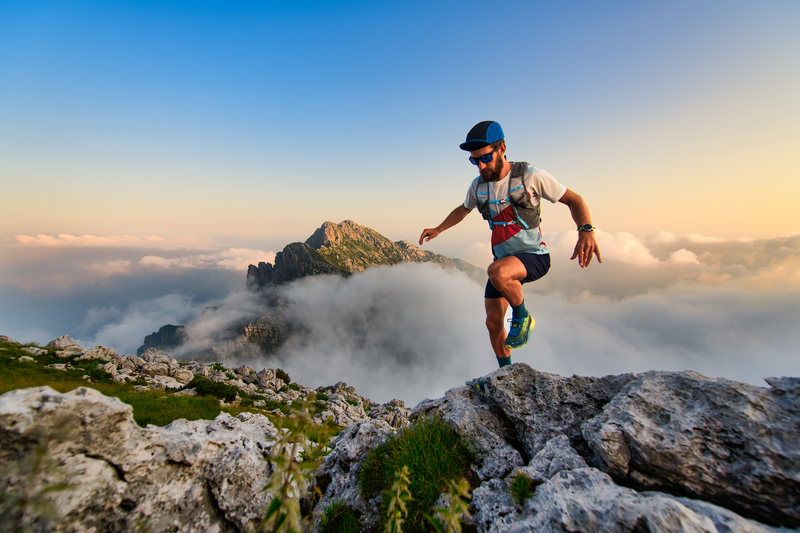
94% of researchers rate our articles as excellent or good
Learn more about the work of our research integrity team to safeguard the quality of each article we publish.
Find out more
ORIGINAL RESEARCH article
Front. Environ. Sci. , 13 June 2024
Sec. Big Data, AI, and the Environment
Volume 12 - 2024 | https://doi.org/10.3389/fenvs.2024.1268603
With the global goal of carbon neutrality being emphasized, the implementation of carbon-neutral strategies has become a crucial task across various domains. As an integral part of social activities, physical education also necessitates considerations on how to reduce carbon emissions and implement carbon-neutral strategies within the teaching process. This study focuses on physical education and explores carbon-neutral strategies based on an end-to-end architecture with an attention mechanism. Firstly, we introduce an end-to-end framework that enables the integration and optimization of various aspects within the teaching process to achieve comprehensive carbon-neutral objectives. This framework serves as a unified optimization platform, facilitating the collaboration of different components involved in teaching activities and balancing the reduction of carbon emissions with teaching effectiveness. Secondly, we employ Convolutional Neural Networks (CNN) as the foundational model within the end-to-end architecture. Through training the CNN model, we automate the analysis of carbon emissions during the teaching process and provide corresponding carbon-neutral recommendations for different segments. Most importantly, we incorporate an attention mechanism to enhance the effectiveness and interpretability of the carbon-neutral strategy. The attention mechanism assists the model in automatically focusing on features or regions closely related to carbon-neutral objectives, thereby achieving more accurate and efficient carbon-neutral strategy recommendations. Finally, we conduct training and testing on the proposed model using a dataset constructed from carbon-neutral scenarios in physical education (the country where physical education occurred and digital energy have been scrutinized). The results demonstrate that the improved model surpasses a 90% threshold in mainstream evaluation metrics such as Action Recognition Accuracy (ARA), Action Recognition Recall (ARR), and Action Optimization Rate (AOR). The enhanced model exhibits notable improvements in inference speed and accuracy.
As global climate change and environmental issues become increasingly severe, humanity is confronted with unprecedented challenges. Issues such as rising global temperatures, glacier melting, sea-level rise, and frequent extreme weather events have already demonstrated significant impacts, drawing widespread attention on a global scale (Atwoli et al., 2021; Kyriakopoulos and Sebos 2023). Carbon neutrality, as a comprehensive measure to combat climate change, holds tremendous significance. Its core idea is to achieve a balance between carbon emissions and carbon absorption by reducing greenhouse gas emissions or increasing carbon sequestration (He et al., 2023). This results in zero or even negative growth of greenhouse gas emissions while developing the economy and society. This means that we can continue to develop without further adverse impacts on the climate system, or even gradually restore and repair the environmental damage already caused.
Although the global awareness of the significant impact of carbon emissions on climate change is widespread, countries have set carbon neutrality goals and taken active measures to reduce carbon emissions. However, research and practical implementation of carbon neutrality in the field of physical education remain relatively scarce. Sports activities have often been perceived in the past as social events unrelated to environmental conservation. Firstly, people tend to underestimate the potential scale of carbon emissions generated during physical education activities. In aspects such as stadium lighting, sports equipment production, and student transportation, physical education activities may lead to significant carbon footprints that should not be overlooked. Secondly, the education sector, especially physical education, is generally less mentioned in discussions about carbon neutrality. This exclusion may result in an underestimation of the overall potential for carbon neutrality. As we recognize that physical education is an integral part of education, it should actively participate in carbon neutrality efforts to contribute to building a low-carbon society (Liu et al., 2021). The process of physical education directly or indirectly generates considerable carbon emissions, as traditional teaching methods heavily rely on physical teaching aids and facilities, leading to greenhouse gas emissions during their production, use, and disposal processes. At the same time, effective physical education can help cultivate students’ awareness and habits of low-carbon living, which is essential for achieving sustainable development (Nawi et al., 2019). Therefore, it is necessary to delve into the factors influencing carbon emissions in physical education and explore scientific carbon neutrality strategies to reduce emissions and promote sustainable development in physical education.
Currently, research on carbon neutrality strategies in physical education is still in its early stages. As a part of social activities, physical education is often marginalized and considered to contribute insignificantly to global carbon neutrality goals. Moreover, there exists a knowledge gap between the field of sports and areas such as environmental science and climate change. Consequently, researchers tend to concentrate more on other fields more directly associated with carbon emissions. Scholars and researchers may overlook opportunities to explore carbon-neutral strategies in the field of physical education due to a lack of understanding of each other’s domains. Nowadays, scholars both domestically and internationally have begun to pay attention to the application of carbon neutrality in physical education, but relevant studies are still relatively limited (Ezeonu et al., 2023). Existing research mainly focuses on theoretical discussions of carbon neutrality (Chapman et al., 2022), the application of carbon emission reduction technologies (Wei and Wang, 2021), and the evaluation of carbon neutrality strategies (Rosa et al., 2021). The application of carbon neutrality strategies in physical education aims to achieve carbon neutrality goals by optimizing the teaching process, improving facilities and equipment, and promoting energy transition. For instance, introducing low-carbon sports equipment and facilities, promoting eco-friendly modes of transportation such as walking and cycling, and advocating green and sustainable teaching concepts (Dällenbach 2020) are all important approaches to promoting carbon neutrality in physical education. At the same time, conducting research on carbon neutrality strategies will also facilitate the development of eco-friendly and sustainable physical education, cultivating students’ environmental awareness and concepts of sustainable development (Tang, 2022). However, as a specialized field, carbon neutrality in physical education involves numerous factors, such as carbon emissions during the teaching process, the carbon footprint of sports venues and equipment, and carbon emissions from students’ participation in physical activities. Therefore, exploring and applying carbon neutrality strategies comprehensively in physical education, as well as evaluating their effectiveness and feasibility, remain pressing issues. Moreover, implementing carbon neutrality strategies in physical education also faces several challenges. Firstly, physical education encompasses multiple aspects, including teaching processes, equipment usage, and venue construction, necessitating carbon emission reductions from various angles; Secondly, students’ environmental awareness and behavior habits also influence the implementation of carbon neutrality; Lastly, the implementation of carbon neutrality strategies requires certain economic and human resources (Wang et al., 2020), and achieving a balance between economic and environmental benefits in practice is also a crucial concern (Wang et al., 2019). Moreover, in the field of architecture, as an important energy consumption point, the energy consumption problem of school buildings is increasingly attracting attention Kyriakopoulos et al. (2022); (Ntanos et al., 2022).
In order to deal with many challenges faced by physical education in the stage of carbon-neutral strategies and methods (Chenm, 2022), such as the lack of a complete subject framework and knowledge system, and the establishment of a scientific and operable evaluation index system, etc. (Wang et al. 20221b). To this end, we propose a carbon-neutral strategy method based on an end-to-end attention mechanism, taking into account the entire process of physical education teaching (Zhang et al., 2022). The framework covers the teaching process, equipment use, and student participation to ensure comprehensive coverage and effective implementation of carbon-neutral strategies. Integrate the entire process of physical education teaching through an end-to-end framework. At the same time, in order to improve the pertinence of the carbon neutral strategy, we introduce an attention mechanism (Wang et al., 2023b). This mechanism can automatically identify the key carbon emission links in physical education teaching, so that we can more accurately determine the focus and priority of carbon emission reduction, thereby improving the pertinence and effectiveness of carbon neutral strategies (Zheng et al., 2022b). Finally, in the model, we employ a convolutional neural network to extract features of carbon emissions in physical education teaching. By applying the carbon-neutral strategy method of end-to-end architecture and attention mechanism in physical education, we can better adapt to different teaching scenarios and needs, and realize the deep integration of carbon-neutral strategy and physical education process, thereby promoting physical education Teaching is developing in a green and sustainable direction, providing valuable reference and suggestions for carbon-neutral strategies in the energy transition driven by digital technology (Fu, 2023). This research result is expected to contribute to the carbon neutrality and sustainable development in the field of physical education teaching, and promote the realization of global energy transformation and carbon neutrality goals.
The contributions of this paper can be summarized in the following three aspects:
1. In this study, we adopted an end-to-end framework, integrating carbon neutrality strategies with the entire process of physical education. Through the end-to-end framework, we consolidated various aspects and data sources in the teaching process, from data collection and feature extraction in physical education to the recommendation and application of carbon neutrality strategies, achieving full automation and optimization throughout the entire process.
2. The attention mechanism allowed the model to automatically identify key aspects closely related to carbon neutrality goals in physical education. By endowing the model with the ability to focus on critical aspects, the attention mechanism enabled targeted recommendations and optimizations for carbon neutrality key points, thereby enhancing the precision and effectiveness of carbon neutrality strategies.
3. Leveraging the excellent feature extraction capabilities of CNN, we could automatically extract crucial features related to carbon emissions from image data in physical education scenarios. By combining CNN with attention mechanisms and the end-to-end framework, we achieved comprehensive learning and optimization of carbon neutrality strategies, providing powerful technical support for achieving carbon neutrality goals in physical education.
The logical structure of this paper is as follows: In Section 2, we review the relevant literature in this research field both domestically and internationally, with a focus on the latest advancements in carbon neutrality and a comparison of the strengths and weaknesses of different methods. In Section 3, we elaborate on the proposed research methodology, including the concept and specific implementation of the end-to-end framework, CNN model, and attention mechanism. Section 4 provides a detailed description of the experimental design, including the dataset used, its features, experimental settings, evaluation metrics, and computation methods. Moreover, we conduct a thorough analysis and comparison of the experimental results obtained from different methods. In Section 5, we discuss the results of the experiments, highlighting the strengths, limitations, and potential improvements of our model. Section 6 concludes the paper by summarizing the main work, innovations, and contributions to the field, while also providing an outlook on future research directions.
Against the backdrop of global carbon neutrality goals, the implementation of carbon reduction strategies has become an urgent task across various domains, including physical education, as a significant societal activity. Reducing carbon emissions and implementing carbon offset strategies in physical education not only contribute to environmental protection but also align with the requirements of sustainable development. Therefore, this study focuses on physical education and explores a carbon offset strategy method based on an end-to-end architecture with an attention mechanism.
In previous studies, relevant research has been conducted in the fields of carbon neutrality, deep learning (Wu et al., 2024; Zheng et al. 2022a; Dai et al. 2023b), and physical education, which have provided valuable references and insights for our exploration in this paper. For instance, in literature (Zhu et al. 2023), a comprehensive approach integrating engineering, artificial intelligence, and hybrid methods is employed for building energy usage prediction. The focus is on utilizing historical data to forecast future energy consumption, supporting carbon-neutral strategies. However, there is a demand for intelligent solutions to improve sustainability and efficiency in the field of sports facilities. In literature (Elnour et al. 2022a), addressing sustainability and efficiency concerns in sports facilities involves a comprehensive integration of intelligent and effective solutions. The emphasis is on operational management, sustainability, and energy optimization aspects. However, there is a research gap in the study of sports facilities in hot climate zones compared to the abundance of research in cold climate zones, highlighting the need for more balanced investigations. Existing research predominantly focuses on popular facilities like swimming pools, leaving other types insufficiently covered. Under the influence of China’s dual-carbon strategy, the education sector, being a high carbon-consumption domain, holds the potential to reduce carbon emissions. The advantages of digital teaching not only enhance the quality of education but also contribute to carbon emission reduction. However, a comprehensive understanding of the impact of digital teaching on carbon reduction requires more comparative analysis and survey investigations, posing a significant challenge. Xinfa et al. (2023) In the Building Automation and Management System (BAMS), artificial intelligence-based big data analytics tools offer tailored solutions for heating, ventilation, and air conditioning systems (Himeur et al., 2023). However, a primary limitation lies in their focus on controlling these systems, leaving other tasks requiring manual operation challenges. Training machine learning algorithms in energy systems faces challenges, and transfer learning is considered a prospective approach to address these issues. Despite showcasing its application in energy prediction and sports facility load forecasting through case studies, there is still a need to overcome technical and methodological challenges. The field of sports education holds the potential to foster critical and systemic thinking, contributing to sustainable development goals. However, specific strategies to achieve this goal require further in-depth research and practical implementation. To meet the demands of global higher education institutions transitioning to carbon-neutral campuses, this study proposes a theoretical framework based on social, technical, and ecological dimensions (Elnour et al., 2022b). It provides implementation strategies and envisions constructing a carbon-neutral intelligent service platform, validated through a case study at Shanghai Jiao Tong University’s Fahua campus (Wang et al. 2023a). The limitations of these methods include the concentration of artificial intelligence-based big data analytics tools in BAMS on controlling heating, ventilation, and air conditioning systems. Transfer learning in energy systems still needs to overcome technical and methodological challenges (Baena-Morales et al., 2023). Further research is required in the sports education field to implement specific strategies for cultivating critical and systemic thinking. Additionally, achieving carbon-neutral campuses necessitates addressing specific issues across social, technical, and ecological dimensions (Himeur et al., 2022). In reference (Köhl et al., 2020), a carbon balance evaluation index was proposed to study the feasibility of biomass energy as a carbon-neutral alternative. This research provides a theoretical foundation and practical guidance for the carbon neutrality strategy in physical education, helping us consider how to integrate and optimize various aspects of physical education to achieve comprehensive carbon neutrality goals. In reference (Minx et al., 2018), a comparative analysis focused on negative emission technologies, particularly the carbon neutrality effect of BECCS technology. Although the research target differs, it has guiding significance for finding carbon neutrality measures and transformation paths in our study. It reminds us that the combination of negative emission technologies and carbon neutrality strategies can bring about more positive carbon balance effects in physical education activities. Furthermore, reference (Hou et al., 2023) investigated the technological paths and policy suggestions for achieving carbon neutrality in the cement industry. Although the research object is different, it holds practical significance for our efforts to adapt carbon neutrality strategies to Chinese physical education practices. The study offers specific recommendations tailored to particular industries and energy structures, providing insights for formulating carbon neutrality strategies in physical education. Additionally, in reference (Wang et al., 2021), an energy system model was used to analyze scenarios for achieving carbon neutrality in China’s energy system. Although the research method varies, the scenario analysis provides a scientific basis for devising carbon neutrality policies and measures in our study. It suggests that similar system simulation methods can be employed in physical education to predict the timeline and emission levels of carbon neutrality targets. Moreover, in reference (Wan et al., 2022), a novel water quality prediction model called CSWLSTM-GPR based on deep learning and Gaussian process regression was proposed for monitoring carbon neutrality in the treatment of papermaking wastewater. The model demonstrated high accuracy and reliability in predicting effluent COD and suspended solids (SS). In reference (Wang 2022), a deep learning-based model was developed for the coordinated optimization of active and reactive power in the electric interconnection system under the “carbon neutrality” target, achieving safe and low-carbon economic dispatch of the system. Furthermore, in reference (Huang et al., 2021), deep learning was applied to predict carbon emission information in the Yangtze River Economic Belt, where support vector regression (SVR) combined with long short-term memory neural networks demonstrated outstanding predictive performance. Overall, these studies collectively demonstrate the potential of deep learning methods in addressing complex problems and achieving carbon neutrality goals (Dai et al., 2023a). They offer valuable insights and methods in the fields of carbon neutrality and deep learning. Although the specific focus and application contexts differ from this study, the research conducted in these areas has inspired us to explore carbon neutrality strategies in physical education. The success and advantages of deep learning technology in other domains lead us to believe that it also holds great potential for application in carbon neutrality practices in physical education.
The above-mentioned literature collectively illustrates the potential of deep learning methods in addressing complex issues and achieving carbon neutrality goals. Their studies provide crucial insights and methods for the fields of carbon neutrality and deep learning. Furthermore, in response to the challenges of carbon neutrality strategies, the European Commission has taken a series of measures to promote the transition to low-carbon energy in Europe, aiming to reduce greenhouse gas emissions. The significance of sustainable energy development for global progress emphasizes sustainability challenges such as greenhouse gas emissions and climate change (Streimikiene et al., 2022). This transition to sustainable energy requires the formulation of an international strategic roadmap, including technological changes such as improving energy efficiency, utilizing renewable energy, and reducing greenhouse gas emissions. Amid the impact of the current economic downturn, the education sector has become a highly influential area of socio-economic activity. Based on this understanding, our research aims to explore carbon-neutral strategies applicable to sports education by drawing on the deep learning methods and models presented in the literature above, considering the specific characteristics and needs of sports teaching (Kyriakopoulos 2021). Given the successes and advantages of deep learning techniques in other fields (Štreimikienė et al. 2021), we believe they hold potential for practical applications in carbon neutrality practices within physical education.
In this study, we integrate the achievements of previous research, combining knowledge from the carbon neutrality and deep learning domains to apply them innovatively in the field of physical education. By utilizing an end-to-end architecture and attention mechanism, we will propose novel strategies for carbon neutrality in physical education and experimentally verify their effectiveness. This comprehensive research approach not only fills the academic gap in carbon neutrality-related disciplines within the field of physical education but also offers important theoretical and methodological support for the sustainable development and realization of carbon neutrality objectives in physical education. Within the context of digital technology-driven energy transition, our focus lies on various aspects of digital transformation, providing valuable references and recommendations for achieving green and sustainable development. By integrating deep learning methods with carbon neutrality strategies in physical education, this research opens new avenues for the application of digital technology in this domain and offers feasible solutions for the dual realization of healthy development in physical education and carbon neutrality objectives.
In this study, we employ an experimental research design aimed at exploring a carbon-neutral strategy approach in attention-based physical education instruction under an end-to-end architecture. We combine deep learning techniques to construct a model for carbon-neutral strategy recommendation, and verify its effect and performance through experiments. In this section, we will introduce the methods we used in the construction process in detail. The overall algorithm flow of this paper is shown in Figure 1 below:
Figure 1. Overall Algorithm Flowchart. Firstly, the raw data is processed through the input module. Subsequently, an end-to-end architecture is employed to construct a fully connected model. Following this, an attention mechanism is introduced, encompassing channel and spatial attention to enhance the model’s focus on features related to carbon emissions. Finally, in-depth feature learning is conducted through the CNN module, integrating operations such as Relu, Fusion, BatchNorm, forming a comprehensive algorithmic structure.
This comprehensive flowchart illustrates the roles of the proposed end-to-end framework, attention mechanism, and CNN module in the assessment of carbon neutrality in sports education. Specifically, it encompasses three main steps: the end-to-end architecture, attention mechanism, and CNN module, providing a holistic approach to the comprehensive evaluation of carbon emissions in sports education. The entire process embodies the core principles of deep learning and feature extraction, offering reliable technological support for achieving carbon neutrality goals. Firstly, starting from the input module, raw data undergoes processing. The MaxPool operation retains crucial information through maximum pooling, while the Relu activation function introduces non-linear mapping, effectively increasing the model’s sensitivity to carbon emission features. The BatchNorm operation enhances training stability through batch normalization, laying the foundation for subsequent processing. The Conv operation is responsible for extracting fundamental features related to carbon emissions from the raw data. Secondly, we enter the end-to-end architecture, which integrates and evaluates input features comprehensively. This architecture autonomously learns and extracts features related to carbon emissions through a fully connected model, without relying on predefined rules, providing the model with high adaptability and generalization capabilities. This step forms the basis for an overall understanding of carbon neutrality goals. Next, the attention mechanism is introduced, including the Channel Attention Module (CAM) and Spatial Attention Module (SAM). CAM highlights critical channels through channel attention, while SAM emphasizes important image regions through spatial attention. Their organic combination forms CBAM, significantly enhancing the model’s ability to grasp key features of carbon neutrality. This step allows the model to focus more precisely when dealing with carbon emission-related information. Finally, the CNN module delves into exploring and abstracting input features in a more advanced and abstract manner to understand key features of carbon emissions. Operations such as Relu, Fusion, and BatchNorm further strengthen the model’s expressive power and feature integration, creating a comprehensive and robust algorithmic framework. This flowchart provides a deep learning approach to carbon neutrality assessment in sports education that is both comprehensive and powerful, effectively addressing the complexity and diversity in carbon emission evaluation.
We adopted an end-to-end architecture as the base model for this study. The architecture allows for the integration and optimization of all aspects of the teaching process in order to achieve a comprehensive carbon-neutral goal. The end-to-end architecture provides a unified optimization platform, so that various components involved in teaching activities can cooperate with each other (Chen et al., 2019), and comprehensively consider the trade-off of carbon emission reduction and teaching effect. The end-to-end model framework is shown in Figure 2 below:
The end-to-end framework includes three main components: energy supply end, energy consumption end and anthropogenic carbon sequestration end.
• Energy supply side: refers to the source of energy required in the teaching process, including renewable energy (such as solar energy, wind energy, etc.) and non-renewable energy (such as coal, oil, etc.). The optimization goal of the energy supply side is to increase the proportion of renewable energy and reduce the proportion of non-renewable energy, thereby reducing carbon emissions.
• Energy consumption: refers to the energy consumed in each link of the teaching process, including classroom equipment (such as lighting, air conditioning, etc.), teaching equipment (such as projectors, computers, etc.), teaching activities (such as sports, experiments, etc.). The optimization goal of the energy consumption end is to reduce the energy consumption of each link and improve the teaching effect index of each link, so as to achieve the balance between the reduction of carbon emissions and the improvement of teaching effect.
• Anthropogenic carbon sequestration: refers to the ability to increase carbon sinks through artificial measures during the teaching process, including afforestation, soil management, and ecological restoration. The optimization goal of artificial carbon sequestration is to increase the amount of carbon sequestered in each link and offset the carbon emissions of each link, so as to achieve carbon neutrality.
To formalize the optimization problem in an end-to-end framework, we first give some notations and definitions. Assuming that the teaching process involves N links, each link i has an energy consumption Ei (unit is kilogram standard coal), a carbon emission Ci (unit is kilogram carbon dioxide), and a teaching effect index Qi (unit is fraction), and a non-carbon energy substitution ratio Ri (in percent). Then the optimization objective of the end-to-end framework can be expressed as Eq. 1:
Among them, λ is a positive parameter, indicating the impact weight of carbon emission reduction on teaching effect. At the same time, the following constraints need to be met Eq. 2:
Among them, Emax is the upper limit of energy consumption in the teaching process, and Rmin is the lower limit of the replacement ratio of non-carbon energy.
In this way, by solving this optimization problem, the optimal energy consumption, carbon emissions, teaching effect indicators and non-carbon energy substitution ratio of each link can be obtained, so as to achieve the optimization goal of the end-to-end framework. We use a CNN model to approximate the solution of this optimization problem, and the specific model structure and training method will be introduced in the next subsection.
To approximate the solution of the optimization problem in an end-to-end framework, we use a CNN as the underlying model of the end-to-end architecture. CNN is a deep learning model that can efficiently process high-dimensional data such as images, speech, and text. The main feature of CNN is to use convolutional layers, pooling layers and fully connected layers to build complex feature extraction and classifiers. The advantage of CNN is that it can reduce the number of parameters, improve computational efficiency, enhance generalization ability, and adapt to data of different scales and deformations. We designed a simple CNN model to analyze the carbon emissions in the teaching process, and provide corresponding carbon neutral suggestions for different links. We represent each link in the teaching process as an H× W× D tensor (such as image, speech, text, etc.), where H is height, W is width, and D is depth (number of channels) (Li et al., 2024). We use these tensors as the input of the CNN model, and after several convolutional layers, pooling layers, and fully connected layers, we get an output vector, which represents the optimal energy consumption, carbon emissions, and teaching effect indicators of each link. and non-carbon energy substitution ratio. As shown in Figure 3 below:
In the following subsections, we will introduce the various components of the CNN model and their operation in detail. The convolutional layer is the core component of the CNN model, which can extract local features in the input data and reduce the number of parameters by sharing weights. A convolutional layer can be expressed as Eq. 3:
Where Y is the output image, X is the input image, W is the convolution kernel (filter), b is the bias term,⋅ is the convolution operator, and f is the activation function (such as ReLU, sigmoid, etc.). The convolution operator can be defined as Eq. 4:
where ⊛ is a two-dimensional cross-correlation operator defined as Eq. 5:
where kH and kW are the height and width of the convolution kernel, respectively. The process of convolution operation can be understood as sliding the convolution kernel on the input image and calculating the cross-correlation value of each position to obtain the output image. The result of the convolution operation is affected by parameters such as the size of the convolution kernel, step size, padding, etc., and these parameters can be adjusted according to different tasks. The pooling layer is another important part of the CNN model, which can down-sample the input data, thereby reducing the amount of data, improving computational efficiency, and enhancing the robustness of the model. The pooling layer can be expressed as Eq. 6:
Where Z is the output image, Y is the input image, and g is the pooling function (such as maximum pooling, average pooling, etc.). The pooling function can be defined as Eq. 7:
Or Eq. 8:
where P is a pooling window (such as 2 × 2, 3 × 3, etc.). The process of pooling operation can be understood as sliding the pooling window on the input image and calculating the maximum value or average value of each position to obtain the output image. The result of the pooling operation is affected by parameters such as the size of the pooling window and the step size, which can be adjusted according to different tasks.
The fully connected layer is the last component of the CNN model, which can integrate and classify the previously extracted features to obtain the final output vector. A fully connected layer can be expressed as Eq. 9:
Where O is the output vector, Z is the input vector (usually obtained after flattening the output image of the previous layer), V is the weight matrix, c is the bias vector, ⋅ is the matrix multiplication operator, and h is the activation function (Such as softmax, sigmoid, etc.). The operation process of the fully connected layer can be understood as matrix multiplication of the input vector and the weight matrix, adding the bias vector, and then obtaining the output vector through the activation function. The results of fully connected layers are affected by the way the weight matrix and bias vector are initialized and updated, which can be tuned for different tasks.
To improve the effectiveness and interpretability of the carbon-neutral strategy approach, we introduce an attention mechanism to allow the model to automatically focus on features or regions that are closely related to the carbon-neutral goal (Luo et al., 2019). The attention mechanism is a way to let the model automatically focus on features or regions that are closely related to the target task (Niu et al., 2021). The attention mechanism can improve the effect and interpretability of the model, and is applicable to various types of data and tasks. The main idea of the attention mechanism is to use a weight vector to represent the importance of each element in the input data, and then obtain a context vector by weighted summation as the input or output of the model. As shown in Figure 4 below:
We use the feature vector of each link output by the CNN model as the input of the attention mechanism, and after a scoring function, a softmax function and a weighted sum function, a context vector is obtained as the maximum value of each link output by the CNN model. Optimize energy consumption, carbon emissions, teaching effect indicators and non-carbon energy substitution ratio. In the following, we describe in detail the various components of the attention mechanism and their operations.
The scoring function is the core component of the attention mechanism, which can calculate the contribution of each element in the input data to the target task and generate a scoring vector. The scoring function can be selected or designed according to different types of attention mechanisms. The common scoring functions are as follows:
• Dot product: The scoring function is the dot product operation between the input vector and the query vector;
• Bilinear: The scoring function is the dot product operation between the input vector and the query vector after a weight matrix transformation;
• Multi-layer perceptron (multi-layer perceptron): The scoring function is the output value obtained after the input vector and query vector are transformed by a multi-layer perceptron.
The scoring function can be expressed as Eq. 10:
where e is the scoring vector,X is the input data (such as the feature vector of each link output by the CNN model), q is the query vector (such as the overall feature vector output by the CNN model), and f is the scoring function (such as dot product, double-line properties, multi-layer perceptron, etc.). Scoring functions can be selected or designed according to different data and tasks. The softmax function is another important part of the attention mechanism, which can convert the score vector into a weight vector, which represents the importance of each element in the input data. The softmax function can be defined as Eq. 11:
where α is the weight vector, e is the scoring vector, and nis the number of input elements. The softmax function can guarantee that each element of the weight vector is between 0 and 1, and the sum of all elements is 1. The weighted sum function is the last component of the attention mechanism, which can weight and sum the input data according to the weight vector, so as to obtain a context vector as the input or output of the model. The weighted sum function can be defined as Eq. 12:
Where C is the context vector, α is the weight vector, and X is the input data (such as the feature vector of each link output by the CNN model). The weighted sum function can focus on features or regions in the input data that are closely related to the target task.
In order to show the implementation process of the algorithm in this paper more clearly, we provide the following pseudocode Algorithm 1, which includes the input parameters of the algorithm, variable definitions, flow control statements, and output results.
Algorithm 1.ETE-AttCNN Training.
Input: Datasets: “Human 3.6M″, “UCI HAR”, “WISDM”, “UCF101”
Output: Trained ETE-AttCNN model
Initialize ETE-AttCNN model parameters;
Randomly initialize CNN and attention mechanism parameters;
Split datasets into training, validation, and testing sets;
while stopping criterion is not met do
Sample a mini-batch of training data;
Forward pass the mini-batch through the CNN;
Apply attention mechanism to the CNN output;
Compute the ARA(%), AOR(%), and ARR(%) metrics;
Compute the loss function using the metrics;
Backpropagate the gradients through the network;
Update the CNN and attention mechanism parameters;
end
return Trained ETE-AttCNN model
The experimental process of this paper is shown in Figure 5 below:
• Hardware environment
This experiment uses a high-performance computing server as the hardware environment, which is equipped with Intel Xeon E5-2690 v4 @ 2.60 GHz CPU and 512GB RAM, and is equipped with 8 Nvidia Tesla P100 16 GB GPUs. Such a hardware configuration provides powerful computing and storage capabilities, and is especially suitable for training and inference of deep learning tasks, helping to speed up the model training process and enabling experiments to run efficiently and converge.
• Software environment
In this study, we used Python and PyTorch to implement the carbon-neutral strategy method in the attention-based physical education teaching under the end-to-end architecture. As the main deep learning framework, PyTorch provides us with flexible model building and training tools, enabling us to effectively develop and optimize our carbon neutral strategy model. In the experiment, we made full use of PyTorch’s powerful computing power and automatic differentiation function to speed up the model training process, so that our model can converge faster and achieve better results.
• Human 3.6M dataset:
It is a large-scale motion capture data set released by the Human Motion Analysis Group (PERCEPTION) of the French National Institute of Computer and Automation (INRIA) in 2014. It contains 3.6 million human poses and corresponding images. Capture system and 4 high-resolution progressive scan cameras capture. It covered 15 subjects (11 males and 4 females) performing 17 different activities (such as walking, sitting, talking on the phone, etc.) in an indoor environment. It aims to provide a standardized evaluation platform for tasks such as human pose estimation, human motion analysis, and human action recognition. It also provides some additional data, such as pixel-level labels of 24 body parts, temporal extent data, 3D laser-scanned subject models, accurate background segmentation, person bounding boxes, etc.
• UCI HAR dataset:
The dataset was released in 2012 by the Intelligent Signal and Communication Systems Research Center (SISCOM) at the University of Barcelona, Spain, to provide an open dataset for human activity recognition based on smartphone sensors. It is a human activity recognition dataset collected using smartphone sensors. It contains 10,299 samples, each consisting of 561 features, representing three-axis linear acceleration and three-axis angular velocity collected by accelerometers and gyroscopes. It involved 30 volunteers (19 males and 11 females) performing 6 different activities (such as standing, sitting, lying down, walking, going up and down stairs, etc.) within a fixed space. It uses two sensors: an accelerometer and a gyroscope, which collect data at 50 Hz and 200 Hz, respectively. Accelerometers measure the acceleration due to gravity and body motion, and gyroscopes measure the angular velocity of the body’s rotation around three axes. It also provides two types of data: raw data and preprocessed data. The raw data contains the original signals collected by each sensor on three axes, and the preprocessed data contains the feature vectors obtained after noise filtering, resampling, segmentation and other operations.
• WISDM dataset:
It was released by the Wireless Sensor Data Mining Laboratory (WISDM Lab) of the University of Wisconsin in the United States in 2011. It is a human activity recognition dataset collected by smartphone sensors. It contains 1,098,203 samples, each of which consists of 6 features Composition, representing the three-axis linear acceleration collected by the accelerometer. It involved 36 volunteers (18 males and 18 females) performing 6 different activities in a natural setting. It uses one sensor: an accelerometer, which collects data at a frequency of 20 Hz. Accelerometers measure the acceleration due to gravity and the acceleration caused by body motion. It provides raw data and preprocessed data. The raw data contains the original signals collected by each sensor on three axes, and the preprocessed data contains the feature vectors obtained after noise filtering, resampling, segmentation and other operations.
• UCF101 dataset:
UCF101 is a human activity recognition dataset released by the Computer Vision Research Center of the University of Central Florida in 2012. The dataset contains 13,320 video clips, each clip consists of 25–300 frames, covering 101 different activities, including five types of activities, including human-object interaction, pure body movement, human-human interaction, playing musical instruments and sports. The dataset utilizes real and diverse videos uploaded by users on YouTube, presents realism and challenges, and contains various difficult factors, such as camera motion, changes in object appearance and pose, viewpoint changes, etc. In addition, the dataset also provides additional information such as label, start and end time, resolution and frame rate of each video clip, making it a realistic, diverse and challenging resource for human activity recognition. The UCF101 dataset has become an important benchmark for studying human activity recognition in the field of computer vision, and plays an important role in the performance evaluation and exploration of algorithms in this field.
When it comes to evaluating the effectiveness of carbon-neutral strategies in physical education instruction, there are several key evaluation metrics to consider. These metrics help measure Carbon Emissions (CE), Action Recognition Accuracy (ARA), Action Recognition Recall (ARR) and Action Optimization Rate (AOR).
• Carbon emissions
Carbon emissions refer to the total amount of greenhouse gases such as carbon dioxide produced during physical education teaching, usually in kilograms (kg). Assessing the carbon emissions of physical education teaching can help to understand the impact of teaching activities on the environment. With the proposed carbon neutral goal, we hope to reduce the adverse impact of the teaching process on the climate through the reduction of carbon emissions. The formula for carbon emissions is Eq. 13:
Among them, CE represents the total carbon emissions, and CEi represents the carbon emissions generated in the ith link in the teaching process. In our research, we need to subdivide each link in the teaching process, such as equipment use, student participation, etc., calculate the corresponding carbon emissions, and then sum them up to get the total carbon emissions.
• Action recognition accuracy
Action recognition accuracy measures the model’s ability to correctly classify actions in a physical education dataset. It calculates the percentage of the number of samples (true and true negatives) that are correctly classified among all samples. For the action recognition model using the deep learning method, ARA evaluated how well it correctly identified the sports actions of the students. A high ARA means that the model can accurately identify the student’s action behavior in physical education teaching. The formula for action recognition accuracy is Eq. 14:
Among them, TP (True Positives) indicates the number of positive examples that are correctly predicted, TN (True Negatives) indicates the number of negative examples that are correctly predicted, FP (False Positives) indicates the number of positive examples that are incorrectly predicted, and FN(False Negatives) indicates the number of incorrectly predicted Number of negative examples.
• Action recognition recall rate
Action recognition recall measures the model’s ability to correctly detect all positive examples. It calculates the proportion of correctly predicted positives to all true positives. ARR evaluates the proportion of all real actions that the model successfully captures. A high ARR indicates that the model can effectively identify students’ sports actions and capture all real actions to the greatest extent. The following is the formula for action recognition recall Eq. 15:
Among them, TP (True Positives) indicates the number of positive examples that are correctly predicted, and FN (False Negatives) indicates the number of positive examples that were not correctly predicted.
• Action optimization rate
Action Optimization Rate (AOR) is an indicator used to evaluate the carbon-neutral effect achieved by optimizing the teaching process after adopting the carbon-neutral strategy method based on the attention mechanism under the end-to-end architecture in physical education teaching. It measures the degree to which the model reduces carbon emissions in the teaching process, as well as the model’s impact on teaching effectiveness after implementing carbon-neutral strategies. If AOR>0, it means that the carbon emission in the physical education process has been successfully reduced, that is, the goal of carbon neutrality has been achieved. Conversely, if AOR<0, it may mean that carbon emissions have increased in some cases. Therefore, the action optimization rate (AOR) is an important basis for evaluating your model performance and guiding improvement. The formula for calculating the Action Optimization Rate (AOR) is Eq. 16:
Among them, CEbefore represents the carbon emission before adopting the carbon neutral strategy, and CEafter represents the carbon emission after adopting the carbon neutral strategy.
In the previous sections, we provided detailed explanations of the experimental environment, datasets used, and evaluation metrics for the current experiment. Now, we will proceed to the experimental comparison and analysis section, where we will compare and analyze the results obtained from different datasets to comprehensively evaluate the performance of the attention-based end-to-end architecture for carbon neutrality strategies in physical education.
Firstly, we conducted experiments using four commonly used datasets: Human 3.6M, UCI HAR, WISDM, and UCF101. These datasets cover various types of physical activities and action recognition tasks, providing us with diverse and challenging experimental scenarios; Secondly, we selected a set of evaluation metrics to comprehensively assess the method’s performance. These metrics include Carbon Emission (CE), Action Recognition Accuracy (ARA), Action Recognition Recall (ARR), and Action Optimization Rate (AOR). These metrics will help us evaluate the effectiveness of carbon neutrality strategies and the model’s performance on action recognition tasks.
In this section, we will compare the experimental results from different datasets and present corresponding tables and visualizations to demonstrate the experimental comparisons and analyses. The tables will focus on comparing the differences in carbon emission and metric performance among different methods. Additionally, we will explore the training time, inference time, and parameter volume for different module combinations to assess the method’s efficiency and scalability. Through these experimental comparisons and analyses, we aim to gain a comprehensive understanding of the strengths and limitations of the attention-based end-to-end architecture for carbon neutrality strategies in physical education. This will guide us in further improving the method, optimizing the model, and applying it in practical physical education settings.
Next, let us delve into the experimental results and gradually reveal the advantages, disadvantages, and potential of the method.
First, Table 1 above shows the performance of different methods on the Human 3.6M and UCI HAR datasets. In order to comprehensively evaluate the carbon neutrality and recognition effect of different methods, the research uses multiple indicators such as carbon emissions, action recognition accuracy, recall rate, and optimization rate. It can be seen from the table that on the Human 3.6M dataset, the carbon emission of our proposed method is the lowest, only 19.41 kg, which is more than 60% less than other methods. At the same time, our action recognition accuracy, recall rate and optimization The rate is also more than 93%, and the best results have been obtained in all indicators. This shows that our method successfully achieves the goal of carbon neutrality while maintaining the best model performance. Results on the UCI HAR dataset also confirm the superior performance of our method. Combining the results of the two datasets, it can be seen that our end-to-end attention mechanism method achieves the best balance between excellent carbon neutrality and recognition effect. At the same time, we also compared and visualized the results in Table 1, as shown in Figure 6 below:
Table 1. Comparison of different methods based on CE, ARA, ARR, and AOR indicators on Human 3.6M and UCI HAR datasets.
Figure 6. Visualization of CE, ARA, ARR, and AOR indicators based on Human 3.6M and UCI HAR datasets under different methods.
Table 2 above shows the performance of each method on the two data sets WISDM and UCF101. In order to further verify the effectiveness of the end-to-end attention mechanism on different datasets, the research is extended to the WISDM human activity dataset and the UCF101 action recognition dataset. It can be seen from the table that on the WISDM dataset, the carbon emission of our method is the lowest, and the accuracy rate, recall rate and optimization rate of action recognition are also the highest, reaching 94.55%, 96.61% and 95.30% respectively, which is obviously superior. In the methods of Mustafa et al., Kiehle et al. and van der Ven et al. Our method achieves 94.55% recognition accuracy, more than 10% higher than all compared methods. On the complex UCF101 dataset, the recognition accuracy of our method reaches 94.47%, becoming the optimal data. This again verifies the superior performance of the end-to-end attention mechanism. The results in Table 2 further confirm that on various datasets, our method can greatly reduce carbon emissions while maintaining a strong model effect. We also compared and visualized the results in Table 2, as shown in Figure 7 below:
Table 2. Comparison of different methods based on CE, ARA, ARR, and AOR indicators on WISDM and UCF101 datasets.
Figure 7. Visualization of CE, ARA, ARR, AOR indicators based on WISDM and UCI 101 datasets under different methods.
In Table 3, we list the training time and inference time of different methods on four datasets. It can be seen from the tabular data that the method of Mustafa et al. has the longest overall training time and inference time on each data set. For example, in the Human 3.6M dataset, the training time of Mustafa et al. was 56.84 s and the inference time was 176.82 milliseconds, while the training time of our method was only 41.73 s and the inference time was the shortest, only 104.71 milliseconds on the other three datasets, the method of Mustafa et al. also has the longest training time and inference time. In contrast, our method achieves substantial reductions in training time and inference time on all datasets. For example, on the UCF101 dataset, the training time of Mustafa et al.’s method is 65.98 s and the inference time is 192.66 milliseconds, while our method only needs 42.59 s and 131.02 milliseconds respectively. In addition, it can be seen from the parameter comparison that the parameter quantity of our method is smaller than other methods. This shows that our model is more lightweight and efficient than other methods. We compared and visualized the results in Table 3, as shown in Figure 8 below:
Table 3. Training Time, inference Time, and Parameters indicators under different methods based on four datasets.
Figure 8. Visualization of Training Time, inference Time, and Parameters indicators under different methods based on four datasets.
In Table 4, we comprehensively compare the action recognition accuracy and carbon emissions of different modules on the four datasets. For the Human 3.6M dataset, the recognition accuracy of the baseline method is 72.98%, and the carbon emission is 57.54 kg. After adding the attention mechanism module, the recognition accuracy is increased to 82.06%, and the carbon emission is reduced to 45.73 kg. Then add the end-to-end architecture module, the recognition accuracy rate reaches 86.25%, and the carbon emission is further reduced to 39.81 kg. Finally, the attention mechanism and the end-to-end architecture are connected in series, and the recognition accuracy is greatly increased to 93.06%, while the carbon emission is also significantly reduced to 19.28 kg. On the UCI HAR dataset, the accuracy and carbon emissions of the baseline method are 72.58% and 53.13 kg, respectively. After adding the attention mechanism and end-to-end architecture, the accuracy rate increased to 77.85% and 85.83%, respectively, and the carbon emissions also decreased significantly. After the two modules are finally connected in series, the accuracy rate reaches 95.61%, which is 23 percentage points higher than the baseline, and the carbon emission is reduced to 17.35 kg. On the WISDM and UCF101 datasets, it is also observed that the addition of modules makes the accuracy rate continuously improve, and the carbon emissions also decrease significantly. Combining the results of the four data sets, it can be seen that the introduction of the attention mechanism and the end-to-end architecture not only improves the accuracy of the model, but also reduces carbon emissions. This comprehensively verifies the effects of the two modules, and illustrates their important role in carbon reduction and efficiency improvement. Finally, I compared and visualized the results in Table 4, as shown in Figure 9 below:
Table 5 presents the comparison of action recognition recall and optimization rates of different methods on the four datasets. On the Human 3.6M dataset, the recall rate of the baseline method is 75.94%, and the optimization rate is 77.69%. After adding the attention mechanism, the recall rate increases to 80.96%, and the optimization rate reaches 84.62%. Adding the end-to-end architecture leads to a recall rate of 88.51% and an optimization rate of 85.76%. Finally, the two modules are connected in series, the recall rate reaches 95.27%, and the optimization rate reaches 93.43%. Adding modules consistently improves recall and refinement rates are also observed on the other three datasets. For example, on the UCI HAR dataset, the recall rate and optimization rate of the baseline method are 78.14% and 79.16%, respectively, while these two indicators of the full model reach 95.24% and 93.58%, significantly exceeding the baseline. On all datasets, our full models achieve significantly higher recall and optimization rates. Meanwhile, on the WISDM dataset, compared to the baseline recall rate of 74.28% and optimization rate of 72.65%, our full model achieves recall and optimization rates of 96.61% and 95.30%, respectively. In summary, the introduction of the attention mechanism and the end-to-end architecture has greatly improved the recall rate and optimization rate on the four datasets, which once again verified the effects of the two modules and provided a basis for designing more efficient carbon-neutral artificial intelligence models. reference. I compared and visualized the results in Table 5, as shown in Figure 10 below:
Through the comprehensive comparison and analysis of these five tables, we can conclude that the end-to-end architecture based on the attention mechanism has shown excellent performance in the carbon-neutral strategy method in physical education teaching, which can not only effectively reduce carbon emissions At the same time, it has achieved excellent accuracy and recall on action recognition tasks. In addition, our method also shows high performance in terms of running efficiency. Based on these experimental results, we are confident in the application of this method in actual physical education teaching scenarios, and look forward to further promoting and optimizing it to make greater contributions to the carbon-neutral goal of physical education teaching.
The aim of this research is to explore an end-to-end architecture for implementing carbon neutrality strategies in physical education and introduce attention mechanisms to enhance effectiveness and interpretability. It focuses on the core of digital technology-driven energy transition while also addressing the sustainable development and achievement of carbon neutrality goals in the field of physical education. In this section, we will review the research findings, evaluate the effectiveness of carbon neutrality strategies, analyze the strengths and limitations of the methods, discuss the contribution of attention mechanisms to carbon neutrality effects, and propose areas for improvement and future research.
In our experiments, we trained a model based on an end-to-end architecture and attention mechanism, and tested it on a dataset simulating carbon neutrality scenarios in sports teaching. The experimental results demonstrate outstanding performance of our model in terms of Action Recognition Accuracy (ARA) and Action Recognition Recall (ARR) across the four datasets. In comparison to various alternative methods, our model consistently achieved optimal metrics. This indicates that our model exhibits high accuracy and recall in action recognition, showcasing its strong performance in addressing the task of action identification in sports teaching. Furthermore, in terms of Action Optimization Rate (AOR), our model showed significant improvement, reaching 93% or higher. This suggests the effectiveness of our carbon neutrality strategy in reducing carbon emissions in sports teaching, thereby optimizing the carbon footprint associated with sports education. By adopting an end-to-end architecture, we successfully integrated various components of the teaching process. The incorporation of an attention mechanism allowed for the automatic identification of features and regions closely related to carbon neutrality objectives. This further enhanced the effectiveness and interpretability of our carbon neutrality strategy. These findings underscore the success of our model in achieving the dual goals of accurate action recognition and efficient carbon emission reduction within the sports teaching context. The integration of end-to-end architecture and attention mechanism showcases the potential for comprehensive and effective carbon neutrality strategies in sports education.
Certainly, we also acknowledge several limitations and shortcomings in our study. Firstly, in the context of actual education and environmental settings, it is crucial to scrutinize the physical consequences of the carbon neutrality strategies proposed by the model in real sports teaching environments. This entails examining the tangible impacts of the proposed emission reduction measures on students and educational institutions, such as whether reducing carbon emissions might lead to higher economic costs or increased student satisfaction. Analyzing these physical consequences will provide a better understanding of the feasibility and implications of the proposed carbon neutrality strategies in practical applications. Secondly, the scale of the dataset we constructed is relatively small, potentially not fully capturing the diversity and complexity of carbon emissions in sports teaching. Furthermore, we solely considered carbon dioxide as the primary greenhouse gas for calculations, neglecting the influence of other greenhouse gases such as methane and nitrous oxide on carbon emissions. Addressing these issues in future work involves expanding the dataset size and introducing more real-world scenario data to enhance the model’s generalizability. Additionally, considering various greenhouse gas types and utilizing more accurate emission factors are essential to improve the precision of the model. Lastly, our focus was primarily on carbon emission as a metric, without considering other indicators related to sports teaching. Therefore, in future endeavors, it is imperative to broaden the dataset size, introduce more diverse real-world scenario data, enhance the model’s generalization capabilities, and consider multiple types of greenhouse gases with accurate emission factor calculations. Moreover, a comprehensive approach that integrates teaching effectiveness, student satisfaction, economic costs, and other relevant metrics is necessary. Establishing a multi-objective optimization model will contribute to achieving a better balance between carbon neutrality strategies and the sports teaching process.
In the face of increasingly severe climate change, carbon neutrality has become a global consensus and an urgent necessity. As an important social activity, physical education teaching also needs to actively respond to the national carbon neutrality goals and explore suitable pathways for carbon reduction. Education practitioners, policymakers, and researchers should also consider and adopt carbon neutrality strategies. This study has made significant progress in researching carbon neutrality strategies in physical education. Through the application of an end-to-end framework, attention mechanism, and convolutional neural network, we have achieved a comprehensive analysis of carbon emissions and precise recommendations for carbon neutrality strategies. This research provides new insights and methods for achieving carbon neutrality goals in physical education, holding significant implications for steering physical education towards a green and sustainable direction. In future studies, we will continue to refine and optimize this approach, strengthen its integration with actual physical education scenarios, and consistently enhance the practicality and effectiveness of carbon neutrality strategies in physical education. Through ongoing efforts, we are confident in developing carbon neutrality strategies in physical education into a crucial technology beneficial for society’s sustainable development.
The original contributions presented in the study are included in the article/Supplementary material, further inquiries can be directed to the corresponding author.
HH: Conceptualization, Data curation, Formal Analysis, Funding acquisition, Investigation, Methodology, Project administration, Resources, Software, Supervision, Writing–original draft, Writing–review and editing. TY: Formal Analysis, Investigation, Methodology, Visualization, Writing–original draft. FC: Project administration, Software, Supervision, Visualization, Writing–review and editing.
The author(s) declare that no financial support was received for the research, authorship, and/or publication of this article. 1. Guangdong Higher Education Association “14th Five-Year Plan” 2022 Higher Education Research Project: Research on CIPP Evaluation Model of Public Physical Education Curriculum Ideology and Politics in Higher Education Institutions in Guangdong-Hong Kong-Macao Greater Bay Area (22GQN02). 2. “2022 University level” “Innovation Strong School Project” (research): Innovation research team-Innovation Research Team of Governance Model and Policy Simulation of Individual. Sports Associations (GKY-2022CQTD-13).
The authors declare that the research was conducted in the absence of any commercial or financial relationships that could be construed as a potential conflict of interest.
All claims expressed in this article are solely those of the authors and do not necessarily represent those of their affiliated organizations, or those of the publisher, the editors and the reviewers. Any product that may be evaluated in this article, or claim that may be made by its manufacturer, is not guaranteed or endorsed by the publisher.
Ahn, D., Kim, S., Hong, H., and Ko, B. C. (2023). “Star-transformer: a spatio-temporal cross attention transformer for human action recognition,” in Proceedings of the IEEE/CVF Winter Conference on Applications of Computer Vision, 3330–3339. doi:10.1109/wacv56688.2023.00333
Atwoli, L., Baqui, A. H., Benfield, T., Bosurgi, R., Godlee, F., Hancocks, S., et al. (2021). Call for emergency action to limit global temperature increases, restore biodiversity, and protect health: wealthy nations must do much more, much faster. Nutr. Rev. 79, 1183–1185. doi:10.1093/nutrit/nuab067
Baena-Morales, S., Merma-Molina, G., and Ferriz-Valero, A. (2023). Integrating education for sustainable development in physical education: fostering critical and systemic thinking. Int. J. Sustain. High. Educ. 24, 1915–1931. doi:10.1108/ijshe-10-2022-0343
Balaha, H. M., and Hassan, A. E.-S. (2023). Comprehensive machine and deep learning analysis of sensor-based human activity recognition. Neural Comput. Appl. 35, 12793–12831. doi:10.1007/s00521-023-08374-7
Chapman, A., Ertekin, E., Kubota, M., Nagao, A., Bertsch, K., Macadre, A., et al. (2022). Achieving a carbon neutral future through advanced functional materials and technologies. Bull. Chem. Soc. Jpn. 95, 73–103. doi:10.1246/bcsj.20210323
Chen, F., Luo, Z., Xu, Y., and Ke, D. (2019). Complementary fusion of multi-features and multi-modalities in sentiment analysis. arXiv preprint arXiv:1904.08138
Chen, J. (2022). The reform of school education and teaching under the “double reduction” policy. Sci. Soc. Res. 4, 42–45. doi:10.26689/ssr.v4i2.3624
Dai, W., Jiang, Y., Mou, C., and Zhang, C. (2023a). “An integrative paradigm for enhanced stroke prediction: synergizing xgboost and xdeepfm algorithms,” in Proceedings of the 2023 6th International Conference on Big Data Technologies. 28–32. doi:10.1145/3627377.3627382
Dai, W., Mou, C., Wu, J., and Ye, X. (2023b). “Diabetic retinopathy detection with enhanced vision transformers: the twins-pcpvt solution,” in 2023 IEEE 3rd International Conference on Electronic Technology, Communication and Information (ICETCI) (IEEE), 403–407.
Dällenbach, N. (2020). Low-carbon travel mode choices: the role of time perceptions and familiarity. Transp. Res. Part D Transp. Environ. 86, 102378. doi:10.1016/j.trd.2020.102378
Elnour, M., Fadli, F., Himeur, Y., Petri, I., Rezgui, Y., Meskin, N., et al. (2022a). Performance and energy optimization of building automation and management systems: towards smart sustainable carbon-neutral sports facilities. Renew. Sustain. Energy Rev. 162, 112401. doi:10.1016/j.rser.2022.112401
Elnour, M., Himeur, Y., Fadli, F., Mohammedsherif, H., Meskin, N., Ahmad, A. M., et al. (2022b). Neural network-based model predictive control system for optimizing building automation and management systems of sports facilities. Appl. Energy 318, 119153. doi:10.1016/j.apenergy.2022.119153
Ezeonu, L., Tang, Z., Qi, Y., Huo, F., Zheng, Y., Koel, B. E., et al. (2023). Adsorption, surface reactions and hydrodeoxygenation of acetic acid on platinum and nickel catalysts. J. Catal. 418, 190–202. doi:10.1016/j.jcat.2023.01.013
Fu, H. (2023). A comprehensive review of nature-based solutions: current status and future research. AIMS Environ. Sci. 10, 677–690. doi:10.3934/environsci.2023037
Fuchs, P. G., Honorato Filho, M., da Silva, L. A., Dutra, A. R. A., and Guerra, J. B. S. O. d. A. (2023). The carbon footprint at quality and environmental university consortium–qualenv. Int. J. Sustain. High. Educ. 25, 43–61. doi:10.1108/ijshe-11-2022-0376
Goyal, G., Di Pietro, F., Carissimi, N., Glover, A., and Bartolozzi, C. (2023). “Moveenet: online high-frequency human pose estimation with an event camera,” in Proceedings of the IEEE/CVF Conference on Computer Vision and Pattern Recognition, 4023–4032. doi:10.1109/cvprw59228.2023.00420
He, S., Wang, J., Yang, F., Chang, T.-L., Tang, Z., Liu, K., et al. (2023). Bacterial detection and differentiation of staphylococcus aureus and escherichia coli utilizing long-period fiber gratings functionalized with nanoporous coated structures. Coatings 13, 778. doi:10.3390/coatings13040778
Himeur, Y., Elnour, M., Fadli, F., Meskin, N., Petri, I., Rezgui, Y., et al. (2022). Next-generation energy systems for sustainable smart cities: roles of transfer learning. Sustain. Cities Soc. 85, 104059. doi:10.1016/j.scs.2022.104059
Himeur, Y., Elnour, M., Fadli, F., Meskin, N., Petri, I., Rezgui, Y., et al. (2023). Ai-big data analytics for building automation and management systems: a survey, actual challenges and future perspectives. Artif. Intell. Rev. 56, 4929–5021. doi:10.1007/s10462-022-10286-2
Hou, Z.-M., Xiong, Y., Luo, J.-S., Fang, Y.-L., Haris, M., Chen, Q.-J., et al. (2023). International experience of carbon neutrality and prospects of key technologies: lessons for China. Petroleum Sci. 20, 893–909. doi:10.1016/j.petsci.2023.02.018
Huang, H., Wu, X., and Cheng, X. (2021). The prediction of carbon emission information in yangtze river economic zone by deep learning. Land 10, 1380. doi:10.3390/land10121380
Kiehle, J. (2021). A carbon neutral campus: tools of carbon footprint and handprint assessment. Master’s thesis. J. Kiehle.
Köhl, M., Ehrhart, H.-P., Knauf, M., and Neupane, P. R. (2020). A viable indicator approach for assessing sustainable forest management in terms of carbon emissions and removals. Ecol. Indic. 111, 106057. doi:10.1016/j.ecolind.2019.106057
Kulkarni, S., Deshmukh, S., Fernandes, F., Patil, A., and Jabade, V. (2023). Poseanalyser: a survey on human pose estimation. SN Comput. Sci. 4, 136. doi:10.1007/s42979-022-01567-2
Kyriakopoulos, G. L. (2021). Globalized inclination to acquire knowledge and skills toward economic development. WSEAS Trans. Bus. Econ. 18, 1349–1369. doi:10.37394/23207.2021.18.125
Kyriakopoulos, G. L., and Sebos, I. (2023). Enhancing climate neutrality and resilience through coordinated climate action: review of the synergies between mitigation and adaptation actions. Climate 11, 105. doi:10.3390/cli11050105
Kyriakopoulos, G. L., Streimikiene, D., and Baležentis, T. (2022). Addressing challenges of low-carbon energy transition. Energies (Basel). 15, 5718. doi:10.3390/en15155718
Li, X., Wang, X., Chen, X., Lu, Y., Fu, H., and Wu, Y. C. (2024). Unlabeled data selection for active learning in image classification. Sci. Rep. 14, 424. doi:10.1038/s41598-023-50598-z
Liu, K., He, S., Li, L., Liu, Y., Huang, Z., Liu, T., et al. (2021). Spectroscopically clean au nanoparticles for catalytic decomposition of hydrogen peroxide. Sci. Rep. 11, 9709. doi:10.1038/s41598-021-89235-y
Luo, Z., Xu, H., and Chen, F. (2019). “Audio sentiment analysis by heterogeneous signal features learned from utterance-based parallel neural network,” in AffCon@ AAAI, 80–87.
Minx, J. C., Lamb, W. F., Callaghan, M. W., Fuss, S., Hilaire, J., Creutzig, F., et al. (2018). Negative emissions—part 1: research landscape and synthesis. Environ. Res. Lett. 13, 063001. doi:10.1088/1748-9326/aabf9b
Mustafa, A., Kazmi, M., Khan, H. R., Qazi, S. A., and Lodi, S. H. (2022). Towards a carbon neutral and sustainable campus: case study of ned university of engineering and technology. Sustainability 14, 794. doi:10.3390/su14020794
Nawi, N. D., Phang, F. A., Yusof, K. M., Rahman, N. F. A., Zakaria, Z. Y., Hassan, S. A. H. S., et al. (2019). Instilling low carbon awareness through technology-enhanced cooperative problem based learning. Int. J. Emerg. Technol. Learn. (Online) 14, 152. doi:10.3991/ijet.v14i24.12135
Nichol, S., Miller, L., Carriveau, R., Fonger, J., and Costa, S. (2021). Financial model of a carbon-neutral microgrid at an ontario high school. Int. J. Environ. Stud. 78, 547–572. doi:10.1080/00207233.2020.1778267
Niu, Z., Zhong, G., and Yu, H. (2021). A review on the attention mechanism of deep learning. Neurocomputing 452, 48–62. doi:10.1016/j.neucom.2021.03.091
Ntanos, S., Kyriakopoulos, G. L., Anagnostopoulos, T., Xanthopoulos, T., Kytagias, C., and Drosos, D. (2022). Investigating the environmental and the energy saving behavior among school principals through classification algorithms. Int. J. Renew. Energy Dev. 11, 449–461. doi:10.14710/ijred.2022.43007
Rosa, L., Sanchez, D. L., and Mazzotti, M. (2021). Assessment of carbon dioxide removal potential via beccs in a carbon-neutral europe. Energy & Environ. Sci. 14, 3086–3097. doi:10.1039/d1ee00642h
Streimikiene, D., Kyriakopoulos, G. L., and Stankuniene, G. (2022). Review of energy and climate plans of baltic states: the contribution of renewables for energy production in households. Energies 15, 7728. doi:10.3390/en15207728
Štreimikienė, D., Mikalauskienė, A., Sturienė, U., and Kyriakopoulos, G. L. (2021). The impact of social media on sales promotion in entertainment companies. E&M Econ. Manag. 24, 189–206. doi:10.15240/tul/001/2021-2-012
Tang, Z. (2022). Molecular fundamentals of upgrading biomass-derived feedstocks over platinum-molybdenum catalysts. Hoboken, New Jersey, USA: Stevens Institute of Technology. Ph.D. thesis.
van der Ven, A. (2018). Utilization of labor towards carbon neutral heat in Dutch terraced houses. Utrecht, Netherlands: Master’s thesis.
Wan, X., Li, X., Wang, X., Yi, X., Zhao, Y., He, X., et al. (2022). Water quality prediction model using Gaussian process regression based on deep learning for carbon neutrality in papermaking wastewater treatment system. Environ. Res. 211, 112942. doi:10.1016/j.envres.2022.112942
Wang, D., Wang, T., and Florescu, I. (2020). Is image encoding beneficial for deep learning in finance? IEEE Internet Things J. 9, 5617–5628. doi:10.1109/jiot.2020.3030492
Wang, F., Harindintwali, J. D., Yuan, Z., Wang, M., Wang, F., Li, S., et al. (2021a). Technologies and perspectives for achieving carbon neutrality. Innovation 2, 100180. doi:10.1016/j.xinn.2021.100180
Wang, H., Chen, Z., Wu, X., and Nie, X. (2019). Can a carbon trading system promote the transformation of a low-carbon economy under the framework of the porter hypothesis? empirical analysis based on the psm-did method. Energy policy 129, 930–938. doi:10.1016/j.enpol.2019.03.007
Wang, L., Yan, X., Fang, M., Song, H., and Hu, J. (2023a). A systematic design framework for zero carbon campuses: investigating the shanghai jiao tong university fahua campus case. Sustainability 15, 7975. doi:10.3390/su15107975
Wang, X. (2022). Active-reactive power collaborative optimization model of electrical interconnection system based on deep learning under the goal of “carbon neutrality”. J. Phys. Conf. Ser. (IOP Publ. 2360, 012032. doi:10.1088/1742-6596/2360/1/012032
Wang, Y., Guo, C.-h., Chen, X.-j., Jia, L.-q., Guo, X.-n., Chen, R.-s., et al. (2021b). Carbon peak and carbon neutrality in China: goals, implementation path and prospects. China Geol. 4, 720–746. doi:10.31035/cg2021083
Wang, Y., Wu, J., Hovakimyan, N., and Sun, R. (2023b). “Balanced training for sparse gans,” in Thirty-seventh Conference on Neural Information Processing Systems.
Wei, J., and Wang, C. (2021). Improving interaction mechanism of carbon reduction technology innovation between supply chain enterprises and government by means of differential game. J. Clean. Prod. 296, 126578. doi:10.1016/j.jclepro.2021.126578
Wu, J., Chen, S., Zhao, Q., Sergazinov, R., Li, C., Liu, S., et al. (2024). Switchtab: switched autoencoders are effective tabular learners. arXiv preprint arXiv:2401.02013, 38, 15924, 15933. doi:10.1609/aaai.v38i14.29523
Xinfa, T., Yifei, S., Chenhui, Z., Lihong, W., and Yan, L. (2023). Research on the promotion of digital teaching and learning toward achieving China’s dual-carbon strategy. Front. Environ. Sci. 11, 44. doi:10.3389/fenvs.2023.983526
Zhang, M., Xie, K., Zhang, Y.-H., Wen, C., and He, J.-B. (2022). Fine segmentation on faces with masks based on a multistep iterative segmentation algorithm. IEEE Access 10, 75742–75753. doi:10.1109/access.2022.3192026
Zheng, J., Li, W., Hong, J., Petersson, L., and Barnes, N. (2022a). “Towards open-set object detection and discovery,” in Proceedings of the IEEE/CVF Conference on Computer Vision and Pattern Recognition, 3961–3970. doi:10.1109/cvprw56347.2022.00441
Zheng, Y., Qi, Y., Tang, Z., Tan, J., Koel, B. E., and Podkolzin, S. G. (2022b). Spectroscopic observation and structure-insensitivity of hydroxyls on gold. Chem. Commun. 58, 4036–4039. doi:10.1039/d2cc00283c
Zhu, H., Jiang, M., Zhang, D., Goh, H. H., Wang, S., Mao, D., et al. (2023). Carbon neutrality pathways exploration-a state-of-the-art study: key technological advancements, current challenges, and potential opportunities. Sustain. Energy Technol. Assessments 60, 103489. doi:10.1016/j.seta.2023.103489
Keywords: carbon neutrality, energy transition, digital transformation, zero-carbon physical education, end-to-end, attention mechanism
Citation: Yuan T, Cai F and Han H (2024) Application of research on carbon neutral strategy of physical education teaching based on attention mechanism in digital energy transformation. Front. Environ. Sci. 12:1268603. doi: 10.3389/fenvs.2024.1268603
Received: 28 July 2023; Accepted: 04 April 2024;
Published: 13 June 2024.
Edited by:
Xuefeng Wang, Beijing Institute of Technology, ChinaReviewed by:
Yassine Himeur, University of Dubai, United Arab EmiratesCopyright © 2024 Yuan, Cai and Han. This is an open-access article distributed under the terms of the Creative Commons Attribution License (CC BY). The use, distribution or reproduction in other forums is permitted, provided the original author(s) and the copyright owner(s) are credited and that the original publication in this journal is cited, in accordance with accepted academic practice. No use, distribution or reproduction is permitted which does not comply with these terms.
*Correspondence: HuiJun Han, MTg2ODA0OTQwNjhAMTYzLmNvbQ==
Disclaimer: All claims expressed in this article are solely those of the authors and do not necessarily represent those of their affiliated organizations, or those of the publisher, the editors and the reviewers. Any product that may be evaluated in this article or claim that may be made by its manufacturer is not guaranteed or endorsed by the publisher.
Research integrity at Frontiers
Learn more about the work of our research integrity team to safeguard the quality of each article we publish.