- 1Department of Finance and Accounting, Henan University of Animal Husbandry and Economy, Zhengzhou, China
- 2School of Business, Central South University, Changsha, China
This study utilizes a panel dataset covering 262 Chinese cities from 2012 to 2019 and employs spatial econometric convergence test models to investigate the impact of public environmental awareness on spatial convergence of urban economic resilience. Empirical results reveal a significant spatial convergence pattern in urban economic resilience, but environmental awareness hampers this convergence. Through a mediation analysis, we find that the role of environmental awareness in the spatial convergence of urban economic resilience is moderated by two critical factors: foreign investment and scientific expenditure. The development of foreign investment and an increase in the scale of scientific expenditure weaken the inhibitory effect of environmental awareness on the spatial convergence of urban economic resilience.
1 Introduction
Over the past decades, the process of urban economic prosperity has encountered risks at various geographic scales (Giannakis and Bruggeman, 2017). Major risk events, exemplified by financial crises and the COVID-19 pandemic, have made people realize the significant value of urban risk resilience and post-crisis recovery capacity within the functioning of the social system (Hill et al., 2008; Hu et al., 2022). Strengthening urban economic resilience has become a fundamental pursuit in modern economic development. However, cities, as micro units within the macro framework of economic development, exhibit spatial interconnections. Urban economic resilience inevitably possesses spatial spillover effects due to these geographical linkages (Jiang et al., 2022; Ren et al., 2023a). Understanding the vulnerability changes and the adaptive capabilities during crises of the economic systems from the spatial dynamic perspective of urban economic resilience is foundational in enhancing overall economic system resilience from the micro to macro level.
An inevitable topic in reshaping urban resilience frameworks and self-recovery structures is the inherent contradiction between the stability of urban economies and environmental sustainability. It is well-known that the development of modern urban economies, which has historically been based on resource consumption and environmental sacrifice, has brought fleeting prosperity on the world economic stage, followed by significant costs in terms of ecological destruction and climate change (Duan et al., 2023). This has led to a sharp increase in environmental awareness among the public. Consequently, current economic development faces the dual challenge of injecting resilient external forces into the economic system while meeting the public’s calls for environmental protection. Public environmental awareness has undoubtedly become a potential factor affecting urban economic resilience. Given the dynamic spatial correlations inherent in economic resilience (Jiang et al., 2022), studying the role of environmental awareness in the spatial evolution of urban economic resilience from the perspective of spatial convergence or divergence is of significant importance in finding a balance between the environment and urban economic development.
Since the concept of economic resilience entered the public consciousness, driven by a sense of urgency, it has become a widely discussed topic in academia. Previous research, when dissecting regional economic resilience, has delved into the specific meaning of economic resilience. For example, Martin and Sunley (2015) proposes that economic resilience should encompass not only the concepts of vulnerability and sensitivity but also adaptability to new development pathways following shocks. Bristow and Healy (2014); Hu and Yang (2019) points out that resilience is an economic behavior, and in this dynamic process, human agency plays a decisive role. On the other hand, specific factors influencing economic resilience have also been thoroughly explored. Notably, the pivotal role of industrial structure in enhancing economic resilience (Brown and Greenbaum, 2017), the stimulation of a demographic dividend on economic resilience (Jiang et al., 2022), and the constraints on the improvement of economic resilience posed by government quality and institutional environment (Tan et al., 2020) are well-documented. Despite the shared understanding in academia regarding the basic framework of economic resilience and a range of related factors, the burning topic of environmental awareness has not yet been incorporated into the discussion. The exploration of its relevance to the dynamic spatial evolution of urban economic resilience is necessary and timely.
Based on this, we first collect panel data from 262 cities in China, calculate the indicator of urban economic resilience, and gather data on the frequency of environmental pollution-related keyword searches by internet users in each city using the Baidu search engine. Subsequently, we utilize convergence tests improved with three major spatial econometric models (SDM, SAR, and SEM) to fit spatial convergence models for urban economic resilience, including absolute and conditional convergence models. Next, we analyze the specific effects of environmental awareness on spatial convergence of urban economic resilience and conduct corresponding robustness tests. Finally, to explore the specific pathways through which environmental awareness influences the spatial convergence of urban economic resilience, we conduct moderation effect analysis.
Through empirical testing, we have the following research findings: 1) Urban economic resilience exhibits significant spatial convergence characteristics, meaning cities with lower resilience have a faster growth rate, leading to a tendency for different cities in adjacent regions to converge. 2) Environmental awareness hampers the spatial convergence of urban economic resilience. In simpler terms, heightened environmental awareness decelerates the pace at which neighboring cities converge regarding economic resilience. 3) Within the context of environmental awareness affecting the spatial convergence of urban economic resilience, foreign investment and scientific expenditure act as moderators. To be more precise, larger foreign capital investments or increased scientific expenditures weaken the inhibitory impact of environmental awareness on the spatial convergence of urban economic resilience.
This study makes significant academic contributions and has practical implications in three main aspects. First, previous research has primarily focused on economic infrastructure when exploring the factors affecting urban economic resilience. This study, for the first time, examines the effects of heightened public environmental awareness, aligning with the contemporary need to balance environmental and economic benefits, which represents a mainstream value. Second, this study monitors how diverse influencing factors affect the dynamic spatial evolution of urban economic resilience. This provides a policy entry point for enhancing the resilience of the entire macroeconomic system by starting from micro-geographic units. Third, this study analyzes the specific channels through which environmental awareness affects the spatial convergence of urban economic resilience. This offers a theoretical basis for building a balanced economic framework that integrates environmental protection and economic development, with a focus on foreign investment and scientific expenditure.
The remainder of this study is organized as follows: Section 2 provides a comprehensive summary and review of relevant literature. Section 3 introduces the empirical methods employed in this study and detail the selected variables. Following that, Section 4 analyzes the empirical test results. Section 5 conducts a mechanism analysis to delve deeper into the findings. Finally, Section 6 draws the conclusions based on the research and offers policy recommendations in line with the study’s outcomes.
2 Literature review
2.1 Economic resilience and its influencing factors
In the field of economic geography, resilience is regarded as a non-equilibrium dynamic process involving multiple actors (Martin, 2012; Bristow and Healy, 2014). Traditionally, resilience comprises three categories: engineering resilience, ecological resilience, and adaptive resilience (Martin, 2012). Engineering resilience can be understood as the speed at which a system returns from a non-equilibrium state to an equilibrium state. Ecological resilience refers to the process of absorbing destabilizing factors after the system departs from stability and moves toward the next equilibrium state (Holling, 1973). Adaptive resilience measures the system’s ability to undergo internal restructuring and adjustments after experiencing expected risks and external shocks to minimize external damage (Martin and Sunley, 2015). Compared to the equilibrium methods used in engineering and ecological resilience, the continuous non-equilibrium dynamic adjustment process emphasized by adaptive resilience aligns better with the contemporary economic geography’s definition of resilience (Hu and Hassink, 2017; 2020). Therefore, in the context of economic geography, resilience typically encompasses four dimensions: resistance to risks, stability in the face of shocks, self-recovery after shocks, and forward innovation after recovery (Martin, 2012). Current research attempts to operationalize economic resilience using existing economic indicators, with employment, exports, GDP, and other data commonly used to construct resilience indices (Di Caro, 2015; Doran and Fingleton, 2016). While there may be multiple interpretations of economic resilience and variations in measurement methods, there is a consensus regarding the regional heterogeneity of economic resilience in geographical space (Giannakis and Bruggeman, 2017).
The differentiation in how different regions handle economic risks in terms of resistance and adaptability has led to extensive discussions about the influencing factors of economic resilience. It is important to note that regions with structural weaknesses do not necessarily exhibit lower economic resilience (Tan et al., 2020). Several key factors affecting the dynamic recovery capacity of regional economic systems have been identified, including human capital, industrial structure, government roles, and technological development (Martin et al., 2016; Tan et al., 2020; Hu et al., 2022; Ren et al., 2023b). Diversification in industrial structure, as opposed to a singular structure, reduces development resistance, disperses external adverse shocks, and acts as a “buffer” (Angulo et al., 2018; Cainelli et al., 2019). The “talent pool” effect created by population concentration provides support in terms of human capital for regional economic development. It accelerates economic structural adjustments and upgrades, enhancing the resilience of regional economic systems (Jiang et al., 2022). Furthermore, the supplementary support provided by national institutions in the aftermath of a crisis accelerates the recovery process after internal equilibrium is disrupted, serving as a significant factor in enhancing economic resilience (Ezcurra Orayen and Ríos Ibáñez, 2019). Given the non-uniform distribution of different influencing factors in geographic space, the rationale for different regional economic systems to exhibit varying features of dynamic adaptability and adjustment capabilities during crises becomes evident.
2.2 Environment and economic resilience
The relationship between the environment and the economy is a dialectical contradiction. The recovery of an impacted economic system relies on favorable environmental conditions, while economic recovery, to some extent, increases the pressure on environmental governance by sacrificing the environment. The interdependence and mutual opposition between the environment and the economy make finding a balance between the two an enduring topic in academia. Gasper et al. (2011); Ren et al. (2023c) emphasize that deteriorating environmental conditions and climate crises are key obstacles to urban economic development and represent the primary challenges facing urban economic development today. Building on this, Dodman et al. (2013) underscore the need to integrate environmental management into urban planning, creating a mutually reinforcing relationship between urban economic development and environmental regulation. Guan et al. (2011) conduct systematic modeling and dynamic assessments of urban environments, economies, and resources, highlighting that maintaining a healthy environment is the foundation for sustainable urban economic development. The symbiotic relationship between urban environments and economic development makes the environment a fundamental element affecting urban economic resilience. As pointed out by Briguglio et al. (2006), the environment is not only a significant component of vulnerability but also contributes to the recovery of the economy after experiencing shocks. Therefore, there exists a certain causality between the environment and urban economic resilience.
2.3 Literature gaps
Previous research has defined the concept of economic resilience in multidimensional ways and attempted to uncover the influencing factors within economic structures. However, environmental issues outside the realm of economic topics have seldom been connected to urban economic resilience. Furthermore, as economic resilience is a dynamic process, it possesses not only temporal dynamics but also spatial dynamics. Previous studies have rarely delved into how economic resilience evolves in geographic space. Therefore, this study incorporates environmental awareness as an emerging element into the framework of factors affecting urban economic resilience and explores its specific impact on the spatial convergence process of urban economic resilience, thus addressing a gap in previous research.
3 Empirical methods and data
This section presents the econometric models used in this study to measure the spatial correlation of urban economic resilience and conduct spatial convergence tests. It also provides information on the basis for selecting variables, data sources, and a basic analysis of each variable.
3.1 Empirical models
3.1.1 Moran’s index
Given that Moran’s Index has been found to be particularly suitable for measuring spatial correlation in economic indicators (Wang et al., 2023), we employ this method to test whether urban economic resilience exhibits spatial correlation. The calculation method for Moran’s Index is as follows:
In Eq. 1,
3.1.2 Spatial σ convergence test
According to the research of Rey and Dev (2006), in order to overcome the limitation of traditional σ convergence tests, which do not consider spatial effects, constructing a spatial σ convergence index by extracting the standard deviation from the Spatial Lag Model (SEM) can better reflect the dynamic changes in the disparities of economic indicators among all individuals. The basic form of SEM is as follows:
In the Eqs 2, 3, λ represents the spatial error coefficient, W is the spatial weight matrix, I is the Moran’s Index,
3.1.3 Spatial β convergence test
Compared to traditional β convergence tests, spatial β convergence models take into account the spatial error effects and spatial lag effects resulting from the mutual influence between different micro-level geographic units. This makes the results of the model testing more in line with economic reality. In order to examine whether cities with different initial levels of economic resilience have different growth rates of economic resilience, which would lead to a spatially convergent trend in economic resilience, we draw inspiration from the research of Cheng et al. (2022) and construct spatial β convergence tests based on Spatial Durbin Model (SDM), Spatial Autoregressive Model (SAR), and Spatial Error Model (SEM):
Eqs 4–6 represent the absolute spatial β convergence tests constructed based on SDM, SAR, and SEM, while Eqs 7–9 represent the conditional spatial β convergence tests constructed based on SDM, SAR, and SEM. The conditional spatial β convergence tests are formed by introducing several control variables into the models of the absolute spatial β convergence tests, aiming to account for potential variations in the impact of different influencing factors on economic resilience across cities.
In Eqs 4–9,
3.2 Data
3.2.1 Variables
3.2.1.1 Dependent variable: urban economic resilience (ER)
In accordance with the definitions and measurement methods of urban economic resilience as outlined in the studies by Faggian et al. (2018), Holl (2018), and Martin (2012), this study defines urban economic resilience as the capacity of an economic system to withstand crises and risks and its dynamic adjustment ability to adapt after facing shocks. The specific calculation method is as follows:
In Eq. 10,
3.2.1.2 Explanatory variables
Core explanatory variable: Environmental awareness (EA). Drawing inspiration from the approach used in the study by Zhu and Wang (2022) to measure government tourism awareness, this study employs the frequency of Baidu search terms related to environmental pollution as a proxy variable for public environmental awareness. The data for this variable is sourced from the Mark Data Network macrodatas.cn.
Other control variable: Industrial structure (IS). Given the complex impact of industrial structure on regional economic resilience (Tan et al., 2020), we include the proportion of the tertiary industry’s value added to GDP as a proxy variable for industrial structure in the control variables. Data for this variable is sourced from the China City Statistical Yearbook.
Human resources (HR). Given that human resources are a key factor influencing urban economic resilience (Zhou and Qi, 2023), we incorporate the number of urban employees as a proxy variable for human resources into the control variables. Data for this variable is obtained from the China City Statistical Yearbook.
Government intervention (GI). Given that government intervention has a significant impact on urban economic resilience (Hu et al., 2022), we include government fiscal expenditure as a proxy variable for government intervention in the control variables. Data for this variable is obtained from the China City Statistical Yearbook.
Foreign investment (FI). Given the beneficial impact of foreign investment on urban economic resilience (Jiang et al., 2022), we include the number of foreign-invested enterprises as a proxy variable for foreign investment in the control variables. Data for this variable is sourced from the China City Statistical Yearbook.
Scientific expenditure (SE). Considering that scientific expenditure effectively supports urban economic resilience (Tan et al., 2017), we include the total scientific expenditure as a proxy variable for scientific expenditure in the control variables. Data for this variable is sourced from the China City Statistical Yearbook.
Considering the data incompleteness following the occurrence of the pandemic, we select panel data at the city level for China from 2012 to 2019 as our research sample. To standardize the data scales across different variables, we apply logarithmic transformations to all control variables. A summary of the selected variables for this study is provided in Table 1.
3.2.2 Initial analysis
The descriptive statistics for each variable are presented in Table 2. First, the overall mean of urban economic resilience (ER) is −0.019, indicating that Chinese cities have room for improvement in their ability to withstand economic downturns and recover in times of crisis. Second, the between-group standard deviation of environmental awareness (EA) is 0.980, significantly higher than the within-group standard deviation of 0.316, suggesting significant differences in the level of environmental pollution control awareness among Chinese cities in the process of economic development. Third, the between-group standard deviations of industrial structure (IS), human resources (HR), government intervention (GI), foreign investment (FI), and scientific expenditure (SE) are all higher than the within-group standard deviations. This indicates that Chinese cities exhibit significant regional heterogeneity in terms of their economic development patterns, resource endowment, and development processes.
4 Empirical analysis
This section systematically provides the outcomes of the spatial correlation analysis for urban economic resilience, the spatial σ convergence test results, absolute spatial β convergence test results, conditional spatial β convergence test results, and robustness test results.
4.1 Spatial correlation measurement
To grasp the geographic distribution of cities in China exhibiting different levels of economic resilience, we initially create spatial status maps of urban economic resilience, as illustrated in Figure 1. Figures 1A, B are generated based on data from 2012 to 2019, respectively. By comparing Figure 1A with Figure 1B, it is evident that urban economic resilience has transitioned from weak spatial correlation to strong clustering over time. As of 2019, cities with elevated economic resilience were primarily clustered in the southern regions, while cities with lower economic resilience were predominantly located in the northern regions. Looking at the color distribution, it is apparent that urban economic resilience exhibits clear spatial correlation characteristics in its geographical distribution.
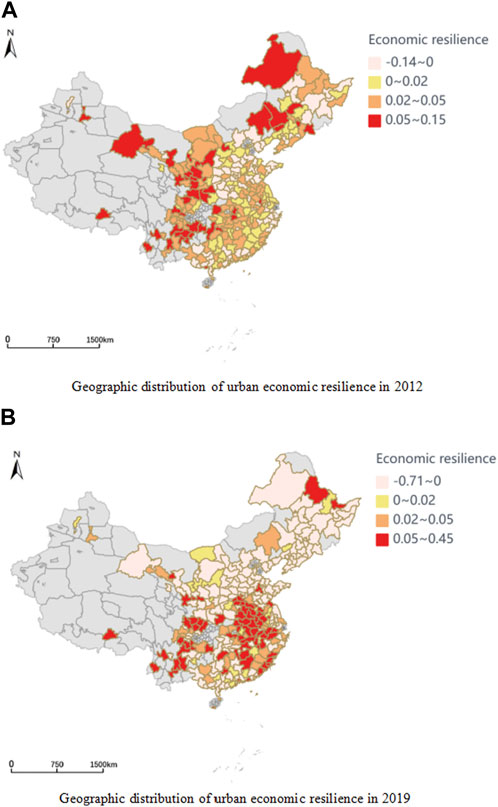
FIGURE 1. Geospatial distribution maps of urban economic resilience in 2012 and 2019. Notes: The darker the color that covers a region, the stronger the urban economic resilience of that area.
To quantitatively measure the spatial correlation of urban economic resilience, we conduct the Moran’s I test and generate Moran scatter plots for all years within the sample period. Table 3 presents the results of the Moran’s I test, showing that the Moran’s I values for the years 2012–2019 are all significantly positive at the 1% level. This indicates that urban economic resilience exhibits significant positive spatial autocorrelation. Figure 2 illustrates the Moran scatter plots for the years 2012–2019. These plots are predominantly concentrated in the first and third quadrants, further confirming the presence of positive spatial autocorrelation in urban economic resilience. Song et al. (2022) also affirm the existence of spatial correlation when examining the spatial evolution characteristics of urban economic resilience in China.
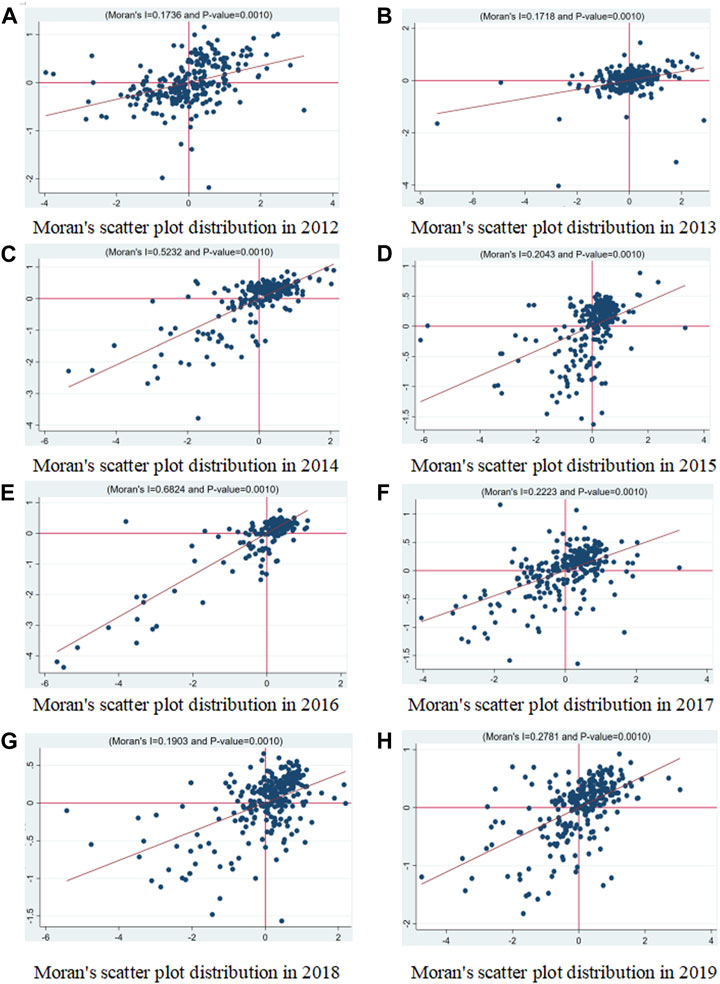
FIGURE 2. Moran’s scatter plots of urban economic resilience from 2012 to 2019. Notes: The majority of Moran’s scatter plots are distributed in the first and third quadrants, confirming the presence of positive spatial autocorrelation in urban economic resilience.
4.2 Spatial σ convergence
In order to examine the dynamic evolution of spatial disparities in urban economic resilience from a national perspective, we create a σ index graph based on the SEM standard deviation to illustrate the situation of spatial σ convergence, as shown in Figure 3. The σ index in Figure 3 fluctuates and undergoes two noticeable turning points: one in 2015 when it transitions from decreasing to increasing, and another in 2017 when it shifts from increasing to decreasing. The potential reasons behind these fluctuations can be attributed to China’s entry into the early stages of the Thirteenth Five-Year Plan after 2015, which brought about comprehensive reforms in areas such as fiscal policy, finance, and businesses. Different cities faced varying impacts due to differences in their economic foundations and resource endowments, resulting in temporary differentiation in urban economic resilience. In 2017, China experienced a significant rebound in the strength of the Chinese yuan exchange rate, alleviating capital outflows, shifting market expectations. From a fundamental perspective, China’s economic resilience demonstrated overall robustness.
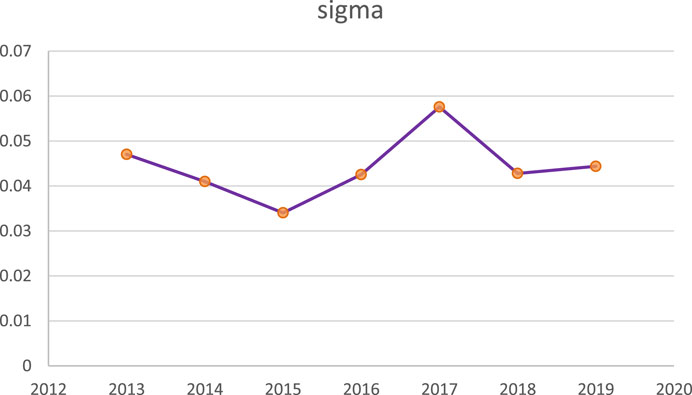
FIGURE 3. Trends in spatial σ convergence index. Notes: The decreasing range of the σ index signifies spatial convergence of urban economic resilience at a national level.
4.3 Spatial β convergence
4.3.1 Absolute spatial β convergence
To examine whether urban economic resilience spontaneously exhibits spatial convergence driven by growth rates, we conduct the absolute spatial β convergence test. Table 4 presents the results of the LM test conducted before the β convergence test. The LM test provides significant spatial error and spatial lag effects at the 1% level, which are prerequisites for the use of spatial econometric models.
Table 5 reports the regression results of the absolute spatial β convergence test for urban economic resilience. As shown in Table 5, the spatial coefficients λ/ρ is significant at the 1% level for all three spatial econometric models, indicating a good fit to the data. The convergence coefficient β, which we are interested in, is −0.827 in SDM, −0.679 in SAR, and −0.822 in SEM, and all are statistically significant at the 1% level. This suggests that, without considering differences in urban endowments and development characteristics, urban economic resilience exhibits spatial convergence (Shi et al., 2022). In other words, cities with lower initial levels of economic resilience experience higher growth rates in economic resilience, resulting in spontaneous convergence across different levels of urban economic resilience in geographical space.
4.3.2 Conditional spatial β convergence
Due to the varying economic foundations and development endowments of different cities, the impact of influencing factors on urban economic resilience differs, resulting in distinct convergence paths across different geographical regions. We further consider the influence of multiple exogenous variables affecting urban economic resilience and conduct the conditional spatial β convergence test. Table 6 reports the regression results of the conditional spatial β convergence test. In Table 6, λ/ρ remains statistically significant at the 1% level for all spatial econometric models. The convergence coefficients β in SDM, SAR, and SEM are −0.881, −0.753, and −0.876, respectively, all statistically significant at the 1% level. This suggests that urban economic resilience exhibits conditional spatial convergence. In other words, even when considering the various exogenous factors affecting urban economic resilience, cities with lower economic resilience still experience relatively faster growth, leading to the convergence of urban economic resilience within a specific geographical region.
This study aims to investigate the impact of environmental awareness on the spatial convergence of urban economic resilience. In Table 6, the coefficients of environmental awareness (EA) in SDM, SAR, and SEM are 0.011, 0.011, and 0.009, respectively, and they are significant. This indicates that an increase in public environmental awareness inhibits the spatial convergence of urban economic resilience. On the one hand, the environment serves as a source of vulnerability in the process of urban economic development. Adverse environmental conditions have a disruptive and damaging impact on the development of the urban economy itself. Therefore, improving the quality of environmental management is one of the important ways to enhance urban economic resilience (Briguglio et al., 2006). The motivation to enhance economic resilience while undergoing environmental governance pressure varies spatially due to differences in the environmental conditions of different cities.
On the other hand, there is an inherent contradiction between the self-recovery of urban economies after crises and the maintenance of a healthy ecological environment. The economic downturn caused by significant public health events like the COVID-19 pandemic implies that urban economies, seeking recovery from such crises, are bound to undergo a brief period of extensive economic development (Wei et al., 2023). This rapid economic development often leads to a significant increase in public environmental concerns (Saadat et al., 2020). The public’s call for environmental responsibility creates resistance to the dynamic adjustments of urban economies, conflicting with the need for economic recovery. Therefore, this aspect of increased environmental awareness hinders the growth rate of urban economic resilience. For these reasons, the enhancement of environmental awareness undoubtedly slows down the spatial convergence of urban economic resilience.
Regarding the control variables, industrial structure (IS), the coefficients under SDM, SAR, and SEM are −0.066, −0.074, and −0.086, respectively, and they are significant. This suggests that the optimization of the industrial structure promotes the spatial convergence of urban economic resilience. First, in the process of urban economic development, the growth of the tertiary sector serves as the driving force behind demand expansion and stimulates consumption. This enables cities with well-developed tertiary sectors to have stronger recovery capabilities after economic crises (Jiang et al., 2022). Second, the service-oriented tertiary sector has strong innovation attributes. Under the influence of high technology and quality management, it equips urban economies with robust market crisis response capabilities and resilience (Hu et al., 2022). Third, the growth of the tertiary sector signifies a break from the traditional single industrial structure of urban economies. Different industries respond differently to economic crises, and diversified economic structures reflect the risk diversification principle of not putting all the eggs in one basket (Tan et al., 2020). This enhances the urban economy’s ability to resist risks. As a result, optimizing the industrial structure by emphasizing the growth of the tertiary sector hastens the enhancement of economic resilience in cities initially possessing lower economic resilience. In essence, it expedites the spatial convergence of urban economic resilience.
The coefficients for human resources (HR) under SAR and SEM is 0.026 and 0.024, respectively, and it is significant. This suggests that the improvement of human resources inhibits the spatial convergence of urban economic resilience. Considering China’s current population and labor force structure, China is currently in a demographic dividend era, with a significant portion of the population being working-age youth, and there has been substantial growth in a highly skilled workforce in recent years. The massive influx of labor into cities has accelerated the urbanization and industrialization processes (Fan, 2005), enhancing cities’ abilities to withstand risks and economic recovery. However, it's evident that China’s urban economy exhibits extreme disparities in development. Major first-tier cities attract the majority of the highly skilled labor force, while economically less developed cities have a limited capacity to absorb high-end technical talent. As a result, this has led to an uneven geographic distribution of labor resources in China (Fang et al., 2009). Therefore, the explosive growth of human resources in China has exacerbated the economic resilience disparities among different cities, which, in turn, hampers the spatial convergence of urban economic resilience.
The coefficients for government intervention (GI) under SDM, SAR, and SEM is 0.087, 0.083, and 0.069, respectively, and all of them are significant. This indicates that an increased level of government intervention hampers the spatial convergence of urban economic resilience. Government intervention in local economies is primarily carried out through fiscal means. For cities with relatively fragile economic systems, their capacity for self-recovery is limited, and the restoration of their economic structure relies mainly on government fiscal adjustments. In the long term, a prolonged dependence on government finances can lead to urban economies gradually losing their immunity to crises, resulting in reduced survival capabilities during crises (Wang and Li, 2022). As discovered by Guo and Xu (2019), government intervention has a negative impact on urban economic resilience. Therefore, the strengthening of government intervention levels accentuates the differences in economic resilience between cities with poor recovery capacity and those with strong economic recovery capabilities. In other words, increased government fiscal intervention suppresses the spatial convergence of urban economic resilience.
4.4 Robustness test
To validate the robustness of our findings regarding the inhibitory effect of environmental awareness on the spatial convergence of urban economic resilience, we perform robustness tests by replacing the original spatial weight matrix with a geographic distance matrix and an economic matrix, respectively, instead of the original squared geographic distance matrix (Lou et al., 2021; Yuan et al., 2023). The results of the robustness tests are presented in Tables 7, 8. Tables 7, 8 demonstrate that the spatial terms λ/ρ and the coefficients for β remain significant at the 1% level. Moreover, the coefficients for the core explanatory variable, environmental attention (EA), and the coefficients for other control variables do not exhibit significant changes in sign or significance when compared to the benchmark regression results. Hence, we can infer that our array of findings concerning the influence of environmental awareness and other factors on the spatial convergence of urban economic resilience is strong and reliable.
5 Mechanism analysis
5.1 The moderating effect of foreign investment
Upon verifying the inhibitory impact of environmental awareness on the spatial convergence of urban economic resilience, we proceed to examine the moderating factors that affect the relationship between environmental awareness and the spatial convergence of urban economic resilience. First, we consider the moderating effect of foreign investment (FI). With the influx of foreign capital, cities benefit from cutting-edge production technology, international market information, and advanced management experience. This enhances energy efficiency in the urban economic development process and reduces the level of environmental degradation (Luo et al., 2021). Consequently, public anxiety about environmental deterioration is alleviated, and the city’s economy can allocate resources more flexibly when responding to crises and making dynamic adjustments, without being constrained by environmental degradation. This ultimately strengthens urban economic resilience. In other words, the expansion of foreign investment acts as a reverse moderator of the inhibitory effect of environmental awareness on the spatial convergence of urban economic resilience.
Based on this, we introduce the interaction term between environmental awareness (EA) and foreign investment (FI), denoted as EA*SE, into the conditional spatial β convergence test model, and the regression results are shown in Table 9. The spatial term coefficients ρ/λ are significant in SDM, SAR, and SEM, and the coefficients of the interaction term EA*FI are significant in SDM, SAR, and SEM, with values of 0.016, 0.016, and 0.017, respectively. Consequently, we conclude that foreign investment has a moderating effect on the inhibitory impact of environmental awareness on the spatial convergence of urban economic resilience. Specifically, foreign investment hinders this inhibition Table 10.
5.2 The moderating effect of scientific expenditure
Additionally, we also consider the moderating effect of scientific expenditure. The scale of scientific expenditure within a city provides ample financial support for businesses to undertake technological improvements and innovation projects, thereby fully unlocking their innovation potential and stimulating advancements in green technology (Liu, 2021). This results in businesses significantly reducing pollution and excessive resource consumption during their production and operations, leading to a gradual reduction in environmental pressure on the city’s economy. Furthermore, the city’s economy can transmit more positive sentiments to society. As a result, public awareness for the environment decreases, and the city’s economic development has a better environmental foundation for risk prevention and structural repair, ultimately strengthening urban economic resilience. In other words, the increase in scientific expenditure serves as a moderating factor that counteracts the inhibitory impact of environmental awareness on the spatial convergence of urban economic resilience.
Based on this, we introduce the interaction term between environmental awareness (EA) and scientific expenditure (SE), denoted as EA*SE, into the conditional spatial β convergence test model, and the regression results are shown in Table 9. The spatial term coefficients ρ/λ are significant in SDM, SAR, and SEM, and the coefficients of the interaction term EA*SE are also significant, with values of 0.010, 0.008, and 0.010, respectively. Consequently, it can be concluded that scientific expenditure moderates the inhibitory effect of environmental awareness on the spatial convergence of urban economic resilience. Specifically, scientific expenditure hinders this inhibition.
6 Conclusion
Since the devastating impact of the 2008 financial crisis on the global economic systems, enhancing economic resilience has become a pivotal aspect of subsequent economic development (Hill et al., 2008). However, the recent rapid growth of urban economies has led to severe ecological and resource challenges, creating a delicate balance between the demand for environmental improvement and the need to strengthen urban economic resilience. Given the strong spatial interconnections of urban economic resilience, environmental awareness naturally has an influence on the spatial dynamics of urban economic resilience. Therefore, in an effort to find a balance between environmental benefits and economic benefits, this study examines the specific role of public environmental awareness in the spatial convergence of urban economic resilience.
Our empirical results confirm a strong spatial convergence trend in urban economic resilience, implying that the growth rates of economic resilience in neighboring cities vary due to different initial levels. Specifically, cities with lower initial resilience levels experience faster growth, leading to a convergence effect in geographical space, where cities become more similar in terms of economic resilience over time. Importantly, we find that increased public environmental awareness hinders the spatial convergence of urban economic resilience, meaning that higher levels of environmental awareness among the public slow down the convergence of urban economic resilience. Additionally, we observe that optimizing industrial structure promotes the spatial convergence of urban economic resilience, while the expansion of human resources and increased government intervention suppress the spatial convergence of urban economic resilience. Furthermore, through mechanism analysis, we confirm that while environmental awareness exerts an inhibitory effect on spatial convergence of urban economic resilience, foreign investment and scientific expenditure play significant negative moderating roles.
Our study holds significant policy implications. First, concerning the spatial convergence trend of urban economic resilience, we should focus on removing policy barriers between neighboring cities and leverage the demonstrative role of major cities. This will enable smaller cities to autonomously enhance their economic resilience driven by spatial spillover effects. Second, due to the inhibitory effect of public environmental awareness on the spatial convergence of urban economic resilience, policymakers should prioritize environmental regulations. Ecological restoration should precede economic recovery, as a healthy ecological environment nurtures stable economic recovery capability. This will help alleviate public environmental anxiety and reduce resistance to policy implementation. Third, considering the moderating effects of foreign investment and scientific expenditure, the government should leverage China’s advantages in foreign investment and allocate a higher proportion of research funding in fiscal expenditure. This will maximize the benefits of foreign investment and scientific spending in stimulating innovation among Chinese enterprises, reduce the burden of environmental governance, and expedite the enhancement of urban economic resilience.
To facilitate further exploration of the dynamic role of public environmental awareness on urban economic resilience, we also outline the limitations of our study. Given that the development opportunities and patterns of urban economies are highly influenced by their geographical locations, coastal cities and inland cities exhibit substantial differences in the spatial behavior of economic resilience. Public environmental awareness also varies due to the differing ecological conditions in the regions where cities are located. This study employes a nationwide sample of cities. In future research, it would be beneficial to conduct heterogeneous analyses to make the research findings more conducive to tailoring economic development policies to specific regional needs, taking into account the differences in environmental concerns and ecological conditions.
Data availability statement
The original contributions presented in the study are included in the article/Supplementary material, further inquiries can be directed to the corresponding author.
Author contributions
YL: Supervision, Writing–review and editing. YX: Data curation, Formal Analysis, Methodology, Software, Writing–original draft.
Funding
The author(s) declare financial support was received for the research, authorship, and/or publication of this article. This study was funded by National Social Science Foundation of China (NO:20BJY248).
Conflict of interest
The authors declare that the research was conducted in the absence of any commercial or financial relationships that could be construed as a potential conflict of interest.
Publisher’s note
All claims expressed in this article are solely those of the authors and do not necessarily represent those of their affiliated organizations, or those of the publisher, the editors and the reviewers. Any product that may be evaluated in this article, or claim that may be made by its manufacturer, is not guaranteed or endorsed by the publisher.
Supplementary material
The Supplementary Material for this article can be found online at: https://www.frontiersin.org/articles/10.3389/fenvs.2023.1326701/full#supplementary-material
References
Angulo, A., Mur, J., and Trívez, F. (2018). Measuring resilience to economic shocks: an application to Spain. Ann. Regional Sci. 60, 349–373. doi:10.1007/s00168-017-0815-8
Briguglio, L., Cordina, G., Farrugia, N., and Vella, S. (2006). “Conceptualizing and measuring economic resilience,” in Building the economic resilience of small states (Malta: Islands and Small States Institute of the University of Malta and London: Commonwealth Secretariat), 265–288. doi:10.22459/PIRIG.11.2005.03
Bristow, G., and Healy, A. (2014). Regional resilience: an agency perspective. Reg. Stud. 48, 923–935. doi:10.1080/00343404.2013.854879
Brown, L., and Greenbaum, R. T. (2017). The role of industrial diversity in economic resilience: an empirical examination across 35 years. Urban Stud. 54, 1347–1366. doi:10.1177/0042098015624870
Cainelli, G., Ganau, R., and Modica, M. (2019). Industrial relatedness and regional resilience in the European Union. Pap. Regional Sci. 98, 755–778. doi:10.1111/pirs.12377
Cheng, J., Xu, L., Wang, H., Geng, Z., and Wang, Y. (2022). How does the marginal abatement cost of CO2 emissions evolve in Chinese cities? An analysis from the perspective of urban agglomerations. Sustain. Prod. Consum. 32, 147–159. doi:10.1016/j.spc.2022.04.013
Di Caro, P. (2015). Recessions, recoveries and regional resilience: evidence on Italy. Camb. J. Regions, Econ. Soc. 8, 273–291. doi:10.1093/cjres/rsu029
Dodman, D., McGranahan, G., and Dalal-Clayton, D. B. (2013). Integrating the environment in urban planning and management: key principles and approaches for cities in the 21st century. Switzerland: United Nations Environment Programme Geneva.
Doran, J., and Fingleton, B. (2016). Employment resilience in Europe and the 2008 economic crisis: insights from micro-level data. Reg. Stud. 50, 644–656. doi:10.1080/00343404.2015.1088642
Duan, X., Xiao, Y., Ren, X., Taghizadeh-Hesary, F., and Duan, K. (2023). Dynamic spillover between traditional energy markets and emerging green markets: implications for sustainable development. Resour. Policy 82, 103483. doi:10.1016/j.resourpol.2023.103483
Ezcurra Orayen, R., and Ríos Ibáñez, V. (2019). Quality of government and regional resilience in the European union. Evidence from the great recession. Pap. Regional Sci. 98, 1267–1290. doi:10.1111/pirs.12417
Faggian, A., Gemmiti, R., Jaquet, T., and Santini, I. (2018). Regional economic resilience: the experience of the Italian local labor systems. Ann. Regional Sci. 60, 393–410. doi:10.1007/s00168-017-0822-9
Fan, C. C. (2005). Interprovincial migration, population redistribution, and regional development in China: 1990 and 2000 census comparisons. Prof. Geogr. 57, 295–311. doi:10.1111/j.0033-0124.2005.00479.x
Gasper, R., Blohm, A., and Ruth, M. (2011). Social and economic impacts of climate change on the urban environment. Curr. Opin. Environ. Sustain. 3, 150–157. doi:10.1016/j.cosust.2010.12.009
Giannakis, E., and Bruggeman, A. (2017). Economic crisis and regional resilience: evidence from Greece. Pap. Regional Sci. 96, 451–476. doi:10.1111/pirs.12206
Guan, D., Gao, W., Su, W., Li, H., and Hokao, K. (2011). Modeling and dynamic assessment of urban economy–resource–environment system with a coupled system dynamics–geographic information system model. Ecol. Indic. 11, 1333–1344. doi:10.1016/j.ecolind.2011.02.007
Guo, J., and Xu, Z. (2019). Research on the influence of industrial related variety on regional economic resilience-Analysis of threshold effect based on regional innovation level. Sci. Technol. Prog. Policy 36, 39–47.
Holl, A. (2018). Local employment growth patterns and the Great Recession: the case of Spain. J. Regional Sci. 58, 837–863. doi:10.1111/jors.12392
Holling, C. S. (1973). Resilience and stability of ecological systems. Annu. Rev. Ecol. Syst. 4, 1–23. doi:10.1146/annurev.es.04.110173.000245
Hu, X., and Hassink, R. (2017). Exploring adaptation and adaptability in uneven economic resilience: a tale of two Chinese mining regions. Camb. J. Regions, Econ. Soc. 10, 527–541. doi:10.1093/cjres/rsx012
Hu, X., and Hassink, R. (2020). Adaptation, adaptability and regional economic resilience: a conceptual framework. Handb. regional Econ. Resil. 2020, 54–68. doi:10.4337/9781785360862.00009
Hu, X., Li, L., and Dong, K. (2022). What matters for regional economic resilience amid COVID-19? Evidence from cities in Northeast China. Cities 120, 103440. doi:10.1016/j.cities.2021.103440
Hu, X., and Yang, C. (2019). Institutional change and divergent economic resilience: path development of two resource-depleted cities in China. Urban Stud. 56, 3466–3485. doi:10.1177/0042098018817223
Jiang, J., Zhang, X., and Huang, C. (2022). Influence of population agglomeration on urban economic resilience in China. Sustainability 14, 10407. doi:10.3390/su141610407
Liu, C. (2021). Infrastructure public–private partnership (PPP) investment and government fiscal expenditure on science and technology from the perspective of sustainability. Sustainability 13, 6193. doi:10.3390/su13116193
Lou, L., Li, J., and Zhong, S. (2021). Sulfur dioxide (SO2) emission reduction and its spatial spillover effect in high-tech industries: based on panel data from 30 provinces in China. Environ. Sci. Pollut. Res. 28, 31340–31357. doi:10.1007/s11356-021-12755-7
Luo, Y., Salman, M., and Lu, Z. (2021). Heterogeneous impacts of environmental regulations and foreign direct investment on green innovation across different regions in China. Sci. total Environ. 759, 143744. doi:10.1016/j.scitotenv.2020.143744
Martin, R. (2012). Regional economic resilience, hysteresis and recessionary shocks. J. Econ. Geogr. 12, 1–32. doi:10.1093/jeg/lbr019
Martin, R., and Sunley, P. (2015). On the notion of regional economic resilience: conceptualization and explanation. J. Econ. Geogr. 15, 1–42. doi:10.1093/jeg/lbu015
Martin, R., Sunley, P., Gardiner, B., and Tyler, P. (2016). How regions react to recessions: resilience and the role of economic structure. Reg. Stud. 50, 561–585. doi:10.1080/00343404.2015.1136410
Ren, X., Cao, Y., Liu, P. J., and Han, D. (2023a). Does geopolitical risk affect firms' idiosyncratic volatility? Evidence from China. Int. Rev. Financial Analysis 90, 102843. doi:10.1016/j.irfa.2023.102843
Ren, X., Zeng, G., and Sun, X. (2023b). The peer effect of digital transformation and corporate environmental performance: empirical evidence from listed companies in China. Econ. Model. 128, 106515. doi:10.1016/j.econmod.2023.106515
Ren, X., Zhong, Y., Cheng, X., Yan, C., and Gozgor, G. (2023c). Does carbon price uncertainty affect stock price crash risk? Evidence from China. Energy Econ. 122, 106689. doi:10.1016/j.eneco.2023.106689
Rey, S. J., and Dev, B. (2006). σ-convergence in the presence of spatial effects. Pap. Regional Sci. 85, 217–234. doi:10.1111/j.1435-5957.2006.00083.x
Saadat, S., Rawtani, D., and Hussain, C. M. (2020). Environmental perspective of COVID-19. Sci. Total Environ. 728, 138870. doi:10.1016/j.scitotenv.2020.138870
Shi, T., Qiao, Y., Zhou, Q., and Zhang, J. (2022). The regional differences and random convergence of urban resilience in China. Technol. Econ. Dev. Econ. 28, 979–1002. doi:10.3846/tede.2022.16721
Song, G., Zhong, S., and Song, L. (2022). Spatial pattern evolution characteristics and influencing factors in county economic resilience in China. Sustainability 14, 8703. doi:10.3390/su14148703
Tan, J., Hu, X., Hassink, R., and Ni, J. (2020). Industrial structure or agency: what affects regional economic resilience? Evidence from resource-based cities in China. Cities 106, 102906. doi:10.1016/j.cities.2020.102906
Tan, J., Zhang, P., Lo, K., Li, J., and Liu, S. (2017). Conceptualizing and measuring economic resilience of resource-based cities: case study of Northeast China. Chin. Geogr. Sci. 27, 471–481. doi:10.1007/s11769-017-0878-6
Wang, X., and Li, M. (2022). Determinants of regional economic resilience to economic crisis: evidence from Chinese economies. Sustainability 14, 809. doi:10.3390/su14020809
Wang, X., Zhu, Y., Ren, X., and Gozgor, G. (2023). The impact of digital inclusive finance on the spatial convergence of the green total factor productivity in the Chinese cities. Appl. Econ. 55, 4871–4889. doi:10.1080/00036846.2022.2131721
Wei, P., Qi, Y., Ren, X., and Gozgor, G. (2023). The role of the COVID-19 pandemic in time-frequency connectedness between oil market shocks and green bond markets: evidence from the wavelet-based quantile approaches. Energy Econ. 121, 106657. doi:10.1016/j.eneco.2023.106657
Yuan, H., Guan, Z., Jiang, C., and Yang, H. (2023). Peer effect in the construction of China’s high-speed rail stations: empirical evidence from spatial econometric analysis. Transp. Res. Part A Policy Pract. 173, 103720. doi:10.1016/j.tra.2023.103720
Zhou, Q., and Qi, Z. (2023). Urban economic resilience and human capital: an exploration of heterogeneity and mechanism in the context of spatial population mobility. Sustain. Cities Soc. 99, 104983. doi:10.1016/j.scs.2023.104983
Keywords: urban economic resilience, spatial convergence, environmental awareness, China, foreign investment (inward and outward FDI)
Citation: Li Y and Xiao Y (2023) How environmental awareness affects the spatial convergence of urban economic resilience: evidence from China. Front. Environ. Sci. 11:1326701. doi: 10.3389/fenvs.2023.1326701
Received: 26 October 2023; Accepted: 27 November 2023;
Published: 11 December 2023.
Edited by:
Yukun Shi, University of Glasgow, United KingdomReviewed by:
Xianming Sun, Zhongnan University of Economics and Law, ChinaYiying Li, Renmin University of China, China
Copyright © 2023 Li and Xiao. This is an open-access article distributed under the terms of the Creative Commons Attribution License (CC BY). The use, distribution or reproduction in other forums is permitted, provided the original author(s) and the copyright owner(s) are credited and that the original publication in this journal is cited, in accordance with accepted academic practice. No use, distribution or reproduction is permitted which does not comply with these terms.
*Correspondence: Ya Xiao, 2495806840@qq.com