- 1School of Management, Northwestern Polytechnical University, Xi’an, China
- 2School of Management, Shenyang Jianzhu University, Shenyang, China
The importance of pollutant abatement has been steadily growing in recent times, prompting an increased focus on developing effective regulatory mechanisms. This paper introduces a novel approach by combining theories of evolutionary games and opinion dynamics to formulate a coevolution model of game and preference. Recognizing the challenges posed by limited supervision ability and enterprises’ heterogeneous risk preferences, we propose a smart supervision mechanism. This mechanism incorporates the concepts of whitelist capability and observation period to establish intelligent supervision. Simulation results demonstrate the regulator’s ability to accurately discern enterprises’ preferences based on decision-making differences. The smart supervision mechanism proves to be more effective in achieving pollutant abatement goals compared to random supervision. Furthermore, our findings indicate that with higher supervision ability, increasing whitelist capability enhances cooperation rates. Conversely, lower supervision ability necessitates a shorter observation period and increased whitelist capability to achieve optimal pollutant abatement results. The study highlights that enterprises with a high cooperation rate experience more significant benefits, while risk-seeking enterprises benefit less due to heightened regulator attention at the same cooperation rate.
1 Introduction
The industrialization process leads to a significant surge in pollutant emissions, particularly pronounced in developing countries (Carraro and Sgobbi, 2008). This surge in pollutant emissions contributes to a myriad of issues encompassing environmental, healthcare, and social concerns (Wang and Yang, 2016). Consequently, addressing pollutant emissions becomes an imperative matter. Various approaches to achieve pollutant abatement have been explored. Globally, certain environmental organizations are dedicated to funding initiatives aimed at reducing pollution and promoting global sustainable development. However, these efforts often lack mandatory mechanisms. On a regional governmental level, pollutant abatement is enforced by government regulators through the imposition of punitive taxes or the closure of non-compliant enterprises (Wu et al., 2020).
Evolutionary game theory has emerged as a prominent framework in recent years for studying pollutant abatement, offering a powerful tool to analyze the dynamics between regulators and enterprises (Xu et al., 2021; Gu et al., 2022; Ning et al., 2022). This paper aims to delve into the practical application of evolutionary game theory in the context of pollutant reduction, specifically examining how businesses evolve their cooperative strategies in the face of collective risks, with a particular focus on navigating the societal dilemma between economic interests and environmental protection. By synthesizing supporting literature, we will conduct an in-depth exploration of the tangible impacts of evolutionary game theory in addressing the complex interactions between regulatory agencies and businesses. The study seeks to unveil the potential contributions of evolutionary game theory in propelling more effective and sustainable solutions for pollutant abatement, providing novel insights for future research endeavors.
The system of regional pollutant abatement contains regulators and a bunch of enterprises. In this system, enterprises cooperate on pollutant abatement with regulators’ supervision under collective risk (Liu et al., 2023; Yu et al., 2023; Zeng et al., 2023). Enterprises, especially energy-intensive ones, are the central responsible bodies of pollutant emissions. However, enterprises put the economic benefits in a more critical position than environmental protection (Bahel, 2018). Achieving more economic benefits requires higher consumption of resources leading to more pollutant emissions, which is a social dilemma putting back cooperation willingness of pollutant abatement (Chen et al., 2014). Due to the lack of supervision, the failure of pollutant abatement occurs in some regions, making human beings suffer from environmental deterioration (Vasconcelos et al., 2014). To some extent, regulators’ supervision promotes enterprises’ pollutant abatement (Liu and Li, 2019). Common supervision ways include rewarding and punishing (Wang et al., 2010; Jeon et al., 2015; Wang and Shi, 2019). However, it is hard for regulators to supervise all enterprises in the region considering the supervision ability. In the absence of supervision, some enterprises may take risks to emit pollutants lured by higher benefits (Xu et al., 2019). Thus, achieving a better effect under limited supervision ability is a valuable research issue.
In reality, different enterprises have heterogeneous opinions or attitudes, namely, preferences, towards pollutant abatement. Some enterprises prefer abating pollution to avoid punishment, and others prefer adopting a non-abatement strategy for potentially higher benefits when regulators fail to supervise them. The hypothesis of bounded rationality holds that decision-makers cannot make completely rational decisions when facing complex problems due to incomplete information, inconsistent preferences, and limited cognitive ability (Di and Liu, 2016). Moreover, due to the influence of the social environment, enterprises’ preferences may change (Acemoglu and Ozdaglar, 2011). Opinion dynamics hold that others influence an agent’s opinion in the system. Agents may change their opinions by comparing with other agents’ performance (Mäs et al., 2010). As a result, both enterprises’ strategies and preferences coevolve in pollutant abatement games. Besides, heterogeneous risk preferences cause behavior differences between enterprises. Regulators should consider the heterogeneous risk preferences when selecting supervised enterprises. Thus, a new supervision mechanism is proposed considering the limited supervision ability and heterogeneous risk preference.
The main contributions of this paper are as follows. First, we consider the enterprises’ preference evolution of pollutant abatement based on opinion dynamics. The coevolution of game and preference runs synchronously. This innovation assists regulators in supervising enterprises pertinently according to the agents’ heterogeneous preferences. Thus, our approach promises to complement the research on pollution control in the case of bounded rationality. Second, we propose a smart supervision mechanism considering heterogeneous risk preference and limited supervision ability and compare the effect of the smart supervision mechanism with the random supervision mechanism: 1) regulators supervise enterprises randomly under the random supervision mechanism; 2) regulators mainly supervise enterprises with a negative attitude towards pollutant abatement under the smart supervision mechanism. Thus, this paper provides new insight into reforming the supervision mechanism to achieve a better abatement effect.
The remainder of this paper is organized as follows. The next section reviews the literature on supervision and preference. In Section 3, we construct the game model for pollutant abatement with two different supervision mechanisms based on preference evolution. Then, Section 4 explores and compares two different supervision mechanisms under different supervision abilities by simulations. In Section 5, we give conclusions and discuss policy recommendations and limitations.
2 Literature review
2.1 Punishment and supervision mechanism
The evolutionary game is a standard tool for studying social dilemmas. The issue of pollutant abatement involving regulators and enterprises using evolutionary game theory has been explored recently (Zu et al., 2018; Wang and Shi, 2019; Zhang et al., 2019; Kou et al., 2021; Zhou et al., 2022). Enterprises select the abatement or non-abatement considering benefits maximization and the risk of punishment (Chang et al., 2015). More recent works studied the threshold public goods game (TPGG) by increasing the collective risk to promote cooperation. In TPGG, enterprises need to achieve common goals to avoid collective risk (Santos and Pacheco, 2011; Tavoni et al., 2011). However, maximizing self-benefits is contrary to common goals, which causes the collapse of cooperation. Thus, punishment is introduced to the TPGG model, and enterprises cooperate to avoid potential losses caused by punishment (Barrett, 2013; Schmidt, 2017).
Some research discusses the enterprises’ strategy selection given the punishment (Zu et al., 2018; Wang and Shi, 2019). For example, Gupta et al. investigated the relationship between environmental protection supervision and factory compliance in developing countries, and proved that supervision and factory pollution discharge are interrelated (Gupta et al., 2019). Under the punishment, Jiao et al. considered an evolutionary game between governments and enterprises with carbon emission constraints. Moreover, some research discusses enterprises’ reactions towards different punishment methods (Jiao et al., 2017). Common forms of punishment include punitive tax, fining, and even shutdown. For example, considering static and dynamic punishment, Wang and Shi constructed an evolutionary game model of industrial pollution between local government and enterprises (Wang and Shi, 2019).
Punishment is a critical method of regulators’ supervision. Recent studies focus on punishment methods considering collective risk and risk preferences, such as collective fining and random fining (Alpízar et al., 2004; Camacho-Cuena and Requate, 2012). Under collective fining, regulators punish the whole polluters if the pollutant emission exceeds the level set by regulators. In contrast, one potential polluter is chosen randomly under random fining, irrespective of being one of the actual polluters. For example, Alpízar et al. presented an experimental study of two different punishment methods: collective and random fining to supervise pollution behavior (Alpízar et al., 2004). Camacho-Cuena and Requate proposed an experimental study including random fining and collective fining considering risk preferences, and the result showed that risk-seeking agents worsen the effect of pollutant abatement (Camacho-Cuena and Requate, 2012). However, the above punishment methods have some deficiencies. First, collective fining does not consider the limited supervision ability and the difficulty of the actual implementation. Second, random fining is hard to optimize the limited supervision resources due to the lack of supervision pertinence.
Some supervision mechanisms based on various factors have been proposed. Fan et al. proposed three supervision strategies for enterprises that apply for subsidies considering the supervision cost (Fan et al., 2017). Xu et al. built an evolutionary game model of third-party supervision, including governments, environmental services companies, and pollutant enterprises, to analyze the effect of critical factors on cooperation (Xu et al., 2019). However, little research is related to optimizing supervision mechanisms under limited supervision ability. Besides, in earlier research, the literature on pollutant emission reduction regulatory mechanisms initiated a preliminary exploration, proposing the concept of supervising agents based on heterogeneous risk preferences under the assumption of limited supervisory ability (Wang et al., 2023). However, these prior studies primarily focused on evolutionary game theory and complex network theory, concentrating on the optimization of regulatory mechanisms while neglecting the influence of the evolution of risk preferences on strategic choices. In comparison, our study emphasizes the application of evolutionary game theory and opinion dynamics, with a specific focus on the evolving risk preferences of enterprises during the game. Simultaneously, this research addresses some gaps present in previous studies by introducing opinion dynamics and the coevolution of strategies and preferences.
Our study is concentrated on revealing how strategies and preferences coevolve in enterprise decision-making. Through the lens of opinion dynamics, we offer a distinctive analytical perspective that accentuates the dynamic processes underlying individual decision-making in the game, thereby expanding our comprehension of pollutant emission reduction regulatory mechanisms.
2.2 Preference evolution
The hypothesis of bounded rationality holds that there are no completely rational decision-makers manifesting as bounded rationality in decision-makers’ preferences (Camerer, 1997; Di and Liu, 2016). Camerer proposed and introduced social preferences into the game theory (Camerer, 1997). Social preferences have been used to explore complex system issues about social dilemmas, such as fair preference (Fan et al., 2017), reciprocal preference (Wu, 2014), and altruistic preference (Fan et al., 2019). Risk preferences demonstrate enterprises’ attitudes towards the potential risk, including risk-averse (RA), risk-neutral (RN), and risk-seeking (RS) (Camacho-Cuena and Requate, 2012; Larue et al., 2017). RA agents prefer a safe strategy to avoid losses caused by potential risks, while RS agents, on the contrary, prefer an adventurous strategy for higher profits. Bontems and Nauges considered a model of pollution supervision for RA agents involving hidden information and moral hazard (Bontems and Nauges, 2019). Camacho-Cuena and Requate proposed three supervision mechanisms, including collective fining, random fining, and a tax-subsidy scheme, and compared the strategy selection of RA and RS enterprises under different supervision mechanisms (Camacho-Cuena and Requate, 2012).
Two aspects have been rarely explored in the aforementioned studies. First, agents in a complex system could be a multi-preference group. Different agents have heterogeneous preferences. For example, by introducing social preference, Chen et al. divided the preferences of Internet users into egoistic, altruistic, and fair preferences (Chen et al., 2020). They studied the effects of different social preferences on public opinion. Second, social preferences are static in most previous studies, but the preferences could be dynamic over time. Agents’ preferences could be influenced by interacting in the system and change in the evolutionary game process. Variability in the external environment might affect agents’ decisions and force a change in agents’ preferences. The theory of opinion dynamics is a new approach to simulating dynamic preference evolution (Acemoglu and Ozdaglar, 2011; Axsen et al., 2013; Liang et al., 2019; Chen et al., 2020). Axsen et al., for example, investigated the roles of social influence in the formation of consumer preferences for pro-environment technologies, and the result showed that preferences change through social interaction (Axsen et al., 2013). The effect of preference evolution on social cooperation is underestimated.
The theory of opinion dynamics is a powerful tool for describing an interactive group and exploring preference evolution (Liang et al., 2019; Zhang et al., 2019). Opinion denotes the agents’ views or attitudes in opinion dynamics (Chen et al., 2023). The Hegselmann and Klause model (HK model) is one of the original opinion dynamics models (Hegselmann and Krause, 2002). In the HK model, opinions are described as real numbers in a fixed interval, and agents interact with each other if their opinions are similar. Agents update their opinions by averaging all similar opinions within the confidence threshold at each discrete time. There are variously improved models based on the original HK model (Fu et al., 2015; Han et al., 2019). In a modified HK model, agents update opinions considering the game payoff (Bauso and Cannon, 2018). Motivated by the above research, this paper introduces a preference evolution model considering the weight based on the game payoff.
Combined with opinion dynamics, some studies on preference evolution involve social issues. Liang et al. argued that preferences would be dynamically evolved and developed a preference evolution model based on online interactions through a communication tool (Liang et al., 2019). The research of preference evolution focuses on a social complex system, but the problem of pollutant abatement with preference evolution is yet to be explored. Therefore, we introduce the theory of opinion dynamics to fill the gap in the study of pollutant abatement among regional enterprises with preference evolution.
3 Model
First, we introduce a preference evolution model using the opinion dynamics theory. Next, we propose an evolutionary game model for pollutant abatement involving regulators and enterprises. Simultaneously, we develop a smart supervision mechanism based on the random supervision mechanism to reduce pollutant emissions.
3.1 Preference evolution of enterprises
After each game, agents update their preferences synchronously considering benefits. If an enterprise has a higher benefit at this time, the enterprise has a strong impact on others’ preferences.
We consider a population composed of
where
We consider a modified HK model to depict preference evolution. In the modified model, each preference has a weight based on the proportion of benefits, that is, the larger the benefit of an agent is, the more influential its preference is. Similarly, agent
where
3.2 Evolutionary game of pollutant abatement
Some enterprises emit pollution in the production process. To protect the environment in the region in charge, regulators supervise the behavior of enterprises’ pollutant abatement. Enterprises select the pollutant abatement strategy (strategy C) or non-abatement strategy (strategy D) according to benefit comparison and their preferences.
There are two goals of pollutant abatement. A maximum goal (
The parameter
In the initial state, each enterprise selects strategy C or D with equal probability.
Based on the above assumptions, the benefit of each enterprise that is shut down is 0. the benefit of each enterprise that is fined is
In previous studies, the Fermi rule is a common strategy updating rule. This paper proposes a modified Fermi rule combining the classic Fermi rule and the enterprise’s preference, as shown in Eq. 3.
where
3.3 Supervision mechanism
There are two supervision mechanisms: general supervision (GS) and smart supervision (SS). In previous studies (Alpízar et al., 2004; Camacho-Cuena and Requate, 2012; Fan et al., 2017), regulators could only randomly choose a part of enterprises to supervise without considering preferences, which is named GS mechanism in this paper.
In the SS mechanism, regulators cannot distinguish the enterprises’ preferences in the initial state. Regulators establish a file for each enterprise, which is described as a vector
The parameter of
The whitelist evolves dynamically: 1) At each time, regulators add enterprises that newly meet the condition to the whitelist. 2) The whitelist capability, namely, the maximum number of enterprises in the whitelist, is
4 Numerical simulation and results
4.1 Initialization settings
According to previous studies of opinion dynamics, we first consider a population with homogeneous bounded confidence
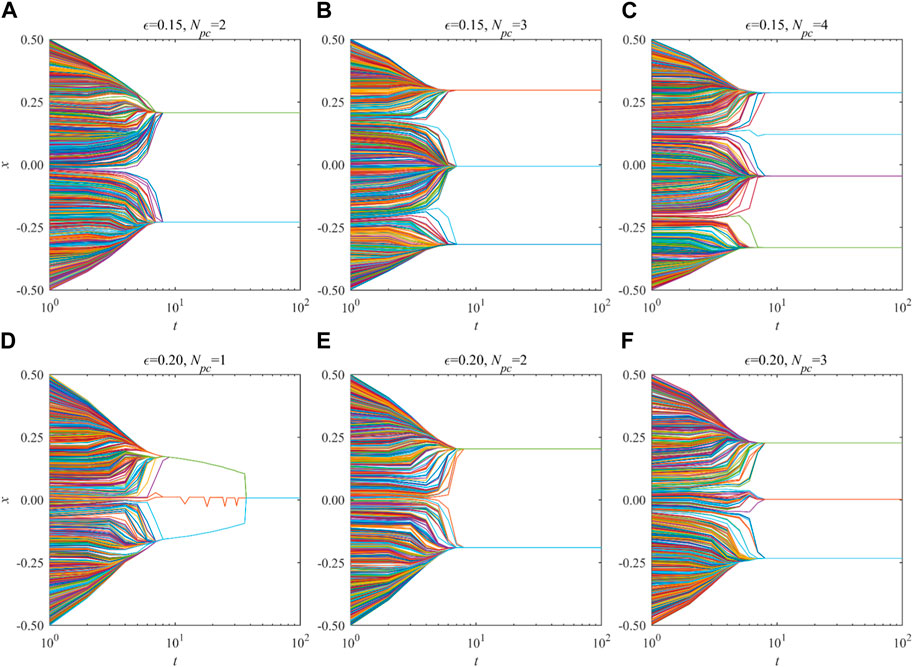
FIGURE 3. Path of preference evolution when
In Figure 3, each line represents the preference evolution path of an enterprise: (1) in Figure 3A–C,
The relevant parameters are set as follows: we set the total number of nodes in the complete graph as
The evolution time is set to 2000 to stabilize the results. The values of supervision ability and whitelist capability are set in the range of
In the first scenario, when
4.2 Effect of pollutant abatement
Figure 4 shows the results of the trend of pollutant abatement with
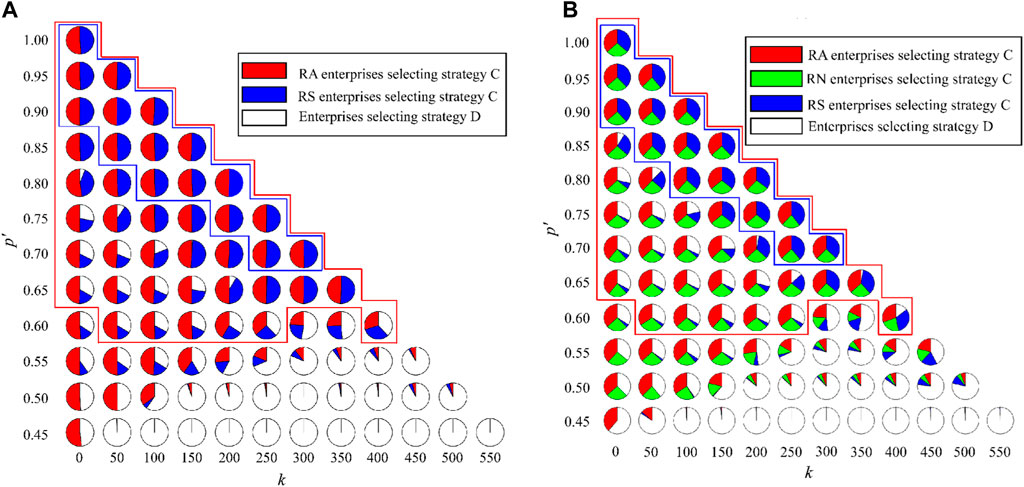
FIGURE 4. Pollutant abatement effect for different
In the scenario of
From the perspective of the subpopulations, in the scenario of
Similarly, in the scenario of
4.3 Effect of enterprises’ benefits
As can be seen in Figure 5A, when
Moreover, the benefits of subpopulations with the same abatement proportion may differ according to supervision mechanisms. For example, in Figure 4B, we can see that pollutant abatement effects of three subpopulations (RA, RN, and RS) are similar if
In Figure 6, the benefit trend with the change of
4.4 Effect of judgment accuracy
Regulators need to judge the enterprise’s preference according to the previous abatement behavior. However, regulators may mistakenly identify RS enterprises as RA ones and add them to the whitelist. We analyse the judgment accuracy of the whitelist member’s preference in different cases based on the simulation result.
In Figure 7, we depict the judgment accuracy in the scenarios of
Compared Figure 7A with 7(b), we can see some differences between different
4.5 Effect of the observation period
As shown in Figure 4, the introduction of the SS mechanism leads to the decline of the cooperation rate if
Figure 8 shows the effect of observation period on the fraction of cooperators. In Figure 8, the change of
Thus, facing the issue of reducing the cooperation rate caused by the SS mechanism, a higher cooperation level can be achieved by appropriately shortening the observation period and increasing the whitelist capability.
5 Conclusion and implications
It is evident that supervision can improve the pollutant abatement effect. To optimize supervision efficiency, we discuss how regulators supervise enterprises under the collective risk of pollutant abatement considering heterogeneous preferences and limited supervision ability. First, a coevolution model for game and preference is proposed based on the theories of the evolutionary game and opinion dynamics. Second, by introducing the concepts of the whitelist and observation period, we propose a smart supervision mechanism considering enterprises’ preferences. Besides, we prove that the smart supervision mechanism can promote pollutant abatement more than the random supervision through the simulation. Furthermore, some recommendations are put forward to improve regulators’ supervision efficiency.
The results show that (1) the effect on pollutant abatement under the smart supervision mechanism is better than that under the random supervision mechanism. Although the smart supervision mechanism leads to the decline of cooperation rate in a few cases compared with the random supervision mechanism, shortening the observation period and increasing the whitelist capability can improve the effect of pollutant abatement. Thus, regulators can achieve the abatement goal with a lower supervision ability in the smart supervision mechanism. (2) Generally, a higher benefit can be achieved if the pollutant abatement is better. However, regulators pay more attention to the risk-seeking enterprises under the smart supervision mechanism, so the risk-seeking ones are punished more. It causes the risk-seeking enterprises have a lower benefit at the same cooperation level compared with other enterprises. (3) Regulators can accurately judge the enterprises’ preferences according to historical decision differences. The judgment accuracy declines if there are risk-neutral enterprises in the population or minor decision differences.
Based on the research conclusions, we put forward the following suggestions. (1) Under the limited supervision ability, regulators should consider enterprises’ differences. Risk preferences can affect the decision behavior and cause enterprises to implement pollutant abatement with different probabilities under the same situation. Thus, regulators should adopt a more targeted supervision mechanism. (2) Regulators can use historical decision-making to judge enterprises’ preference type. Moreover, regulators can strengthen the supervision of enterprises that adopt the strategy of non-abatement more. In this way, regulators can make the limited supervision ability more efficient. (3) Combined with the supervision ability, setting appropriate whitelist capacity and observation period can achieve a better supervision effect. If the supervision is higher, a larger whitelist capability can improve the cooperation level. Notably, if the supervision ability is lower, shortening the observation can achieve a better effect.
Considering practical applications, a similar supervision mechanism has been implemented in 2023. In Xingtai City, Hebei Province, China, the Market Supervision Administration conducted a joint random inspection of key emission units from November 6 to 30 November 2023 (Xingtai, 2023). The inspection covered all key emission units (enterprises) in the city, with a sampling rate of 10%. Leveraging the national Internet + regulatory credit information, the Market Supervision Administration employed a differentiated random inspection based on enterprise credit risk. For those with lower credit risk, the inspection rate was reasonably reduced, while for those with higher credit risk, the inspection rate was increased. The Market Supervision Administration categorized enterprise risks into four levels, from low to high: A, B, C, and D. The sampling rate for B-risk was 6%, for C-risk was 13%, and for D-risk was 40%. Whether the supervision mechanism considering the differences in enterprises’ preferences can improve the environment in practice still requires long-term observation. However, the regulatory authorities’ ability to use differentiated inspections based on credit information marks a progress in management.
Nevertheless, some limitations still exist. We assume enterprises have a preference for selecting strategies in this paper. Besides, the amount of pollutant abatement is unchanged if the enterprise is a cooperator. Namely, we only consider the influence of preferences on strategy selection and neglect the impact on the amount of pollutant abatement. A better understanding of decision preference is needed to enrich further the research on the supervision mechanism of pollutant abatement among regional multi-enterprises. Besides, this study places a greater emphasis on theoretical framework construction and exploration, laying the foundation for empirical research. Future research endeavors may pivot towards empirical data collection and analysis to substantiate and enhance the feasibility and applicability of our theoretical model.
Data availability statement
The raw data supporting the conclusion of this article will be made available by the authors, without undue reservation.
Author contributions
XW: Conceptualization, Data curation, Formal Analysis, Funding acquisition, Writing–original draft. DZ: Methodology, Software, Supervision, Writing–review and editing.
Funding
The author(s) declare financial support was received for the research, authorship, and/or publication of this article. This research was funded by the National Natural Science Foundation of China (71672145 and 72171195).
Conflict of interest
The authors declare that the research was conducted in the absence of any commercial or financial relationships that could be construed as a potential conflict of interest.
Publisher’s note
All claims expressed in this article are solely those of the authors and do not necessarily represent those of their affiliated organizations, or those of the publisher, the editors and the reviewers. Any product that may be evaluated in this article, or claim that may be made by its manufacturer, is not guaranteed or endorsed by the publisher.
References
Acemoglu, D., and Ozdaglar, A. (2011). Opinion dynamics and learning in social networks. Dyn. Games Appl. 1, 3–49. doi:10.1007/s13235-010-0004-1
Alpízar, F., Requate, T., and Schram, A. (2004). Collective versus random fining: an experimental study on controlling ambient pollution. Environ. Resour. Econ. 29, 231–252. doi:10.1023/b:eare.0000044608.66145.0c
Axsen, J., Orlebar, C., and Skippon, S. (2013). Social influence and consumer preference formation for pro-environmental technology: the case of a UK workplace electric-vehicle study. Ecol. Econ. 95, 96–107. doi:10.1016/j.ecolecon.2013.08.009
Bahel, E. (2018). Cooperation and subgame perfect equilibria in global pollution problems with critical threshold. Environ. Resour. Econ. 70, 457–481. doi:10.1007/s10640-017-0129-4
Barrett, S. (2013). Climate treaties and approaching catastrophes. J. Environ. Econ. Manag. 66, 235–250. doi:10.1016/j.jeem.2012.12.004
Bauso, D., and Cannon, M. (2018). Consensus in opinion dynamics as a repeated game. Automatica 90, 204–211. doi:10.1016/j.automatica.2017.12.062
Bontems, P., and Nauges, C. (2019). Production choices with water markets and risk aversion: the role of initial allocations and forward trading. Eur. Rev. Agric. Econ. 46, 579–608. doi:10.1093/erae/jby033
Camacho-Cuena, E., and Requate, T. (2012). The regulation of non-point source pollution and risk preferences: an experimental approach. Ecol. Econ. 73, 179–187. doi:10.1016/j.ecolecon.2011.10.019
Camerer, C. F. (1997). Progress in behavioral game theory. J. Econ. Perspect. 11, 167–188. doi:10.1257/jep.11.4.167
Carraro, C., and Sgobbi, A. (2008). Modelling negotiated decision making in environmental and natural resource management. Automatica 44, 1488–1503. doi:10.1016/j.automatica.2007.12.021
Chang, S., Wang, X., and Wang, Z. (2015). Modeling and computation of transboundary industrial pollution with emission permits trading by stochastic differential game. PLoS One 10, e0138641. doi:10.1371/journal.pone.0138641
Chen, J., Li, P., Wang, X., and Yi, K. (2023). Above management: scale development and empirical testing for public opinion monitoring of marine pollution. Mar. Pollut. Bull. 192, 114953. doi:10.1016/j.marpolbul.2023.114953
Chen, T., Li, Q., Fu, P., Yang, J., Xu, C., Cong, G., et al. (2020). Public opinion polarization by individual revenue from the social preference theory. Int. J. Environ. Res. Public Health 17, 946. doi:10.3390/ijerph17030946
Chen, X., Zhang, Y., Huang, T.-Z., and Perc, M. (2014). Solving the collective-risk social dilemma with risky assets in well-mixed and structured populations. Phys. Rev. E 90, 052823. doi:10.1103/physreve.90.052823
Di, X., and Liu, H. X. (2016). Boundedly rational route choice behavior: a review of models and methodologies. Transp. Res. Pt. B-Methodol. 85, 142–179. doi:10.1016/j.trb.2016.01.002
Fan, R., Dong, L., Yang, W., and Sun, J. (2017). Study on the optimal supervision strategy of government low-carbon subsidy and the corresponding efficiency and stability in the small-world network context. J. Clean. Prod. 168, 536–550. doi:10.1016/j.jclepro.2017.09.044
Fan, R., Lin, J., and Zhu, K. (2019). Study of game models and the complex dynamics of a low-carbon supply chain with an altruistic retailer under consumers’ low-carbon preference. Phys. A 528, 121460. doi:10.1016/j.physa.2019.121460
Fu, G., Zhang, W., and Li, Z. (2015). Opinion dynamics of modified Hegselmann–Krause model in a group-based population with heterogeneous bounded confidence. Phys. A Stat. Mech. Its Appl. 419, 558–565. doi:10.1016/j.physa.2014.10.045
Gu, Q., Hang, L., and Sun, S. (2022). Behavioral game theory model in pollution control with additional supervision. Algorithms 15, 137. doi:10.3390/a15050137
Gupta, S., Saksena, S., and Baris, O. F. (2019). Environmental enforcement and compliance in developing countries: evidence from India. World Dev. 117, 313–327. doi:10.1016/j.worlddev.2019.02.001
Han, W., Huang, C., and Yang, J. (2019). Opinion clusters in a modified Hegselmann-Krause model with heterogeneous bounded confidences and stubbornness. Phys. A 531, 121791. doi:10.1016/j.physa.2019.121791
Hegselmann, R., and Krause, U. (2002). Opinion dynamics and bounded confidence: models, analysis and simulation. JASSS 5, 2.
Jeon, C., Lee, J., and Shin, J. (2015). Optimal subsidy estimation method using system dynamics and the real option model: photovoltaic technology case. Appl. Energy 142, 33–43. doi:10.1016/j.apenergy.2014.12.067
Jiao, J., Chen, J., Li, L., and Li, F. (2017). A study of local governments’ and enterprises’ actions in the carbon emission mechanism of subsidy or punishment based on the evolutionary game. Chin. J. Manag. Sci. 25, 140–150. doi:10.16381/j.cnki.issn1003-207x.2017.10.015
Kou, P., Han, Y., and Li, Y. (2021). An evolutionary analysis of corruption in the process of collecting environmental tax in China. Environ. Sci. Pollut. Res. 28, 54852–54862. doi:10.1007/s11356-021-13104-4
Larue, B., West, G. E., Singbo, A., and Tamini, L. D. (2017). Risk aversion and willingness to pay for water quality: the case of non-farm rural residents. J. Environ. Manag. 197, 296–304. doi:10.1016/j.jenvman.2017.03.050
Liang, H. M., Li, C. C., Jiang, G. Y., and Dong, Y. C. (2019). Preference evolution model based on Wechat-like interactions. Konwl. Based Syst. 185, 104998. doi:10.1016/j.knosys.2019.104998
Liu, C., Tang, C., Liu, Z., and Huang, Y. (2023). How does public environmental supervision affect the industrial structure optimization? Environ. Sci. Pollut. Res. 30, 1485–1501. doi:10.1007/s11356-022-22163-0
Liu, Q., and Li, L. (2019). Spatial heterogeneity of government regulation, spatial distance and enterprise carbon information disclosure: an analysis based on the heavy pollution industry in China. Int. J. Environ. Res. Public Health 16, 4777. doi:10.3390/ijerph16234777
Mäs, M., Flache, A., and Helbing, D. (2010). Individualization as driving force of clustering phenomena in humans, PLoS comput. Biol 6, e1000959. doi:10.1371/journal.pcbi.1000959
Ning, X., Qiu, Y., Wu, C., and Jia, K. (2022). Developing a decision-making model for construction safety behavior supervision: an evolutionary game theory-based analysis. Front. Psychol. 13, 861828. doi:10.3389/fpsyg.2022.861828
Santos, F. C., and Pacheco, J. M. (2011). Risk of collective failure provides an escape from the tragedy of the commons. Proc. Natl. Acad. Sci. U. S. A. 108, 10421–10425. doi:10.1073/pnas.1015648108
Schmidt, R. C. (2017). Dynamic cooperation with tipping points in the climate system. Oxf. Econ. Papers-New Ser. 69, 388–409. doi:10.1093/oep/gpw070
Tavoni, A., Dannenberg, A., Kallis, G., and Loeschel, A. (2011). Inequality, communication, and the avoidance of disastrous climate change in a public goods game. Proc. Natl. Acad. Sci. U. S. A. 108, 11825–11829. doi:10.1073/pnas.1102493108
Vasconcelos, V. V., Santos, F. C., Pacheco, J. M., and Levin, S. A. (2014). Climate policies under wealth inequality. Proc. Natl. Acad. Sci. U. S. A. 111, 2212–2216. doi:10.1073/pnas.1323479111
Wang, C., and Shi, F. (2019). An evolutionary game model for industrial pollution management under two punishment mechanisms. Int. J. Environ. Res. Public Health 16, 2775. doi:10.3390/ijerph16152775
Wang, J., Fu, F., and Wang, L. (2010). Effects of heterogeneous wealth distribution on public cooperation with collective risk. Phys. Rev. E 82, 016102. doi:10.1103/physreve.82.016102
Wang, Q., and Yang, Z. M. (2016). Industrial water pollution, water environment treatment, and health risks in China. Environ. Pollut. 218, 358–365. doi:10.1016/j.envpol.2016.07.011
Wang, X., Guo, P., and Guo, N. (2023). Optimization of supervision mechanism for pollutant abatement under limited supervision ability and heterogeneous risk preference. Operations Res. Manag. Sci. 32, 23–28. doi:10.12005/orms.2023.0144
Wu, G. D. (2014). Project-based supply chain cooperative incentive based on reciprocity preference. Int. J. Simul. Model 13, 102–115. doi:10.2507/ijsimm13(1)co3
Wu, H., Li, Y., Hao, Y., Ren, S., and Zhang, P. (2020). Environmental decentralization, local government competition, and regional green development: evidence from China. Sci. Total Environ. 708, 135085. doi:10.1016/j.scitotenv.2019.135085
Xingtai (2023). Xingtai key greenhouse gas emission units sector Implementation plan for joint random sampling work. https://scjg.xingtai.gov.cn/index.php?m=article&a=view&id=8632 (Accessed February 3, 2024).
Xu, L., Di, Z., and Chen, J. (2021). Evolutionary game of inland shipping pollution control under government co-supervision. Mar. Pollut. Bull. 171, 112730. doi:10.1016/j.marpolbul.2021.112730
Xu, R., Wang, Y., Wang, W., and Ding, Y. (2019). Evolutionary game analysis for third-party governance of environmental pollution. J. Ambient. Intell. Humaniz. Comput. 10, 3143–3154. doi:10.1007/s12652-018-1034-6
Yu, L., Zheng, S., and Gao, Q. (2023). Government environmental regulation strategy for new pollutants control in mariculture. Mar. Policy 150, 105545. doi:10.1016/j.marpol.2023.105545
Zeng, M., Zheng, L., Huang, Z., Cheng, X., and Zeng, H. (2023). Does vertical supervision promote regional green transformation? Evidence from Central Environmental Protection Inspection. J. Environ. Manag. 326, 116681. doi:10.1016/j.jenvman.2022.116681
Zhang, B., Dong, Y., and Herrera-Viedma, E. (2019). Group decision making with heterogeneous preference structures: an automatic mechanism to support consensus reaching. Group Decis. Negot. 28, 585–617. doi:10.1007/s10726-018-09609-y
Zhou, Z., Feng, H., Wang, H., and Wang, K. (2022). Influence of heterogeneous environmental regulation policies on the strategy of pollutant discharge for enterprise: an evolutionary game approach. Environ. Res. Commun. 4, 095002. doi:10.1088/2515-7620/ac8971
Keywords: pollutant abatement, risk preference, evolutionary game, opinion dynamics, supervision mechanism
Citation: Wang X and Zhang D (2024) Integrating coevolutionary strategies and risk preferences: a novel supervision insight for pollutant abatement. Front. Environ. Sci. 12:1330187. doi: 10.3389/fenvs.2024.1330187
Received: 31 October 2023; Accepted: 19 February 2024;
Published: 28 February 2024.
Edited by:
Pierre Glynn, United States Geological Survey (USGS), United StatesCopyright © 2024 Wang and Zhang. This is an open-access article distributed under the terms of the Creative Commons Attribution License (CC BY). The use, distribution or reproduction in other forums is permitted, provided the original author(s) and the copyright owner(s) are credited and that the original publication in this journal is cited, in accordance with accepted academic practice. No use, distribution or reproduction is permitted which does not comply with these terms.
*Correspondence: Duo Zhang, zhangduosjz@163.com