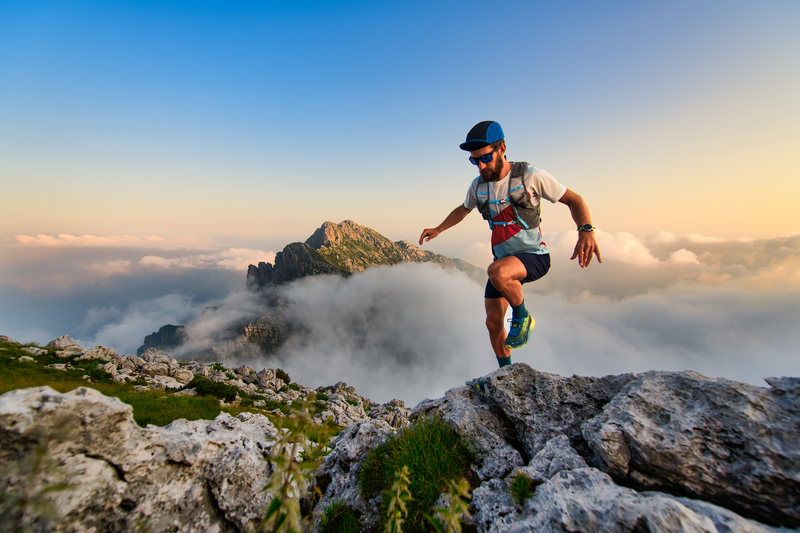
95% of researchers rate our articles as excellent or good
Learn more about the work of our research integrity team to safeguard the quality of each article we publish.
Find out more
ORIGINAL RESEARCH article
Front. Environ. Sci. , 13 March 2024
Sec. Environmental Economics and Management
Volume 12 - 2024 | https://doi.org/10.3389/fenvs.2024.1320318
Marine fishery, with its duality of carbon emission and carbon sink, is an industry that needs full attention during achieving carbon neutrality. In this paper, the decoupling index between net CO2 emissions and gross domestic product of marine fishery in China is calculated using Tapio model, and its evolution characteristics are analyzed by means of nuclear density map and Markov matrix. Some problems are found, such as the decoupling state lacked significant improvement during this period and was unstable. Through theoretical analysis, this paper puts forward the view that solving these problems requires support from green finance. Then, this paper selects panel data from 11 coastal provinces and cities in China from 2010 to 2020 and uses the Logit model and EKC model to investigate the impact of green finance on the carbon decoupling state of marine fishery. The main results of this study are as follows: 1) Green finance can increase the odds ratio of strong or weak decoupling in marine fishery. This effect is more significant in regions with a high degree of digital finance development and the eastern marine economic circle. The effect of green investment is more significant than green insurance. In addition, boosting technological innovation and reducing the proportion of coal consumption can strengthen this effect. 2) The EKC curve between net CO2 emissions and gross domestic product of marine fishery is N-shape, which has a deterioration point. Green finance can delay the arrival of the deterioration point, meaning it can prevent the rapid deterioration of the decoupling state with the development of marine fishery. This paper provides empirical evidence and decision-making reference for resolving the dilemma of carbon decoupling in China’s marine fishery.
With the advancement of global industrialization, fossil fuel resources have been exploited in large quantities, and greenhouse gas emissions have soared (Francey et al., 2013). This problem has been widely discussed by the international community (Zhang and Da, 2015). In 2016, 175 countries signed the Paris Agreement, committing to achieve a balance between CO2 emitted by humans and absorbed by sinks in the latter half of this century. However, if achieving this goal requires sacrificing economic growth, then the incentive and effort of countries will be far less than they have promised (Wang and Zhang, 2021). Therefore, the international community urgently needs methods and approaches to decouple development from net CO2 emissions. As a responsible country, the Chinese government has also set targets for greenhouse gas emissions, aiming to reach peak carbon emissions by 2030 and achieve carbon neutrality by 2060. However, China is currently in a period of rapid urbanization and industrialization, which needs to consume a lot of energy (Lu et al., 2011; Lin and Ouyang, 2014). In addition, China’s coal-dominated energy structure makes China face tremendous pressure to reduce emissions (Zhang et al., 2018). China is facing an arduous mission of balancing the goal of building a socialist modernized country and the international responsibility of tackling global climate change. There is also an urgent need for China to find solutions that can both reduce carbon emissions and boost economic development.
Increasing carbon sinks is a crucial way to achieve “carbon neutrality.” The oceans are the largest pool of carbon, storing 93% of the global CO2 and absorbing about a third of the CO2 emitted by human activities each year (IPCC, 2019). As an essential marine industry, marine fishery is a vital carbon sink way, but it is also a typical energy-intensive industry, with fuel costs that can account for 60% of total costs (Tyedmers et al., 2005). In 2016, global marine fishery fuel engines emitted about 207 million tons of CO2, which corresponds to the CO2 emissions of 51 power plants during the same period (Guangliang et al., 2023). What’s worse, from 1990 to 2011, the global fishing industry’s CO2 emissions increased by 28%, while production barely kept pace (Parker et al., 2018). It can be seen that marine fishery is an obvious source of CO2 emissions, but they are often ignored in global assessments of greenhouse gases. It will lead to a naive optimism about the current state of global greenhouse gas emissions and continue to allow marine fishery to develop in this extensive mode, thereby reducing the effectiveness of marine carbon sinks, putting pressure on climate change, and exacerbating the ecological challenges. Therefore, to make better use of marine carbon pools to assist in the completion of “carbon peak and carbon neutrality”, marine fishery’s duality needs urgent attention.
Green finance was born to solve the contradiction between environment and development (Kumar et al., 2023). It is an innovative financial tool that provides financial support, risk management, and other financial services for environmental protection projects (Yu et al., 2021). In order to ensure the effective role of green finance, China has gradually established the green finance standard system, financial institution supervision and information disclosure requirements, incentive and restraint mechanism, green finance product and market system, and green finance international cooperation system. At the end of June 2022, the green loan balance of domestic and foreign currency in China reached 19.55 trillion yuan, and the stock size of green bonds reached 1.2 trillion yuan, ranking second in the world. With the development of green finance, the impact of green finance on emission reduction has attracted the attention of the majority of scholars. It has been confirmed to be an effective means to reduce CO2 emissions (Ren et al., 2020; Meo and Karim, 2022). It can inhibit carbon emissions through financial constraints, promoting green technological innovation, adjusting industrial and energy structures, and improving energy intensity (Chen and Chen, 2021; Hu and Zheng, 2022).
There is a broad consensus that green finance can drive emission reduction, but there are few studies on its impact on the state of carbon decoupling. That is to say, it is unclear whether the emission will be reduced at the expense of development. However, the goal of sustainable development should not only focus on “sustainability” but “development.” Therefore, it holds significant theoretical and practical importance to examine the influence of green finance on the decoupling state of net CO2 emissions from the gross domestic product (GDP) of marine fishery (hereinafter the decoupling state of net CO2 emissions from the GDP of marine fishery is referred to as carbon decoupling state of marine fishery, which is abbreviated as
This paper makes the following contributions: Firstly, this paper focuses on the marine fishery, an important but often overlooked industry, and uses the Tapio model to calculate the decoupling index of net CO2 emissions (
Given the increasing importance of environmental issues, scholars have come to realize that to achieve green development and carbon neutrality, marine fishery can no longer be ignored. Researchers gradually began to pay attention to the carbon sink function of marine fishery. Yuan et al. (2023), Krabbe et al. (2022), and Wang et al. (2022) identified several factors that influence the carbon sink capacity of the marine fishery, including national policies, fishing output, number of employees, area of shellfish and large-scale seaweed aquaculture farms, total power of fishing vessels, and reform of international fishery law. In addition, Zhang et al. (2023a) and Li et al. (2023c) argued that collaborative efforts among multiple stakeholders and financial support are more advantageous in realizing the ecological value of blue carbon in marine ranches, whereas Alsaleh et al. (2023) discovered that the utilization of fossil fuels serves as a crucial driving force behind the degradation of carbon sinks. Research on carbon emissions in marine fishery is also a hot topic. Some scholars have established a simulation model for the low-carbon development of China’s marine fishery by using the system dynamics method to find the factors affecting carbon emissions. Research by Chen et al. (2022a) showed that rapid economic growth has significantly increased carbon emissions of the marine fishery. At the same time, adjustments in energy and industrial structure have contributed to carbon emission reduction. Chen (2023) demonstrated that optimizing the industrial structure of the marine fishing industry can improve carbon emission efficiency. Research on carbon decoupling gradually extended to marine fishery. Li et al. (2022b) argued that industrial structure plays a significant role in contributing to CO2 emissions, and carbon intensity is the main negative driving factor. Wang and Wang (2022) also pointed out that carbon intensity acts as a key driver for carbon reduction and advanced decoupling. Liu et al. (2023a) found that the social and economic system, as well as the scale of marine fishery development, are essential factors driving carbon emissions, and adjusting industry structure will inhibit marine fishery carbon emissions. What’s more, Zhang et al. (2023) found that the economic and trade development of marine fishery would increase CO2 emissions, and the technological progress and income growth of fishermen could reduce carbon emissions.
In addition to the aforementioned factors influencing decoupling, green finance is believed to have a positive impact. Zhou et al. (2020) found that green finance promotes economic growth while limiting environmental degradation, shifting the turning point of the Environmental Kuznets Curve (EKC) to the left. This implies that green finance can achieve decoupling at a lower level of economic growth. In addition, scholars generally believe that green finance can curb CO2 emissions (Hu and Zheng, 2022; Meo and Karim, 2022; Li et al., 2023b). Lin et al. (2023) employed spatial measurement methods to investigate the linear and non-linear relationship between green finance and carbon emissions. The study demonstrated that green finance is an effective pathway for reducing carbon emissions. Du (2023) demonstrated the negative impact of green finance on carbon intensity across all quantiles, both in the short and long term. Liang and Song (2022) pointed out that green finance not only has a “local effect” on carbon emission efficiency, but also has a “neighborhood effect.” Moreover, Zhang et al. (2022c) constructed indicators to comprehensively measure carbon emission efficiency from the input-output perspective, providing further evidence of the emission reduction effect of green finance. Li et al. (2022a) used CO2 as an undesirable output to measure low-carbon total factor productivity, highlighting the crucial role of green finance in transitioning from a high-carbon to a low-carbon economy. Specifically at the industry level, Ren et al. (2023) proved that green finance can facilitate the low carbonization of industries in pilot zones by using the difference-in-difference method. Xu et al. (2023a) demonstrated that green finance can drive the low-carbon development of industry not only in the region but also in surrounding regions. The research results of Guo et al. (2022) indicate that green finance is expected to play a substantial role in mitigating fertilizer usage and decreasing carbon emissions in the agricultural sector within a decade. Liu et al. (2023b) demonstrated the effectiveness of green finance in promoting emissions reduction in the transportation sector through the construction of a discontinuous regression model.
EKC is a tool that describes the connection between the quality of the environment and income levels (Grossman and Krueger, 1991). According to EKC theory, economic development is eventually expected to lessen the environmental degradation caused by earlier stages of development (Kaika and Zervas, 2013a). Subsequently, the EKC curve theory has been verified in different countries and different industries (Bölük and Mert, 2015; Bento and Moutinho, 2016; Gokmenoglu and Taspinar, 2018; Eyup and Roula, 2020; Qadri et al., 2023). As the research progresses, a series of empirical studies have revealed the existence of non-regular EKC patterns, including U-shaped and N-shaped curves (Kaufmann et al., 1998; Friedl and Getzner, 2003; Jahanger et al., 2023). This also overturns the original EKC theory. Some scholars believe that this may be due to the differences in the economic structure of different economies (Kaika and Zervas, 2013b; Eyup and Roula, 2020). Based on the assumption of EKC, Tapio (2005) proposed the Tapio decoupling model. The same as the EKC model, the Tapio model can also directly reflect the contradiction between economic growth and environmental degradation The difference is that the Tapio decoupling model has a more specific concept and a more concise calculation. This model has been increasingly adopted to analyze the relationship between economic development and environmental pollution (Ren and Hu, 2012; Hao et al., 2019; Luo et al., 2020). After analyzing the decoupling state with the Tapio model, some papers will explore the decoupling state drivers with the LMDI model (Wang and Zhang, 2020; Apeaning, 2021; Chun et al., 2023). In addition, Wang et al. (2021) combined the Tapio model and EKC model, and believed that the combination of these two models can provide mutual testing and support.
Reviewing the literature reveals numerous studies on the impact of green finance on carbon emission reduction, low-carbon transformation, and low-carbon development in industries. However, there are few studies on the marine industry, especially marine fishery. Secondly, previous studies have tried to prove that green finance can reduce carbon emissions, improve carbon emission efficiency, or promote high-quality development, etc., but few literatures have studied whether green finance can alleviate the contradiction between economic development and environmental pressure in a more direct way. Thirdly, previous studies primarily focus on internal characteristics of the economic system when decomposing factors using the LMDI model, with limited examination of the impact of external policies. Simply waiting for economies to adjust spontaneously to maintain strong decoupling state can be time-consuming and laborious. We need tools to help. Therefore, it is imperative to investigate the impact of green finance on the state of marine fishery decoupling.
Green finance has three major functions: resource allocation, risk management, and market pricing. First, the resource allocation function guides limited capital resources to environmental protection industries. Specifically for marine fishery, green finance can impose financing constraints and increase financing costs to limit the development of energy-intensive sectors like the marine fishing. Simultaneously, financial support is provided to relatively low-carbon industries such as marine leisure fishing and mariculture. By guiding the allocation of resources to reduce the overall carbon dioxide emissions of the marine fisheries industry. Secondly, green insurance can reduce the risk of green innovation in enterprises, promote green innovation, and thus reduce CO2 emissions (Wang et al., 2017). At the same time, its risk management function can reduce the failure risk of enterprises’ low-carbon transformation. Third, the market pricing function of green finance enables the internalization of external pollution costs and facilitates the valuation of ecological environmental products. This helps address market failures by internalizing externalities and promoting environmental protection. For instance, high-emission enterprises in the marine fishing industry may need to purchase carbon emission rights in the carbon trading market, thereby considering the price of carbon emission rights in their costs. And, for leisure fisheries with relatively low carbon emissions and carbon sink fisheries can sell surplus carbon emission rights and obtain additional profits for research and development (Liu et al., 2019).
Besides, green finance has the basic functions of traditional financial instruments and can also promote economic growth in marine fishery through the mobilization of savings, stimulation of capital accumulation, facilitation of risk management, and facilitation of trade. Ngo et al. (2022) found a positive relationship between green finance and economic growth in the Association of Southeast Asian Nations (ASEAN). Xu and Gao (2022) pointed out that green finance is instrumental in facilitating the high-quality development of the marine economy. Su et al. (2021) found financial development is conducive to the growth of marine economy, particularly in the well-established financial system of the eastern marine economic circle, where existing financial resources can be fully utilized. Zheng et al. (2021) argued that the development of fishery economy requires financial instruments, and fishery financial policies are crucial tools to promote its growth.
In a word, green finance can reduce emissions of marine fishery while promoting growth, so this paper posits that green finance can promote carbon decoupling in marine fishery.
Hypothesis 1:. Green finance exhibits a “promoting decoupling effect” on marine fishery.
According to the EKC hypothesis, in the early stage of economic development, increased income will lead to increased pollution. However, once a certain income threshold is reached, higher income levels can contribute to improved ecological conditions, following an inverted U-shaped EKC curve. This phenomenon stems from scale effect, component effect, and technical effect (Sinha et al., 2017). The scale effect suggests that higher output results in increased pollution. Later, as economies develop, the scale effect is masked by a combination of component effects (services may grow faster than manufacturing, which can change the pollution intensity of output) and technical effects (market-driven technological progress or, as a result of government regulation, less polluting technologies may be adopted by various sectors of the economy), and environmental quality begins to improve with income rose (Torras and Boyce, 1998). However, when the marginal return of industrial structure transformation is exhausted, or when the diminishing return of technological change, the scale effect will once again exceed the combined impact of the component effect and the technical effect, then an N-type EKC curve will appear (Lorente and Alvarez-Herranz, 2016). This process is graphically depicted in Figure 1.
FIGURE 1. Relationship between the decoupling state and EKC (Note: ①
According to studies on carbon decoupling in marine fishery, most of the provinces that achieved strong decoupling did not stabilize at strong decoupling and some experienced deterioration (Guan et al., 2022; Wang and Wang, 2022). However, Wang et al. (2021) analyzed the relationship between the Tapio index and the EKC. If the EKC is inverted U-shaped, the state of carbon decoupling will gradually improve with the development. If the EKC is N-shaped, the deterioration phenomenon will occur, as shown in Figure 1. Therefore, we infer that the EKC of marine fishery is N-shaped, which is also consistent with the related research of Fang and Gao (2023).
Hypothesis 2a:. The EKC curve of
If the EKC curve is N-shaped, according to the analysis of Wang et al. (2021), there will be an inflection point of decoupling elasticity on the curve, which is called the deterioration point in this paper, as shown by the point
Green finance can enhance the component effect and technical effect of marine fishery. Regarding component effect, green finance facilitates the promotion of ecological and advanced structures within the marine fishery industry. For the primary industry, carbon finance can reduce the cost of carbon sink fishery, thereby increasing the proportion of carbon sink fishery. And the marine fishing industry will be subject to financing constraints and reduce its scale. For the secondary industry, the processing industry can extend the industrial chain and increase the added value by obtaining green investment for technological innovation. For the low-energy tertiary industry, it is easier to get the financial support from green finance, so as to attract the inflow of labor, capital, and technology to drive its rapid development. At the same time, through coordinated investment and financing, green finance can foster the development of coordination and management to promote the organization of marine fishery, improve the level of scientific research and innovation to reduce resource consumption and production costs, and solve the obstacles faced during the industrial restructuring of marine fishery (Yang and Su, 2010).
In terms of technical effects, green finance directly invests in green technologies (Kumar et al., 2022). In addition, green finance can effectively address market failures during the green technology innovation process. First of all, green technology innovation has dual externalities, that is, knowledge externality caused by the spillover of innovative technology and adoption externality caused by the application of green innovation technology to produce positive social benefits. Double externalities will benefit society and other enterprises, but make green innovators bear the cost of innovation alone, thus discouraging green innovation. Through its market pricing function, green finance can internalize externalities and promote green innovation. Secondly, enterprises are facing incomplete information issues in green technology innovation. Technological innovation is faced with a large number of capital needs and risks. Due to information asymmetry, investors are skeptical about the promised returns. They may demand a premium for investments with such risks, aggravating the difficulty of obtaining investment in green innovation. Green finance, on the other hand, uses its risk management function to transfer risks that may be faced in innovation, while alleviating financial difficulties by strengthening green investment. The risk management function can transfer the risks, while its resource allocation function can alleviate financial difficulties (Jaffe et al., 2005).
In summary, green finance serves as a catalyst for the structural transformation and advancement of green technological innovation in the marine fishery industry, which can better unleash the component and technical effects, delay the rate of marginal decline in component and technical effects, and thus delay the arrival of deterioration points.
Hypothesis 2b:. Green finance has a “delaying deterioration effect” on marine fishery.
The “promoting decoupling effect” and “delaying deterioration effect” of green finance are concisely reflected in Figure 2.
According to scholars’ viewpoints, we undertake a comprehensive evaluation of the green financing system, focusing on green credit, green securities, green insurance, and green investment (Yang et al., 2021; Zhang et al., 2022a). The assessment is quantified using the entropy method, and Table 1 presents the specific indicator system.
CO2 emissions from marine fishing motor vessels account for 70% of the industry’s CO2 emissions, and many studies have used the CO2 emissions from marine fishing motor vessels as a proxy for the total CO2 emissions from the industry. We refer to the methods of Li et al. (2022b) and Shao et al. (2018) to calculate carbon emissions from marine fisheries. The calculation method is shown in Eq. 1.
Then, we assessed the carbon sink capacity of marine fishery, particularly through the cultivation of shellfish and algae. Previous studies have identified these as the primary sources of carbon sinks. Due to the relatively small scale of mariculture in Tianjin and Shanghai, this paper assumes a negligible carbon sink contribution from these regions. The specific calculation method is presented in Eq. 2.
Where
Net CO2 emissions from marine fishery can be calculated as Eq. 3.
Where
Since
Where
Based on the definition provided by Tapio (2005), the
Where
(a) Technological innovation (
(a) Marine fishery scale (
The IPAT identity is widely used to analyze the impact of human activities on the environment (York et al., 2003). According to the IPAT identity, besides the degree of affluence (It is represented by
This study utilizes panel datasets from 11 coastal provinces in China, covering the period from 2010 to 2020. The provinces included are Liaoning, Tianjin, Hebei, Shandong in the northern marine economic circle, Jiangsu, Shanghai, Zhejiang in the eastern marine economic circle, and Fujian, Guangdong, Guangxi, Hainan in the southern marine economic circle. The data sources of this study are the China Fishery Statistical Yearbook, the China Energy Statistical Yearbook, the National Bureau of Statistics website, and the Wind database. The sporadic missing data have been filled using linear interpolation. And all economic data has been adjusted to the 2010 price level to account for inflation.
Descriptive statistics for the variables can be found in Table 3. As can be seen, the average value of
This paper aims to examine the influence of green finance on the carbon decoupling state of marine fishery (
Where
It is worth noting that the regression coefficients (
To further examine the moderating effect, we will construct the following models, as shown in the Eq. 8.
Where
According to the analysis in Section 2.2.2 “Delaying deterioration effect” of green finance, it can be seen that there is a deterioration point on the N-type EKC curve, before which the decoupling state improves with economic development. After that point of deterioration, decoupling progressively deteriorates with economic development. Delaying the arrival of the point of deterioration therefore prevents the rapid deterioration of the decoupling state with economic development and thus enhances the stability of the decoupling state to a certain extent. Next, we will examine the delayed deterioration effect of green finance. Eq. 9 shows the logarithmic transformation of the EKC model.
It is easy to understand when
If the EKC is N-shape, then there will be an inflection point
According to the method of calculating the inflection point, let
That is,
Then we draw on the methods of Wang et al. (2021) to study the impact of green finance on the shape of the EKC curve. We establish the following model, as shown in the Eq. 13.
If the value of
To ensure the robustness of the results, we then build the following model, as shown in the Eq. 14. The derivation of the verification method is shown in Eqs 15–18 (Yin et al., 2015; Gao and Wei, 2022).
Then,
It can be deduced that,
We can see that
It equals to
In other words, if
For ease of analysis, we rank the eight decoupling states. Firstly, this paper believes that emission reduction should not come at the expense of development, so the four states with ∆
FIGURE 3. Trend of carbon decoupling state of marine fishery (A) marine fishery in China (B) marine fishery in the northern marine economic circle (C) marine fishery in the eastern marine economic circle (D) marine fishery in the southern marine economic circle.
From an overall perspective, as shown in Figure 3A, the
From a comparative perspective of the three marine economic circles,
FIGURE 4. Stability of the carbon decoupling state (Note: Based on Liu and Xiao (2022),
As shown in Figure 4, the stability of the eastern marine economic circle is in the middle and lower reaches. Figure 3C shows that from 2010–2015, the
As can be seen from Figure 3D, provinces in the southern marine economic circle exhibit a higher number of years in the state of strong decoupling, indicating that the eastern marine economic circle is relatively less dependent on fossil energy and has a relatively intensive development mode. It can be seen from Figure 4 that, except for Fujian, the stability of
This paper further uses kernel density to describe the overall characteristics and dynamic evolution of
In terms of distribution, the main peak is distributed on the right side of the curve, indicating that there are more provinces with good decoupling states. However, the position of the main peak is slightly shifted to the left. In detail, initially, the main peak moves to the right, then to the left, and there is an overall trend to the left, indicating that the improvement effect of the
From a polarization trend perspective, the polarization phenomenon of China’s
Limited by the amount of data, we divided the decoupling types into three categories to construct the Markov matrix of
It can be seen that 23.08% of provinces and cities are still in the negative decoupling state after 1 year, and the probability of transferring from “negative decoupling” to “coupling” and “decoupling state” is 3.85% and 73.08%, respectively. The probability of the transition from the coupling state to the “negative decoupling” or “decoupling state” is 50.00%. Of the provinces and cities that were initially in decoupling state, 19.51% were transferred to the “negative decoupling state”, 2.44% were transferred to the “coupling state”, and 78.05% remained in the “decoupling state.”
Based on the thorough analysis presented above, the following conclusions can be drawn: Firstly, the stability of
Based on the above analysis, there are two major problems in China’s marine fishery carbon decoupling state: the improvement effect of decoupling state is not obvious and the decoupling state is unstable. Drawing upon the hypothesis presented in Chapter 2, we posit that green finance has the potential to exert a “promoting decoupling effect” and a “delaying deterioration effect” on the problems prevalent in the Chinese
This study utilizes STATA 16.0 to estimate the Logit model. The results are shown in Table 5. As depicted in columns (1)–(5), when control variables are gradually added, the coefficient of core explanatory variable
Regarding the control variables, the scale of marine fishery is significant at the 1% level, and all are less than 0, which means that under current conditions, blindly expanding the scale of marine fishery is not conducive to decoupling. This observation aligns with several studies (Li Z. et al., 2022; Wang and Wang, 2022; Guangliang et al., 2023), which suggests that the current development model of China’s marine fishery is still relatively extensive, and it is imperative to find clean development ways and transform to intensive. In addition, both financial development and urbanization levels have a significant positive impact on the carbon decoupling state of marine fishery. However, the effect of environmental regulation is not significant. This may be because environmental regulation will not only control the carbon emissions of fishing vessels but also increase the cost burden on enterprises. The decoupling index is a composite indicator of environmental and economic indicators. The dual impact of environmental regulation may lead to insignificant effects. In addition, this may also be due to poor effectiveness, lagging nature, or inadequate enforcement of environmental regulations.
Technological innovation is widely acknowledged as a driving force and catalyst for achieving sustainability goals (Dwivedi et al., 2023). Technological innovation has the potential to enhance the conversion rate of capital gains, improve the productivity of low-carbon enterprises, lead the orderly flow of capital to low-carbon industries, and strengthen the resource allocation effect of green finance (Xu Y. et al., 2023). Therefore, technological innovation may have a moderating effect in the process of green finance promoting decoupling. To examine this hypothesis, this paper introduces the interaction term of technological innovation and green finance on the basis of the benchmark regression model to analyze the moderating effect, which is listed in columns (6) of Table 5. The results reveal that the interaction term between technological innovation and green finance is positive and is significant at the level of 5%. This implies that technological innovation plays a positive moderating role in the process of green finance promoting carbon decoupling in marine fishery.
In addition, the promoting decoupling effect of green finance may be largely influenced by energy structure. This is because, on the one hand, over-reliance on fossil energy will hinder regional financial development. On the other hand, the large-scale development of clean energy will not only increase the input cost of original fossil energy, but also drive the carbon emission pricing mechanism within the carbon trading market (Yi et al., 2022). Therefore, we further estimated the moderating effect of energy structure. In Table 5 (7), we observe that the coefficient of the interaction term is significantly negative and passes the significance test of 5%. This suggests that the increasing share of coal consumption is hindering the positive impact of green finance on decoupling.
To ensure the robustness of the above conclusions, several robustness tests were conducted. First, we refer to Yang et al. (2021) to reconstruct the measurement system of green finance. Green credit is measured by the ratio of green credit of listed companies to total credit of listed companies. Green securities are represented by the ratio of the market value of listed companies in the environmental protection industry to the total market value. Green investment is set as the ratio of China’s fiscal expenditure on energy conservation and environmental protection to China’s total fiscal expenditure. Carbon finance is measured by the ratio of carbon dioxide emissions to GDP. And the results are presented in Table 6 (1). Then, to eliminate the influence of outliers on measurement results, we carry out a 1% bilateral tail reduction for all indicator variables. The results are displayed in Table 6 (2). Finally, considering the unique characteristics of Tianjin and Shanghai as municipalities directly under the central government, which differ significantly from other cities in terms of infrastructure and preferential policies, and notably have a small marine fishery and aquaculture industry, these two municipalities were excluded from the analysis. Subsequently, regression analysis was performed on the remaining samples, and the outcomes are presented in Table 6 (3). Remarkably, the significance and direction of the results obtained from these Robustness tests are largely consistent with the previous findings, indicating the robustness and credibility of the regression results in this study.
Various types of green financial instruments may have distinct impacts on the
Digital finance, which integrates the Internet and information technology, represents a novel form of financial services that enhances the accessibility and convenience of financial services. It overcomes the limitations caused by information asymmetry, thus having the potential to facilitate the expansion of green financial products and improve service efficiency (Ye et al., 2023). By utilizing the median of the digital financial development index, this study categorizes the 11 coastal provinces into two groups based on their levels of digital financial development. Subsequently, we employed the data used in the basic regression to conduct regression analysis on the two groups, aiming to investigate whether digital finance can enable green finance to promote carbon decoupling of marine fishery. The findings, presented in column (3)–(4) of Table 7, demonstrate that the coefficient of green finance in both groups of regions is significantly positive. However, the positive effect of green finance is more evident in regions with high development degree. In addition, in regions with a high level of digital finance development, the impact of green finance is greater, with a coefficient of 52.0427. And the coefficient of the regions with a low level is only 42.0769, indicating the empowering effect of digital finance.
The test of heterogeneity according to the classification of the three marine economic circles is classic and necessary. However, after classification, the amount of data in each group is small, and if too many variables are added, the Logit model cannot be estimated scientifically. Therefore, this paper only adds one core explanatory variable and compares it with column (1) of Table 5. This regression uses the same data as the basic regression. The results are presented in Table 7 (5)–(7). The results indicate that a significant positive relationship between green finance and
The regression results for the EKC are reported in Table 8 and column (1) shows the basic EKC model. Column (2) introduced the green finance development index into the model to investigate the influence of green finance on the shape of EKC. Column (3) further introduces the interaction terms between
First of all, according to the coefficients in columns (1)–(3), it is confirmed that the EKC curve between the
In columns (1)–(3), the coefficient of the fishing population is significantly negative, that is, the increase of the fishing population also significantly inhibits the
It is important to highlight that the effects of green technologies in this paper seem to contradict common sense, but studies that include technological variables in the EKC analysis have been mixed. The explanation of Lantz and Feng (2006) for this phenomenon is that time-dependent technological innovation changes regional investment patterns, thereby increasing the demand for fossil fuels. In this paper, we believe that the overall increase in green technology stimulates investment related to marine fishery, thus increasing CO2 emissions. However, at present, the number of green innovations for marine fishery is relatively small. Hence, the effect of green technology on reducing emissions in marine fishery is not apparent, leading to this phenomenon.
Analysis of the evolution trend of
The impact of green finance on the
The promoting decoupling effect of green investment is more significant than green insurance. It may be because green insurance mainly allocates capital into assets with green features through risk management means, which may take a considerable amount of time to yield tangible results (Klapkiv and Ülgen, 2023). Secondly, China’s green insurance system is not mature, and there are problems such as insufficient incentive measures, few compound talents and lack of innovation, resulting in the function of green insurance cannot be effectively played (Chen et al., 2022b). Besides, the effect is more pronounced in regions with advanced digital finance development. As we all know, digital finance facilitates the efficient sharing of factor resources, and make use of the convenient advantages of digital technology, so that enabling effectiveness of green finance. Moreover, the decoupling effect is stronger in the eastern marine economic circle compared to the northern marine economic circle, while it is not significant in the southern marine economic circle. This disparity may be attributed to the more mature financial system in the eastern marine economic circle, which enables the optimal utilization of financial resources, consistent with the findings of Su et al. (2021). Additionally, as previously analyzed, the southern marine economic circle exhibits a relatively robust
The EKC curve between the
In a word, green finance is conducive to promoting carbon decoupling in marine fishery and solving the problem that it is difficult to balance economic development and emission reduction. At the same time, attention should be paid to the enabling role of technological innovation, energy structure upgrading, and digital finance on green finance, as well as the differences in instruments and regions of green finance. This will help to formulate targeted policies, to better meet the challenges under the background of low-carbon economy.
Based on the analysis of the
Firstly, the improvement of
Secondly, green finance has a “promoting decoupling effect”, which passes the robustness test. In addition, enhancing technological innovation and reducing the reliance on coal can enhance the effect. The heterogeneity test results indicate that this effect is particularly pronounced in regions with a high level of digital finance development, as well as in the eastern marine economic circle. Additionally, the impact of green investment appears to be more significant than that of green insurance.
Thirdly, the EKC curve between carbon emissions from marine fishery and their output value shows an “N” shape, which means that there are deterioration points on this EKC curve. Green finance has a “delaying deterioration effect”, which can reduce the possibility of decoupling deterioration and enhance the stability of the decoupling state to a certain extent.
The raw data supporting the conclusion of this article will be made available by the authors, without undue reservation.
WX: Conceptualization, Funding acquisition, Investigation, Project administration, Resources, Supervision, Validation, Writing–review and editing. YZ: Data curation, Formal Analysis, Investigation, Methodology, Software, Visualization, Writing–original draft.
The author(s) declare financial support was received for the research, authorship, and/or publication of this article. This paper is funded by the General Project of Humanities and Social Sciences Research of the Ministry of Education “Study on Mechanism Analysis, Effect Evaluation, and Countermeasure Research of Carbon Unlocking in Manufacturing Empowered by Digital Economy” (Project Approval Number: 23YJC790164).
The authors are grateful to the editor and the reviewers of this paper.
The authors declare that the research was conducted in the absence of any commercial or financial relationships that could be construed as a potential conflict of interest.
All claims expressed in this article are solely those of the authors and do not necessarily represent those of their affiliated organizations, or those of the publisher, the editors and the reviewers. Any product that may be evaluated in this article, or claim that may be made by its manufacturer, is not guaranteed or endorsed by the publisher.
Alsaleh, M., Wang, X., and Nan, Z. (2023). Toward marine sustainability: unveiling the effect of the fishery industry on blue carbon sequestration. Sustain. Dev., 1–15. doi:10.1002/sd.2659
Apeaning, R. W. (2021). Technological constraints to energy-related carbon emissions and economic growth decoupling: a retrospective and prospective analysis. J. Clean. Prod. 291, 125706. doi:10.1016/j.jclepro.2020.125706
Bento, J. P. C., and Moutinho, V. (2016). CO2 emissions, non-renewable and renewable electricity production, economic growth, and international trade in Italy. Renew. Sust. Energ. Rev. 55, 142–155. doi:10.1016/j.rser.2015.10.151
Bölük, G., and Mert, M. (2015). The renewable energy, growth and environmental Kuznets curve in Turkey: an ARDL approach. Renew. Sust. Energ. Rev. 52, 587–595. doi:10.1016/j.rser.2015.07.138
Chen, D. (2023). Analysis of influence factors of marine fishery industry structure optimization on carbon emission efficiency based on depth feedforward model. J. Exp. Nanosci. 18, 2174696. doi:10.1080/17458080.2023.2174696
Chen, J., Wang, Y., Song, M., and Zhao, R. (2017). Analyzing the decoupling relationship between marine economic growth and marine pollution in China. Ocean. Eng. 137, 1–12. doi:10.1016/j.oceaneng.2017.03.038
Chen, X., and Chen, Z. (2021). Can green finance development reduce carbon emissions? Empirical evidence from 30 Chinese provinces. Sustainability 13, 12137. doi:10.3390/su132112137
Chen, X., Di, Q., Hou, Z., and Yu, Z. (2022a). Measurement of carbon emissions from marine fisheries and system dynamics simulation analysis: China’s northern marine economic zone case. Mar. Policy 145, 105279. doi:10.1016/j.marpol.2022.105279
Chen, X., Wei, Y., and Zhang, N. (2022b). Thinking on accelerating the development of green insurance to help our country's green transformation. Shanghai Insur. Mon. 12.
Chun, T., Wang, S., Xue, X., Xin, H., Gao, G., Wang, N., et al. (2023). Decomposition and decoupling analysis of multi-sector CO2 emissions based on LMDI and Tapio models: case study of Henan Province, China. Environ. Sci. Pollut. Res. 30, 88508–88523. doi:10.1007/s11356-023-28609-3
Du, G. (2023). Nexus between green finance, renewable energy, and carbon intensity in selected Asian countries. J. Clean. Prod. 405, 136822. doi:10.1016/j.jclepro.2023.136822
Dwivedi, A., Sassanelli, C., Agrawal, D., Gonzalez, E. S., and D'Adamo, I. (2023). Technological innovation toward sustainability in manufacturing organizations: a circular economy perspective. Sustain. Chem. Pharm. 35, 101211. doi:10.1016/j.scp.2023.101211
Eyup, D., and Roula, I.-L. (2020). The impact of economic structure to the environmental Kuznets curve (EKC) hypothesis: evidence from European countries. Environ. Sci. Pollut. Res. Int. 27, 12717–12724. doi:10.1007/s11356-020-07878-2
Fang, X., and Gao, S. (2023). An empirical study on relationship between island ecological environment and socio-economic development from perspective of environmental Kuznets curve (EKC). Ocean. Coast. Manage. 244, 106819. doi:10.1016/j.ocecoaman.2023.106819
Francey, R. J., Trudinger, C. M., Van Der Schoot, M., Law, R. M., Krummel, P. B., Langenfelds, R. L., et al. (2013). Atmospheric verification of anthropogenic CO2 emission trends. Nat. Clim. Chang. 3, 520–524. doi:10.1038/nclimate1817
Friedl, B., and Getzner, M. (2003). Determinants of CO2 emissions in a small open economy. Ecol. Econ. 45, 133–148. doi:10.1016/s0921-8009(03)00008-9
Gao, X., and Wei, L. (2022). How green technology innovation contributes to green development in Yellow River Basin: a new interpretation based on the connotation of EKC inflection point. J. Gansu Adm. Univ. 152, 90–102+127.
Gokmenoglu, K., and Taspinar, N. (2018). Testing the agriculture-induced EKC hypothesis: the case of Pakistan. Environ. Sci. Pollut. Res. Int. 25, 22829–22841. doi:10.1007/s11356-018-2330-6
Grossman, G. M., and Krueger, A. B. (1991). Environmental impacts of a north American free trade agreement. Natl. Bureau Econ. Res., 3914.
Guan, H., Sun, Z., and Wang, J. (2022). Decoupling analysis of net carbon emissions and economic growth of marine aquaculture. Sustainability 14, 5886. doi:10.3390/su14105886
Guangliang, L., Yang, X., Wenfeng, G., Su, X., Shen, B., Xiaodong, Y., et al. (2023). How can marine fishery enable low carbon development in China? Based on system dynamics simulation analysis. Ocean. Coast. Manage. 231, 106382. doi:10.1016/j.ocecoaman.2022.106382
Guo, L., Zhao, S., Song, Y., Tang, M., and Li, H. (2022). Green finance, chemical fertilizer use and carbon emissions from agricultural production. Agricultural 12, 313. doi:10.3390/agriculture12030313
Hao, Y., Zhang, T., Jing, L., and Xiao, L. (2019). Would the decoupling of electricity occur along with economic growth? Empirical evidence from the panel data analysis for 100 Chinese cities. Energy 180, 615–625. doi:10.1016/j.energy.2019.05.014
Hu, Y., and Zheng, J. (2022). How does green credit affect carbon emissions in China? A theoretical analysis framework and empirical study. Environ. Sci. Pollut. Res. 29, 59712–59726. doi:10.1007/s11356-022-20043-1
Huang, Y., Chen, C., Lei, L., and Zhang, Y. (2022). Impacts of green finance on green innovation: a spatial and nonlinear perspective. J. Clean. Prod. 365, 132548. doi:10.1016/j.jclepro.2022.132548
IPCC (2019). IPCC special report on the ocean and cryosphere in a changing climate. Switzerland: IPCC.
Jaffe, A. B., Newell, R. G., and Stavins, R. N. (2005). A tale of two market failures: technology and environmental policy. Ecol. Econ. 54, 164–174. doi:10.1016/j.ecolecon.2004.12.027
Jahanger, A., Hossain, M. R., Onwe, J. C., Ogwu, S. O., Awan, A., and Balsalobre-Lorente, D. (2023). Analyzing the N-shaped EKC among top nuclear energy generating nations: a novel dynamic common correlated effects approach. Gondwana Res. 116, 73–88. doi:10.1016/j.gr.2022.12.012
Kaika, D., and Zervas, E. (2013a). The Environmental Kuznets Curve (EKC) theory—Part A: concept, causes and the CO2 emissions case. Energy Policy 62, 1392–1402. doi:10.1016/j.enpol.2013.07.131
Kaika, D., and Zervas, E. (2013b). The environmental Kuznets curve (EKC) theory. Part B: critical issues. Energy Policy 62, 1403–1411. doi:10.1016/j.enpol.2013.07.130
Kaufmann, R. K., Davidsdottir, B., Garnham, S., and Pauly, P. (1998). The determinants of atmospheric SO2 concentrations: reconsidering the environmental Kuznets curve. Ecol. Econ. 25, 209–220. doi:10.1016/s0921-8009(97)00181-x
Khan, Z., Hussain, M., Shahbaz, M., Yang, S., and Jiao, Z. (2020). Natural resource abundance, technological innovation, and human capital nexus with financial development: a case study of China. Resour. Pol. 65, 101585. doi:10.1016/j.resourpol.2020.101585
Klapkiv, L., and Ülgen, F. (2023). Green products in the insurance market. Wiadomości Ubezpieczeniowe 2. doi:10.33995/wu2023.2.4
Krabbe, N., Langlet, D., Belgrano, A., and Villasante, S. (2022). Reforming international fisheries law can increase blue carbon sequestration. Front. Mar. Sci. 9, 800972. doi:10.3389/fmars.2022.800972
Kumar, B., Kumar, L., Kumar, A., Kumari, R., Tagar, U., and Sassanelli, C. (2023). Green finance in circular economy: a literature review. Environ. Dev. Sustain., 1–41. doi:10.1007/s10668-023-03361-3
Kumar, L., Nadeem, F., Sloan, M., Restle-Steinert, J., Deitch, M. J., Ali Naqvi, S., et al. (2022). Fostering green finance for sustainable development: a focus on textile and leather small medium enterprises in Pakistan. Sustainability 14, 11908. doi:10.3390/su141911908
Lantz, V., and Feng, Q. (2006). Assessing income, population, and technology impacts on CO2 emissions in Canada: where's the EKC? Ecol. Econ. 57, 229–238. doi:10.1016/j.ecolecon.2005.04.006
Li, C., Jia, H., Wan, Y., Hu, Y., Zeng, B., Zhang, W., et al. (2023a). The impacts of extreme marine weather and marine scientific and technological innovation on marine economic development: evidence form China’s coastal regions. Front. Mar. Sci. 10, 1104045. doi:10.3389/fmars.2023.1104045
Li, C., Solangi, Y. A., and Ali, S. J. S. (2023b). Evaluating the factors of green finance to achieve carbon peak and carbon neutrality targets in China: a delphi and fuzzy AHP approach. Sustainability 15, 2721. doi:10.3390/su15032721
Li, S., Ding, J., and Sui, Y. (2023c). Mechanism and path of the value realization of blue carbon sink of coastal wetlands in China from perspective of carbon sink tradin. Mar. Environ. Sci. 42, 55–63.
Li, W., Fan, J., and Zhao, J. (2022a). Has green finance facilitated China’s low-carbon economic transition? Environ. Sci. Pollut. Res. 29, 57502–57515. doi:10.1007/s11356-022-19891-8
Li, Z., Zhang, L., Wang, W., and Ma, W. (2022b). Assessment of carbon emission and carbon sink capacity of China’s marine fishery under carbon neutrality target. J. Mar. Sci. Eng. 10, 1179. doi:10.3390/jmse10091179
Liang, J., and Song, X. (2022). Can green finance improve carbon emission efficiency? Evidence from China. Front. Environ. Sci. 10, 955403. doi:10.3389/fenvs.2022.955403
Lin, B., and Ouyang, X. (2014). Energy demand in China: comparison of characteristics between the US and China in rapid urbanization stage. Energy Convers. manage. 79, 128–139. doi:10.1016/j.enconman.2013.12.016
Lin, Z., Wang, H., Li, W., and Chen, M. (2023). Impact of green finance on carbon emissions based on a two-stage LMDI decomposition method. Sustainability 15, 12808. doi:10.3390/su151712808
Liu, C., Sun, Z., and Zhang, J. (2019). Research on the effect of carbon emission reduction policy in China's carbon emissions trading pilot. China Population,Resources Environ. 29, 49–58.
Liu, G., Xu, Y., Ge, W., Yang, X., Su, X., Shen, B., et al. (2023a). How can marine fishery enable low carbon development in China? Based on system dynamics simulation analysis. Ocean. Coast. Manage. 231, 106382. doi:10.1016/j.ocecoaman.2022.106382
Liu, L., Cheng, Y., Guan, N., Liu, Y., Zhang, X., Li, Y., et al. (2023b). Impact of COVID-19 and green finance on transportation energy carbon emissions in China: from the perspective of an automobile energy consumption structure. Front. Environ. Sci. 11, 1138111. doi:10.3389/fenvs.2023.1138111
Liu, Q., and Xiao, H. (2022). The dynamic relationship between different income types and carbon emissions from livestock and poultry breeding in rural China: an analysis based on Tapio and SVAR model. J. Ecol. Ru. Environ. 38, 453–465.
Lorente, D., and Alvarez-Herranz, A. (2016). An approach to the effect of energy innovation on environmental Kuznets curve: an introduction to inflection point. Bul. Energy Econ. 4, 224–233.
Lu, Q., Liang, F., Bi, X., Duffy, R., and Zhao, Z. (2011). Effects of urbanization and industrialization on agricultural land use in Shandong Peninsula of China. Ecol. Indic. 11, 1710–1714. doi:10.1016/j.ecolind.2011.04.026
Luo, H., Li, L., Lei, Y., Wu, S., Yan, D., Fu, X., et al. (2020). Decoupling analysis between economic growth and resources environment in Central Plains Urban Agglomeration. Sci. Total Environ. 752, 142284. doi:10.1016/j.scitotenv.2020.142284
Meo, M. S., and Karim, M. Z. A. (2022). The role of green finance in reducing CO2 emissions: an empirical analysis. Borsa istanb. Rev. 22, 169–178. doi:10.1016/j.bir.2021.03.002
Ngo, Q.-T., Tran, H. A., and Tran, H. T. T. (2022). The impact of green finance and Covid-19 on economic development: capital formation and educational expenditure of ASEAN economies. China Financ. Rev. Int. 12, 261–279. doi:10.1108/cfri-05-2021-0087
Parker, R. W., Blanchard, J. L., Gardner, C., Green, B. S., Hartmann, K., Tyedmers, P. H., et al. (2018). Fuel use and greenhouse gas emissions of world fisheries. Nat. Clim. Chang. 8, 333–337. doi:10.1038/s41558-018-0117-x
Patel, S. H., Pinckney, T. C., and Jaeger, W. K. (1995). Smallholder wood production and population pressure in east africa: evidence of an environmental Kuznets curve? Land Econ. 71, 516. doi:10.2307/3146715
Qadri, S. U., Li, M., Anees, A., Ali, M. S. E., Brancu, L., Nayel, A. N., et al. (2023). Green finance and foreign direct investment–environmental sustainability nexuses in emerging countries: new insights from the environmental Kuznets curve. Front. Environ. Sci. 11, 1074713. doi:10.3389/fenvs.2023.1074713
Ren, S., and Hu, Z. (2012). Effects of decoupling of carbon dioxide emission by Chinese nonferrous metals industry. Energy Policy 43, 407–414. doi:10.1016/j.enpol.2012.01.021
Ren, X., Shao, Q., and Zhong, R. (2020). Nexus between green finance, non-fossil energy use, and carbon intensity: empirical evidence from China based on a vector error correction model. J. Clean. Prod. 277, 122844. doi:10.1016/j.jclepro.2020.122844
Ren, Y., Yu, J., Xu, S., Tang, J., and Zhang, C. (2023). Green finance and industrial low-carbon transition: evidence from a quasi-natural experiment in China. Sustainability 15, 4827. doi:10.3390/su15064827
Selden, T., and Daqing, S. (1994). Environmental quality and development: is there a Kuznets curve for air pollution emissions? J. Environ. Econ. Manage. 27, 147–162. doi:10.1006/jeem.1994.1031
Shao, G., Chu, R., and Li, C. (2018). Research on carbon balance of marine fishery in Shandong Province using the calculation results of carbon emission and carbon sink. Chin. Fish. Econ., 105032.
Sinha, A., Shahbaz, M., and Balsalobre, D. (2017). Exploring the relationship between energy usage segregation and environmental degradation in N-11 countries. J. Clean. Prod. 168, 1217–1229. doi:10.1016/j.jclepro.2017.09.071
Su, C., Song, Y., and Umar, M. (2021). Financial aspects of marine economic growth: from the perspective of coastal provinces and regions in China. Ocean. Coast. Manage. 204, 105550. doi:10.1016/j.ocecoaman.2021.105550
Sun, C., Wang, L., Zou, W., and Zhai, X. (2023). The high-quality development level assessment of marine economy in China based on a “2+ 6+ 4” framework. Ocean. Coast. Manage. 244, 106822. doi:10.1016/j.ocecoaman.2023.106822
Tapio, P. (2005). Towards a theory of decoupling: degrees of decoupling in the EU and the case of road traffic in Finland between 1970 and 2001. Transp. Policy 12, 137–151. doi:10.1016/j.tranpol.2005.01.001
Torras, M., and Boyce, J. K. (1998). Income, inequality, and pollution: a reassessment of the environmental Kuznets curve. Ecol. Econ. 25, 147–160. doi:10.1016/s0921-8009(97)00177-8
Tyedmers, P. H., Watson, R., and Pauly, D. (2005). Fueling global fishing fleets. AMBIO J. Hum. Environ. 34, 635–638. doi:10.1579/0044-7447-34.8.635
Wang, B., Ni, G., and Han, L. (2019). Impact of the evolution of industry structure on the economic fluctuation of marine fishery. Resour. Sci. 41, 289–300.
Wang, C., Nie, P.-y., Peng, D.-h., and Li, Z. h. (2017). Green insurance subsidy for promoting clean production innovation. J. Clean. Prod. 148, 111–117. doi:10.1016/j.jclepro.2017.01.145
Wang, K., Zhu, Y., and Zhang, J. (2021). Decoupling economic development from municipal solid waste generation in China’s cities: assessment and prediction based on Tapio method and EKC models. Waste Manage 133, 37–48. doi:10.1016/j.wasman.2021.07.034
Wang, Q., and Wang, S. (2022). Carbon emission and economic output of China’s marine fishery–a decoupling efforts analysis. Mar. Policy 135, 104831. doi:10.1016/j.marpol.2021.104831
Wang, Q., and Zhang, F. (2020). Does increasing investment in research and development promote economic growth decoupling from carbon emission growth? An empirical analysis of BRICS countries. J. Clean. Prod. 252, 119853. doi:10.1016/j.jclepro.2019.119853
Wang, Q., and Zhang, F. (2021). The effects of trade openness on decoupling carbon emissions from economic growth – evidence from 182 countries. J. Clean. Prod. 279, 123838. doi:10.1016/j.jclepro.2020.123838
Wang, Y., Guo, T., Cheng, T., and Wang, N. (2022). Evolution of blue carbon trading of China's marine ranching under the blue carbon special subsidy mechanism. Ocean. Coast. Manage 222, 106123. doi:10.1016/j.ocecoaman.2022.106123
Wu, J., and Li, B. (2022). Spatio-temporal evolutionary characteristics of carbon emissions and carbon sinks of marine industry in China and their time-dependent models. Mar. Policy 135, 104879. doi:10.1016/j.marpol.2021.104879
Xu, L., Liu, Y., Zhang, B., and Xiang, B. (2023a). Study on the impact of green finance on low carbon development of manufacturing industry from the perspective of multidimensional space: evidence from China. Environ. Sci. Pollut. Res. 30, 50772–50782. doi:10.1007/s11356-023-25690-6
Xu, S., and Gao, K. (2022). Green finance and high-quality development of marine economy. Mar. Econ. Manage. 5, 213–227. doi:10.1108/maem-01-2022-0001
Xu, Y., Ge, W., Liu, G., Su, X., Zhu, J., Yang, C., et al. (2023b). The impact of local government competition and green technology innovation on economic low-carbon transition: new insights from China. Environ. Sci. Pollut. Res. 30, 23714–23735. doi:10.1007/s11356-022-23857-1
Yang, L., and Su, X. (2010). Study on the objective and implementation path of Marine fishery industrial structure optimization and upgrading from the perspective of industrial ecology. Iss. Agr. Econ. 10, 99–105.
Yang, Y., Su, X., and Yao, S. (2021). Nexus between green finance, fintech, and high-quality economic development: empirical evidence from China. Resour. Pol. 74, 102445. doi:10.1016/j.resourpol.2021.102445
Ye, J., Xu, W., and Hu, L. (2023). Digital inclusive finance, consumption structure upgrading and carbon emissions. Front. Environ. Sci. 11. doi:10.3389/fenvs.2023.1282784
Yi, Y., Wu, Z., and Ma, H. (2022). Research on the impact of China's pilot carbon emission trading policy on regional carbon decoupling effect. Shanghai Finance 05, 70–79.
Yin, J., Zheng, M., and Chen, J. (2015). The effects of environmental regulation and technical progress on CO2 Kuznets curve: an evidence from China. Energy Policy 77, 97–108. doi:10.1016/j.enpol.2014.11.008
York, R., Rosa, E. A., and Dietz, T. (2003). STIRPAT, IPAT and ImPACT: analytic tools for unpacking the driving forces of environmental impacts. Ecol. Econ. 46, 351–365. doi:10.1016/s0921-8009(03)00188-5
Yu, C.-H., Wu, X., Zhang, D., Chen, S., and Zhao, J. (2021). Demand for green finance: resolving financing constraints on green innovation in China. Energy Policy 153, 112255. doi:10.1016/j.enpol.2021.112255
Yuan, L., Tianhui, L., Jinshui, L., Faxiang, L., and Changfa, L. (2023). Carbon budget and driving factors in marine fisheries in Liaoning Province, China. Chin. J. Eco-Agriculture 31, 253–264.
Zhang, H., Geng, C., and Wei, J. (2022a). Coordinated development between green finance and environmental performance in China: the spatial-temporal difference and driving factors. J. Clean. Prod. 346, 131150. doi:10.1016/j.jclepro.2022.131150
Zhang, J., Li, F., and Ding, X. (2022b). Will green finance promote green development: based on the threshold effect of R&D investment. Environ. Sci. Pollut. Res. 29, 60232–60243. doi:10.1007/s11356-022-20161-w
Zhang, P., Zhang, L., Tian, X., Hao, Y., and Wang, C. (2018). Urban energy transition in China: insights from trends, socioeconomic drivers, and environmental impacts of Beijing. Energy Policy 117, 173–183. doi:10.1016/j.enpol.2018.02.039
Zhang, W., Zhu, Z., Liu, X., and Cheng, J. (2022c). Can green finance improve carbon emission efficiency? Environ. Sci. Pollut. Res. 29, 68976–68989. doi:10.1007/s11356-022-20670-8
Zhang, X., Cheng, J., and Zheng, S. (2023a). Can multi-agent cooperation promote the ecological value realization of blue carbon in marine ranching? Heliyon 9, e18572. doi:10.1016/j.heliyon.2023.e18572
Zhang, X., Ye, S., and Shen, M. (2023). Driving factors and spatiotemporal characteristics of CO2 emissions from marine fisheries in China: a commonly neglected carbon-intensive sector. Int. J. Environ. Res. 20, 883. doi:10.3390/ijerph20010883
Zhang, Y.-J., and Da, Y.-B. (2015). The decomposition of energy-related carbon emission and its decoupling with economic growth in China. Renew. Sust. Energ. Rev. 41, 1255–1266. doi:10.1016/j.rser.2014.09.021
Zheng, H., Li, J., and Zhao, X. (2021). How does financial policy support the development of China's fishery? Characteristics, experience and prospects. Mar. Policy 132, 104678. doi:10.1016/j.marpol.2021.104678
Keywords: carbon decoupling, marine fishery, green finance, Tapio model, EKC model
Citation: Xu W and Zhu Y (2024) The effects of green finance on the carbon decoupling of marine fishery: analysis based on Tapio method and EKC model. Front. Environ. Sci. 12:1320318. doi: 10.3389/fenvs.2024.1320318
Received: 30 October 2023; Accepted: 23 February 2024;
Published: 13 March 2024.
Edited by:
Claudio Sassanelli, Politecnico di Bari, ItalyReviewed by:
Zhang Hongzhi, Shandong Foreign Trade Vocational College, ChinaCopyright © 2024 Xu and Zhu. This is an open-access article distributed under the terms of the Creative Commons Attribution License (CC BY). The use, distribution or reproduction in other forums is permitted, provided the original author(s) and the copyright owner(s) are credited and that the original publication in this journal is cited, in accordance with accepted academic practice. No use, distribution or reproduction is permitted which does not comply with these terms.
*Correspondence: Weicheng Xu, eHV3ZWljaGVuZ0BvdWMuZWR1LmNu
Disclaimer: All claims expressed in this article are solely those of the authors and do not necessarily represent those of their affiliated organizations, or those of the publisher, the editors and the reviewers. Any product that may be evaluated in this article or claim that may be made by its manufacturer is not guaranteed or endorsed by the publisher.
Research integrity at Frontiers
Learn more about the work of our research integrity team to safeguard the quality of each article we publish.