- 1Center for Infectious Disease Dynamics and Department of Biology, The Pennsylvania State University, University Park, PA, United States
- 2Center for Western Weather and Water Extremes, Scripps Institution of Oceanography, University of California, San Diego, La Jolla, CA, United States
- 3School of Integrated Sciences, James Madison University, Harrisonburg, VA, United States
The advancement of computing and information collection technologies has created vast amounts of data describing the world surrounding us. Yet, our planet continues to face unprecedented challenges, including climate change and biodiversity loss. How do we effectively use this information in an open and collaborative way to tackle these planetary-scale issues? We propose a stronger synergistic integration between people and data as we work toward a healthy planet: crowd empowerment in the collection and analysis of data as well as in the identification and implementation of actionable solutions. We use our unique experience as a diverse winning team of the EY Better Working World 2022 global data challenge to illustrate the great potential and current limitations of such an approach. With the objective of fighting biodiversity loss, we were asked to develop a predictive frog multi-species distribution model using occurrence data, gathered from the FrogID app, and environmental conditions. Despite the great potential of global data challenges focused on planetary health, they comprise about 6% of all challenges in the last 5 years. Moreover, though open in principle, in practice, pre-existing disparities limit the inclusion of a diverse crowd. To address existing gaps, we propose practical guidelines to realize the “People and Data” vision: fostering collaboration, seeking funding, enhancing diversity, and ensuring long-term sustainability. In this way, we can tackle the great challenges our world is facing.
Introduction
In the last decades, substantial technological advancements have revolutionized the way we gather data on the world around us. New technologies have made available an incredible amount of information regarding phenomena happening at both the macro- and microscopic scales. The launch of satellites and similar remote-sensing devices in space has allowed the monitoring of our planet from a new perspective and a grander scale (Chuvieco, 2020), providing key information on the status of natural habitats (Corbane et al., 2015) as well as the climate system and its rapid changes (Yang et al., 2013). Next-generation sequencing, metabolomics, and other tools used in the study of molecular processes have enabled significant advances in biomedical and bioinformatic research. For example, advanced nanotechnology allowed for the identification of the complete genome sequence and protein structure of SARS-CoV-2 in record time (Shin et al., 2020). At the same time, computing power and data storage have drastically increased (Nordhaus, 2007; Cai et al., 2016). Online data repositories and cloud-shared big data have become incredibly popular, and these databases are often open-access, i.e., available for anyone without restriction. The latter is an example of the process called “technology democratization”, which refers to rapidly increasing access to technology (Sclove, 1995). The most striking example is the use of smartphones and their applications, which are not only a way to access the internet but are also a tool to collect information through images, audio or video always available in our pockets.
Despite novel data sources, advances in computing power, and increasing access to technology, our world continues to face unprecedented challenges, including climate change, food insecurity, pandemics, environmental pollution, and biodiversity loss. The term “tipping point” has been used recently to identify a big and often irreversible system-wide impact that small changes might have (Gladwell, 2006; Lenton, 2013). Nevertheless, due to our reliance on the many services that ecosystems provide, there is a growing concern over these critical tipping points in ecological systems (Gladwell, 2006; Oliver et al., 2015). The response of ecosystems to environmental changes is rarely gradual and predictable, instead, changes can lead to an abrupt shift into a new catastrophic equilibrium with high societal costs (Scheffer et al., 2001). For example, a shift of equilibria has been observed in vegetation collapse that led to desertification (Reynolds et al., 2007) and in the change of state of shallow lakes from clear to turbid water (Scheffer et al., 1993). Given the high-stakes consequences of climate change, it is crucial to monitor the health of ecosystems and devise strategies to preserve and protect our planet.
How do we effectively use the new technology and the large amount of available information to tackle these planetary-scale issues? We believe the answer lies in a collaborative data-informed approach, that empowers a diverse crowd to develop and implement solutions (Figure 1). There is a large literature across many domains on collective problem-solving approaches. One of the most popular of these concepts is “crowdsourcing”, which was first defined by Howe as a new web-based business model that harnesses the creative solutions of a distributed network of individuals (Howe, 2006). Although crowdsourcing is designed to leverage diverse perspectives and skillsets, these initiatives have historically aimed to create profit-generating products and solutions that benefit companies and usually have little to no gain or further involvement for participants (Brabham, 2008; Doan et al., 2011). Understanding the motivations of participants and engaging those participants is becoming increasingly recognized as important to maximize the utility of crowdsourcing initiatives (Hossain, 2015; Liu, 2017; Cricelli et al., 2022).
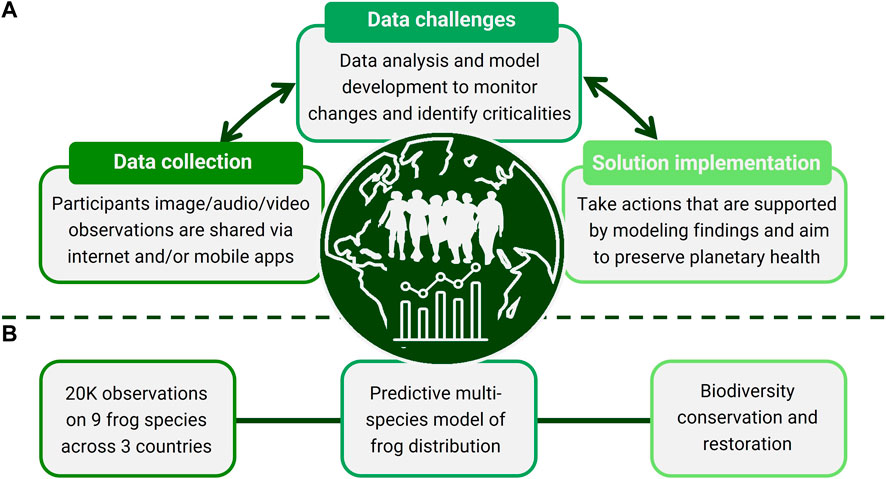
FIGURE 1. The proposed vision: People and Data. (A) Schematic workflow of the proposed crowd-empowerment vision (data collection, data challenges, and solution implementation); (B) Ernst and Young Better Working World 2022 data challenge as an example of our vision.
Science has also exploited a version of the crowdsourcing model called “citizen science”, where volunteer citizens collect large amounts of data to monitor different phenomena that are otherwise resource-intensive to observe, from species distribution (Sullivan et al., 2014) to water quality (Jollymore et al., 2017). Much like crowdsourcing in business domains, this type of crowd involvement in scientific research projects is often limited; data analysis, interpretation of results and potential repercussion on policies and resource management is left to scientists. A complementary approach, community-based monitoring (Khair et al., 2021), engages communities to identify and solve local problems. However, given the pressing and ubiquitous global threats we face, collective involvement in generating large-scale solutions is equally essential.
Here, we propose a shift from crowdsourcing to a more equitable and effective vision of crowd empowerment, integrating proactive participation into each step of the scientific process, from data collection to solution implementation. Our approach requires a strong collaboration between scientists and experts from academia, private companies, and public agencies. Working together, stakeholders from a range of domains can build solutions more impactful than any could achieve alone.
We build from our experience as recent winners of the 2022 open data challenge focused on biodiversity loss hosted by Ernst and Young Global Limited (EY) in collaboration with the United Nations Environmental Programme (UNEP) and the International Union for Conservation of Nature (IUCN). The data challenge was focused on frog species, which are a reliable indicator of ecosystem health, given that areas where frogs have disappeared are usually associated with a degradation in ecosystem stability (Whiles et al., 2006). Specifically, participants were tasked with developing a predictive species distribution model for nine frog species across South Africa, Costa Rica, and Australia starting from occurrence data collected by the FrogID mobile application, developed by the Australian Museum (Figures 1B, 3A, (Rowley et al., 2019)). Indeed, among the 9,000 participants from more than 100 countries, our team was selected by a judging panel with members from NASA, Microsoft, EY, and UNEP as one of the three global winners and awarded a $6,000 prize. The challenge allowed us to apply our technical skills to an important, global problem, but with hindsight, we found ourselves wondering whether our work would have any impact, let alone the impact we had hoped for.
Data collection: citizen science
Citizen science involves volunteers in a scientific research project, a long-standing practice that has become increasingly utilized in the past decade. The primary reasons that people participate in citizen science include the desire to learn new things, to share knowledge with others, and to help the environment, science and other people (West and Pateman, 2016; Maund et al., 2020). These efforts have been enabled by the ability to track the ecological and social impacts of global environmental changes through the internet (Lepczyk et al., 2009; Dickinson et al., 2010). The wide use of technology and the development of targeted open-access apps have facilitated the involvement of the public in ecological research activities, from observing the abundance of organisms to building a data-sharing platform (Silvertown, 2009; Dickinson et al., 2010).
There are several successful examples of citizen science projects that facilitate data collection from a global network of volunteers. In most cases, these efforts provide an open repository where individuals can submit images and audio files accessible via the internet or a ready-to-use mobile phone application. For example, the eBird project collects information about the distribution and abundance of birds (Sullivan et al., 2014), the Evolution MegaLab asks people across Europe to upload information about two distinct-colored snail species (Cepaea nemoralis and C. hortensis) to test predation hypothesis from birds (Worthington et al., 2012), and the extremely popular iNaturalist app is used for biodiversity conservation purposes (Seltzer, 2019).
The dataset on occurrence of frog species that our team analyzed in the EY Better Working World (BWW) Data Challenge originated from FrogID, a popular citizen science project developed by the Australian Museum. FrogID aims to establish a global database to monitor frog distributions over time and to understand how frogs, and the ecosystems they represent, are responding to a changing planet ((Rowley et al., 2019), https://www.frogid.net.au/). Frogs, like other amphibians, are extremely sensitive to changes in their environment due to their biphasic (aquatic and terrestrial) lifestyle, and their dependence on specific environmental conditions for reproduction (Lemckert and Penman, 2012). The decline in frog populations, with already one-third of the 7,000 known species at risk of extinction, has been associated with ecological degradation, indeed frog species represent a key indicator for ecosystem health (Stuart et al., 2004; Hopkins, 2007). FrogID asks users to submit short audio recordings of frog calls to the FrogID smartphone app, which are then processed by experts that identify the frog species that are present (Rowley et al., 2019).
Citizen science has proved to be a useful practice that has the potential to i) increase the temporal and spatial scale of ecological research, ii) build open-access data-sharing platforms that can be used to address multiple scientific questions, iii) create a stronger relationship between scientists and the public, in particular the youngest generation and now an increasing older population with time to spare (Aristeidou et al., 2021), and iv) raise awareness for sensitive topics that have involved environmental change and planetary health. Thus, citizen science represents an important first step in our novel vision of crowd empowerment to solve grand ecological problems.
Data analysis: global data challenges
Many open data challenges have emerged across a variety of fields, from detecting extraterrestrial signals from space sounds to increasing the media visibility of puppy pictures. The increasing popularity of data challenges has been made possible through open access to large datasets and growing coding literacy in almost every domain, from financial marketing to biomedical sciences. Data challenges are open competitions, usually with a monetary prize, that leverage powerful minds across disciplines to analyze a certain dataset and identify solutions to a specific problem, which may otherwise evade field experts. Winners are generally chosen based on the performance of their model using an out-of-sample dataset, which is only available to the data challenge organizers.
This challenge-based format of data analysis has been widely implemented by private companies like Google or H&M. One of the most well-known data challenges was hosted by Netflix in 2006, which promised a $1 million prize to anyone who could achieve one seemingly simple task: improve predictions of user ratings by 10%. After 3 years of intense work and an incredible number of attempts, an expert team led by AT&T research engineers called BellKor’s Pragmatic Chaos won the challenge and the grand prize among more than 50,000 participants. Private corporations are willing to invest in data challenges since the solutions provided by challenge participants have the potential to increase profitability by a margin much larger than the prize itself. In addition, a data challenge represents an incredible opportunity to identify bright talents and investigators.
Despite the potential of open data challenges, very few have addressed planetary health problems. Of data challenges launched in the last 5 years on two of the most popular online repositories (www.kaggle.com and www.drivendata.org, Figure 2), less than 6% of challenges addressed planetary health issues (Figure 2B). Planetary health challenges had comparable participation to those on other topics, with an average of 1,800 competitors per challenge (Figures 2A, C), despite the prize money for planetary health challenges being on average half that of challenges in other fields (less than 30,000 dollars compared to more than 60,000 dollars, Figure 2D). These patterns may represent a recognition of the need to find impactful, data-driven solutions to such pressing global issues.
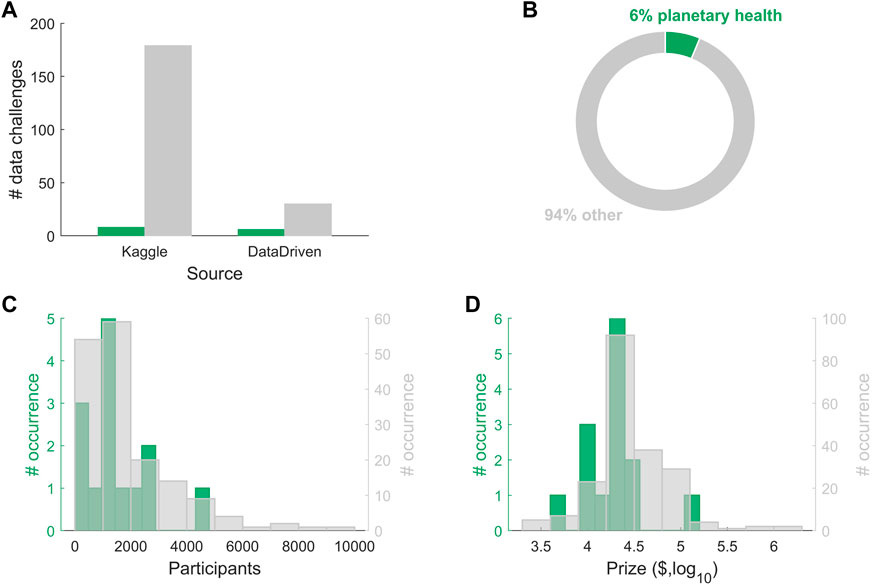
FIGURE 2. The undervalued potential of data challenges in planetary health. (A) Data challenges in planetary health (green) and in other topics (gray) launched in the last 5 years from two popular data challenge repositories Kaggle (https://www.kaggle.com/) and Driven Data (https://www.drivendata.org/); (B) Percentage composition of all data challenges between planetary health and other topics; (C) Data challenge participant distribution of planetary health data challenges (left y-axis) and others (right y-axis); (D) Prize distribution ($, in log10 scale) of planetary health data challenges (left y-axis) and others (right y-axis).
EY Building a Better Working World data challenge on biodiversity loss: methods and results
We were motivated by the drive to make an impact when we participated in the EY Building a Better Working World open data challenge on the topic of biodiversity loss. The challenge aimed to develop species distribution models that could accurately predict the occurrence of frog species based on environmental variables that could be used to inform biodiversity conservation and restoration.
Through the FrogID app data, we were given locations of 20,137 historical occurrences across nine frog species (Agalychnis callidryas, Austrochaperina pluvialis, Chiromantis xerampelina, Crinia glauerti, Crinia signifera, Cyclorana australis, Dendrobates auratus, Litoria fallax and Xenopus laevis) from South Africa, Costa Rica, and Australia. As participants, we were evaluated first on our model’s ability to predict the presence or absence of each frog species at 2,500 other, out-of-sample and unknown, locations (Figures 3A, B). Models were evaluated using the F1-score (Fujino et al., 2008), a measure of predictive performance for binary classification tasks. To develop the model and predict frog occurrence based on environmental variables, EY also provided access to the Microsoft Planetary Computer data repository, through which we had more than 500 GB of potential covariate data (temperature, precipitation, soil moisture, Palmer drought index, evapotranspiration, water deficit, vapor pressure, runoff, terrain and gradient elevation, JRC Water Surface index, normalized difference water index, and normalized difference vegetation index) in the period 2015-2019. We calculated the minimum, maximum and mean for each month of the climate data to include potential seasonality in the effects of climate on frog occurrence.
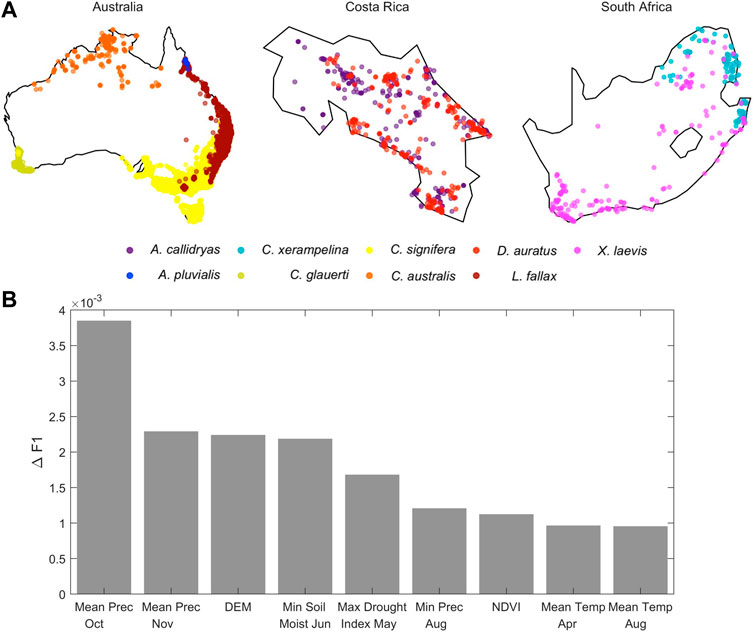
FIGURE 3. Ernst and Young Better Working World data challenge on biodiversity loss. (A) FrogID occurrence observations of different frog species made available during the data challenge; (B) Rank of single predictors, identified from our frog occurrence model exercise, based on their contribution to improve model performance (F1-score).
First, we performed an exploratory analysis to eliminate predictors that were uniformly distributed across all species according to the Kolmogorov-Smirnov test. Then, we implemented an Akaike Information Criterion (AIC) forward stepwise model selection: from the null model, we included predictors and interactions that decrease AIC step by step (Symonds and Moussalli, 2011). Then, we evaluated and included the predictors that improved the F1-score of the in-sample data. At the end of this selection process, our fitted multinomial logistic regression model was able to estimate the probability of occurrence of the nine frog species at a given spatial location and to predict the presence/absence of frog species with an F1-score of 0.88 in the unknown out-of-sample locations.
Our model indicated eleven interpretable predictors (maximum temperature in April and June, minimum precipitation in August, mean precipitation in October and November, minimum soil moisture in June, drought, vegetation cover, and elevation) as key biological features impacting frog occurrence (Figure 3), which are in agreement with previous knowledge on frog breeding and biology (Hero and Morrison, 2004; Whitfield et al., 2016). Our framework was not only able to capture the important predictors differentiating environmental conditions across frog species but also to identify suitable areas for frog species co-occurrence and potential biodiversity hotspots where preservation efforts should be focused. We found that predictors relating to fall precipitation (October and November) to be the most important in differentiating the nine species. The next most important predictors for differentiation were elevation, soil moisture, and drought in summer months (June and May, respectively) (Figure 3B).
After being selected as global semi-finalists based on the predictive accuracy of our model, the challenge involved a second step, where we were asked to submit a short, written report and video presentation emphasizing the novelty of our approach, as well as a documented code repository. The parsimony and biological realism of our model were central to our pitch.
Solution implementation: the missing link
A robust dataset and a strong insight from modeling and data analysis are just the prelude to the most critical part of the proposed process: taking action. Yet, this important step to make a real impact and to close the loop of solution implementation simply fails to happen. There are a multitude of reasons for these implementation difficulties, including practical considerations overlooked by those designing solutions. For example, despite the considerable effort in developing the winning Netflix algorithm, it could not be used due to computational inefficiencies that inhibited implementation and deployment on the Netflix platform (Netflix, 2023). To avoid the development of models that might fail to be implemented, we propose a more cohesive collaboration between data challengers and the agencies implementing their proposed solutions. Constant feedback and an in-depth evaluation of solutions may be more difficult to achieve, but it will be essential to overcome practical constraints that inevitably arise. The accomplishment of winning a data challenge should not mark the end of the scientific road towards the development of optimal solutions nor the collaboration between participants and those implementing solutions. Even more, the entirety of this intellectual and cooperative process would benefit from being fully open-sourced.
Our experience in the EY BWW open data challenge provides an example. We were told our model scripts would be passed to UNEP and IUCN, and we were excited by the potential for our winning model to guide and support on-the-ground restoration and conservation policies. After winning, however, we have no way to track if and how our model is used. Moreover, registration for the data challenge required we agree that all intellectual property belonged to EY, so the model is not freely accessible. This not only prevents collaborative learning and iterative improvement between conservation agencies, the FrogID team and us, the model developers, but it also limits the application of our approach to other domains. Our experience advocates open-sourced collaboration across multiple stakeholders.
Involving data challenge participants and a broader crowd into solution identification and implementation represents a key resource whose potential is often underestimated. A successful example comes from the Project Milkweed (Xerces Society in collaboration with the native seed industry (Borders and Lee-Mäder, 2015)) to fight the decline of monarch butterflies, a key species for pollination and food security. Thanks to the planting of native milkweeds in strategic areas, which provide the nectar nourishment and breeding sites for monarchs but also produce colorful and aesthetic flowers, it is possible to guarantee the ecological corridors for monarch breeding, stopping their population decline (Landis, 2014; Borders and Lee-Mäder, 2015; Lewandowski and Oberhauser, 2017). Data challenges and crowd empowerment have the potential to enhance ecosystem health via data-informed conservation and restoration strategies protect fragile ecosystems and biodiversity, especially in residential and urban landscapes that not only represent the living areas for most people but are also facing urban sprawl and green space disappearance (Cooper et al., 2007).
Discussion
The big challenges that our world is facing ask for a collective effort toward sustainable solutions that safeguard planetary health. The proactive participation of a larger, more empowered crowd from data collection to solution implementation offers great promise. Such “People and Data”-centered approach can better i) monitor the health of ecosystems, ii) advance our understanding of how anthropogenic and environmental disturbances affect our planet, iii) identify criticalities and problematic areas where intervention is needed and iv) guide policy in decision making and interventions (Figure 1). Specifically, we have highlighted here the great potential of citizen science strategies that empower people to participate in the collection of large datasets and in the possibilities of data challenges to develop data-informed mathematical modeling tools (DeWalt, 2024), which are incredibly popular in the private sector and underused for planetary health topics (Figure 2). To achieve the “People and Data” vision, we propose the practical guidelines reported in Box 1 and discussed below.
Solutions to global problems will require us to go beyond individual actions and to act as a community in an open collaborative framework. Collaboration is powerful because of the unique opportunities created by the synergies of bringing multiple groups together, not only creating teams with diverse skills and talents but also connecting stakeholders that have different roles in our society. Our experience with the EY data challenge provided a forum to bring together our diverse skills, across our training in biology, computer science, environmental engineering, neuroscience, mathematics, and geoinformatics. The challenge also represented an opportunity to bring to bear a range of computational techniques to a real-world problem. Using our expertise as biologists, we were able to connect these techniques with an understanding of frog biology to provide a comprehensive solution. More importantly, the data challenge leveraged participation across a range of sectors, including the citizen science FrogID team (Australian Museum of Science), private companies (EY, Microsoft), environmental agencies (IUCN, UNEP, NASA) and academia (with the involvement of students from universities all over the world). Important to the implementation of our vision will be identifying the funding sources of this large collaborative approach. Large foundations should cooperate with environmental agencies to collectively identify such global-scale projects and fund each step for their successful realization. Acting as a community could encourage parties from both the public and the private sectors to bring together resources, creating new opportunities to attract donors and economic supporters.
Despite the great promise of our approach, there are important limitations that will need to be overcome. First, pre-existing disparities such as unequal access to technology and quantitative training usually limit the inclusion of a diverse crowd. For example, among the winners of the EY data challenge in 2022, we were the only majority female team and the only team composed of diverse nationalities (China, Italy, US and Vietnam), despite efforts on behalf of EY to promote inclusion. Such disparities have been identified in other global environmental crowd-sourcing initiatives (Gellers, 2016). Efforts toward a more open and inclusive collaboration should be taken, such as providing coding tutorials and targeted training. Second, we acknowledge the difficulty in designing data challenges that can lead to scientifically sound findings and global impact. Data challenges often focus on a simplified representation of more complex issues, which could have drastic consequences on model applicability. Throughout the development of our model, we realized the tension between optimizing predictability on out-of-sample locations and identifying deep insights about frog biology that matter for conservation. Involving managers in the design of the data challenge and including model interpretability into evaluation criteria (like in our challenge) is crucial for models to have impact.
Global, planetary health challenges call for collective action at a large scale. We advocate for a stronger cooperation among different stakeholders, including private industries, governmental agencies, academia, and local communities. Realizing the vision of “People and Data”, we can collectively find solutions to these great challenges and build a better world.
BOX 1 How do we realize the “People and Data” vision?
• Fostering collaboration: A coordination team should be responsible for designing data challenges to align the interests of a diverse set of stakeholders and seeing solutions are implemented; within-community stakeholders should be at the center of these collaborations.
• Seeking funding: Planetary health focused data challenges should become common practice, which requires support. In addition to bringing together funds from public and private sources, large foundations should support the broader “People and Data” infrastructure and the end-to-end implementation of individual projects.
• Enhancing diversity: Careful attention should be paid to barriers that inhibit participation; creative solutions to improve access to technology, recruit underrepresented participants, and build quantitative training will be important.
• Broadening access to solutions: Solutions and scientific insights generated through planetary health open data challenges should be openly accessible to ensure insights can be improved, extended, and applied to new systems.
• Ensuring long-term sustainability: Building and maintaining an infrastructure to support open data challenges will allow the “People and Data” framework to easily be applied to new questions; doing so further enables learning across projects, engagement of new stakeholders, and proposal of innovative initiatives.
Data availability statement
The data analyzed in this study is subject to the following licenses/restrictions: The FrogID™ data used with the permission of the Australian Museum and Dr. Jodi Rowley. Requests to access these datasets should be directed to https://www.frogid.net.au/.
Author contributions
CV: Conceptualization, Formal Analysis, Investigation, Methodology, Supervision, Visualization, Writing–original draft, Writing–review and editing. EH: Conceptualization, Formal Analysis, Investigation, Methodology, Supervision, Visualization, Writing–original draft, Writing–review and editing. FY: Conceptualization, Formal Analysis, Investigation, Methodology, Visualization, Writing–original draft, Writing–review and editing. T-AT: Conceptualization, Formal Analysis, Investigation, Methodology, Supervision, Visualization, Writing–original draft, Writing–review and editing. WH: Conceptualization, Formal Analysis, Investigation, Methodology, Supervision, Visualization, Writing–original draft, Writing–review and editing.
Funding
The author(s) declare that no financial support was received for the research, authorship, and/or publication of this article.
Acknowledgments
The FrogID™ data used with the permission of the Australian Museum and Dr. Jodi Rowley. We deeply thank Dr. Peter Hudson for his support and guidance throughout the conceptualization and drafting of this perspective. We acknowledge the Department of Biology and the Huck Institutes of the Life Sciences at Penn State for their constant support. Also, we would like to thank our advisors Dr. Maciej Boni, Dr. Isabella Cattadori and Dr. Katriona Shea. Special thanks to Cole Hons for his insightful conversations and intellectual contributions that influenced the development of this manuscript. We would like to thank Ernst and Young for hosting the data challenge, providing us with such wonderful collaborative experience and reflections on the solutions of global challenges.
Conflict of interest
The authors declare that the research was conducted in the absence of any commercial or financial relationships that could be construed as a potential conflict of interest.
Publisher’s note
All claims expressed in this article are solely those of the authors and do not necessarily represent those of their affiliated organizations, or those of the publisher, the editors and the reviewers. Any product that may be evaluated in this article, or claim that may be made by its manufacturer, is not guaranteed or endorsed by the publisher.
References
Aristeidou, M., Herodotou, C., Ballard, H. L., Young, A. N., Miller, A. E., Higgins, L., et al. (2021). Exploring the participation of young citizen scientists in scientific research: the case of iNaturalist. PloS One 16, e0245682. doi:10.1371/journal.pone.0245682
Borders, B., and Lee-Mäder, B. B. (2015). Project milkweed. Monarchs chang. World Biol. Conserv. Iconic Butterfly, 190–196.
Brabham, D. C. (2008). Crowdsourcing as a model for problem solving: an introduction and cases. Convergence 14, 75–90. doi:10.1177/1354856507084420
Cai, H., Xu, B., Jiang, L., and Vasilakos, A. V. (2016). IoT-based big data storage systems in cloud computing: perspectives and challenges. IEEE Internet Things J. 4, 75–87. doi:10.1109/jiot.2016.2619369
Chuvieco, E. (2020). Fundamentals of satellite remote sensing: an environmental approach. USA: CRC Press.
Cooper, C. B., Dickinson, J., Phillips, T., and Bonney, R. (2007). Citizen science as a tool for conservation in residential ecosystems. Ecol. Soc. 12, art11. doi:10.5751/es-02197-120211
Corbane, C., Lang, S., Pipkins, K., Alleaume, S., Deshayes, M., Millán, V. E. G., et al. (2015). Remote sensing for mapping natural habitats and their conservation status–New opportunities and challenges. Int. J. Appl. Earth Obs. Geoinformation 37, 7–16. doi:10.1016/j.jag.2014.11.005
Cricelli, L., Grimaldi, M., and Vermicelli, S. (2022). Crowdsourcing and open innovation: a systematic literature review, an integrated framework and a research agenda. Rev. Manag. Sci. 16, 1269–1310. doi:10.1007/s11846-021-00482-9
Dickinson, J. L., Zuckerberg, B., and Bonter, D. N. (2010). Citizen science as an ecological research tool: challenges and benefits. Annu. Rev. Ecol. Evol. Syst. 41, 149–172. doi:10.1146/annurev-ecolsys-102209-144636
Doan, A., Ramakrishnan, R., and Halevy, A. Y. (2011). Crowdsourcing systems on the world-wide web. Commun. ACM 54, 86–96. doi:10.1145/1924421.1924442
Fujino, A., Isozaki, H., and Suzuki, J. (2008). “Multi-label text categorization with model combination based on f1-score maximization,” in Proceedings of the Third International Joint Conference on Natural Language Processing, USA, June 23 - 26, 2002 (Springer).
Gellers, J. C. (2016). Crowdsourcing global governance: sustainable development goals, civil society, and the pursuit of democratic legitimacy. Int. Environ. Agreem. Polit. Law Econ. 16, 415–432. doi:10.1007/s10784-016-9322-0
Hero, J., and Morrison, C. (2004). Frog declines in Australia: global implications. Herpetol. J. 14, 175–186.
Hopkins, W. A. (2007). Amphibians as models for studying environmental change. ILAR J. 48, 270–277. doi:10.1093/ilar.48.3.270
Hossain, M. (2015). Crowdsourcing in business and management disciplines: an integrative literature review. J. Glob. Entrep. Res. 5, 21. doi:10.1186/s40497-015-0039-2
Howe, J. (2006). The rise of crowdsourcing, Wired: Available at: http://www.wired.com/wired/archive/14.06/crowds.html.
Jollymore, A., Haines, M. J., Satterfield, T., and Johnson, M. S. (2017). Citizen science for water quality monitoring: data implications of citizen perspectives. J. Environ. Manage. 200, 456–467. doi:10.1016/j.jenvman.2017.05.083
Khair, N. K. M., Lee, K. E., and Mokhtar, M. (2021). Community-based monitoring for environmental sustainability: a review of characteristics and the synthesis of criteria. J. Environ. Manage. 289, 112491. doi:10.1016/j.jenvman.2021.112491
Landis, T. D. (2014). Monarch waystations: propagating native plants to create travel corridors for migrating monarch butterflies. Native Plants J. 15, 5–16. doi:10.3368/npj.15.1.5
Lemckert, F., and Penman, T. (2012). Climate change and Australia’s frogs: how much do we need to worry. Wildl. Clim. Change Robust Conserv. Strateg. Aust. Fauna R. Zool. Soc. N. S. W. Mosman Aust., 92–98.
Lenton, T. M. (2013). Environmental tipping points. Annu. Rev. Environ. Resour. 38, 1–29. doi:10.1146/annurev-environ-102511-084654
Lepczyk, C. A., Boyle, O. D., Vargo, T. L., Gould, P., Jordan, R., Liebenberg, L., et al. (2009). Symposium 18: citizen science in ecology: the intersection of research and education. Bull. Ecol. Soc. Am. 90, 308–317. doi:10.1890/0012-9623-90.3.308
Lewandowski, E. J., and Oberhauser, K. S. (2017). Contributions of citizen scientists and habitat volunteers to monarch butterfly conservation. Hum. Dimens. Wildl. 22, 55–70. doi:10.1080/10871209.2017.1250293
Liu, H. K. (2017). Crowdsourcing government: lessons from multiple disciplines. Public Adm. Rev. 77, 656–667. doi:10.1111/puar.12808
Maund, P. R., Irvine, K. N., Lawson, B., Steadman, J., Risely, K., Cunningham, A. A., et al. (2020). What motivates the masses: understanding why people contribute to conservation citizen science projects. Biol. Conserv. 246, 108587. doi:10.1016/j.biocon.2020.108587
Netflix (2023). Netflix technology blog. Available at: https://netflixtechblog.com/netflix-recommendations-beyond-the-5-stars-part-1-55838468f429 (Accessed October 3, 23).
Nordhaus, W. D. (2007). Two centuries of productivity growth in computing. J. Econ. Hist. 67, 128–159. doi:10.1017/s0022050707000058
Oliver, T. H., Heard, M. S., Isaac, N. J., Roy, D. B., Procter, D., Eigenbrod, F., et al. (2015). Biodiversity and resilience of ecosystem functions. Trends Ecol. Evol. 30, 673–684. doi:10.1016/j.tree.2015.08.009
Reynolds, J. F., Smith, D. M. S., Lambin, E. F., Turner, B. L., Mortimore, M., Batterbury, S. P., et al. (2007). Global desertification: building a science for dryland development. science 316, 847–851. doi:10.1126/science.1131634
Rowley, J. J., Callaghan, C. T., Cutajar, T., Portway, C., Potter, K., Mahony, S., et al. (2019). FrogID: citizen scientists provide validated biodiversity data on frogs of Australia. Herpetol. Conserv. Biol. 14, 155–170.
Scheffer, M., Carpenter, S., Foley, J. A., Folke, C., and Walker, B. (2001). Catastrophic shifts in ecosystems. Nature 413, 591–596. doi:10.1038/35098000
Scheffer, M., Hosper, S. H., Meijer, M. L., Moss, B., and Jeppesen, E. (1993). Alternative equilibria in shallow lakes. Trends Ecol. Evol. 8, 275–279. doi:10.1016/0169-5347(93)90254-m
Seltzer, C. (2019). Making biodiversity data social, shareable, and scalable: reflections on iNaturalist and citizen science. Biodivers. Inf. Sci. Stand.
Shin, M. D., Shukla, S., Chung, Y. H., Beiss, V., Chan, S. K., Ortega-Rivera, O. A., et al. (2020). COVID-19 vaccine development and a potential nanomaterial path forward. Nat. Nanotechnol. 15, 646–655. doi:10.1038/s41565-020-0737-y
Silvertown, J. (2009). A new dawn for citizen science. Trends Ecol. Evol. 24, 467–471. doi:10.1016/j.tree.2009.03.017
Stuart, S. N., Chanson, J. S., Cox, N. A., Young, B. E., Rodrigues, A. S. L., Fischman, D. L., et al. (2004). Status and trends of amphibian declines and extinctions worldwide. Science 306, 1783–1786. doi:10.1126/science.1103538
Sullivan, B. L., Aycrigg, J. L., Barry, J. H., Bonney, R. E., Bruns, N., Cooper, C. B., et al. (2014). The eBird enterprise: an integrated approach to development and application of citizen science. Biol. Conserv. 169, 31–40. doi:10.1016/j.biocon.2013.11.003
Symonds, M. R. E., and Moussalli, A. (2011). A brief guide to model selection, multimodel inference and model averaging in behavioural ecology using Akaike’s information criterion. Behav. Ecol. Sociobiol. 65, 13–21. doi:10.1007/s00265-010-1037-6
West, S. E., and Pateman, R. M. (2016). Recruiting and retaining participants in citizen science: what can be learned from the volunteering literature? Citiz. Sci. Theory Pract. 1, 15. doi:10.5334/cstp.8
Whiles, M. R., Lips, K. R., Pringle, C. M., Kilham, S. S., Bixby, R. J., Brenes, R., et al. (2006). The effects of amphibian population declines on the structure and function of Neotropical stream ecosystems. Front. Ecol. Environ. 4, 27–34. doi:10.1890/1540-9295(2006)004[0027:teoapd]2.0.co;2
Whitfield, S. M., Lips, K. R., and Donnelly, M. A. (2016). Amphibian decline and conservation in Central America. Copeia 104, 351–379. doi:10.1643/ch-15-300
Worthington, J. P., Silvertown, J., Cook, L., Cameron, R., Dodd, M., Greenwood, R. M., et al. (2012). Evolution MegaLab: a case study in citizen science methods. Methods Ecol. Evol. 3, 303–309. doi:10.1111/j.2041-210x.2011.00164.x
Keywords: crowd empowerment, global data challenges, big data, planetary health, collaboration, data-informed solutions
Citation: Vanalli C, Howerton E, Yang F, Tran TN-A and Hu W (2024) People and Data: solving planetary challenges together. Front. Environ. Sci. 12:1332844. doi: 10.3389/fenvs.2024.1332844
Received: 03 November 2023; Accepted: 22 January 2024;
Published: 07 February 2024.
Edited by:
Dan Rubenstein, Princeton University, United StatesReviewed by:
Margaret Gold, Leiden University, NetherlandsCopyright © 2024 Vanalli, Howerton, Yang, Tran and Hu. This is an open-access article distributed under the terms of the Creative Commons Attribution License (CC BY). The use, distribution or reproduction in other forums is permitted, provided the original author(s) and the copyright owner(s) are credited and that the original publication in this journal is cited, in accordance with accepted academic practice. No use, distribution or reproduction is permitted which does not comply with these terms.
*Correspondence: Chiara Vanalli, ckv5097@psu.edu