- 1Graduate School, Baekseok University, Seoul, Republic of Korea
- 2School of Architecture, Southeast University, Nanjing, China
- 3China Village Culture Research Center, Central South University, Changsha, China
- 4School of Urban Construction, Yangtze University, Jingzhou, China
Quantitatively measuring rural digitalization performance and development sustainability, identifying their key influencing factors and figuring out their driving mechanisms are of great value to policy design for rural revitalization and management. This paper analyzed the sustainable development degree, spatial patterns, and influencing factors of rural digitization in Hunan Province, China, based on a combination of PSR, TOPSIS, ESDA, GWR and GeoDetector, in an attempt to provide a basis for the planning and policy design of rural management. The sustainability and construction performance of rural digitalization in Hunan were characterized by significant spatial inequality and positive autocorrelation, with coefficients of variation of 0.33 and 0.24, and Moran’s I values of 0.29 and 0.34, respectively. The rural digitalization in Hunan showed significant non-equilibrium across different dimensions and brought forward diversified combination patterns, including single dimensional leadership, dual dimensional leadership, three-dimensional leadership, and all-round development. The pattern dual dimensional leadership, especially PS (pressure + state), was dominant in the sustainability of rural digitalization, compared to the pattern single dimensional leadership dominant in the construction performance, especially I (rural infra-structure digitalization), IL (rural infrastructure + life digitalization), IG (rural infrastructure + governance digitalization). The sustainability and construction performance of rural digitalization in Hunan were subject to a complex driving mechanism, with different factors differing significantly in their action nature, force, spatial effects and interactions. Notably, economic development (gross domestic product) is a positive key factor, while government intervention capacity (fiscal self-sufficiency rate) is an important factor, and natural environment (relief amplitude) is a mixed auxiliary factor (both positive and negative). Factor interactions were mainly characterized by nonlinear enhancement and a large number of super factor pairs. Therefore, the policy design should take into account both localized and differentiated management; and also emphasize enhanced cooperation with adjacent counties and synergistic management. It is suggested to divide Hunan into four planning zonings of leading, potential, warning and general zone, and design the spatial policies for each of them according to the driving mechanism, so as to develop a more reasonable and practical combination of development projects and management policies.
1 Introduction
1.1 Background
With the integration, development and innovative application of cloud computing, big data, artificial intelligence and other technologies and services, a new round of scientific and technological revolution and urban and rural development are rapidly taking shape and rising. In the context of rapid digital development around the world, the accelerated extension of information technology to the countryside, represented by 5G and 6G, has contributed to the fact that the center of gravity of digital development is gradually shifting from smart cities to digital villages (Ferrari, Wang, 2022). The application of digital and information technology to promote the transformation of villages and realize sustainable and high-quality development of villages has become a new development trend, with the goal of creating digital villages, smart villages, intelligent villages, and villages 4.0 (Malik, 2022). Rural digitalization is a process of continuous integration of digital technology with rural productivity, production relations and production factors. Its development helps to overcome the limitations of traditional rural “space-time, resources and talents,” and promote the comprehensive upgrading of agriculture, the comprehensive progress of rural areas and the comprehensive development of farmers (Naldi, 2015; Li et al., 2023). Due to the outbreak of COVID-19, more countries around the world have embarked on the project to formulate rural digitalization and smart village development policies (including spatial planning, action plans, construction guidelines and standards), and some pioneering nations such as the United States, China, Russia, the European Union, and Australia are ready to optimize and update their policies on the digitalization of villages. Therefore, scientifically and quantitatively measuring the rural digitalization sustainability and revealing its driving mechanisms will provide a basis and obvious value for village management and revitalization.
In the context of smart earth, digital nation and smart city construction, digitalization is rising as a key variable affecting the sustainable development of rural areas, and an important foundation and prerequisite for villages to be smart. Countries around the globe have currently set off a boom in rural digitization, constantly innovating new modes, formats, technologies and products for the development of digital and smart villages. It is a remarkable fact that governments’ management and planning of the development of the emerging rural digitization is generally not well founded, leading to overlapping projects, overbuilding, coexistence of underinvestment and waste of resources, and failure of pilots and experiments in the construction of digital and smart villages, which are obstacles and threats to the high-quality development of rural digitization. To accelerate and better promote rural digitalization, there is an urgent need to carry out rural digital sustainability assessment and reveal the driving mechanism behind it, so as to provide a basis for the government’s decision-making on the construction of digital and smart village projects, as well as the policy and spatial planning for rural digitalization. This paper conducted an empirical study of Hunan, China on three areas. First, it quantitatively measured the sustainability and construction performance of rural digitalization in Hunan, based on the PSR model and TOPSIS model. Second, it analyzed the spatial characteristics of rural digitalization in Hunan, including spatial patterns and spatial effects, using ESDA and GIS models. Third, it analyzed the impact of different factors on rural digitalization, including the nature of the role, intensity, spatial variation characteristics and factor interaction effects, using the GWR model and GeoDetector. Notably, the sustainability evaluation of rural digitalization is an improvement of the analysis of its construction performance, and in order to reveal the similarities and differences between the two, a comparative analysis of their spatial characteristics and influencing factors was carried out during the study.
The important contribution of this study to rural revitalization and management is the shift from rural digitalization construction performance to development sustainability and the revealing of their driving mechanisms. Rural digitization construction performance and development sustainability, two different concepts, share some common points including large regional differences in rural digitization, significant spatial effects, low overall level, diversified modes of dimensional combinations, and high complexity of driving mechanisms. However, rural digitalization sustainability and construction performance significantly vary in evaluation results, dimension combinations, and driving mechanisms. The analytical framework, research methodology, findings and conclusions of this paper are applicable to the construction of digital villages and smart villages in Hunan, and also have inspirational value and implications for planning and policy making in similar regions in China and the world. In addition, this study is of great value to the construction of digital planning and rural planning disciplines, as well as to the development of digital and smart village theories. From the perspective of sustainable development of the spatial planning discipline, rural planning is a secondary discipline alongside urban planning and regional planning. Digitalization and intelligence, the latest trends in rural development, are driving changes in rural planning, and digital planning is transforming into a new direction for rural planning. This paper tries to summarize the regular features and the hidden order behind rural digitalization based on the case study of digital village construction in Hunan, helping to reshape the value of the industry, reconfigure the connotation of the discipline, fill the theoretical gap, to innovate the planning methodology and to upgrade the planning tools through rural planning and digital planning. According to their whole-life development trend, the construction of digital and smart villages is still in the ascendant and countries all over the world are in the exploratory stage. As a result, it is urgent for government decision makers to identify the actual conditions, ideal ways, and implementation path of rural digitalization. The case study on rural digitalization in Hunan will promote the research in this regard to be practical (what is the current actual situation), actual (what should be the theoretical or ideal situation) and made (how can we promote the integration of the current real situation towards the theoretical/ideal situation), which is of great significance for the construction and refinement of the theoretical system of digital and smart villages.
1.2 Literature review
Current research on rural digitalization focuses on the following three areas:
First, regarding digitalization as a revolutionary tool to lead the high-quality development of the countryside, the research analyzes the penetration and application of digital and information technology in multiple fields such as economy, society, culture and life in the countryside, committed to exploring the impacts of different technologies on the sustainable development of the countryside as well as the specific application schemes or processes (Budziewicz-Guzlecka, 2022). Scholars mainly discuss the impact of emerging technologies such as the Internet of Things, Big Data, and Artificial Intelligence on farmers’ lives, agriculture, and rural development processes, and propose application scenarios, programs, and pathways based on case studies and the characteristics of the study area (Murty, 2020; Ram, 2021). Currently, a large number of studies are on the digitization of tourism-based (Ciolac, 2022; Rodrigues, 2023), climate change-responsive (Ho, 2014; Galiwango, 2022), and historical and cultural preservation-based (Huang, 2020; Qi, 2022) villages because the market and the government provide sufficient capital support for their digitization and smartening. Some scholars also attribute the research related to rural e-commerce and smart characteristic towns to the field of rural digitalization (Leong, 2016; Li, 2022).
Second, the research on the characteristics, evolution mode and influencing factors of rural digitalization provides the basis for the planning and policy design of digital village, smart village and village 4.0. Most papers in this field mainly discuss national scale rural digitalization planning, policies, experiences, lessons, opportunities, and barriers in the paradigm of case studies and empirical research (Fennell, 2018; Alabdali, 2023). For example, scholars from different countries, based on case studies or local development actuality, have analyzed the practice and progress of rural digitization in pioneer or leading countries, including the United States (Li, 2020), the European Union (Adesipo, 2020), Australia (Park, 2017; Randell-Moon, 2022), Slovenia (Zavratnik, 2018), Canada (Spicer, 2018), Ireland (McGuire, 2022), Poland (Adamowicz, Wojcik, 2021), Spain (Escalona-Orcao, 2016), and China (Li, 2022). A few scholars have analyzed the driving mechanism of rural digitization and its evolution, investigating the influence and nature of the role of different factors from economic, social, environmental and other dimensions. For example, Cao (2023) measured the rural digitalization in China and its driving mechanism at the national level based on entropy method and Tobit model; Zhang (2023a) analyzed the spatial differences of digital village construction and its influencing factors in Gansu of China from at the regional level using Geographic Information System (GIS) and GeoDetector.
Third, the research focuses on the relationship between rural digitization and agricultural modernization, farmers’ income growth, and regional economic development, trying to find ways to promote their common development. In the practice of national and local governments, rural digitalization is commonly implemented together with other development strategies, such as agricultural modernization, industrialization, common prosperity, domestic demand upgrading, innovation and entrepreneurship (Mei, 2022). Therefore, the inevitable interaction between rural digitization and these development strategies has led scholars to realize that the study of rural digitalization cannot be confined to the “village.” In recent years, a small number of scholars have begun to try relevant exploratory studies, including the coupling relationship between rural digitalization and high-quality development of agriculture (Wang, 2023), the synergistic relationship between rural digitalization and farmers’ income (Cai, 2023), and the interactive relationship between rural digitalization and financial technology (Zhang, 2023b).
A review of published papers shows that rural digitalization research is increasingly emphasized by scholars and governments, and it is continuing to expand in breadth and depth (Zhao, 2022a; Rahoveanu, 2022). It is worth noting that, alongside the considerable progress and achievements, there are also clear gaps in existing research, mainly in the following two areas. First, scholars have taken an emphasis on rural digitalization construction performance (RDCP) and its driving mechanisms, with less attention paid to the evaluation of the rural digitalization sustainability and its influencing factors. Most scholars, only regarding digitalization as a key way to sustainable development in the countryside (Putri, Fernandez, 2023) in their study, are still in the conceptual discussion stage in the evaluation and management of rural digitalization sustainable development research (Agusta, 2023), with many blind spots remaining in areas such as technical route, methodological model and indicator system. In addition, most of the studies are limited to internal performance evaluation, neglecting the analysis of the external environmental pressure and multi-body active behavior faced by rural digitalization, resulting in incomplete and inaccurate conclusions. Second, the existing research has not paid enough attention to the spatial effect of rural digitization. Different regions and countries have different starting times for rural digitalization, construction paths, development models, planning objectives, management policies, government and resident attitudes and their participation, leading to large differences in development between regions (Maja, 2020). Some scholars have also found that there is a certain spatial correlation in rural digitalization, with significant mutual influence between neighboring regions. However, the existing research is mainly based on linear regression models and GeoDetector. The former directly ignores the spatial effects, while the latter only responds to the spatial effects as a whole without analyzing the local spatial effects, which hinders the accuracy of the results (Li et al., 2023).
2 Materials and methods
2.1 Study area: Hunan province in China
Hunan is a provincial-level administrative region located in southern China, with Changsha as its capital. It is geographically bordered by Jiangxi, Guizhou, Guangdong, Guangxi, Hubei, and Chongqing (a municipality directly under the central government). With jurisdiction over 13 prefecture-level cities and 1 minority autonomous prefecture, it has 122 county-level administrative districts. The study area of this paper is 105 county-level administrative districts, which is highly representative with coverage of more than 86% of the province (Figure 1). It should be noted that Furong, Tianxin, Yuelu, Kaifu, Yuhua, Hetang, Lusong, Shifeng, Tianyuan, Yuhu, Yuetang, Shigu, Zhengxiang, Yueyanglou, Yunxi, Wuling, Hecheng, Hongjiang and Datonghu were excluded mainly due to the fact that they are all municipal districts of prefecture-level cities as the core areas of urban construction, with a small rural population and small-scale agricultural development.
2.2 Research methods
The establishment of index system and the selection of appropriate measurement model are the preconditions for empirical research. The selected indicator system contains principal component analysis, factor analysis and PSR models. The PSR model was selected in this paper due to the systemic nature of rural digitalization and the causality between different subsystems. For performance evaluation and sustainability assessment, approaches such as Hierarchical Analysis, Technique for Order Preference by Similarity to Ideal Solution (TOPSIS) and VlseKriterijumska Optimizacija I Kompromisno Resenje (VIKOR) can be used, and in this study TOPSIS was used to ensure the objective results of the calculations and to balance the actual conditions of the optimal and the worst cities. In the comparative analysis of multiple cities, the spatial effect is a potential influencing factor that should not be ignored. Therefore, we chose Exploratory Spatial Data Analysis (ESDA) to detect spatial heterogeneity and autocorrelation in this paper, and decided whether to choose a spatial regression model based on the results. Least squares linear regression was chosen when the spatial effect was absent or very weak; otherwise Geographically Weighted Regression (GWR) and GeoDetector were chosen to calculate the local regression equations and the interactions of the different factors to enhance the accuracy of the regression analysis results.
2.2.1 PSR and TOPSIS model
The PSR model is a causal chain composed of pressure, state, and response together, which originated from environmental science and is now widely used in sustainable development research. We evaluated rural digitalization sustainability by TOPSIS, which was created by C.L. Hwang and K. Yoon in 1981 as a method for ranking a limited number of evaluation objects based on their proximity to an idealized target (Li, 2023a). To analyze rural digitization using the PSR model requires a systems-theoretic perspective that captures the key features of digital and smart villages’ construction status, the pressures they face, and their response, as well as rationalizing the logical relationships among the three. As for development pressure, the population demand is a key environmental factor influencing rural digitalization, including population size and structure (Lofving, 2021). The size consists of the resident population and the lost population, and the structure includes both age and education structures. The elderly is generally lower than the young in acceptance and adoption of digital products and services, so the impact of population aging is more important in the age structure (Arroyo-Menendez, 2022). Most digital products and services involve some emerging technology, and illiteracy is a key indicator affecting the matching between rural digitalization and population knowledge structure (Sun, 2023). Rural digitization is a core element of new urbanization construction and holds the same position as smart cities, so the impact of urbanization cannot be underestimated. Population urbanization is used to represent it in this study (Ren, 2023). Given that rural digitization is closely related to the regional industrialization, it is represented by GDP per capita (Chinn, 2007). Population loss, population aging and illiteracy are negative indicators, based on the data from the Hunan Provincial Statistical Yearbook and the Seventh Population Census. Rural digitalization is a typical complex adaptive system, and it can be further divided into many subsystems based on the state of construction, such as rural infrastructure digitization, rural economy (industry) digitization, rural life digitization, and rural governance digitization. The rural digitalization index represents the construction level of digital villages, with the data based on the research report published by Peking University (Chen et al., 2022; Liu et al., 2022). It is necessary to consider the act of the government, residents, professionals and technicians in an integrated manner in the response of interest subjects. The government act is mainly expressed in terms of both investment and policy. The government is currently investing heavily in digital infrastructure, using fixed asset investment to represent it (Hou, 2023). Policies include generic and pilot policies, and this paper represents the differences in support by different levels of pilots (Stojanova, 2021). The act of the population is mainly manifested as consumption, and represented by per capita consumption (Zhang, 2023a) (Table 1).
Attaching great importance to the rural digitization, Hunan has issued special development policies such as the Action Plan for the Development of Digital Countryside in Hunan Province (2023–2025), the Pilot Work Program for Digital Countryside in Hunan Province. The rural digitalization in Hunan is promoted in a hierarchical pattern of national pilot - provincial pilot—non-pilot, including both comprehensive and characteristic pilots, which coincide s with the development concept of the central government and is highly representative in the construction of digital villages in China. The four national pilots are Xiangxi Tujia and Miao Autonomous Prefecture, Daxiang in Shaoyang City, Shuangpai in Yongzhou City, and Shaoshan in Xiangtan City. According to the results of the final evaluation of the national digital village pilot (initiated by the Cyberspace Administration of China together with the Ministry of Agriculture and Rural Affairs, the National Development and Reform Commission, the Ministry of Industry and Information Technology, the Ministry of Science and Technology and other departments, and evaluated by invited experts), Huayuan in Xiangxi Tujia and Miao Autonomous Prefecture was rated as excellent. There are 40 provincial pilots, including 9 counties and cities as comprehensive rural digitalization pilots, 6 towns and villages as rural information infrastructure featured pilots, 6 towns and streets as digital agriculture featured pilots, and 19 towns and villages as rural digital governance featured pilots. In the quantitative analysis, national and provincial pilot and non-pilot projects were assigned values of 5, 3, and 1 respectively.
2.2.2 Exploratory spatial data analysis
ESDA is a classical method to detect spatial effects. In this paper, coefficient of variation (CV) and spatial cluster analysis diagram were introduced to measure spatial heterogeneity and characterize regional differences; Moran’s I index and cold and hotspot analysis chart were used to measure spatial correlation and characterize regional dependence. A larger coefficient of variation indicates a higher level of the regional difference in rural digitalization. In general, 0.16 and 0.36 are used as thresholds to classify them into low, medium and high levels. A quantile model was used in the spatial cluster analysis to visualize the geographic patterns of rural digitization. A larger absolute value of Moran’s I index indicates a higher level of rural digitalization, and it is classified into spatial positive autocorrelation and negative autocorrelation using zero as the threshold. The cold and hotspot analysis map visualizes the spatial correlation characteristics of rural digitization. The counties in the study area were classified into four types of hot, sub-hot, sub-cold, and coldspots according to the index Getis-Ord
where:
2.2.3 Geographically weighted regression and GeoDetector
In this study, 105 cities in Hunan were selected to carry out case studies, and spatial heterogeneity and autocorrelation need to be prioritized in the multi-city comparative analysis to provide a basis for regression model selection. In the case of weak spatial effects, the influencing factor analysis can be directly performed based on the traditional least squares linear regression model; otherwise, the spatial regression model should be used. GWR, GeoDetector, spatial lag model and spatial error model are the most commonly used spatial regression models. The first two are used for analyzing both cross-sectional and panel spatial data, while the latter two are only applicable to panel spatial data. GWR enables the calculation of local regression coefficients, and GeoDetector detects the interaction effects between different factors. It can be seen that they fit with the type of interface spatial data used in this study and match each other with the goal of the driving mechanism analysis (revealing the nature, intensity, and interaction of the factors) in the study, so that GWR and GeoDetector are appropriate research methods. The former was used to analyze the direct influence intensity and properties of a single factor, while the latter was used to measure the interaction between different factors. GWR explores the driving factors of rural digitalization sustainable development degree by establishing the local regression equation of each county in space. Since it takes into account the spatially localized effects of rural digitization, it offers a higher computational accuracy than a traditional linear regression model (by least squares). GeoDetector is able to measure the explanatory power of different factors for rural digitization by characterizing the similarity of the spatial patterns of the independent variable (
where,
China is still practicing growthism, and economic development is still the key indicator for county government assessment. The capacity for economic development is also a fundamental driver of rural digitization, so the impact of the scale and stage of economic development should be taken into account, which is represented by the GDP indicator chosen in this paper (Perez-Martinez, 2023). Rural digitization is currently at the stage of self-sufficiency driven by government investment, and in this paper the fiscal self-sufficiency rate is chosen to represent the government’s ability to intervene (Zhao, 2021c; Li, 2022). Education level determines digital literacy and urban earning ability, and the average length of education is chosen to represent their impact on rural digitalization and population urbanization (Cambra-Fierro, 2022; Li, 2023b). The government expects to reduce the urban-rural income gap through the Digital Village project, so as to achieve the goal of common prosperity and development (Li et al., 2023). Natural environmental factors, especially the complex of relief amplitude, have a significant impact on the sustainable development of rural digitization. Digitalization is a new way to break through the constraints and obstacles of inconvenient transportation (Zhu, 2023). The data of GDP, fishery self-sufficiency rate and urban-rural income ratio came from Hunan Provincial Statistical Yearbook, with the fiscal self-sufficiency rate calculated as the ratio of fiscal revenue to expenditure. The average length of education was derived from the census. The terrain undulation data is sourced from relief degree of land surface dataset of China (1 km) (You, 2018; Dang, 2022).
3 Results
3.1 Construction performance
3.1.1 Spatial pattern analysis
The spatial clustering results showed that the digitization of rural infrastructure in Hunan had obvious spatial hierarchical characteristics. The spatial pattern was characterized by the formation of aggregation areas with high values, banded aggregation areas with low values, and a random distribution of the median values. Most of the counties at low level of rural infrastructure digitalization were in the northeast and northwest corner of Hunan, including Jishou, Changsha and Wangcheng. Most of the counties at low level of rural infrastructure digitalization were in the central part of Hunan, concentrated distribution along the Huaihua-Shaoyang-Hengyang continuous line, including Changning, Yanling, Shuangfeng, Wulingyuan, Longhui, Beita and Mayang (Figure 2).
The results of spatial clustering showed that the rural economy digitalization in Hunan was prominently characterized by spatial hierarchization and agglomeration. Most of the counties at low level of rural economy digitalization were concentrated in the Yiyang-Changsha region, including Changsha, Dingcheng and Taojiang. Counties at low level of rural economic digitization have formed large clusters in Huaihua-Shaoyang, and two small clusters in Zhangjiajie and Yongzhou, with members including Jiahe, Xintian and Linxiang. The counties at the medium level are concentrated around the high values, including Shaodong, Pingjiang and Yuanling.
The results of spatial clustering showed that the rural governance digitalization in Hunan was prominently characterized by spatial hierarchization and clustering. The high-level digital counties for rural governance in Hunan were concentrated in the central and eastern parts of the province, and penetrated into the north, with members including Changsha, Wangcheng and Heshan. Most of the counties at low level of rural governance digitalization were concentrated in Huaihua and Chenzhou, including Yanfeng, Chenxi, Lengshuitan and Luxi. Those at medium or high level formed a central peripheral structure and they were concentrated in the Changde-Yiyang-Loudi region, including Xinhua, Wugang, Anxiang and Cili.
The spatial clustering results showed that no counties at high or medium level of rural life digitization in Hunan formed a cluster, except those at low level forming a horizontal “U” cluster belt along Yueyang-Changde-Huaihua-Shaoyang-Hengyang-Zhuzhou. The members of the agglomeration belt included Yizhang, Beihu, Linwu and Shimen. Counties with a high rural life digitalization index included Louxing, Xinhua and Heshan; and those with a low index included Chaling, Yongshun, Shuangpai and Jiahe.
3.1.2 Dimensional difference analysis
There is a significant difference in the development level of the four dimensions (rural infrastructure digitization, rural economy digitization, rural governance digitization, and rural life digitization) for rural digitization, with only Qidong, Heshan, Qiyang, Ningxiang, and Changsha having a coefficient of variation of less than 0.16, indicating negligible heterogeneity. In theory, the relationship between the four dimensions can form 15 combination patterns, including single dimensional leadership, dual dimensional leadership, three-dimensional leadership, and all-round development (Figure 3). The determination steps for the combination pattern are as follows: Firstly, calculate the sum of the four dimensions for each county in Hunan Province. Secondly, calculate the proportion of each dimension to represent their status. Finally, determine the combination pattern of the four dimensions based on their proportional relationship. The all-round development type requires that the proportion of each dimension be greater than 20%; The threshold for other types of classification is 25%.
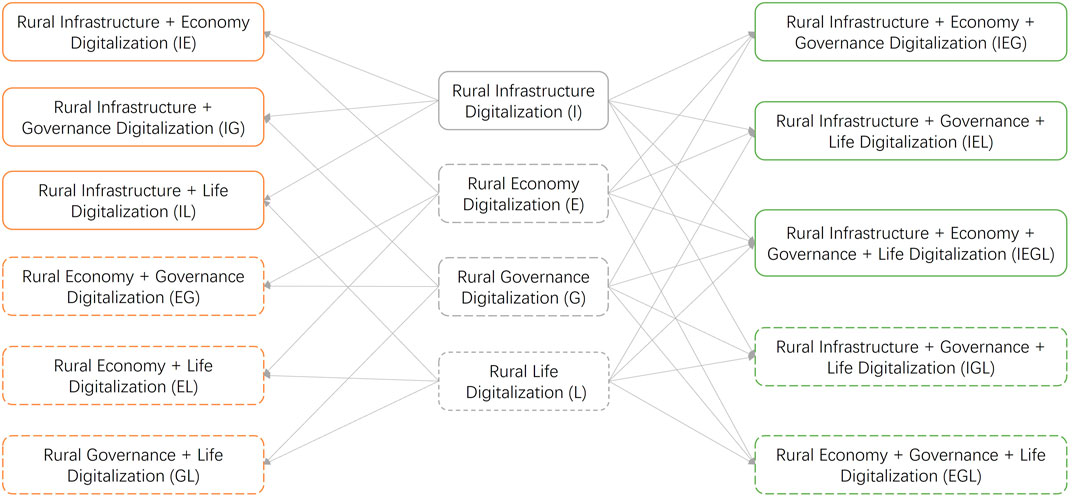
FIGURE 3. Combination patterns analysis of different dimensions in rural digitalization construction performance. Note: The dashed line represents that this pattern has not appeared in Hunan province.
Among the 15 combination patterns, 8 are not present in Hunan, including rural economy digitization (E), rural government digitization (G), rural life digitization (L), rural economy + government digitization (EG), rural economy + life digitization (EL), rural government + life digitization (GL), rural infrastructure + government + life digitization (IGL), Rural economy + governance + life digitization (EGL). Among the seven of combination patterns, the single dimensional leadership type represented by rural infrastructure digitization (I) holds a dominant position, accounting for 37.14%. It has the largest number of members, including Lukou, You, Chaling, Yanling and Xiangtan. In the dual dimensional leadership type, the proportion of rural infrastructure + life digitalization (IL) is the highest, reaching 20.95%, with members including Zhu, Nanyue, Leiyang and Shuangqing. The proportion of rural infrastructure + governance digitization (IG) reaches 17.14, with members including Wangcheng, Liling, Hengyang, Shaoyang, Yueyang, Pingjiang and Li. The proportion of rural infrastructure + economic digitization (IE) is 8.57%, and its members include Yanfeng, Hengdong, Hanshou, Yongding, Guiyang, Zixing, Jingzhou, Lianyuan, and Guangzhou. The number of members in the three-dimensional leading type is very small, only Hengnan, Huarong, and Taojiang belong to rural infrastructure + economy + governance digitization (IEG), while Junshan, Dingcheng, Linwu, and Yuanling belong to rural infrastructure + governance + life digitization (IEL). The proportion of all-round development type (IEGL) is 9.52%, with members including Changsha, Liuyang, Ningxiang, Hengshan, Qidong, Xinshao, Dongkou, Heshan, Anhui, and Shuangfeng (Figure 4).
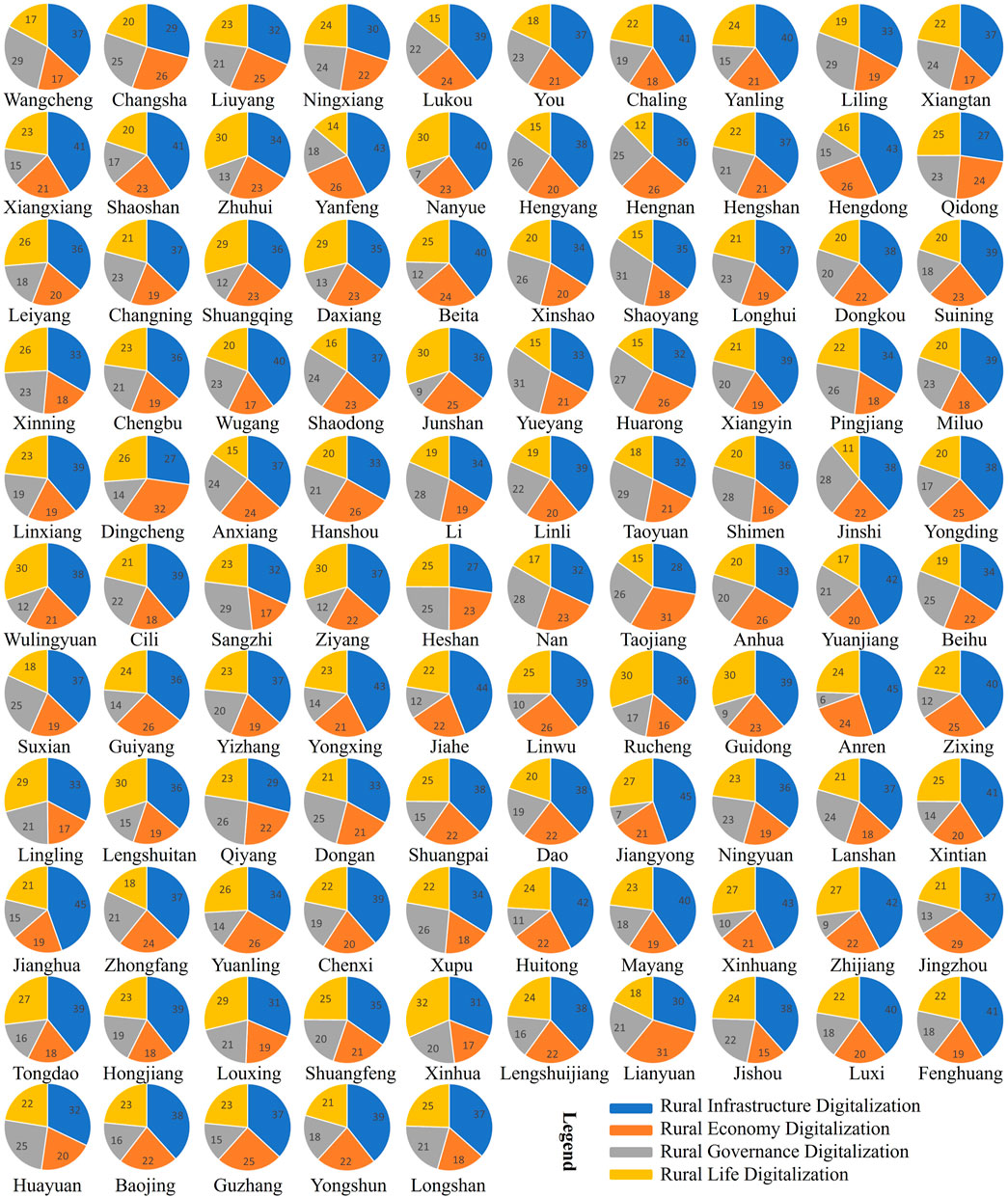
FIGURE 4. Construction performance of rural digitalization in Hunan province from different dimensions.
3.2 Sustainability evaluation
3.2.1 Spatial pattern analysis
The results of spatial clustering showed that the rural digitalization sustainable development in Hunan was prominently characterized by spatial hierarchization and clustering. Most of the counties with a high rural digitalization sustainable development total index were clustered in the eastern and central part of Hunan, especially the provincial capital metropolitan area, including Changsha, Wangcheng, Shaoshan and Daxiang. Most of the counties at low level of rural digitalization sustainable development were in the northwest and south part of province, especially in the mountainous areas where ethnic minorities live in the center of Xiangxi-Huaihua-Zhangjiajie, including Yongding, Changning and Taoyuan. Most counties at moderate level of rural digitalization sustainable were concentrated in the Huaihua-Shaoyang and Hengyang-Chenzhou clusters, including Jiangyong, Hongjiang, Sangzhi and Fenghuang (Figure 5).
The results of spatial clustering showed that the rural digitalization pressure in Hunan was prominently characterized by spatial hierarchization and clustering. Counties with a high rural digitalization pressure index formed two clusters - the eastern provincial capital finger cluster and the southern Chenzhou Hengyang cluster, including Changsha, Liuyang and Wangcheng. Most of the counties at low level of rural digitalization pressure were in the banded agglomeration zone in the west of Hunan, including Xintian, Lukou and Anren. Most counties at moderate level of rural digitalization pressure were concentrated in the central belt agglomeration area, and penetrated northward to Yueyang and Zhuzhou, including Pingjiang, You and Longhui.
Most high-value members were clustered and concentrated around the capital of Hunan, including Changsha, Liuyang, Dingcheng and Ningxiang. They were concentrated in Changsha-Yiyang-Loudi and extended to Zhangjiajie, Changde, Yueyang, and Hengyang. Most low-value members were concentrated in the Huaihua-Shaoyang-Yongzhou-Chenzhou-Zhuzhou continuous belt, including Shaoyang, Yueyang and Jinshi. Medium-value members were in random distribution, including Huarong, Dongkou and Xiangyin.
Two high-value large agglomeration areas appeared in the eastern and western parts of Hunan, centered on the provincial capital and Huaihua-Shaoyang, with members including Changsha, Daxiang and Shaoshan. The median-value forms two clusters in the southern and northern parts of Hunan, centered on Hengyang and Changde, with members including Jiangyong, Louxing and Liling. The clustering areas of low value members are located in the northwest, southeast, and southwest corners of Hunan, with members including Linxiang, Linli and Beita.
3.2.2 Dimensional difference analysis
The development level of the three dimensions (pressure, state, and response) for evaluating the sustainability of rural digitalization also varied greatly, with only Shimen, Pingjiang, Dongkou, Li, Jiangyong, Jishou, Jianghua, Fenghuang, Heshan, Anhui, Hongjiang, Wangcheng, and Longhui having a coefficient of variation of less than 0.16, indicating negligible heterogeneity. About 90% of members had a moderate to high level of heterogeneity, with significant differences in the three dimensions. The relationship between the three dimensions was in seven combination patterns, including single dimensional leadership, dual dimensional leadership, and all-round development (Figure 6). The steps for determining the combination model were the same as for rural digitization performance, but the thresholds were determined differently. The all-round development required that the proportion of each dimension was greater than 30%; the threshold for other types was 33%.
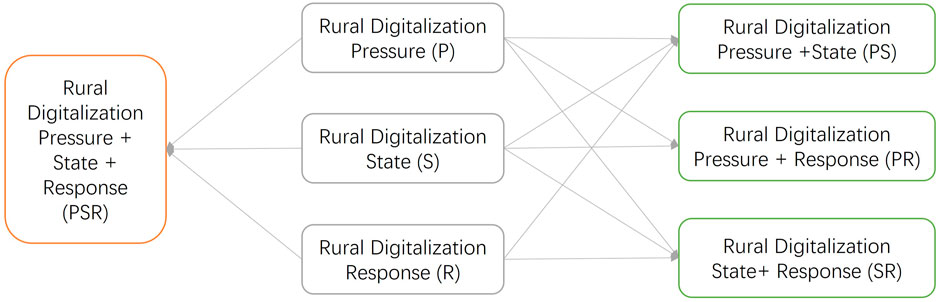
FIGURE 6. Combination patterns analysis of different dimensions in rural digitalization sustainability.
About 25% of members were in the single dimensional leadership type, with 17.14% in the rural digitization pressure (P) type, including Yanling, Liling, Yanfeng, Nanyue, Hengyang, Leiyang, Changning, Shaodong, Yueyang, Linxiang, Jinshi, Yuanjiang, Suxian, Guiyang, Yizhang, Yongxing, Anren and Dao. There were fewer members in the rural digitization state (S) and rural digitization response (R), with only Changsha and Dingcheng for the former and Shaoshan, Daxiang, Li, Shuangpai, Hongjiang, Huayuan for the latter. In the dual dimensional leadership model, the number of rural digitalization pressure + state (PS) was the highest, accounting for over 50%, including Liuyang, Ningxiang, Lukou, Xiangtan, Zhuhui, Hengnan, Hengshan, Qidong, Shuangqing, Beita, Xinshao, Shaoyang, Wuang, Junshan, Huarong, Xiangyin, Anxiang, Hanshou, Linli, Taoyuan, Yongding, Wulingyuan, Cili, Nan. Taojiang, Beihu, Linwu, Rucheng, Guidong, Lingling, Lengshuitan, Qiyang, Dongan, Ningyuan, Lanshan, Xintian, Zhongfang, Yuanling, Chenxi, Xupu, Mayang, Xinhuang, Jingzhou, Tongdao, Louxing, Shuangfeng, Xinhua, Lengshuijiang, Luxi, Baojing, Guangxi, Yongshun, Longshan. Wangcheng, You, Chaling, Xiangxiang, Hengdong, Longhui, Suining, Xinning, Chengbu, Miluo, Ziyang, Jiahe, Zixing, Jianghua, Huitong, Zhijiang belong to the rural digitization pressure + response (PR), while the members of the rural digitization state + response (SR) were the least, with only Shimen, Sangzhi, and Fenghuang. Dongkou, Pingjiang, Heshan, Anhui, Jiangyong, Lianyuan, Jishou in all-round development type, and the pressure, state, and response index of rural digitalization sustainability were balanced (Figure 7).
3.3 Impact factors
3.3.1 Overall effect analysis of factors
For the rural digitalization sustainable development index, the coefficient of variation and Moran’s I were 0.33 and 0.29 (Z = 5.02, p < 1%), respectively, indicating moderate spatial heterogeneity and high positive spatial autocorrelation in rural digitalization sustainability. Hotspots were concentrated in the provincial capital metropolitan area, with members including Liuyang, Pingjiang, Miluo, Ningxiang, Taojiang Xiangxiang and Shaoshan. While coldspots were concentrated in the southwest of Hunan, including Longshan, Yongshun, Yuanling, Guzhang, Lingxi and Chenxi (Figure 8). For the rural digitization state index, the coefficient of variation and Moran’s I were 0.24 and 0.34, respectively (Z = 5.61, p < 1%), indicating moderate spatial heterogeneity and high positive spatial autocorrelation in rural digitization construction performance. Hotspots were also concentrated in the provincial capital metropolitan area, with members including Longshan, Yongshun, Yuanling, Hanshou, Guzhang, Lingxi and Chenxi. While coldspots formed three clusters in the western, southeastern, and southwestern corners of Hunan. The first cluster included members such as Yongshun, Yuanling, Guzhang, Chengbu, Xinning and Suining; the second cluster included Jiangyong, Daoxian, Shuangpai, Ningyuan, Lanshan, Xintian and Jiahe; and the third cluster included members such as Chaling, Yanling, Guidong, Zixing, Yongxing and Anren. Overall, the rural digitization in Hunan showed significant spatial effects, and it is necessary to analyze its driving mechanisms using spatial econometric models.
The nature of the factor’s effect can be determined based on the maximum and minimum values of the regression coefficients. Gross domestic product, fiscal self-sufficiency rate, and average length of education play a positive role in the rural digitalization sustainability index; urban-rural income ratio plays a negative role, and relief amplitude is both positive and negative. Gross domestic product and average length of education play a positive role in the rural digitalization state index; fiscal self-sufficiency rate plays a negative role, and urban-rural income ratio and relief amplitude are both positive and negative. The strength of the direct influence of the factor can be determined from the average of the regression coefficients. Gross domestic product has a much higher direct influence on the rural digitalization sustainable development index than the other factors, playing a key role, while fiscal self-sufficiency rate, average length of education and fiscal self-sufficiency rate are important factors, and urban-rural income ratio and relief amplitude have a weak influence and play more of an auxiliary role. For the rural digitalization state index, gross domestic product is a key factor, fiscal self-sufficiency rate, average length of education and urban-rural income ratio are importance factors, and relief amplitude is an auxiliary factor (Table 3).
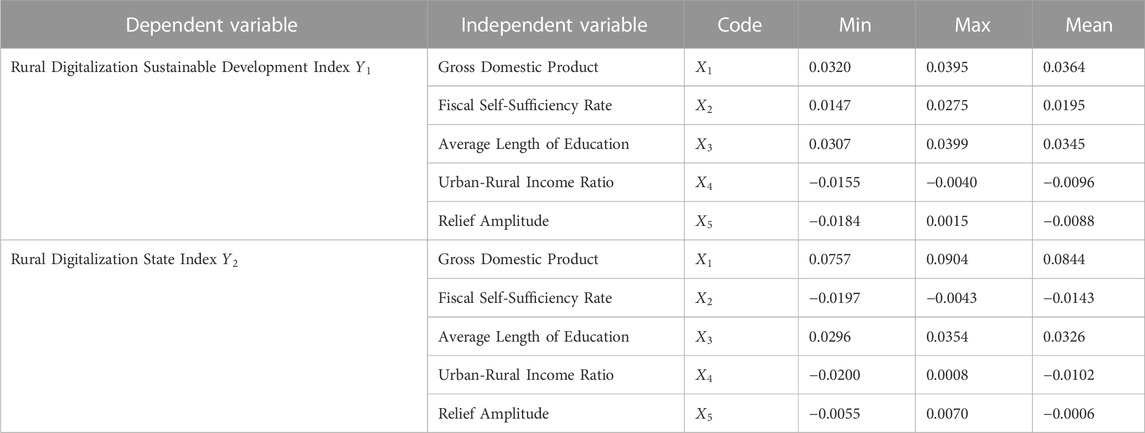
TABLE 3. Descriptive statistical analysis of geographically weighted regression results in hunan province.
3.3.2 Spatial effect analysis of factors
Economic development is a positive driver of rural digitization in Hunan, including sustainability and performance, and is characterized by gradient changes. For the rural digitization sustainable development index, the influence of gross domestic products decreases from south to north. It has the strongest influence on Changde, Yueyang, Yiyang, and Zhangjiajie regions, and the weakest influence on Yongzhou and Chenzhou. For the rural digitization state index, the influence of gross domestic products increases from west to east. It has the weakest influence on the marginal areas of Huaihua, Shaoyang, and Yongzhou, and the strongest influence on the regions of Yueyang, Changsha, Xiangtan, and Zhuzhou (Figure 9).
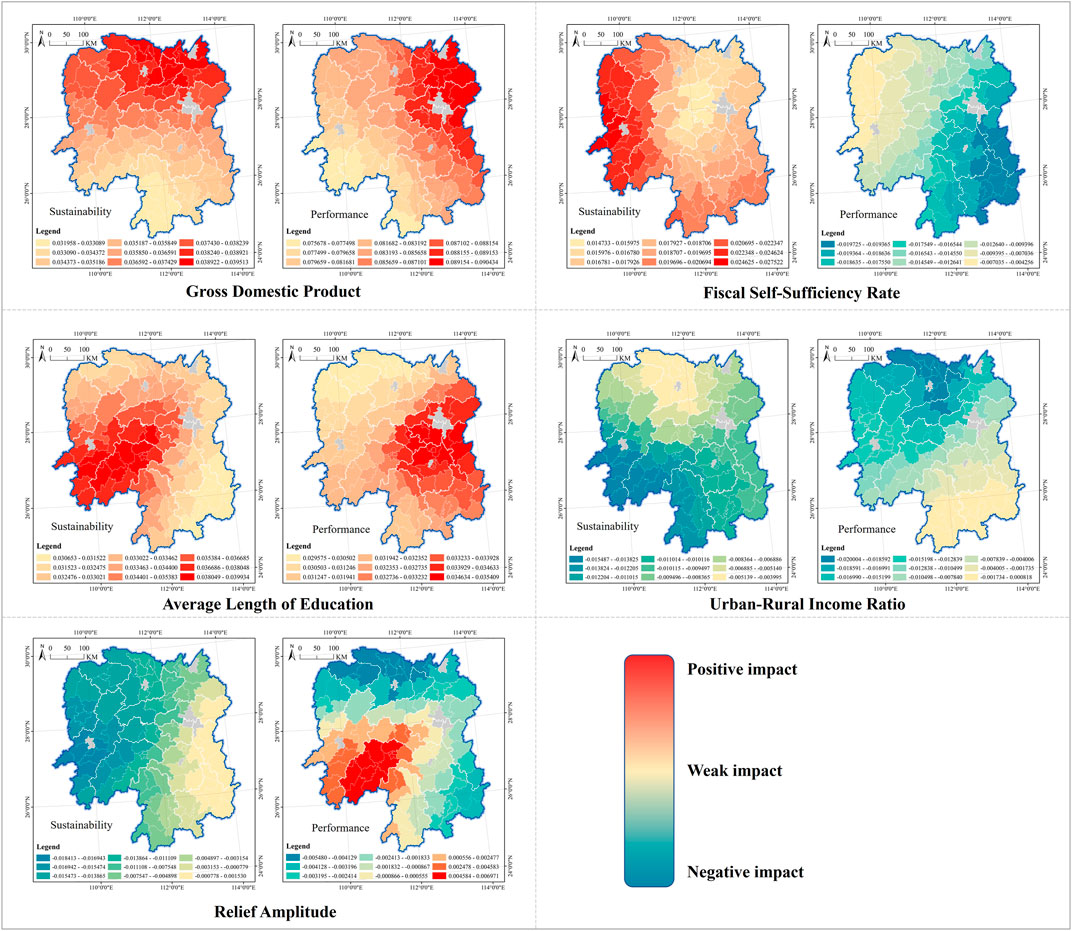
FIGURE 9. Geographical weighted regression results of impact factors on the performance and sustainability of rural digitization in Hunan province.
The impact of government intervention capacity on the sustainability of rural digitalization in Hunan is in a hierarchical ring structure, and the impact on performance is characterized by gradient changes. The fiscal self-sufficiency rate has a strong influence on the rural digitization sustainable development index in the peripheral areas, especially in the western regions of Xiangxi and Huaihua. The influence on the central regions is weak, especially in Loudi and Yiyang. The influence of the fiscal self-sufficiency rate on the rural digitization state index is gradually increasing from northwest to southwest, with Xiangxi and Zhangjiajie being depressions, while Chenzhou and Zhuzhou being highlands. It is worth noting that the impact of government intervention capacity on the sustainability and performance of rural digitization development is opposite in nature, with the former being positive and the latter being negative.
The matching of residents’ knowledge structure has a gradient effect on rural digitalization, and the spatial pattern of sustainability and performance is symmetrical. The average length of education has the highest impact on the rural digitization sustainable development index in Shaoyang and Huaihua, and the lowest impact on Chenzhou and Zhuzhou. It has the highest influence on the rural digitization state index in Changsha, Xiangtan, Hengyang, and Zhuzhou, and the lowest influence on the Xiangxi and Zhangjiajie. The highlands of its impact on the sustainability and performance of rural digitalization are located in the west and east, and the depressions are located in the southeast and northwest corners of the province, respectively.
The common specialty social policy has gradient spatial characteristics for rural digitalization, and there are significant differences in the geographical patterns of sustainability and performance. The influence of urban income ratio on rural digitization sustainable development index is concentrated in the southwest corner, including Huaihua, Shaoyang, and Yongzhou. The depressions are concentrated in the north, including Changde and Zhangjiajie. The highland of urban income ratio’s influence on the rural digitalization state index is located in Changde, while depressions are concentrated in Chenzhou and Yongzhou. It is worth noting that highlands have a negative impact, while depressions shift from negative to positive.
The natural environment has a very complex impact on rural digital development, in terms of sustainability and performance, both in positive and negative directions, with quite different geographic patterns of driving forces. The impact of relief amplitude on the rural digitization sustainable development index shows a spatial feature of gradient changes from west to east. Its highlands are located in the western part of the province, especially in Huaihua and Xiangxi, playing a negative restraining role. Its depressions are in the eastern part, including Zhuzhou, Chenzhou, and Changsha, with the force changing from negative to positive. The impact of relief sampling on the rural digitization state index is characterized by clustering, forming three clusters (in embryonic form only) in the northern, southwestern, and southeastern parts of Hunan. The highlands of positive influence are located in Shaoyang, while the depressions of negative influence are mostly in Changde and Zhangjiajie.
3.3.3 Interaction effect analysis of factors
Different factors had significant synergy effect rather than antagonistic effect when they worked together, but there was variability in different aspects. For the rural digitization sustainable development index, all interaction relationships between factor pairs are nonlinearly enhanced. For the rural digitization state index, except for the gross domestic product (
4 Discussion
4.1 Moving from performance to sustainability evaluation
4.1.1 Commonality
First, whether for rural digitalization sustainability or construction performance, the development gap in different regions should be emphasized, and the geographical distribution is characterized by significant spatial heterogeneity and correlation. It should be a general rule, supported by similar conclusions reached by other scholars in their study. For example, a similar phenomenon is found in provinces such as Guangxi (Li, 2023c) and Gansu (Zhang, 2023c), and also found at macroscopic provincial scales (Hao, 2022; Zhang, 2023a), regional scales (Yellow River Basin) (Ren, 2022), and national scales (Billon, 2010; Zhu, 2022).
Second, rural digitalization is at a low level in both sustainability and construction performance, and the large heterogeneity across different dimensions contributes to a diversified combination pattern. Most scholars now agree that rural digitization performance and development sustainability levels need to be improved, but there is less attention paid to the research on its systemic complexity, making it difficult to put forward appropriate policy recommendations. The construction of digital villages is a systematic project that involves a number of subsystems, such as the construction of new digital infrastructures, agricultural production, farmers’ lives and spatial governance. The timing and difficulty of building the different subsystems varies greatly, and few regions are currently able to synchronize the development of all subsystems. The asynchrony of construction in different dimensions creates great constraints on the rural digitalization sustainability. Most regions need to identify the development status of different dimensions to choose the model that suits their development capacity and conditions from the 16 combinations. In addition, the behavior of interest subjects and regional development environment have a great impact on the rural digitalization sustainable development, and a complex adaptive system with causal interaction relationship has come into being among pressure, state and response. Management policy design requires identifying the PSR portfolio model for each region and developing targeted measures and projects in accordance with the driving mechanisms.
4.1.2 Difference
First, the performance of rural digital construction is inconsistent with the evaluation results of development sustainability. For example, Liuyang, Changsha, Linxiang, Nan, Yuanjiang, Lengshuitan, Zhijiang have good construction performance against low development sustainability. The inconsistency is the result of the influence of national and provincial government policies, such as the “face-saving projects” of local governments during rural digitization. Especially in the construction of new digital infrastructure, the government focuses a large amount of funds on short-term blitz construction, while less investment in later utilization and maintenance leads to a mismatch between the supply of digital facilities and the demand of residents, and a low application rate. For example, the intelligent large screen and the rural digitalization one map system have become the “standard configurations” of most digital villages, especially the national and provincial pilots. However, despite the large scale of investment, they have become “instagrammable spots” for leaders and experts in their visit instead of locations with much value for many villages, resulting in investment waste. In addition, Pingjiang, Dongan, Anren, Fenghuang, Xinhuang, Xupu, Yongxing, Shuangqing, Hengshan, Xiangtan, Lukou, and You have low construction performance but high levels of development sustainability. Inconsistency may be due to the fact that they have found a construction model that works for them. Although not in a leading position in the region, the level of digitization of facilities, production, life and governance matches their own basic conditions and environmental pressures, and the needs and behaviors of the stakeholders, thus achieving high-quality development.
Second, there are large differences in the mechanisms driving rural digitalization performance and development sustainability, and the nature, intensity, spatial effects and interactions of the same factor on them are not identical. For example, average length of education (matching of residents’ knowledge structure) playing a positive role is a key factor for the rural digitalization sustainable development index (rural digitalization sustainability), and a key factor for the rural digitalization state index (rural digitalization construction performance); the spatial effect changes from high levels in the southwest and low levels elsewhere to high levels in the east and low levels in the northwest (Figure 10).
4.2 Spatial policy: planning zoning management
In the past, the central and local governments preferred to design unified planning and policies in the practice of rural digitalization and urbanization, leading to poor implementation performance (Zhang, 2021). The rural digitalization in Hunan is geographically unequal and unbalanced, with geographical clustering and spatial heterogeneity characterizing both construction performance and development sustainability. Therefore, in the process of policy design, it is necessary to adapt to local conditions and implement differentiated management based on the evaluation results of construction performance and sustainability in Sections 3.1, 3.2. Based on the coordination between rural digitalization construction performance and development sustainability as well as their geographic distribution and spatial effect characteristics, Hunan is divided into four policy zonings: leading, potential, warning, and general zones (Table 5). For the leading zone, the performance of rural digitalization construction is in harmony with the sustainability of development, and they have at least one index more than 0.5. Bwangcheng, Changsha, Liuyang, Ningxiang, Shaoshan and other regions are leading zones and they should further invest in the future to cultivate themselves as a business card of Hunan to participate in national and global competition. Counties with the sustainability of rural digitalization much higher than construction performance are assigned to the potential zone, including You, Chaling, Yanfeng, Nanyue, Hengdong, Daxiang, Suining, and Chengbu. In the future, they should be given priority in related fields such as funds, policies and talents, so as to drive further improvements in construction performance and development sustainability, and to build themselves into new provincial leaders in Hunan with high-quality rural digitization. Counties with rural digitalization sustainability far lower than the construction performance are put into the warning zone, including Qidong, Dingcheng, Cili, Nan, Taojiang, Beihu, and Lingling. In the future, it is necessary to limit investment in their constructive projects and increase investment in the maintenance and management of facilities, so as to raise the level of development sustainability, and to weaken and avoid the waste of resources. All other counties are classified in the general zone, which has a large number of members, including Lukou, Yanling, Liling, Xiangtan, Xiangxiang, Zhuhui, Hengyang, Hengnan, Hengshan, Leiyang, and Changning. The majority of general zone members rely on the market to explore and establish endogenous development models; while a few realize leapfrog development with the help of external assistance based on the rural tourism and scenic spot development, rural cultural heritage, historical heritage and traditional village protection projects. It should be noted that as the rural digitalization is still in its infancy and faces many dilemmas and challenges. Therefore, in order to improve efficiency and effectiveness, Hunan should prioritize development resources and factors towards the first three types of policy zones to better play the role of guidance and control (Adamowicz, 2020; Wang, 2021).
Rural digital development policy and planning used to be prepared independently by local governments and was highly closed. The multi-city comparative analysis of Hunan reveals strong spatial autocorrelation of rural digitalization between different cities, and interactions between neighboring cities in terms of construction performance and sustainability. Therefore, rural digitalization policies should be integrated and coordinated with spatial planning and design in the future, and local governments should strengthen cooperation with other cities in policy and planning design, implementation and management. Cooperation is available in both intra-group and inter-group forms, with the former referring to cooperation between different cities within the same policy area, and the latter referring to cooperation between cities that are in different policy areas but highly spatially correlated with each other. Intra-group cooperation highlights regularity and commonality, with its focus on the generalized work of rural digital construction and the joint promotion of regional projects; inter-group cooperation highlights distinctiveness and differences, focusing on seeking staggered development and learning from other leading cities to catch up with them. For cities with a high degree of inter-group correlation, those lagging behind in rural digitalization should, by benchmarking, clarify their own future development goals and directions, and the road map and timetable for catching up; those in the lead should timely summarize their own successful experience, design the standards and norms for the construction of digital villages, and, by taking the initiative to establish a “peer-to-peer” partnership with those lagging behind, give full play to their own regional demonstration effect and driving power, and create a brand image.
Rural digital development policies and spatial planning were generally made in the past by agriculture and rural administration alone, and they were therefore monolithic and independent. The previous analysis shows that rural digitization performance and development sustainability are affected by many factors, and there are significant interactions between different factors. Therefore, it is necessary to introduce more government departments in the design, implementation and management of future rural digitalization policies and spatial planning, and strengthen the coordination and cooperation between different government departments, so as to effectively exert policy effects and release policy dividend based on a “combination of policies.” According to the acting forces of influencing factors, combined with the main tasks of rural digitalization, Hunan should establish a leading group for digital village construction early in the future. The leader of the group should be the first person in charge of the local government, and the participants should include the Bureau of Agriculture and Rural Affairs, the Office of Internet Information, the Development and Reform Commission, the Science and Technology Bureau, the Bureau of Industry and Information Technology, the Bureau of Finance, and the Bureau of Transportation. Besides, highly related companies such as China Telecom, China Unicom and China Mobile, and administration of power supply, should be members of the group. In response to the problems facing rural digitalization of Hunan, all departments should work together to develop policies on finance, land, human resources, information infrastructure, smart agriculture, e-commerce, population, and clarify the division of labor and responsibilities among the departments, the routes of implementation, and the timeline. And they should design multi-policy combination schemes based on the interactions between different factors to enhance the relevance and synergy between different policies and to gain optimal policy performance.
5 Conclusion
Rural digitization has become the new trend of rural revitalization, and this paper empirically investigated Hunan Province of China based on a combination of many methods such as PSR, TOPSIS, ESDA, GWR and GeoDetector. The findings are as follows:
First, sustainability and construction performance in rural digitalization in Hunan were characterized by significant spatial inequality, with coefficients of variation of 0.33 and 0.24. The cities, varying greatly from each other, were classified into high, medium and low levels and were distributed in clusters or bands. In addition, rural digitalization sustainable development and construction performance showed significant spatial correlation effects, with Moran’s I of 0.29 and 0.34, respectively. Most of the hotspots were clustered in the provincial capital metropolitan area, while the coldspots were in the west and extended to the southwest and southeast corners.
Second, the rural digitalization in Hunan showed significant non-equilibrium across different dimensions and brought forward diversified combination patterns, including single dimensional leadership, dual dimensional leadership, three-dimensional leadership, and all-round development. From the perspective of rural digitalization construction performance, 15 combination patterns are possible theoretically in the four dimensions of infrastructure, economy, governance and life. More than 95% of the counties in Hunan had coefficients of variation more than 0 in the four dimensions, and 7 combination patterns of I (rural infrastructure digitalization), IL (rural infrastructure + life digitalization), IG (rural infrastructure + governance digitalization), IE (rural infrastructure + economy digitalization), IEG (rural infrastructure + economy + governance digitalization), IEL (rural infrastructure + governance + life digitalization), and IEGL (infrastructure + economy + governance + life) came into being. From the perspective of sustainability of rural digitalization, seven combination patterns are possible in theory in three dimensions of pressure, state, and response. More than 90% of the counties in Hunan had coefficients of variation more than 0.16 in the three dimensions, and all combination patterns appeared, that is, P (pressure), S (state), R (response), PS (pressure + state), PR (pressure + response), SR (state + response), and PSR (pressure + state + response).
Third, the sustainability of rural digitalization and construction performance in Hunan is subject to complex driving mechanisms, and there are great differences in the nature, intensity, spatial effects and interactions between different influencing factors, which have a significant impact on the multi-policy combination scheme and its implementation. Economic development (gross domestic product) is a positive key factor, and the spatial effects of development sustainability are high in the south and low in the north, and high in the northeast and low in the southwest for construction performance. Government intervention capacity (fiscal self-sufficiency rate) is an important factor, playing a positive driving role of edge high and center low on development sustainability, and a negative constraining role of high in the southeast and low in the northwest on construction performance. Matching of residents’ knowledge structure (average length of education) is a positive key factor affecting the rural digitalization sustainability, with a spatial effect of high levels in the southwest and low levels elsewhere; it is also a positive important factor affecting construction performance, with a spatial effect of high levels in the east and low levels in the northwest. Common prosperity social policy (urban-rural income ratio) is a negative auxiliary factor affecting the rural digitalization sustainability, characterized by high levels in the southwest and low levels in the north; it is also a mixed (both positive and negative) important factor affecting construction performance, characterized by high levels in the north and low levels in the south. The natural environment (relief amplitude) is a mixed auxiliary factor, with spatial effects of high levels in the east and low levels in the west in development sustainability, and positive high levels in the southwest and negative high levels in the north in construction performance. There are significant synergistic effects between different factors, and their interaction is mainly characterized by nonlinear enhancement, with the factor-pair interaction effect of development sustainability stronger than that of construction performance.
Fourth, it is recommended that policy design should be tailored to local conditions with implementation of differentiated management, while emphasis should be placed on integration and coordination, and cooperation with neighboring counties should be strengthened. Based on the coordination of rural digital construction performance and development sustainability, as well as their geographic distribution and spatial effect characteristics, Hunan is divided into four planning zonings of leading, potential, warning and general zones, and it is recommended that the government implement differentiated spatial management policies. And sufficient attention should be given to the spatial and interactive effects of factor actions in policy design, especially super factor pairs such as gross domestic product (
It should be noted that there are some limitations in this paper. For example, due to the shortage of data, this paper only carries out the analysis of cross-sectional data with no analysis of time-series and panel data, resulting in impossibility to determine the changing trend of rural digitalization sustainability and construction performance. In summary, sustainability of rural digitalization has a richer connotation than construction performance. The former is more appropriate for the current and future mature stages of rural digitalization, while the latter is more appropriate for its past start-up stages. Digital and smart villages are complex adaptive systems, and promoting mutual synergy and coupling of different subsystems is an important way to realize sustainable development and also an important direction for our future research. For example, Rural Infrastructure Digitalization, Rural Economy Digitalization, Rural Governance Digitalization and Rural Life Digitalization are four sub-systems of digital and smart villages, and the coupling coordination between them is an important indicator to show the soundness of the entire rural digitalization system. Scholars are not currently paying enough attention to it. In the context of high-quality and sustainable rural digitalization, the analysis of the coupling coordination between different subsystems is a new direction worthy of future in-depth research. Therefore, we call on more scholars to shift their focus of research from construction performance to development sustainability, to promote rural digitization towards high-quality development from a broader perspective and based on comprehensive systematic thinking.
Data availability statement
Publicly available datasets were analyzed in this study. This data can be found here: The data used in this paper mainly came from the Hunan Provincial Bureau of Statistics (http://www.hunan.gov.cn/hnszf/zfsj/tjnj/tygl.html, 18th April 2023) and Peking University Open Research Data Platform (https://opendata.pku.edu.cn/file.xhtml?fileId=12781&datasetVersionId=1020, 23th January 2023).
Author contributions
ZX: Conceptualization, Data curation, Formal Analysis, Funding acquisition, Investigation, Methodology, Project administration, Resources, Validation, Writing–original draft. SZ: Data curation, Formal Analysis, Methodology, Software, Validation, Visualization, Writing–original draft, Writing–review and editing. DW: Conceptualization, Formal Analysis, Funding acquisition, Investigation, Methodology, Project administration, Resources, Supervision, Validation, Writing–review and editing.
Funding
The authors declare financial support was received for the research, authorship, and/or publication of this article. This research was funded by Scientific Research Project of Hunan Provincial Department of Education (21C1087).
Acknowledgments
All the authors are grateful to the reviewers and editors.
Conflict of interest
The authors declare that the research was conducted in the absence of any commercial or financial relationships that could be construed as a potential conflict of interest.
Publisher’s note
All claims expressed in this article are solely those of the authors and do not necessarily represent those of their affiliated organizations, or those of the publisher, the editors and the reviewers. Any product that may be evaluated in this article, or claim that may be made by its manufacturer, is not guaranteed or endorsed by the publisher.
References
Adamowicz, M. (2021). The potential for innovative and smart rural development in the peripheral regions of eastern Poland. Agriculture-Basel 11 (3), 188. doi:10.3390/agriculture11030188
Adamowicz, M., and Zwolinska-Ligaj, M. (2020). The "smart village" as a way to achieve sustainable development in rural areas of Poland. Sustainability 12 (16), 6503. doi:10.3390/su12166503
Adesipo, A., Fadeyi, O., Kuca, K., Krejcar, O., Maresova, P., Selamat, A., et al. (2020). Smart and climate-smart agricultural trends as core aspects of smart village functions. Sensors 20 (21), 5977. doi:10.3390/s20215977
Agusta, Y. (2023). Managing the development of a sustainable digital village. Sustainability 15 (9), 7575. doi:10.3390/su15097575
Alabdali, S. A., Pileggi, S. F., and Cetindamar, D. (2023). Influential factors, enablers, and barriers to adopting smart technology in rural regions: a literature review. Sustainability 15 (10), 7908. doi:10.3390/su15107908
Arroyo-Menendez, M., Gutierrez-Laiz, N., and Criado-Quesada, B. (2022). The digitization of seniors: analyzing the multiple confluence of social and spatial divides. Land 11 (6), 953. doi:10.3390/land11060953
Billon, M., Lera-Lopez, F., and Marco, R. (2010). Differences in digitalization levels: a multivariate analysis studying the global digital divide. Rev. World Econ. 146 (1), 39–73. doi:10.1007/s10290-009-0045-y
Budziewicz-Guzlecka, A., and Drozdz, W. (2022). Development and implementation of the smart village concept as a challenge for the modern power industry on the example of Poland. Energies 15 (2), 603. doi:10.3390/en15020603
Cai, Z., Li, S. S., and Cheng, D. (2023). Has digital village construction improved rural family resilience in China? Evidence based on China Household finance survey. Sustainability 15 (11), 8704. doi:10.3390/su15118704
Cambra-Fierro, J. J., and Perez, L. (2022). (Re)thinking smart in rural contexts: a multi-country study. Growth Change 53 (2), 868–889. doi:10.1111/grow.12612
Cao, X. Z., Yan, M. Y., and Wen, J. (2023). Exploring the level and influencing factors of digital village development in China: insights and recommendations. Sustainability 15 (13), 10423. doi:10.3390/su151310423
Chen, W., Wang, Q. Z., and Zhou, H. (2022). Digital rural construction and farmers' income growth: theoretical mechanism and micro experience based on data from China. Sustainability 14 (18), 11679. doi:10.3390/su141811679
Chinn, M. D., and Fairlie, R. W. (2007). The determinants of the global digital divide: a cross-country analysis of computer and internet penetration. Oxf. Econ. Papers-New Ser. 59 (1), 16–44. doi:10.1093/oep/gpl024
Ciolac, R., Iancu, T., Popescu, G., Adamov, T., Feher, A., and Stanciu, S. (2022). Smart tourist village-an entrepreneurial necessity for maramures rural area. Sustainability 14 (14), 8914. doi:10.3390/su14148914
Dang, Y., Wang, C. J., and Chen, P. R. (2022). Identification and optimization strategy of urban park service areas based on accessibility by public transport: Beijing as a case study. Sustainability 14 (12), 7112. doi:10.3390/su14127112
Escalona-Orcao, A. I., and Escolano-Utrilla, S. (2016). El potencial para el desarrollo inteligente de las áreas rurales: los clústeres espaciales de actividades creativas en España. Rev. De. Geogr. Norte Gd. 65, 91–107. doi:10.4067/S0718-34022016000300005
Fennell, S., Kaur, P., Jhunjhunwala, A., Narayanan, D., Loyola, C., Bedi, J., et al. (2018). Examining linkages between smart villages and smart cities: learning from rural youth accessing the internet in India. Telecommun. Policy 42 (10), 810–823. doi:10.1016/j.telpol.2018.06.002
Fernandez, C. G., and Peek, D. (2023). Connecting the smart village: a switch towards smart and sustainable rural-urban linkages in Spain. Land 12 (4), 822. doi:10.3390/land12040822
Ferrari, A., Bacco, M., Gaber, K., Jedlitschka, A., Hess, S., Kaipainen, J., et al. (2022). Drivers, barriers and impacts of digitalisation in rural areas from the viewpoint of experts. Inf. Softw. Technol. 145, 106816. doi:10.1016/j.infsof.2021.106816
Galiwango, H., Miiro, R. F., Turyahabwe, N., and Egeru, A. (2022). Does the climate-smart village extension model enhance farmer empowerment? Outlook Agric. 51 (3), 281–292. doi:10.1177/00307270221113600
Hao, A. M., Hou, Y. R., and Tan, J. Y. (2022). How does digital village construction influences carbon emission? case China 17 (12), e0278533. doi:10.1371/journal.pone.0278533
Ho, W. S., Hashim, H., and Lim, J. S. (2014). Integrated biomass and solar town concept for a smart eco-village in Iskandar Malaysia (IM). Renew. Energy 69, 190–201. doi:10.1016/j.renene.2014.02.053
Hou, X. F., Zhang, D. F., Fu, L. Y., Zeng, F., and Wang, Q. (2023). Spatio-Temporal evolution and influencing factors of coupling coordination degree between urban-rural integration and digital economy. Sustainability 15 (12), 9718. doi:10.3390/su15129718
Huang, Z. M., and Liang, Y. M. (2020). Digital protection and inheritance of ancient villages in southwest minority areas under the strategy of rural revitalization. Technol. Forecast. Soc. Change 160, 120238. doi:10.1016/j.techfore.2020.120238
Jiang, C. J., and Jin, B. H. (2023). Does the development of digital economy infrastructure reduce the urban-rural income gap? Theoretical experience and empirical data from China. Kybernetes. doi:10.1108/K-12-2022-1744
Leong, C., Pan, S. L., Newell, S., and Cui, L. L. (2016). The emergence of self-organizing E-commerce ecosystems in remote villages of China: a tale of digital empowerment for rural development. MIS Q. 40(2):475–484. doi:10.25300/MISQ/2016/40.2.11
Li, H., and Yang, S. G. (2023). The road to common prosperity: can the digital countryside construction increase Household income? Sustainability 15 (5), 4020. doi:10.3390/su15054020
Li, H. J., Shi, Y., Zhang, J. X., Zhang, Z. K., Zhang, Z. S., and Gong, M. G. (2023c). Digital inclusive finance & the high-quality agricultural development: prevalence of regional heterogeneity in rural China. Plos One 18 (3), e0281023. doi:10.1371/journal.pone.0281023
Li, J. (2022). Application of spatial-temporal behavioral trajectory analysis in the space design of digital villages. Wirel. Commun. Mob. Comput. 2022, 1–10. doi:10.1155/2022/2304820
Li, L., Zhao, K., Wang, X., Zhao, S., Liu, X., and Li, W. (2022). Spatio-Temporal evolution and driving mechanism of urbanization in small cities: case study from Guangxi. Land 11, 415. doi:10.3390/land11030415
Li, R. P., Chen, K., and Wu, D. (2020). Challenges and opportunities for coping with the smart divide in rural America. Ann. Am. Assoc. Geogr. 110 (2), 559–570. doi:10.1080/24694452.2019.1694402
Li, T., Wang, S., Chen, P., Liu, X., and Kong, X. (2023b). Geographical patterns and influencing mechanisms of digital rural development level at the county scale in China. Land 12, 1504. doi:10.3390/land12081504
Li, W., Zhang, P., Zhao, K., Chen, H., and Zhao, S. (2023a). The evolution model of and factors influencing digital villages: evidence from Guangxi, China. Agriculture 13, 659. doi:10.3390/agriculture13030659
Li, W. Z., and Zhong, H. (2022). Development of a smart tourism integration model to preserve the cultural heritage of ancient villages in Northern Guangxi. Herit. Sci. 10 (1), 91. doi:10.1186/s40494-022-00724-3
Liu, L. H., Zhang, Y. X., and Bi, Y. M. (2022). Digital village construction boosts rural revitalization: internal mechanism and empirical test. World Agric. (08), 51–65. doi:10.13856/j.cn11-1097/s.2022.08.005
Lofving, L., Kamuf, V., Heleniak, T., Weck, S., and Norlen, G. (2021). Can digitalization be a tool to overcome spatial injustice in sparsely populated regions? The cases of Digital Vasterbotten (Sweden) and Smart Country Side (Germany). Eur. Plan. Stud. 30 (5), 917–934. doi:10.1080/09654313.2021.1928053
Maja, P. W., Meyer, J., and Von Solms, S. (2020). Development of smart rural village indicators in line with industry 4.0. IEEE Access 8, 152017–152033. doi:10.1109/ACCESS.2020.3017441
Malik, P. K., Singh, R., Gehlot, A., Akram, S. V., and Das, P. K. (2022). Village 4.0: digitalization of village with smart internet of things technologies. Comput. Industrial Eng. 165, 107938. doi:10.1016/j.cie.2022.107938
Marquaridt, D. W. (1970). Generalized inverses, ridge regression, biased linear estimation, and nonlinear estimation. Technometrics 12 (3), 591–612. doi:10.1080/00401706.1970.10488699
McGuire, R., Longo, A., and Sherry, E. (2022). Tackling poverty and social isolation using a smart rural development initiative. J. Rural Stud. 89, 161–170. doi:10.1016/j.jrurstud.2021.11.010
Mei, Y., Miao, J. Y., and Lu, Y. H. (2022). Digital villages construction accelerates high-quality economic development in rural China through promoting digital entrepreneurship. Sustainability 14 (21), 14224. doi:10.3390/su142114224
Murty, V. K., and Shankar, S. S. (2020). Towards a scalable architecture for smart villages: the discovery phase. Sustainability 12 (18), 7580. doi:10.3390/su12187580
Naldi, L., Nilsson, P., Westlund, H., and Wixe, S. (2015). What is smart rural development? J. Rural Stud. 40, 90–101. doi:10.1016/j.jrurstud.2015.06.006
Park, S. (2017). Digital inequalities in rural Australia: a double jeopardy of remoteness and social exclusion. J. Rural Stud. 54, 399–407. doi:10.1016/j.jrurstud.2015.12.018
Perez-Martinez, J., Hernandez-Gil, F., San Miguel, G., Ruiz, D., and Arredondo, M. T. (2023). Analysing associations between digitalization and the accomplishment of the sustainable development goals. Sci. Total Environ. 857 (3), 159700. doi:10.1016/j.scitotenv.2022.159700
Putri, C. M., Argiles-Bosch, J. M., and Ravenda, D. (2023). Thirty years of village corruption research: accounting and smart villages for village sustainability as future research direction. Sustainability 15 (12), 9264. doi:10.3390/su15129264
Qi, T. J., Ma, L. Q., Zhou, W. H., and Dai, L. X. (2022). Traditional village digital archival conservation: a case study from Gaoqian, China. Archives Records-the J. Archives Rec. Assoc. 44, 202–220. doi:10.1080/23257962.2022.2104236
Rahoveanu, M. M. T., Serban, V., Zugravu, A. G., Rahoveanu, A. T., Cristea, D. S., Nechita, P., et al. (2022). Perspectives on smart villages from a bibliometric approach. Sustainability 14 (17), 10723. doi:10.3390/su141710723
Ram, S. K., Das, B. B., Mahapatra, K., Mohanty, S. P., and Choppali, U. (2021). Energy perspectives in IoT driven smart villages and smart cities. IEEE Consum. Electron. Mag. 10 (3), 19–28. doi:10.1109/MCE.2020.3023293
Randell-Moon, H. E. K., and Hynes, D. (2022). Too smart': infrastructuring the Internet through regional and rural smart policy in Australia. Policy Internet 14 (1), 151–169. doi:10.1002/poi3.286
Ren, J. M., Zheng, C. R. Y., Guo, F. Y., Zhao, H. B., Ma, S., and Cheng, Y. (2022). Spatial differentiation of digital rural development and influencing factors in the yellow River Basin, China. Int. J. Environ. Res. Public Health 19 (23), 16111. doi:10.3390/ijerph192316111
Ren, Y. (2023). Rural China staggering towards the digital era: evolution and restructuring. Land 12, 1416. doi:10.3390/land12071416
Rodrigues, S., Correia, R., Goncalves, R., Branco, F., and Martins, J. (2023). Digital marketing's impact on rural destinations' image, intention to visit, and destination sustainability. Sustainability 15 (3), 2683. doi:10.3390/su15032683
Satola, L., and Milewska, A. (2022). The concept of a smart village as an innovative way of implementing public tasks in the era of instability on the energy market-examples from Poland. Energies 15 (14), 5175. doi:10.3390/en15145175
Shrestha, A., and Luo, W. (2017). Analysis of groundwater nitrate contamination in the central valley: comparison of the geodetector method, principal component analysis and geographically weighted regression. ISPRS Int. J. Geo-Information 6 (10), 297. doi:10.3390/ijgi6100297
Spicer, Z., Goodman, N., and Olmstead, N. (2018). The frontier of digital opportunity: smart city implementation in small, rural, and remote communities in Canada. Urban Stud. 58 (3), 535–558. doi:10.1177/0042098019863666
Stojanova, S., Lentini, G., Niederer, P., Egger, T., Cvar, N., Kos, A., et al. (2021). Smart villages policies: past, present and future. Sustainability 13 (4), 1663. doi:10.3390/su13041663
Sun, D. P., Yu, B. T., and Ma, J. (2023). Research on the impact of digital empowerment on China's human capital accumulation and human capital gap between urban and rural areas. Sustainability 15 (6), 5458. doi:10.3390/su15065458
Tian, Y., Liu, Q., Ye, Y. T., Zhang, Z. F., and Khanal, R. (2023). How the rural digital economy drives rural industrial revitalization-case study of China's 30 provinces. Sustainability 15 (8), 6923. doi:10.3390/su15086923
Wang, H., and Tang, Y. T. (2023). Spatiotemporal distribution and influencing factors of coupling coordination between digital village and green and high-quality agricultural development-evidence from China. Sustainability 15 (10), 8079. doi:10.3390/su15108079
Wang, J. F., and Hu, Y. (2012). Environmental health risk detection with GeogDetector. Environ. Model. Softw. 33, 114–115. doi:10.1016/j.envsoft.2012.01.015
Wang, J. F., Li, X. H., Christakos, G., Liao, Y. L., Zhang, T., Gu, X., et al. (2010). Geographical detectors-based health risk assessment and its application in the neural tube defects study of the Heshun region, China. Int. J. Geogr. Inf. Sci. 24 (1), 107–127. doi:10.1080/13658810802443457
Wang, Q., Luo, S. X., Zhang, J., and Furuya, K. (2022). Increased attention to smart development in rural areas: a scientometric analysis of smart village research. Land 11 (8), 1362. doi:10.3390/land11081362
Wang, Z. K., and Li, X. Q. (2021). Research on the practice and innovation of smart villages under the background of rural vitalization based on ecological theory. Fresenius Environ. Bull. 30 (7A), 9084–9090.
Wojcik, M., Dmochowska-Dudek, K., and Tobiasz-Lis, P. (2021). Boosting the potential for GeoDesign: digitalisation of the system of spatial planning as a trigger for smart rural development. Energies 14 (13), 3895. doi:10.3390/en14133895
You, Z., Feng, Z., Yang, Y., and Yang, Y. (2018). Relief degree of land surface dataset of China (1km). Digital J. Glob. Change Data Repos. doi:10.3974/geodb.2018.03.16.V1
Zavratnik, V., Kos, A., and Duh, E. S. (2018). Smart villages: comprehensive review of initiatives and practices. Sustainability 10 (7), 2559. doi:10.3390/su10072559
Zhang, C. K., Zhang, Y. J., Li, Y., and Li, S. (2023b). Coupling coordination between fintech and digital villages: mechanism, spatiotemporal evolution and driving factors-an empirical study based on China. Sustainability 15 (10), 8265. doi:10.3390/su15108265
Zhang, P., Li, W., Zhao, K., and Zhao, S. (2021). Spatial pattern and driving mechanism of urban–rural income gap in Gansu province of China. Land 10, 1002. doi:10.3390/land10101002
Zhang, P., Li, W., Zhao, K., Zhao, Y., Chen, H., and Zhao, S. (2023a). The impact factors and management policy of digital village development: a case study of Gansu province, China. Land 12, 616. doi:10.3390/land12030616
Zhang, X. J., and Zhang, Z. G. (2021). How do smart villages become a way to achieve sustainable development in rural areas? Smart village planning and practices in China. Sustainability 12 (24), 10510. doi:10.3390/su122410510
Zhang, Y. Q., Ma, G. F., Tian, Y., and Dong, Q. Y. (2023c). Nonlinear effect of digital economy on urban-rural consumption gap: evidence from a dynamic panel threshold analysis. Sustainability 15 (8), 6880. doi:10.3390/su15086880
Zhao, S., Li, W., Zhao, K., and Zhang, P. (2021a). Change characteristics and multilevel influencing factors of real estate inventory—case studies from 35 key cities in China. Land 10, 928. doi:10.3390/land10090928
Zhao, S., Yan, Y., and Han, J. (2021c). Industrial land change in Chinese silk road cities and its influence on environments. Land 10, 806. doi:10.3390/land10080806
Zhao, S., Zhang, C., and Qi, J. (2021b). The key factors driving the development of new towns by mother cities and regions: evidence from China. ISPRS Int. J. Geo-Inf. 10, 223. doi:10.3390/ijgi10040223
Zhao, S., Zhao, K., Yan, Y., Zhu, K., and Guan, C. (2022b). Spatio-Temporal evolution characteristics and influencing factors of urban service-industry land in China. Land 11, 13. doi:10.3390/land11010013
Zhao, W., Liang, Z. Y., and Li, B. R. (2022a). Realizing a rural sustainable development through a digital village construction: experiences from China. Sustainability 14 (21), 14199. doi:10.3390/su142114199
Zhu, Hg, and Chen, H. (2022). Level measurement, spatial-temporal evolution and promotion path of digital village development in China. Agric. Econ. issues 7, 210–223. doi:10.13246/j.cnki.iae.20220728.001
Keywords: digital village, smart village, developing evaluation, driving mechanism, China
Citation: Xing Z, Zhao S and Wang D (2023) Performance and sustainability evaluation of rural digitalization and its driving mechanism: evidence from Hunan province of China. Front. Environ. Sci. 11:1326592. doi: 10.3389/fenvs.2023.1326592
Received: 23 October 2023; Accepted: 16 November 2023;
Published: 05 December 2023.
Edited by:
Xunpeng (Roc) Shi, University of Technology Sydney, AustraliaReviewed by:
Otilia Manta, Romanian Academy, RomaniaYantuan Yu, Guangdong University of Foreign Studies, China
Copyright © 2023 Xing, Zhao and Wang. This is an open-access article distributed under the terms of the Creative Commons Attribution License (CC BY). The use, distribution or reproduction in other forums is permitted, provided the original author(s) and the copyright owner(s) are credited and that the original publication in this journal is cited, in accordance with accepted academic practice. No use, distribution or reproduction is permitted which does not comply with these terms.
*Correspondence: Di Wang, wangdi@yangtzeu.edu.cn