1091-x
Zheng, P., Li, Z., and Zhuang, Z. (2023). The impact of judicial protection of intellectual property on digital innovation: evidence from China. Finance Research Letters 58, 104257. doi:10.1016/j.frl.2023.104257
Zhou, P., Ang, B. W., and Han, J. Y. (2010). Total factor carbon emission performance: a Malmquist index analysis. Energy Economics 32 (1), 194–201. doi:10.1016/j.eneco.2009.10.003
Keywords: intellectual property pilot, carbon emissions, innovation level, industrial structure, China
Citation: Han H (2024) Can intellectual property rights pilots reduce carbon emissions? Evidence from China. Front. Environ. Sci. 12:1336803. doi: 10.3389/fenvs.2024.1336803
Received: 11 November 2023; Accepted: 12 March 2024;
Published: 22 March 2024.
Edited by:
Jing Lan, Nanjing Agricultural University, ChinaCopyright © 2024 Han. This is an open-access article distributed under the terms of the Creative Commons Attribution License (CC BY). The use, distribution or reproduction in other forums is permitted, provided the original author(s) and the copyright owner(s) are credited and that the original publication in this journal is cited, in accordance with accepted academic practice. No use, distribution or reproduction is permitted which does not comply with these terms.
*Correspondence: Haodong Han, NDczNzAwMjU2QHFxLmNvbQ==
Disclaimer: All claims expressed in this article are solely those of the authors and do not necessarily represent those of their affiliated organizations, or those of the publisher, the editors and the reviewers. Any product that may be evaluated in this article or claim that may be made by its manufacturer is not guaranteed or endorsed by the publisher.
Research integrity at Frontiers
Learn more about the work of our research integrity team to safeguard the quality of each article we publish.