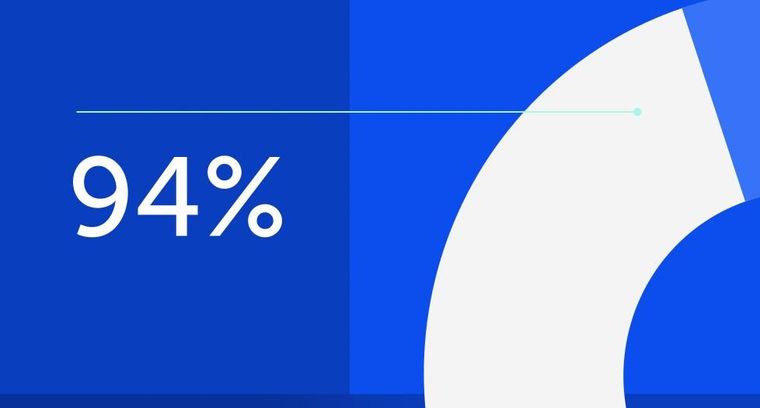
94% of researchers rate our articles as excellent or good
Learn more about the work of our research integrity team to safeguard the quality of each article we publish.
Find out more
REVIEW article
Front. Environ. Sci., 30 November 2023
Sec. Toxicology, Pollution and the Environment
Volume 11 - 2023 | https://doi.org/10.3389/fenvs.2023.1326425
The ecosystem services approach offers a more ecologically relevant method to establish environmental conservation goals and implement ecological risk assessment (ERA). The emergence of bibliometrics has facilitated the development of new systematic review techniques. In this study, we utilised CiteSpace, VOSviewer, and SciMAT software, based on the Web of Science database, to qualitatively and quantitatively analyse the ecosystem service-based ecological risk assessment (ESRA) literature knowledge map spanning from 1994 to 2023. This article explored the field’s evolution from macro to micro perspectives, incorporating background information, current trends, and knowledge structure. The findings demonstrate that ESRA has progressed from an initial stage to a phase of global cooperation and policy applications. This transition between stages has been characterised by a shift from focusing on natural processes to understanding human impacts on ecosystems. Key themes identified include ecosystem services, landscape ERA, aquatic ERA and ecosystem health. The overall development of ERA can be observed as a progression through different periods, namely, the traditional era, regional era, and landscape era. Currently, landscape ERA methods based on changes in land use and land cover are widely employed. The study also revealed various challenges in the ESRA field, such as data availability, scale issues, and uncertainty. Future ESRA studies should consider holistic ecosystem services, interdisciplinary approaches, ecological models, and advanced technologies to address complexity. Using big data and informatisation for research offers new opportunities but requires integration and innovation. It is anticipated that ESRA holds promise for ecological sustainability and human wellbeing.
Ecological risk assessment (ERA; called environmental risk assessment in many parts of the globe (Munns Jr et al., 2017)) aims to estimate the adverse impacts of human activities on ecosystems and their components (Galic et al., 2012a). However, ERA practices rarely incorporate ecology and instead rely on standard test results of several indicator species to assess risks. One of the main challenges facing ERA with stronger ecological relevance is the lack of legible conservation objectives and the inherent intricacies of ecosystems, making it difficult to address these issues empirically. The conceptual structure of ecosystem service (ES) offers a conducive platform for delineating explicit and environmentally pertinent goals pertaining to conservation. ERA constitutes a methodological procedure aimed at appraising the prospective detrimental repercussions and vulnerabilities induced by human endeavours and diverse anthropogenic pressures upon ecosystems or their constituent elements (Munns Jr et al., 2016). While ERA theoretically encompasses any activity, it traditionally focuses on evaluating risks associated with chemical pollution. This assessment is based on existing ecotoxicological data that examine the effects of a single pollutant on the growth, reproduction, or survival of a single species or the processes performed by microorganisms. Additionally, the current ERA lacks thorough consideration of ecological factors, such as species’ life history traits, population structure, density-dependent regulations, species interactions, and landscape structure (Van den Brink, 2008). Nevertheless, habitat loss, degradation, and fragmentation threaten ecosystems worldwide (Hope, 2006; Tittensor et al., 2014). The significance of these established and emerging matters emphasises the requirement for flexible strategies in addressing problems, effectively linking ecological measurements and data with the decision-making demands of environmental managers. ERA is increasingly recognised as an integrated science, policy, and management approach to address these broad ecological issues (Sommers et al., 1999). However, an exclusive focus on protecting ecological entities, without considering their closely associated impacts on human wellbeing, is challenging to implement at a specific decision-making level. Consequently, as human living standards improve, the concept of ERA gradually extends to ES, closely linked to the quality of human life. Some scholars argue that improving ERA aims to provide decision-makers with more information, ultimately establishing a relationship between ecosystem processes and services (Thomsen et al., 2012).
Recently, there has been a robust surge in utilising the ES concept within environmental management. This trend holds the promise of serving as a valuable instrument for formulating purposeful objectives in ecological preservation. ES encompass the advantages extracted by individuals from ecosystems. These advantages can be influenced either directly or indirectly by the driving forces of alteration (Assessment, 2005). The millennium ecosystem assessment (Assessment, 2005) and previous notable efforts (Costanza et al., 1997, Daily 1997) have garnered global awareness of ecosystems’ importance for human welfare. In addition to species-specific conservation endeavours, there is a growing emphasis on conserving ecosystems at a broader scale (Rodríguez et al., 2011; Holdaway et al., 2012). Using ecosystem service-based ecological risk assessment (ESRA) can help mitigate biases associated with species data, such as spatial sampling biases (Maddock et al., 1999; Pressey, 2004). ESRA also directly addresses the primary causes of biodiversity loss, namely, habitat destruction (Noss and Peters 1995; Orians, 1993). In related research, there has been a shift towards more comprehensive approaches in ERA, including the incorporation of vulnerability concepts (De Lange et al., 2010), population modelling in ERA (Forbes et al., 2010), and the use of ES as endpoints in ERA (Munns Jr et al., 2016).
Given the increasing significance of ESRA in the 21st century, there is a need for a comprehensive and systematic review. This paper focuses on conducting a multidimensional analysis of the different disciplines reflected in English literature on “ESRA” using three kinds of bibliometric software. The main objective of this paper is to provide a thorough understanding and systematic overview of ESRA research. Quantitative and qualitative analyses were performed using bibliometric methods and visualisation tools to gain insights into ESRA research’s current status and future directions. The study evaluated key characteristics and mapped knowledge domains, such as co-word analysis, to identify significant themes in ESRA research. Additionally, it tracked the knowledge base in recent years and made scientific predictions about future research directions.
This paper’s initial step involved selecting reference journal articles from the Web of Science (WoS) database to create a unified analysis database. WoS is widely recognised as a primary source of authoritative and representative citation data (Mongeon and Paul-Hus, 2016). Moreover, it allows for a more comprehensive citation analysis (Falagas et al., 2008). To enhance the scientific rigour of this study, the search was constrained by constructing search strings (Birkle et al., 2020).
The second phase aimed to retrieve database records. This article utilised specific criteria to select papers from academic journals in numerous databases relevant to the research topic. Data were collected from the WoS by inputting the search terms into WoS’s topic field (TS). This selection procedure follows the latest advancements in search string retrieval strategies (Ng et al., 2022). The complete search query used was (TS=(“risk assessment” OR ″ ecological risk assessment") AND TS= (“ecosystem service” OR “environmental service” OR “ecosystem services”) AND DT=(“ARTICLE” OR “REVIEW”) AND LA=(“ENGLISH”)). The retrieval date was 6 June 2023. In bibliometric research, articles and reviews are considered more significant scientific contributions than conference proceedings or book reviews, making them worth considering (Su et al., 2019).
The third stage was literature selection. Firstly, three redundant articles were excluded from the initial 847 articles obtained and verified that they met the inclusion criteria. Subsequently, the core content of each article was thoroughly read and analysed for its relevance to ESRA or ERA topics. Two types of literature were excluded: 1) literature that referred to the content of ES and ERA but only as Supplementary information, e.g., assessing flood risk dynamics, and 2) content that referred to the terms ES and risk assessment but the research was not part of ERA or ESRA, e.g., part of the content referred to analysing the impacts on the safety of agricultural products through soil and environmental risks. Ultimately, 815 peer-reviewed articles were selected for subsequent bibliometric analyses (Figure 1).
Bibliometric analysis constitutes a quantitative approach to assessing scholarly literature, accomplished through examining reference bibliographies. Its objective is to furnish a depiction, assessment, and surveillance of research that has been disseminated (Garfield et al., 1964). This method is commonly employed to analyse trends in the field of ESRA. By analysing publications, citations, and sources of information, bibliometric analysis allows for answering various research questions through scientific mapping (Aria and Cuccurullo, 2017). This paper has opted for the most suitable methods to achieve our research goal, as described by Donthu et al. (2021). This analysis can detect the evolutionary trends in specific research fields and identify emerging topics that shape the progression of knowledge in those fields.
CiteSpace and VOSviewer are commonly used software applications for bibliometric analysis and visualisation. They aid researchers in constructing knowledge networks and identifying significant achievements in their respective fields (Van Eck and Waltman, 2010; Zhang J. et al., 2020). Since VOSviewer utilises more advanced algorithms and computational logic, it is well-suited for processing and visualising extensive datasets, thereby ensuring the production of high-quality visualisations (Van Eck and Waltman, 2010). Additionally, there is SciMAT, an open-source scientific mapping software that describes the evolution of topics and concepts in research fields. It can generate thematic evolution cluster maps based on time series data (Cobo et al., 2012). This study integrates these three software tools to collect, organise, and visualise ESRA-related research data. Through these analyses, researchers can objectively summarise and evaluate the evolution of research backgrounds, significant topics, and knowledge structures. This process facilitates a comprehensive comprehension of the field’s research foundation, frontiers, and hotspots and enables predictions of potential research trends. In Figure 1, distinct colours denote the application contents of various bibliometric software applications.
According to the scientific output from 1994 to 2023 (Figure 2), the research process can be divided into four distinct periods, each characterised by increasing production and significant indicators.
• The first stage, the initial development stage (1994–2005), experienced slow and fluctuating growth. The annual publication volume did not exceed 10 during this period. However, over time, the concepts and importance of ES were gradually recognised and understood. The release of the Millennium Ecosystem Assessment at this stage served as a significant milestone, laying the foundation for subsequent research (Assessment, 2005).
• The second stage (2006–2012) witnessed a notable growth trend. Following the publication of the Millennium Ecosystem Assessment in 2005, ES gained more attention, resulting in a considerable increase in relevant literature. Within this period, research on ES assessment methods expanded in breadth and depth, encompassing their application in ERA. Countries further acknowledged the significance of ES in environmental management and decision-making. Hence, this stage is commonly called the expansion and application stage.
• The third stage (2013–2019) was characterised by global cooperation and policy implementation. The CBD COPs 10 (Sugiyama and Sugiyama, 2010) and the 2013 IUCN red list of ecosystems (Keith et al., 2015) played pivotal roles in expediting this process. The former emphasised the application of ecosystem-based approaches and risk assessment, while the latter established global standards for environmental risk assessment (Keith et al., 2015). International attention towards ES and ecosystem risk assessment continued to grow throughout this stage.
• The fourth stage (2020–2023) signifies the stage of promotion and innovation. The research focus on ESRA experienced rapid growth, and ESAR displayed significant expansion since 2019, with annual publication volumes exceeding 80. Upon examining the data based on publication years, limited publications were found for 2023 due to time restrictions. However, the research literature on ERA peaked in 2022 with over 120 articles (Figure 2). This indicates that the integration of ES into risk assessment presently represents a pressing area of focus exhibiting a solid trend. Within this stage, research and application of ES and ecosystem risk assessment continued to expand and innovate. This includes the development of new assessment methods, technologies, and tools, as well as the broader application of ES in areas such as climate adaptation, ecological restoration, and sustainable development (Asmus et al., 2019; Huang et al., 2022; Liang and Song, 2022).
An analysis of the thematic evolution in ESRA from 1994 to 2023 reveals significant inflexion points. Figure 3 illustrates four distinct periods: 1994–2005, 2006–2012, 2013–2019, and the latest period of 2020–2023.
• From 1994 to 2005 (Period I): This period had 11 themes, with 9% of them persisting in subsequent periods.
• From 2006 to 2012 (Period II): During this period, there were 47 themes, including 46 new themes that emerged and 21 themes that were lost while retaining 55% of the original themes.
• From 2013 to 2019 (Period III): The number of themes increased to 121, consisting of 95 new and 47 lost themes. The 2020–2023 period preserved 61% of the themes.
• From 2020 to 2023 (Period IV): The latest period encompasses 140 themes, of which 66 are new.
These data illustrate the trend of thematic evolution in ESRA, including the emergence of new themes and the loss of old themes across various periods. This analysis aids in comprehending the knowledge structure and identifying changes in research trends within this field, offering valuable insights to researchers.
Figure 4 displays the cooperation among countries regarding ESRA themes. The size of the dots in Figure 4 corresponds to the number of publications from each country on ESRA themes, while the thickness of the lines represents the degree of inter-country collaboration. Numerous countries have actively collaborated in ESRA research. China, indicated by the giant dot, is the most active country in the ESRA field, highlighting its substantial contribution to ESRA research. China collaborates closely with other countries, especially the United States and Australia. The United States, closely collaborating with the United Kingdom, Sweden, and others, ranks as the second most active country in the ESRA field. Germany, Italy, France, and the Netherlands have also contributed to ESRA research. Despite their relatively small publication numbers, these countries actively collaborate with others. This study primarily focuses on developed countries, underscoring their dominant ESRA research and collaboration position. This collaborative effort fosters knowledge exchange and advances research in ESRA.
Table 1 ranks the top 10 institutions with the highest citation counts. The total link strength indicates the level of cooperation between institutions, with a higher quantity representing more collaborative partnerships. The Chinese Academy of Sciences stands out as the institution with the highest citation count, having received 1,562 citations. The Helmholtz Centre for Environmental Research follows it with 1,411 citations and NOAA with 714 medals. However, the number of sources an institution receives does not always directly correlate with its level of collaboration with others. Among the top three institutions, the Chinese Academy of Sciences stands out with the highest citation count and a relatively high frequency of partnerships with other institutions. The University of Sheffield and the US EPA closely trail behind in total link strength, scoring 45 and 44, respectively.
Figure 5 displays the overlapping double maps of ESRA thematic journals, illustrating the distribution of research topics and citation relationships among journals from 1994 to 2023. This map reflects the primary research topics and journal interrelationships in the field. The blue path originates from the “environmental science” field and encompasses aspects such as ecosystem, earth, and marine environments. It extends to ecology (including botany and zoology), environmental toxicology, and geography. It reveals close citation relationships among these fields, indicating the potential for researchers to draw inspiration from ecological science and publish their research in related areas. The green path originating from the field of veterinary medicine connects to ecology (including botany and zoology) and environmental toxicology. This indicates an intersection between veterinary medicine and ecology and environmental toxicology research, potentially involving matters concerning wildlife health and environmental issues. Furthermore, the blue and green paths extend to social sciences and medicine fields, encompassing disciplines such as social psychology and economics. This map aids in comprehending the research dynamics and interdisciplinary communication among researchers in the ESRA field. It demonstrates the citation relationships among diverse journals and underscores the field’s multidisciplinary nature.
The keywords in this research field can be classified into five categories based on the co-occurrence keyword network, with each type closely associated with a specific theme. Firstly, the red keywords are closely associated with ES, encompassing management, biodiversity, and climate change. These keywords mainly pertain to the research, management, and evaluation of ES. Secondly, the blue keywords encompass the themes related to landscape ERA, including land use, ecological risk, landscape pattern, and vegetation. These keywords are relevant to the research on landscape ERA and land use. Thirdly, the orange keywords are associated with aquatic ERA, encompassing rivers, heavy metals, and toxicity. These keywords are closely linked to topics such as aquatic ERA and the toxicity of heavy metals. The purple keywords correspond to ERA and ecosystem health themes, encompassing risk assessment, diversity, exposure, protection goals, and pesticides. Finally, the green keywords are associated with ERA in the context of climate change, encompassing climate change, vulnerability, and sea-level rise. These keyword classifications enhance the comprehension of the primary themes and research directions within the field while offering valuable insights into the knowledge structure and research trends for researchers. Figure 6 presents the distribution and interrelationships among these keywords, reinforcing their significance in distinct themes.
CiteSpace was utilised to perform cluster analysis, resulting in 11 clusters. The modularity Q of the clustered network is 0.8781, which suggests significant network associations within each cluster (Q > 0.3). The overall silhouette (S) value is 0.943, indicating reasonable intra-cluster similarity (0.5 < S < 1).
11 primary clusters were identified. These clusters are labelled with their indexed terms, such as “ERA”, “ecological risk”, “related ES supply”, “mellifera Bombus spp”, “non-native tree species”, “urban agglomeration”, “regional assessment,” “solitary bee”, “combined effect”, “sufficiency evaluation” and “mapping ES”. All clusters, except for cluster #0, demonstrate improved structure and more reasonable cluster similarity. Cluster #0 (ERA), cluster #1 (ecological risk), cluster #2 (related ES supply), and cluster #3 (mellifera bombus spp) are the most significant clusters, with cluster #0 being the largest of them all. Based on their average formation time, the most recently formed clusters are cluster #6 (urban agglomeration), cluster #2 (ecological risk), cluster #8 (regional assessment), and cluster #10. Conversely, cluster #2 (related ecosystem service supply) and cluster #3 (mellifera bombus spp) formed earlier (Table 2; Figure 7).
The evolution of citation clusters can be observed through the timeline graph (Figure 8). In this graph, the size of nodes corresponds to the citation count, and the connecting lines illustrate citation relationships. Initially, clusters #9 and #15 exhibited a prolonged absence of relevant publications, implying a decline in activity or the cessation of new research within these clusters during that period. Furthermore, cluster #0 had the longest duration, encompassing 2010 to 2021. This cluster demonstrates the closest relationship with other clusters, signifying its sustained activity throughout the research period. This cluster may represent a significant research direction or a field of high interest. Clusters #0, #1, and #3 have maintained activity for over a decade, underscoring their representation of enduring research topics. Conversely, cluster #7 is described as having the highest level of continuity, implying its status as a persistent research hotspot that garners sustained interest and citations. These observations facilitate our comprehension of the research dynamics and evolution within various clusters and identify which topics retain long-term activity.
The top 5 citations and referenced articles for the four most significant clusters (Cluster #0, Cluster #1, Cluster #2, and Cluster #3) are as follows:
• Cluster #0: “environmental risk assessment (ENRA)”
Cluster #0 is the largest cluster related to ESRA research. ENRA is a spatially explicit approach to assessing risks posed by human activities or interventions to human health and ecological receptors, such as animals, plants, or entire ecosystems. The endpoint of ENRA revolves around protecting the receptor, which includes ecological entities and their attributes. Among the literature closely related to this cluster, the most frequently cited authors are Nienstedt et al. (2012), Munns et al. (2016), Benford et al. (2016), Cardinale et al. (2012), and Faber et al. (2019), representing foundational research in this field. The top 5 cited references in this field are Benford et al. (2016), Devos et al. (2019), Streissl et al. (2018), Devos et al. (2016), and Brock et al. (2018), representing cutting-edge research in this field (Table 3).
• Cluster #1: “ecological risk”
The title of Cluster #1 is “ecological Risk” and refers to the potential adverse impacts on ecosystem structure and functioning caused by uncertain accidents or disasters in specific areas (Landis, 2003a). It signifies the negative effects of human activities and natural environmental changes on ecosystems (Depietri, 2020). Additionally, it highlights the potential of ecosystems to maintain their basic structure and functioning when faced with external disturbances (Renn, 2008). Research on this cluster has gained significant attention in recent years and has emerged as a prominent topic. Among the most cited literature in this cluster, Kang et al. (2018), Jin et al. (2019), Zhang et al. (2020), Wang et al. (2021), and Gong et al. (2021) represent the foundational research in this field. The top 5 cited references, Liu et al. (2022), Guo et al. (2022), Wang et al. (2022), Cui et al. (2022), and Pan et al. (2022), contribute to the forefront of research in this field (Table 4).
• Cluster #2: “related ES supply”
The main focus of Cluster #2 is to incorporate ES into the assessment of ecological risks, utilising ES for evaluation and valuation and using ES for deriving environmental quality standards to provide information for integrated human health and ecological risk assessment. Among the most relevant literature cited in this cluster, Galic et al. (2012), Faber and van Wensem (2012), Munns et al. (2017), Munns et al. (2009), and de Groot et al. (2010) are the most frequently cited, representing the research foundation in this field. Maltby. (2013), Kaiser et al. (2013), Apitz (2012), Galic et al. (2012), and Gret-Regamey et al. (2013) are the top 5 citing articles, representing the forefront of research in this field (Table 5).
• Cluster #3: “mellifera bombus spp”
Cluster #3 refers to Apis mellifera and Bombus spp. The research mainly focuses on using bees (Apis mellifera or Bombus spp.) as examples of service providers to illustrate how the proposed ESRA framework works in practice. Among the most relevant literature cited in this cluster, R Core Team (2022), Henry et al. (2012), Krupke et al. (2012), Potts et al. (2010), and Mommaerts et al. (2010) are the most frequently cited, representing the research foundation in this field. Sandrock et al. (2014), Fauser-Misslin et al. (2014), Luttik et al. (2012), Gill and Raine (2014), and Gill et al. (2012) are the top 5 citing articles, representing the forefront of research in this field (Table 6).
Literature with high centrality refers to literature frequently cited or co-cited by literature from diverse disciplines. These papers are potentially crucial to the research field, the intersection of multiple disciplines, or critical nodes in the evolution of various knowledge domains over time. Additionally, literature with high betweenness centrality implies their importance lies in their structure, implying that they play a crucial role in connecting other nodes or diverse clusters. The analysis revealed that the five highest-ranked articles with high betweenness centrality are presented in Table 7 and Figure 9. These papers can be considered as milestones within the ESRA field.
Citation bursts refer to literature with a sudden increase in citations over time, which to some extent represents the research hotspots and essential works of attention at that time. According to the results (Figure 10), the top five articles with the highest citation rates are Nienstedt et al. (2012), R Core Team (2022), Xu et al. (2016), Kang et al. (2018), and Cardinale et al. (2012). Among these articles is a technical software website, which may represent the widespread application of an important tool or resource in the research community. Additionally, two articles belong to the type of review opinions, indicating that papers with comprehensive summaries and theoretical viewpoints on a specific topic or field hold an essential citation position. Lastly, two articles are case study papers, suggesting that particular case studies have generated widespread interest and discussion in the relevant field.
Theme evolution analysis is employed to construct co-occurrence matrices, perform thematic clustering analysis, and generate maps of cluster strategy coordinates based on density and centrality. This analysis method assists in identifying research hotspots across different periods and predicts potential topics for research. Additionally, by evaluating the correlation among thematic clusters, a map showcasing the temporal evolution of issues can be created to illustrate the process of evolution. In conducting this analysis, SciMATv1.1.04 was utilised, with the following parameters configured: the frequency reduction threshold set to 1,1,1,1, the network reduction threshold set to 1,1,1,1, association strength as the measure of similarity in normalised networks, and a simple centrality algorithm employed for clustering. The g-index was used for bibliometric measurements, and the core document mapper was utilised for calculation. Regarding metrics in longitudinal mapping, the Jaccard and inclusion indexes were selected. These analytical tools and configurations aid researchers in gaining a comprehensive understanding of the dynamics and trends in evolution within their respective research fields.
Figure 11 presents the evolutionary paths of research topics in the ESRA field, revealing the relationships and critical evolutionary paths between different issues. This helps to better understand the knowledge structure and research trends in the field. Each node represents a clustered topic, and the node size is proportional to the number of relevant articles. The lines represent the evolutionary relationships between topics, where solid lines indicate that adjacent topics share primary keywords, typically core keywords, representing the mainstream evolution. Dashed lines indicate that they share minor keywords, representing branch evolution. The colour and thickness of the lines are proportional to the similarity between topics, with darker and thicker lines indicating higher association strength. The graph shows that new issues are generated at each time interval, reflecting the continuous development and evolution of research in the ESRA field. Among them, the topics “ES” and “adverse outcome pathway (AOP)" are highly correlated with other issues, indicating evolutionary relationships. A clear evolutionary path is from “ES” to “ES” → “ES”, indicating that this topic receives continuous attention throughout the entire period. Another crucial evolutionary path is from “ES” → “ES” → “social-ecological system” and “index.” This path illustrates the evolutionary relationships between topics related to social-ecological systems and indices. Finally, there are also several branch paths, such as “ES” → “ES → “biological invasion” → “genetically modified organism,” which indicate associations between minor keywords in the evolutionary process of the ESRA field.
The strategic coordinate diagram generated using SciMAT visually displays the evolutionary status of research topics within four-time intervals. In this graph, nodes represent topic clusters, and the numbers indicate the number of articles associated with each topic, with larger numbers indicating more popular topics. The horizontal axis of the coordinate map represents centrality, which denotes the strength of the connections between a topic and other topics. A higher centrality value indicates that a topic is more central in the research field and has closer relationships with different issues. The vertical axis represents density, showing the degree of association between keywords within a topic. Higher density signifies a tighter internal connection within the topic and suggests a more mature development. The plane is divided into different regions by four quadrants: the top-left quadrant contains mature but isolated topics, the bottom-left quadrant includes emerging or declining issues, the top-right quadrant represents driving issues that are well-developed and highly associated with other issues, and the bottom-right quadrant contains important yet unexplored issues that may be potential research hotspots.
Based on the observations from Figure 12, there are differences in the driving topics and topics with development potential across different periods. From 1994 to 2004, the driving topic was “ecosystem”, while issues with development potential included “ES”. From 2005 to 2012, the potential driving topic could be “estuary, " located at the far right of the vertical axis. Other driving topics included “natural enemy,” “pesticide residue,” and “invertebrate”, while issues with development potential included “honey bee” and “ES”. From 2013 to 2019, the driving topic was “Bt crops”, while issues with development potential had “ES”, “biological invasion”, “biomarkers”, “social-ecological system”, and “neonicotinoid” (based on centrality ranking). Lastly, from 2020 to 2023, the driving topics included “policy”, “trace metal”, “index”, “contaminant”, and “bioeconomic”, while issues with development potential included “ES”, “urban soil”, “sediment”, “genetically modified organisms”, “pollinator”, “multiple stressors”, and “ecological network”. These observations contribute to understanding the evolution of research topics and changes in research focus over different periods while providing clues for researchers to predict potential research hotspots.
From 1994 to 2023, the number of publications in the ESRA field fluctuated and peaked in 2022, showing an overall increasing trend. This indicates that research activities in this field are gradually increasing and growing over time. The overlapping thematic map (Figure 3) shows that the number of new keywords is always more significant than the lost keywords, suggesting that the research field is constantly evolving and progressing. The increase in the total number of keywords and the rate of keyword retention also reflects the growing maturity and substantial disciplinary inheritance in research directions. Based on the overall quantity of publications and the trend of keyword evolution, research in the ESRA field has great potential and provides a broad space for future development. Regarding active countries, China and the United States are the major contributors to the ESRA field, with many publications and close collaborative relationships with other countries in terms of authors or institutions. This may be due to the abundant ecosystem resources, robust scientific research capabilities, and shared concerns about global environmental issues in both countries. Active international collaborations contribute to the sharing of scientific knowledge and the resolution of global environmental issues. In terms of institutions, the Chinese Academy of Sciences from China ranks first in terms of citation quantity and cooperative networks. Other institutions do not correspond to the amount of publications or cooperative networks. For example, NOAA has a high citation quantity but infrequent collaborative networks, lacking prominent research networks. Overlaying the dual map of journals, ESRA research exhibits interdisciplinary characteristics, with universities, research institutions, and government departments integrating various disciplines such as landscape ecology, environmental toxicology, and geography, serving as the bridge to enhance collaboration among authors and institutions in different fields. Simultaneously, translating and applying research outcomes in different fields may encourage interdisciplinary cooperation to some extent.
VOSviewer identifies ES, landscape ERA, aquatic ERA, and ecosystem health as the four most relevant categories to ESRA. This study elucidates the formation of these co-words. Firstly, ES refer to ecosystems’ direct and indirect contributions to human wellbeing through supporting biodiversity (Bishop et al., 2010). The concept of ES has attracted attention in the regulatory and policy entities of landscape management and risk assessment (Maltby, 2013), and the impact of ES can currently be used as an assessment endpoint, linking ecological processes and ecological risk sources (Faber and van Wensem, 2012; Nienstedt et al., 2012). Keywords such as “biodiversity” and “climate change” are relevant. Secondly, since 2010, Landscape ERA has received significant attention, and related keywords become prominent in the third and fourth stages. Landscape ERA is considered an essential branch of ERA at the regional scale, focusing on the coupling and correlation between ecological processes and spatial patterns in landscape ecology. This approach emphasises the spatiotemporal heterogeneity of risk and scale effects, aiming to comprehensively represent multiple sources of risk and visualise their spatial distribution (Liu et al., 2020). Therefore, it can provide decision support and practical guidance for comprehensive risk prevention, optimisation, and management of regional landscape patterns. It is reasonable that related keywords frequently appear as ERAs increasingly focus on the regional level. Thirdly, the primary purpose of aquatic ERA is to address complex water resource management challenges related to population growth and climate change, notably metal pollution, one of the most severe and prominent issues in water bodies. Therefore, in risk assessments, metals in water and sediments of freshwater ecosystems have always been the focus of researchers’ attention (Rahman et al., 2014; Qu et al., 2016; Väänänen et al., 2018). This is why “heavy metal” and “toxicity” are the main keywords in the study. The fourth category primarily focuses on the ERA and ecosystem health. Ecosystems should provide diverse ES and maintain a high level of ecosystem health (Kang et al., 2018). A healthy ecosystem is considered the goal of ecological environmental management, emphasising ecosystem integrity and providing a basis for ecosystem assessment (Rapport et al., 1999). Current research focuses on building an ERA framework guided by ES and ecosystem health integration. The combination of ES and ecosystem health can be seen as the ideal endpoint of ERA (Kang et al., 2018). Therefore, “diversity,” “exposure,” and “protection goals” become the main keywords. Furthermore, significant climate change and its major impacts have become visible worldwide with rising sea levels. Global climate change is increasingly recognised by the scientific community, regulatory agencies, and the informed public as having long-term impacts on humans and ecosystems (Boko et al., 2007; West et al., 2009). The nature of climate change is a multiple-stressor process (Landis et al., 2013). Therefore, the main goal of climate change ERA is to conduct uncertainty prediction and assessment, focusing on the new climate and ecosystems generated under climate change conditions, followed by risk management and adaptation measures. Hence, “climate change” and “vulnerability” are the corresponding keywords.
To illustrate the citation situation, this article selected the top 5 cited references and citing articles from the four most significant clusters (#0, #1, #2, and #3) identified by CiteSpace. By reading the influential literature with high co-citation or citation rates, we determined each cluster’s research foundation and cutting-edge and organised their knowledge structure.
Cluster #0: “environmental risk assessment”
(1) Research Base
Risk assessment (RA) is a widely employed process that investigates and monitors risks associated with diverse activities, such as fossil fuel extraction, financial investments, and events like natural disasters (Aven, 2016). ENRA serves as a more specialised facet of RA, primarily focusing on evaluating potential environmental hazards presented by human activities to human wellbeing or ecological receptors. ENRA adopts a spatially explicit approach to assess the risks posed by human actions or intervention measures to human health and ecological receptors, encompassing fauna, flora, and entire ecosystems. The central objective of ENRA revolves around safeguarding receptors, including the entities and attributes of the ecological system. Although ENRA can quantify overall or cumulative risks, it generally overlooks risks at higher levels of ecological organisation. It does not endeavour to establish connections between ecological risks and human wellbeing through environmental and social welfare considerations (Barnthouse et al., 2007).
Justifiably, including ES in policy objectives or ascertaining the termination point of risk assessment is reasonable (Munns Jr and Rea, 2015). Accordingly, this study integrates traditional ENRA endpoints with ES into a significant cluster. This research cluster aims to explore opportunities for employing the concept of ES in environmental risk assessment and developing a framework based on the ES approach. Given the discernible interconnection between human wellbeing and the structure and processes of ecosystems, it is hypothesised to provide a more robust foundation for decision-making (Nienstedt et al., 2012; Munns Jr et al., 2016). Researchers primarily focus on incorporating ES into policy objectives or determining the termination point of risk assessment. The prevalent approach they adopt involves linking traditional ENRA endpoints with ES, where they establish a relationship between the supply of ES and biological and physical structures or Service Providing Units (SPUs) by developing ecosystem functioning species (Nienstedt et al., 2012; Benford et al., 2016). Preceding the conduct of ENRA, they explicitly define the assessment questions and identify relevant service-providing regions and SPUs based on varying landscapes and environmental concerns. These encompass specific species, habitats, environmental compartments, and other ecological entities vital for providing ES. One approach to establishing this connection is utilising ecosystem functioning species to assess specific ES, as these species can be associated with various ES like pollination, biological pest control, and nitrogen cycling (Faber et al., 2019).
(2) Research Frontier
ERA has undergone significant advancements. The most noteworthy evolution is the expansion of ERA from its initial focus on the impact of single pollutants to encompass the combined effects of multiple stressors (Benford et al., 2016). The research emphasis has gradually shifted from chemical pollution to non-chemical factors (Brock et al., 2018). Furthermore, the scope of assessment has broadened from local to regional and even global scales (Streissl et al., 2018). To comprehensively and scientifically evaluate the potential environmental impact of regulated products, extensive research has been conducted to enhance the methods and approaches of ERA, aiming to better account for multiple stress sources and environmental benefits (Devos et al., 2016). Some studies have outlined vital points, including the need for more precise definitions of environmental hazards, reliance on problem formulation to determine necessary information, adherence to quality standards to minimise errors and uncertainties, consideration of multiple stress factors and landscape characteristics to identify risk disparities under different environmental conditions (Streissl et al., 2018). It is stressed that ERA is an integral part of the regulatory decision-making process, and future decisions must strike a balance between risks and potential benefits.
The ES approach has gained wide acceptance in international scientific and risk assessment fields and is extensively employed by policymakers to safeguard biodiversity and ensure ecological sustainability (Devos et al., 2016; Munns Jr et al., 2016). This approach connects multiple stressors to a single ES (Syberg et al., 2017), establishes the relationship between biodiversity and ES (Devos et al., 2019), and considers the biophysical structures/processes and functions that dictate ES provision (Galic et al., 2012b; Munns Jr et al., 2016). Furthermore, challenges may arise when conducting ESRA due to changes in ES and its component definitions (Devos et al., 2019). Consequently, establishing standardised definitions for ES and their components is proposed to enhance the uniformity and universality of ERA processes and outcomes while addressing associated challenges concerning data and models (Brock et al., 2018). Suggestions have been made to establish standardised definitions for ES and its components, aiming to improve the uniformity and universality of ERA processes and outcomes. Additionally, researchers are examining strategies to tackle data and model-related challenges, including selecting relevant indicators, methods, and models, exploring the objectives of ecological risk assessments in different regions, improving the measurement and integration of cultural ESs, and assessing variations in ES provision.
• Cluster #1: “ecological risk”
(1) Research Base
Ecological risk refers to the risks faced by ecosystems and their components due to external disturbances and threats (Depietri, 2020). It represents the potential of ecosystems to maintain their fundamental structure and functionality when subjected to such disturbances (Renn, 2008). ERA helps us understand the unfavourable effects and extent of harm caused by external disturbances on ecosystem functions. Within this research cluster, a spatially oriented approach for regional ERA involves land use/land cover change assessment. Land is the primary indicator reflecting human impact on natural ecosystems (Yue et al., 2016). The land structure reflects urban development outcomes driven by environmental, socioeconomic, and policy factors, providing an appropriate scale to evaluate the impact of human activities on the environment. Land use change has been linked to various ecological issues, including land degradation, biodiversity loss, and ecological vulnerability (Bhattachan et al., 2018). Different types and intensities of land use have regional and cumulative effects on the ecological environment (Serveiss, 2002; Turner et al., 2013). Land use and land cover and their changes reflect the interaction between natural elements and human activities in the regional ecological environment. They significantly impact regional ecological processes (Fan et al., 2016). Land-use landscape types act as carriers of ecosystems, displaying notable spatial heterogeneity and offering insights into the structural characteristics and spatial variations of regional ecosystems. Numerous studies have explored the relationships among landscape pattern indices, ecological risk, and assessment methods (Tuholske et al., 2017; Jin et al., 2019). Currently, methods based on land use data and landscape indices are widely used (Zhang W. et al., 2020; Wang H. et al., 2021).
(2) Research Frontier
Currently, research in ERA has increasingly focused on regional-level analysis, employing regional environments as indicators for assessing ecological risks. This approach has gained significance in ecological and geographical research and is crucial in evaluating regional ecological risks. ERA involves assessing the direct and long-term damages or impacts on ecosystems caused by specific stress factors within a regional context (Chen et al., 2013). With the progress of global changes and ecological risk research, landscape ecological risk assessment based on land use and land cover changes has become mainstream. Studies on land use simulation and landscape ERA have emerged as prominent topics, offering practical tools for risk control and sustainable ecosystem management (Wu et al., 2016; Xing et al., 2020). The landscape, consisting of heterogeneous or mosaic spatial units comprising various ecosystem types, is an appropriate scale to examine the impact of human activities on the natural environment (Wang B. et al., 2020). Landscape pattern changes are deeply interconnected with disturbances and ecological processes across different scales within ecosystems (Kwon et al., 2021). The Landscape Ecological Risk Index (ERI) integrates multiple sources of risk arising from natural or human-induced disturbances (Zhang et al., 2021). It is primarily constructed based on landscape pattern indices to evaluate various potential factors and their cumulative levels of damage in landscape patches. Consequently, combining land use and land cover changes with ERI has been widely employed. Ongoing studies encompass diverse areas, such as wetlands (Hou et al., 2020; Xie et al., 2021), river basins (Gong et al., 2021; Cui et al., 2022), protected areas (Wang H. et al., 2021; Wang et al., 2022a), administrative regions (Gong et al., 2015; Liu et al., 2022), and urban agglomerations (Kang et al., 2018; Wang X. et al., 2021; Liu et al., 2022).
On the other hand, over time, changes in land use and land cover (LULC) can instigate instability in ecosystem structure and function, raising concerns about regional ecological security (Li et al., 2020). The alterations in LULC are closely associated with the level of ecological protection (Chai et al., 2017). The type and distribution of LULC directly impact ecosystem functions and values, as they disrupt the structure and processes of ecosystems (Peng et al., 2017), thereby directly or indirectly affecting the resources and services necessary for biological activities on Earth, consequently posing harm to ecosystem health (Huang et al., 2019) and leading to regional ecological risks. A mutually beneficial relationship exists between ERA and ecological security (Xiao and Chen, 2002). ERA examines and evaluates the degree of pressure and uncertainty confronting the ecological environment or regional ecosystems. It formulates strategies to mitigate risks and ensure ecological stability. Simultaneously, ecological security assessment evaluates the resilience of the ecological environment to maintain a safe state and development trajectory. This process aims to forestall unforeseen events while safeguarding and enhancing environmental integrity (Wang M.-E. et al., 2020). The scope of ERA encompasses two dimensions: an analysis of ecological environmental security and an assessment of ecological protection (CHEN Xing, 2005). Ecological environmental security analysis primarily focuses on the natural and social domains. In the realm of environmental analysis, it encompasses natural ecosystems, ecological landscapes, and ecological risks. The social domain contains politics, national affairs, diplomacy, economic globalisation, law, etc. By quantifying the effects of environmental risks, ERA establishes a scientific foundation for comprehensive ecological prevention and control strategies, providing compelling guidance for enhancing the framework of ecological security within the landscape.
• Cluster #2: “related ES supply”
(1) Research Base
In recent decades, significant efforts have been devoted to researching the assessment of supply and demand for ES (Costanza et al., 1997; Mensah et al., 2017). The supply of ES refers to the capacity of a specific area to provide particular ecosystem products and services within a defined timeframe. It encompasses utilising various natural resources and services (Burkhard et al., 2012). Within the current research cluster, relevant studies highlight the incorporation of ES as endpoints in ERA and their integration throughout various stages of risk assessment and management (Galic et al., 2012b), simultaneously analysing the diverse benefits they generate (Faber and van Wensem, 2012; Munns Jr et al., 2017). For instance, the conversion between ERA and natural resource damage assessment (NRDA) can be more direct. When considering ES, generic ecological assessment endpoints can be tailored to meet the decision support requirements specific to respective locations (Munns Jr et al., 2017). Furthermore, considering ES facilitates the integration of ecological risks with human health risks, resulting in a more comprehensive evaluation of potential hazards (Munns Jr et al., 2009). Additionally, the quantification, assessment, and valuation of these services have increasingly gained importance as research priorities (Munns Jr et al., 2009; Galic et al., 2012b), particularly in situations where endpoint risks cannot be directly assessed in ERA and must be inferred from changes in measured surrogate endpoints such as exposure and effects (Munns Jr et al., 2009).
(2) Research Frontier
The recognition of ES and the benefits derived by people from ecosystems within decision-making frameworks is becoming increasingly apparent. Individual or multiple stressors (e.g., chemicals (Maltby, 2013), sedimentation in aquatic ecosystems (Apitz, 2012), etc.) can result in adverse ecological impacts and diminish the provision of ES. There is an increasing adoption of the concept of ES among environmental policymakers; however, employing this approach in the protection, restoration, and management of ecosystems necessitates the development of novel understandings, tools, and frameworks. Understanding and predicting the impacts of single and multiple stressors on the provision of ES across varying spatial scales (ranging from local to global), devising indicators for quantifying and mapping service provisioning, assessing and managing risks to ES, establishing protection and restoration goals aligned with the desired type and level of services, and considering the life cycle of products and processes are of paramount importance (Apitz, 2012; Galic et al., 2012b; Maltby, 2013). Traditional risk assessments that concentrate on a single or a few related stressors (e.g., pollutants) are limited in evaluating the risks to various biotic and abiotic endpoints posed by multiple stressors; furthermore, they do not facilitate the assessment of both beneficial and detrimental effects that a specific group of stressors may have, contingent upon the endpoints, locations, and conditions (Apitz, 2012). Relevant research encompasses assessing the risks associated with the higher-level ecological organisation in ERA, utilising ecological models as instruments to address the necessary ecological complexity required for connecting measurement endpoints to ES and quantifying the adverse impacts that may emerge from the provisioning of services and human activities (Galic et al., 2012b). The science of assessing ES is progressing to tackle issues such as disregarding parameter uncertainty, which can influence decisions and result in the omission of crucial management aspects. Additional research suggests novel approaches for evaluating the uncertainties associated with multiple assessments of ES, including using Bayesian network model structures (Ayre and Landis, 2012).
• Cluster #3: “mellifera bombus spp”
(1) Research Base
Pollination agents play a vital role in global biodiversity, offering essential ES to cultivated and wild plants. Pollinating insects contribute significantly to ES for crops and wild plants, which are economically and ecologically critical. Nevertheless, global declines in pollination agents, especially bees (Apis mellifera, Bombus spp., and solitary bees), are occurring as a result of multiple driving factors, including the extensive use of insecticides for crop protection (Henry et al., 2012; Krupke et al., 2012). In this regard, pertinent literature has examined the potential causes of pollination agent decline, encompassing habitat loss and fragmentation (Carvell et al., 2006) as well as the use of agricultural chemicals (Henry et al., 2012; Krupke et al., 2012). The decline in pollination can potentially lead to the loss of valuable pollination services. This loss can significantly impact ecological and economic aspects, wild plant diversity, overall ecosystem stability, crop production, food security, and human wellbeing.
(2) Research Frontier
Crop pollination primarily depends on managed bee populations rather than solely on wild bee populations. However, in recent decades, there has been a decline in globally managed bee populations due to multiple factors, including diseases (Watanabe, 1994), pesticide use (Gill and Raine, 2014), and socio-economic factors (Meixner, 2010). As a result, the role of wild pollinators in crop production has become a research and conservation priority (Meixner, 2010). The concern regarding bees has led to ecological models focusing on the dynamics of bee populations and colonies and investigations into their decline’s specific causes and mechanisms (Becher et al., 2010).
The article with the highest betweenness centrality, according to CiteSpace, is a study by Henry et al. (2012), which has a betweenness centrality value of 0.14. Although this value is considerably smaller than values in other fields, it signifies the paper’s significant structural position. The research aims to elucidate the causes of declining bee populations attributable to insecticides (Henry et al., 2012). The extensive decline in wild and managed insect pollinators has severe implications for global ES and agricultural productivity. This body of literature serves as a foundation for agricultural ERA and the establishment of precise protection objectives for pesticide environmental risk assessment. Ranked second is Munns et al. (2016), who views ES as the endpoint of ERA and demonstrates that including ES endpoints can complement traditional ecological assessment endpoints by elucidating the benefits specific decisions bring to stakeholders and the public (Munns Jr et al., 2016). In a few other articles, two examine the primary pathways through which non-target arthropods are exposed and propose recommendations for their inclusion in risk assessments (Plant Protection Products and their Residues, 2014; Plant Protection Products and their Residues, 2015). Two additional articles concentrate on habitat ERA (Caro et al., 2020) and the effects of invasive species on ecosystems (Blackburn et al., 2014). The majority of the articles accurately address the endeavours to integrate ES as evaluation endpoints to enhance the assessment process, encompassing ecological entities and attributes. Entities encompass the structure of ES providers and the ecological functions they engage in, while details encompass the respective functionalities and services of these unit entities (Munns Jr et al., 2009). The research emphasises integrating the ES concept into ERA and employing them as evaluation endpoints to delineate the protection targets and attributes explicitly. For researchers seeking a comprehensive understanding of the most impactful literature in this field, it is advisable to peruse the aforementioned articles. Nevertheless, for this particular study, the highest betweenness centrality is a mere 0.14, illustrating feeble associations among research objects within this field. As the research field progresses, this value may rise or be supplanted by other prominent articles in the future due to the generally low betweenness centrality.
Examining the top 5 articles in Figure 10 based on citation bursts. The ESRA field mainly includes the second, third, and fourth phases. The article with the highest burst value is by Nienstedt et al. (2012), with a burst value of 11.67. This literature utilises the concept of ES to derive specific protection goals for assessing environmental risks caused by pesticides (Nienstedt et al., 2012). Another article with a high burst value is by R Core Team (2022), appearing primarily in the fourth phase. Researchers often use the R statistical computing language and software for ES correlation analysis, assessment, and data visualisation (R Core Team, 2022). Xu et al. (2016) developed an interdisciplinary approach and established an ESRA model to assess comprehensive degradation risks in the Taihu Basin in China. This approach integrates remote sensing and field monitoring data and combines land-use and ecological models to obtain key indicators of crucial environmental factors (Xu et al., 2016). This citation has been widely referenced from 2019 to 2021, reflecting the formalisation of the field at this stage. In the 2020–2023 phase, the article by Kang,et al. (2018) has a high burst value reaching 6.78. The study establishes an ecological-driven ERA framework by integrating ES and ecosystem health. To achieve this, an interdisciplinary approach was developed to comprehensively assess the degradation risks of regional-scale ES and ecosystem health (Kang et al., 2018). As for the phase from 2013 to 2017, the article with a high burst value is by Cardinale et al. (2012), investigating the impact of biodiversity loss on ecosystem functionality and its ability to provide necessary goods and services for social prosperity (Cardinale et al., 2012). The progression of studies signifies the development of ERA from fundamental integrated research towards a focus on regional ERA.
According to the thematic evolution map produced by SciMAT (Figure 11), there are mainly two paths in the evolution of this field. One is focused on the theme of “ES,” indicating the most significant number of publications on this theme. Many new themes emerged during this period related to the evolution of “ES,” showing the continuous improvement of research over time. The other evolutionary path is related to “social-ecological systems”. To fully utilise ES in ecological risk management communication, researchers have attempted to incorporate ESs into the ecological risk management framework using the driving forces-pressures-state-impacts-responses (DPSIR) theory (Atkins et al., 2011; Lozoya et al., 2011; Cooper, 2013). Research on landscape ES and risks has been conducted based on landscape ecology pattern processes and other theories (Davis et al.). It covers many studies and is a significant focus in ESRA’s third and fourth stages. Besides the main evolutionary paths, the field of study also shows a broader trend. In each period, new themes emerge that evolve from other themes, especially in the fourth stage, where multiple themes such as “policy,” “contaminant,” and “pollinator” appear. This indicates that the research explores multiple directions, including ecosystem health, habitat ERA, and chemical ERA.
Based on the strategic coordinate map produced by SciMAT (Figure 12), “ES” has always been closely related to other themes in this field, which is consistent with the evolution map. However, its development is insufficient, indicating significant potential for further research in this area. The theme of “honey bee” showed potential from 2005 to 2012. “Bt crops” appeared in the upper-right quadrant from 2013 to 2019, receiving attention in the context of transgenic environmental risk assessment for Bacillus thuringiensis (Bt) toxin transgenic crops and biological control (Skinner et al., 2014). It recently published significant related research nodes, connecting widely with other fields. “Biological invasion” became the most central driving theme outside of ES in the period from 2013 to 2019. At present, regional ERA is extensively employed in the biological invasion risk assessment, offering a broader perspective encompassing regional elements, sources, habitats, and impacts (Cadotte et al., 2006; Hulme et al., 2008; Goldsmit et al., 2019). Additionally, “social ecological systems” is the driving theme from 2013 to 2019 and closely related to other topics, showing good research progress. One can focus on literature from this period if one wants to gain a deeper understanding of ERA based on landscape ES.
This paper summarises the research literature of ESRA from 1994 to 2023 using CiteSpace, VOSviewer, and SciMAT, providing a quantitative and intuitive evaluation of the academic achievements and progress in this field. By presenting a knowledge map, the evolution of the knowledge structure in this field from macro to micro levels is demonstrated, further exploring potential research trends. This addresses a core issue in previous research in this field, where previous studies analysed ESRA from multiple perspectives but did not reveal the overall changes over time. By studying the research trends in the field, the development and research directions of ESRA during its evolution can be tracked. This paper analyses the entire developmental history of ESRA research, providing support for a more scientific and accurate prediction of its future development. Therefore, it also offers guidance for decision-makers and provides in-depth insights into the future growth of this field.
Our analyses reveal four distinct phases of development in ESRA research, from the initial developmental phase to one marked by global collaboration and policy application. The most recent phase (2019–2023) witnessed a significant surge in publications and research diversification, with a particular emphasis on the application of ES concepts to environmental risk assessment. ERA has changed dramatically over the years, transitioning from traditional approaches to regional and landscape-based assessments. One of the most notable findings has been the growing emphasis on integrating ES into ERA. This integration helps to go beyond traditional limitations and provide a more comprehensive protection of the environment. ES is considered as the bridge between human wellbeing and ecosystem processes. An increasing number of ERA consider the introduction of ES or the pathways of adverse ES. ERA is commonly used to assess the effectiveness of environmental protection. It is a scientific method used to evaluate the potential risks and consequences of negative impacts caused by human activities and climate change. Based on the overall development trend of the complete text, the development of ERA exhibits changes over time characterised by traditional, regional, and landscape eras.
The core elements of ERA studies include ES, land use and climate change. Over time, the scope of ERA has evolved from studying natural processes to considering the impacts of human activities. Traditional methods have been replaced by regional and landscape approaches, and LANDSCAPE ERA has become an important subfield and landscape ERA has become an important branch of environmental, social and ecological impact assessment. Landscape ERA is based on the theory of landscape ecology and requires a comprehensive assessment of the cumulative impacts of landscape change on human wellbeing. Landscape patterns and composition play a key role in the provision of ES, making landscape ecology a key component of ESRA research. Landscape ecology combines the broad principles of geographical ecology and the macro-scale methods of ecology, facilitating the integration of spatial heterogeneity in geography and correlation in ecological studies (Peng et al., 2015). The integration of geographical ecological processes on a regional scale has emerged as a pivotal concern in ecological risk investigation (Wang et al., 2023). This impetus has prompted the exploration of ecological risk models and the subsequent development of risk management strategies, thereby shaping the trajectory of ecological risk research. To delve into this matter comprehensively, the research focus has transitioned from singular ecological systems to a broader regional outlook. This shift underscores an emphasis on the spatial arrangement of diverse ecological types, marking a transition to the landscape-oriented era. In this landscape era, the emphasis lies in commencing analysis from the perspective of landscape elements, the evolutionary patterns they undergo, and the amalgamation of ecological processes (Wang et al., 2023). This analytical approach is aimed at understanding how these landscapes respond to internal vulnerabilities and external perturbations, with the ultimate goal of assessing or predicting the influence of human activities or natural calamities on the composition, structure, functionality, and processes of specific regional landscapes (Wei et al., 2020). In terms of assessment methods, implementing a standardised framework has introduced the genesis of two principal approaches: source-sink analysis and landscape pattern analysis (Gong et al., 2021; Yan et al., 2021). The source-sink research adheres to a structured framework that entails the sequential processes of “identifying sources of risk and stress factors, analysing receptors of risk, establishing ecological endpoints, and conducting assessments of exposure and hazard”. This method is particularly well-suited for evaluating regional ecological stress while maintaining clear objectives (Shirvani et al., 2019). Conversely, landscape pattern analysis does not confine itself to specific pressure factors, instead examining broader disruption and pressure influences. It broadens the spectrum of risk receptors from individual ecological components to comprehensive indicators that encompass spatial heterogeneity. Furthermore, it incorporates changes in land use and land cover as factors impacting ecological risk, investigating their spatial configuration, dynamic alterations, and the dispersion of associated risks.
Furthermore, as mentioned earlier, the ERA approach integrates geographic and ecological processes. It focuses on the spatial and temporal heterogeneity of specific regional ecological risks, combining environmental and geographical processes. However, there are limitations in the spatial and temporal scales of ESRA assessments in practical application. For example, interpreting the impact of stressors on service-providing units and the delivery of ES across multiple spatial and temporal scales may be constrained by scale issues (Committee, 2016; Devos et al., 2019). Additionally, specific semi-quantitative approaches may rely heavily on expert judgment (Munns Jr et al., 2016), and the application of weights may also pose challenges. Assuming equal weights for ES correlations (e.g. (Cabral et al., 2015)) is not always representative, as their importance may vary in different contexts (Xu et al., 2016).
Lastly, ESRA faces challenges such as data availability (Cabral et al., 2015; Caro et al., 2020), complexity and high data requirements (Maltby et al., 2018; Xing et al., 2020), and uncertainties (Xu et al., 2016; Forbes et al., 2019). These uncertainties can arise from the data used (Galic et al., 2012b; Pártl et al., 2017) and the assumptions and models employed (Galic et al., 2012b). Additionally, quantitative and conceptual models describing the relationships between human activities, pressures, and ES need to be verified (Forbes et al., 2017). This study shows that interdisciplinary approaches that draw on ecology, remote sensing and numerical modelling are essential to further advance PES. Innovative quantitative methods that address the complexity of data analysis should be developed. Combining computational science, artificial intelligence algorithms and economic and statistical modelling can help to address the intricacies of linkages in ecosystem service assessments.
Firstly, due to the close connection between ES and human wellbeing, incorporating the concept of ES into ERA can alleviate some traditional constraints (Schäfer et al., 2012). For example, Munns et al. argue that ES can serve as the “endpoint of assessment” in ERA (Munns Jr et al., 2016), thus achieving more comprehensive environmental protection and helping to clarify the benefits of environmental decision-making, policies, and actions. In the future, further development of ESRA theory and analytical frameworks is needed to implement comprehensive assessments that not only focus on the impact linkage of ES but also consider the condition of ecosystems (Xu et al., 2016), such as maintaining a high level of ecosystem health (Kang et al., 2018). In regional ERA, the endpoint of assessment should integrate ES with ecosystem health.
Secondly, future assessment methods focus on the holistic ES system, studying multiple ES systems and favouring integrated assessment frameworks. Therefore, in terms of methodology, ESRA needs to adopt interdisciplinary approaches, drawing insights from disciplines such as ecology, remote sensing, and numerical simulation. Ecological models have great potential in addressing the challenges of ERA by linking measurement endpoints with ES through inherent ecological complexity. With the exponential growth of ES research and advanced techniques in ES classification, assessment, and mapping, future research needs to fully explore the linkage between ecological mechanisms and the creation of ES final products (Wong et al., 2015). Furthermore, it is necessary to quantify the adverse impacts of service provision and human activities, such as urbanisation (Kang et al., 2018). Therefore, there is a need for improvement in modelling methods to overcome the limitations of current ERA approaches. Complex risk assessment methods have been proposed, such as PETAR (Risk Ecological Stratified Assessment Procedure) (Moraes and Molander, 2004), WOE (Weight of Evidence) (Landis, 2003b), RRM model (Chen et al., 2012), and Bayesian network methods (Ayre and Landis, 2012). For instance, BN also have great potential for climate change impact assessment and can capture uncertainty and compare the influence of multiple pressures on valuable resources (Carriger et al., 2016; Sperotto et al., 2017).
Moreover, for large-scale socio-ecological systems, traditional ERA requires a large amount of long-term monitoring data. Existing assessment methods, such as relative risk assessment, can better address the quantitative and semi-quantitative issues in large-scale risk assessment but pose significant challenges in the unified representation of multiple risk sources in the spatial domain and understanding the spatial interaction between risk sources and receptors. Landscape spatial patterns and compositions largely determine the supply of ES, and the changes in landscape patterns and processes caused by high-intensity socio-economic activities may affect the output of ES types. In recent years, with the development of geographic information technology and landscape pattern-process theory, the evaluation of landscape ES has made significant progress, and there will be more attempts to apply landscape ES to socio-ecological system ecological risk management. The ESRA, grounded in landscape ecology theory, demands a holistic assessment of the cumulative influence of landscape changes on human welfare, surpassing the confines of current or ongoing risk incidents. There is also a requisite for research to shift its focus towards forthcoming ecological risk trends (Gong et al., 2021).
The advent of the information age and the proliferation of big data have engendered novel technologies and interdisciplinary tools, ushering in inventive techniques for ESRA research. For instance, the widespread adoption of open-source statistical software R fosters cross-disciplinary cooperation within the academic realm (R Core Team, 2022). The amalgamation of computer science and artificial intelligence algorithms, like Artificial Neural Network (ANN) models, enables the handling of intricate non-linear associations and delivers precise outcomes (Jiang et al., 2013). Economic and statistical models augment the intricacies of data analysis (Desquilbet et al., 2021; Tredennick et al., 2021). It is imperative to amalgamate interdisciplinary strategies, cultivate inventive quantitative methodologies for ESRA, and examine ecosystem multifunctionality while being mindful of existing methodologies’ virtues, constraints, and mathematical underpinnings. Looking ahead, the development of groundbreaking spatial assessment technologies that cater to practical exigencies, coupled with indicators and evaluation techniques linked to human wellbeing, will be crucial. This progress will reinforce the interdisciplinary deployment of 3S technologies (Geographic Information Systems, Global Positioning Systems, Remote Sensing), statistics, and spatial analysis.
There are some limitations in this study. First and foremost, we relied solely on the SCI-E and SSCI publications in the WOS core collection database for data collection, without including other databases such as Scopus and Google Scholar, which may limit the breadth of the research sample. Future studies could consider integrating multiple databases to validate the reliability of the research findings. This analysis only taught English articles, excluding papers in other languages. Secondly, in analysing the scientific knowledge graph, we only used the LLR algorithm for cluster analysis. However, combining multiple association analysis algorithms may help improve accuracy. In addition, this study focused on the analysis and interpretation of critical clusters, with limitations in terms of space, and did not delve into non-focus clusters in depth. Finally, while identifying research frontiers and future development trends through software analysis has objectivity, it still requires further reading and meticulous organisation of literature to obtain more accurate conclusions.
Employing bibliometric techniques, along with qualitative and quantitative analysis, this study assesses the focal points, cutting-edge domains, and prospective trajectories within ESRA research spanning the last three decades (1994–2023). The findings of this investigation furnish a conceptual underpinning for forthcoming research endeavours in the realm of ESRA and further offer insights that can aid managers and decision-makers in their pursuit of adept management, informed decision-making, and safeguarding efforts. Ultimately, this contributes to the advancement of ecological sustainability and human welfare. In summation, our study yields the subsequent deductions:
• The research in this field can be divided into four stages: from 1994 to 2005, the initial development stage; from 2006 to 2012, the expansion and application stage; from 2013 to 2019, the global cooperation and policy application stage; and from 2019 to 2023, the publication quantity grows the fastest, and the research deepens and diversifies rapidly. According to the publication trend, people are paying increasing attention to the application of the concept of ES in environmental risk assessment, the development trend of ERA has transformed traditional methods into regional and landscape approaches.
• The primary content of ESRA research involves ES, land use, and climate change. Key themes identified in ESRA research include ES, landscape ERA, aquatic ERA and ecosystem health. With the development of recent decades, the scope of ERA has gradually expanded from considering natural processes to the impacts caused by human activities. The research methods and directions of ESRA have undergone significant evolution, now placing more emphasis on spatial arrangement, landscape elements, and ecological processes. Landscape ERA has become an essential branch of ESRA research.
• The citation burst analysis identified key literature with highly cited references that play an essential role at different stages of the ESRA field. This included various topics ranging from environmental risk assessment to ecosystem health and social-ecological systems. This finding highlights the diverse and multifaceted nature of ESRA research and its evolution across multiple fields and timeframes; using the SciMAT tool to produce a thematic evolution map and a strategic coordinate map, the study found that ES and landscape ecological systems were the two core themes. In contrast, the other themes covered various directions, from policy to ERA. This suggests that research in the field of ESRA has begun to explore multiple key aspects, providing a more holistic perspective on addressing ecosystem risk.
• ESRA aims to incorporate ES into ecological assessment better. However, there are still many challenges in combining it with ERA. Further exploration and improvement of ESRA theories and analytical frameworks are still needed, especially in modelling approaches, interdisciplinary strategies, and the use of advanced spatial assessment techniques.
• Future ESRA research needs to focus on the overall ES and interdisciplinary method applications. The combination of ecological models and advanced technologies may provide approaches to address issues in ESRA. At the same time, big data and informatisation provide new research tools and methods for ESRA, but integration and innovation are needed to meet practical needs.
LQ: Conceptualization, Data curation, Funding acquisition, Software, Visualization, Writing–original draft. XZ: Data curation, Formal Analysis, Supervision, Writing–original draft. YD: Supervision, Validation, Visualization, Writing–review and editing. LP: Supervision, Validation, Visualization, Writing–review and editing.
The author(s) declare financial support was received for the research, authorship, and/or publication of this article. This research was funded by the National Social Science Foundation in Art, PRC, grant number 22BG117, and this work was supported by Qing Lan Project in Jiangsu Province.
The authors declare that the research was conducted in the absence of any commercial or financial relationships that could be construed as a potential conflict of interest.
All claims expressed in this article are solely those of the authors and do not necessarily represent those of their affiliated organizations, or those of the publisher, the editors and the reviewers. Any product that may be evaluated in this article, or claim that may be made by its manufacturer, is not guaranteed or endorsed by the publisher.
Apitz, S. E. (2012). Conceptualizing the role of sediment in sustaining ecosystem services: sediment-ecosystem regional assessment (SEcoRA). Sci. Total Environ. 415, 9–30. doi:10.1016/j.scitotenv.2011.05.060
Aria, M., and Cuccurullo, C. J. J. (2017). bibliometrix: an R-tool for comprehensive science mapping analysis. J. Informetr. 11 (4), 959–975. doi:10.1016/j.joi.2017.08.007
Asmus, M. L., Nicolodi, J., Anello, L. S., and Gianuca, K. (2019). The risk to lose ecosystem services due to climate change: a South American case. Ecol. Eng. 130, 233–241. doi:10.1016/j.ecoleng.2017.12.030
Assessment, M. E. (2005). Ecosystems and human well-being: wetlands and water. United States: World resources institute.
Atkins, J. P., Burdon, D., Elliott, M., and Gregory, A. J. (2011). Management of the marine environment: integrating ecosystem services and societal benefits with the DPSIR framework in a systems approach. Mar. Pollut. Bull. 62 (2), 215–226. doi:10.1016/j.marpolbul.2010.12.012
Aven, T. (2016). Risk assessment and risk management: review of recent advances on their foundation. Eur. J. Operational Res. 253 (1), 1–13. doi:10.1016/j.ejor.2015.12.023
Ayre, K. K., and Landis, W. G. (2012). A Bayesian approach to landscape ecological risk assessment applied to the Upper Grande Ronde Watershed, Oregon. Hum. Ecol. Risk Assess. An Int. J. 18 (5), 946–970. doi:10.1080/10807039.2012.707925
Barnthouse, L. W., Munns, W. R., and Sorensen, M. T. (2007). Population-level ecological risk assessment. United States: CRC Press.
Becher, M. A., Hildenbrandt, H., Hemelrijk, C. K., and Moritz, R. F. (2010). Brood temperature, task division and colony survival in honeybees: a model. Ecol. Model. 221 (5), 769–776. doi:10.1016/j.ecolmodel.2009.11.016
Benford, D., Halldorsson, T., Hardy, A., Jeger, M. J., Knutsen, K. H., More, S., et al. (2016). Guidance to develop specific protection goals options for environmental risk assessment at EFSA, in relation to biodiversity and ecosystem services. Efsa J. 14 (6), 50. doi:10.2903/j.efsa.2016.4499
Bhattachan, A., Emanuel, R. E., Ardon, M., Bernhardt, E. S., Anderson, S. M., Stillwagon, M. G., et al. (2018). Evaluating the effects of land-use change and future climate change on vulnerability of coastal landscapes to saltwater intrusion. Elem. Sci. Anth 6, 62. doi:10.1525/elementa.316
Birkle, C., Pendlebury, D. A., Schnell, J., and Adams, J. J. Q. S. S. (2020). Web of Science as a data source for research on scientific and scholarly activity. Web Sci. as a data source Res. Sci. Sch. activity 1 (1), 363–376. doi:10.1162/qss_a_00018
Bishop, J., Brink, P. t., Gundimeda, H., Kumar, P., Nesshöver, C., Schröter-Schlaack, C., et al. (2010). The economics of ecosystems and biodiversity: mainstreaming the economics of nature: a synthesis of the approach, conclusions and recommendations of TEEB. Available at: https://wedocs.unep.org/20.500.11822/7851.
Blackburn, T. M., Essl, F., Evans, T., Hulme, P. E., Jeschke, J. M., Kühn, I., et al. (2014). A unified classification of alien species based on the magnitude of their environmental impacts. PLoS Biol. 12 (5), e1001850. doi:10.1371/journal.pbio.1001850
Boko, M., Niang, I., Nyong, A., Vogel, A., Githeko, A., Medany, M., et al. (2007). Africa climate change 2007: impacts, adaptation and vulnerability: contribution of working group II to the fourth assessment report of the intergovernmental panel on climate change. Cambridge: Cambridge University Press.
Brock, T., Bigler, F., Frampton, G., Hogstrand, C., Luttik, R., Martin-Laurent, F., et al. (2018). Ecological recovery and resilience in environmental risk assessments at the European Food Safety Authority. Integr. Environ. Assess. Manag. 14 (5), 586–591. doi:10.1002/ieam.4079
Burkhard, B., Kroll, F., Nedkov, S., and Müller, F. (2012). Mapping ecosystem service supply, demand and budgets. Ecol. Indic. 21, 17–29. doi:10.1016/j.ecolind.2011.06.019
Cabral, P., Levrel, H., Schoenn, J., Thiébaut, E., Le Mao, P., Mongruel, R., et al. (2015). Marine habitats ecosystem service potential: a vulnerability approach in the Normand-Breton (Saint Malo) Gulf, France. Ecosyst. Serv. 16, 306–318. doi:10.1016/j.ecoser.2014.09.007
Cadotte, M. W., Murray, B. R., and Lovett-Doust, J. (2006). Ecological patterns and biological invasions: using regional species inventories in macroecology. Biol. Invasions 8, 809–821. doi:10.1007/s10530-005-3839-4
Cardinale, B. J., Duffy, J. E., Gonzalez, A., Hooper, D. U., Perrings, C., Venail, P., et al. (2012). Biodiversity loss and its impact on humanity. Nature 486 (7401), 59–67. doi:10.1038/nature11148
Caro, C., Marques, J. C., Cunha, P. P., and Teixeira, Z. (2020). Ecosystem services as a resilience descriptor in habitat risk assessment using the InVEST model. Ecol. Indic. 115, 106426. doi:10.1016/j.ecolind.2020.106426
Carriger, J. F., Barron, M. G., and Newman, M. C. (2016). Bayesian networks improve causal environmental assessments for evidence-based policy. Environ. Sci. Technol. 50 (24), 13195–13205. doi:10.1021/acs.est.6b03220
Carvell, C., Roy, D. B., Smart, S. M., Pywell, R. F., Preston, C. D., and Goulson, D. (2006). Declines in forage availability for bumblebees at a national scale. Biol. Conserv. 132 (4), 481–489. doi:10.1016/j.biocon.2006.05.008
Chai, J., Wang, Z., and Zhang, H. (2017). Integrated evaluation of coupling coordination for land use change and ecological security: a case study in Wuhan City of Hubei Province, China. Int. J. Environ. Res. Public Health 14 (11), 1435. doi:10.3390/ijerph14111435
Chen, Q., Liu, J., Ho, K. C., and Yang, Z. (2012). Development of a relative risk model for evaluating ecological risk of water environment in the Haihe River Basin estuary area. Sci. Total Environ. 420, 79–89. doi:10.1016/j.scitotenv.2011.09.044
Chen, S., Chen, B., and Fath, B. D. (2013). Ecological risk assessment on the system scale: a review of state-of-the-art models and future perspectives. Ecol. Model. 250, 25–33. doi:10.1016/j.ecolmodel.2012.10.015
Chen Xing, Z. C. (2005). Review of the studies on ecological security. Prog. Geogr. 24 (6), 8–20. doi:10.11820/dlkxjz.2005.06.002
Cobo, M. J., López-Herrera, A. G., Herrera-Viedma, E., and Herrera, F. (2012). SciMAT: a new science mapping analysis software tool. J. Am. Soc. Inf. Sci. Technol. 63 (8), 1609–1630. doi:10.1002/asi.22688
Committee, E. S. (2016). Guidance to develop specific protection goals options for environmental risk assessment at EFSA, in relation to biodiversity and ecosystem services. Efsa J. 14 (6), e04499. doi:10.2903/j.efsa.2016.4499
Cooper, P. (2013). Socio-ecological accounting: DPSWR, a modified DPSIR framework, and its application to marine ecosystems. Ecol. Econ. 94, 106–115. doi:10.1016/j.ecolecon.2013.07.010
Costanza, R., d'Arge, R., De Groot, R., Farber, S., Grasso, M., Hannon, B., et al. (1997). The value of the world's ecosystem services and natural capital. nature 387 (6630), 253–260. doi:10.1038/387253a0
Cui, B., Zhang, Y., Wang, Z., Gu, C., Liu, L., Wei, B., et al. (2022). Ecological risk assessment of transboundary region based on land-cover change: a case study of gandaki river basin, himalayas. Land 11 (5), 638. doi:10.3390/land11050638
Daily, G. C. (1997). Introduction: what are ecosystem services[J]. Nature’s services: Societal dependence on natural ecosystems 1 (1).
de Groot, R. S., Alkemade, R., Braat, L., Hein, L., and Willemen, L. (2010). Challenges in integrating the concept of ecosystem services and values in landscape planning, management and decision making. Ecol. Complex. 7 (3), 260–272. doi:10.1016/j.ecocom.2009.10.006
De Lange, H., Sala, S., Vighi, M., and Faber, J. H. (2010). Ecological vulnerability in risk assessment—a review and perspectives. Sci. total Environ. 408 (18), 3871–3879. doi:10.1016/j.scitotenv.2009.11.009
Depietri, Y. (2020). The social–ecological dimension of vulnerability and risk to natural hazards. Sustain. Sci. 15 (2), 587–604. doi:10.1007/s11625-019-00710-y
Desquilbet, M., Cornillon, P.-A., Gaume, L., and Bonmatin, J.-M. (2021). Adequate statistical modelling and data selection are essential when analysing abundance and diversity trends. Nat. Ecol. Evol. 5 (5), 592–594. doi:10.1038/s41559-021-01427-x
Devos, Y., Gaugitsch, H., Gray, A. J., Maltby, L., Martin, J., Pettis, J. S., et al. (2016). Advancing environmental risk assessment of regulated products under EFSA's remit. EFSA J. 14, e00508. doi:10.2903/j.efsa.2016.s0508
Devos, Y., Munns, W. R., Forbes, V. E., Maltby, L., Stenseke, M., Brussaard, L., et al. (2019). Applying ecosystem services for pre-market environmental risk assessments of regulated stressors. EFSA J. 17, e170705. doi:10.2903/j.efsa.2019.e170705
Donthu, N., Kumar, S., Mukherjee, D., Pandey, N., and Lim, W. M. (2021). How to conduct a bibliometric analysis: an overview and guidelines. J. Bus. Res. 133, 285–296. doi:10.1016/j.jbusres.2021.04.070
Faber, J. H., Marshall, S., Van den Brink, P. J., and Maltby, L. (2019). Priorities and opportunities in the application of the ecosystem services concept in risk assessment for chemicals in the environment. Sci. Total Environ. 651, 1067–1077. doi:10.1016/j.scitotenv.2018.09.209
Faber, J. H., and van Wensem, J. (2012). Elaborations on the use of the ecosystem services concept for application in ecological risk assessment for soils. Sci. Total Environ. 415, 3–8. doi:10.1016/j.scitotenv.2011.05.059
Falagas, M. E., Pitsouni, E. I., Malietzis, G. A., and Pappas, G. J. T. F. j. (2008). Comparison of PubMed, Scopus, Web of science, and Google scholar: strengths and weaknesses. Google scholar strengths weaknesses 22 (2), 338–342. doi:10.1096/fj.07-9492lsf
Fan, J., Wang, Y., Zhou, Z., You, N., and Meng, J. (2016). Dynamic ecological risk assessment and management of land use in the middle reaches of the heihe river based on landscape patterns and spatial statistics. Sustainability 8 (6), 536. doi:10.3390/su8060536
Fauser-Misslin, A., Sadd, B. M., Neumann, P., and Sandrock, C. (2014). Influence of combined pesticide and parasite exposure on bumblebee colony traits in the laboratory. J. Appl. Ecol. 51 (2), 450–459. doi:10.1111/1365-2664.12188
Forbes, V. E., Calow, P., Grimm, V., Hayashi, T., Jager, T., Palmqvist, A., et al. (2010). Integrating population modeling into ecological risk assessment. Lincoln: University of Nebraska.
Forbes, V. E., Railsback, S., Accolla, C., Birnir, B., Bruins, R. J., Ducrot, V., et al. (2019). Predicting impacts of chemicals from organisms to ecosystem service delivery: a case study of endocrine disruptor effects on trout. Sci. Total Environ. 649, 949–959. doi:10.1016/j.scitotenv.2018.08.344
Forbes, V. E., Salice, C. J., Birnir, B., Bruins, R. J., Calow, P., Ducrot, V., et al. (2017). A framework for predicting impacts on ecosystem services from (sub) organismal responses to chemicals. Environ. Toxicol. Chem. 36 (4), 845–859. doi:10.1002/etc.3720
Galic, N., Schmolke, A., Forbes, V., Baveco, H., and van den Brink, P. J. (2012a). The role of ecological models in linking ecological risk assessment to ecosystem services in agroecosystems. Sci. Total Environ. 415, 93–100. doi:10.1016/j.scitotenv.2011.05.065
Galic, N., Schmolke, A., Forbes, V., Baveco, H., and van den Brink, P. J. (2012b). The role of ecological models in linking ecological risk assessment to ecosystem services in agroecosystems. Sci. Total Environ. 415, 93–100. doi:10.1016/j.scitotenv.2011.05.065
Garfield, E., Sher, I. H., and Torpie, R. J. (1964). The use of citation data in writing the history of science. Institute for Scientific Information Inc Philadelphia PA.
Gill, R. J., and Raine, N. E. (2014). Chronic impairment of bumblebee natural foraging behaviour induced by sublethal pesticide exposure. Funct. Ecol. 28 (6), 1459–1471. doi:10.1111/1365-2435.12292
Gill, R. J., Ramos-Rodriguez, O., and Raine, N. E. (2012). Combined pesticide exposure severely affects individual-and colony-level traits in bees. Nature 491 (7422), 105–108. doi:10.1038/nature11585
Goldsmit, J., McKindsey, C., Archambault, P., and Howland, K. L. (2019). Ecological risk assessment of predicted marine invasions in the Canadian Arctic. PloS one 14 (2), e0211815. doi:10.1371/journal.pone.0211815
Gong, J., Cao, E., Xie, Y., Xu, C., Li, H., and Yan, L. (2021). Integrating ecosystem services and landscape ecological risk into adaptive management: insights from a western mountain-basin area, China. J. Environ. Manag. 281, 111817. doi:10.1016/j.jenvman.2020.111817
Gong, J., Yang, J., and Tang, W. (2015). Spatially explicit landscape-level ecological risks induced by land use and land cover change in a national ecologically representative region in China. Int. J. Environ. Res. public health 12 (11), 14192–14215. doi:10.3390/ijerph121114192
Grêt-Regamey, A., Brunner, S. H., Altwegg, J., and Bebi, P. (2013). Facing uncertainty in ecosystem services-based resource management. J. Environ. Manag. 127, S145–S154. doi:10.1016/j.jenvman.2012.07.028
Guo, H., Cai, Y., Li, B., Tang, Y., Qi, Z., Huang, Y., et al. (2022). An integrated modeling approach for ecological risks assessment under multiple scenarios in Guangzhou, China. Ecol. Indic. 142, 109270. doi:10.1016/j.ecolind.2022.109270
Henry, M., Beguin, M., Requier, F., Rollin, O., Odoux, J.-F., Aupinel, P., et al. (2012). A common pesticide decreases foraging success and survival in honey bees. Science 336 (6079), 348–350. doi:10.1126/science.1215039
Holdaway, R. J., Wiser, S. K., and Williams, P. A. J. C. B. (2012). Status assessment of New Zealand's naturally uncommon ecosystems. Status Assess. New Zeal. Nat. uncommon Ecosyst. 26 (4), 619–629. doi:10.1111/j.1523-1739.2012.01868.x
Hope, B. K. (2006). An examination of ecological risk assessment and management practices. An Exam. Ecol. risk Assess. Manag. Pract. 32 (8), 983–995. doi:10.1016/j.envint.2006.06.005
Hou, M., Ge, J., Gao, J., Meng, B., Li, Y., Yin, J., et al. (2020). Ecological risk assessment and impact factor analysis of alpine wetland ecosystem based on LUCC and boosted regression tree on the Zoige Plateau, China. Remote Sens. 12 (3), 368. doi:10.3390/rs12030368
Huang, A., Xu, Y., Sun, P., Zhou, G., Liu, C., Lu, L., et al. (2019). Land use/land cover changes and its impact on ecosystem services in ecologically fragile zone: a case study of Zhangjiakou City, Hebei Province, China. Ecol. Indic. 104, 604–614. doi:10.1016/j.ecolind.2019.05.027
Huang, X., Wang, X., Zhang, X., Zhou, C., Ma, J., and Feng, X. (2022). Ecological risk assessment and identification of risk control priority areas based on degradation of ecosystem services: a case study in the Tibetan Plateau. Ecol. Indic. 141, 109078. doi:10.1016/j.ecolind.2022.109078
Hulme, P. E., Bacher, S., Kenis, M., Klotz, S., Kühn, I., Minchin, D., et al. (2008). Grasping at the routes of biological invasions: a framework for integrating pathways into policy. J. Appl. Ecol. 45 (2), 403–414. doi:10.1111/j.1365-2664.2007.01442.x
Jiang, Y., Nan, Z., and Yang, S. (2013). Risk assessment of water quality using Monte Carlo simulation and artificial neural network method. J. Environ. Manag. 122, 130–136. doi:10.1016/j.jenvman.2013.03.015
Jin, X., Jin, Y., and Mao, X. (2019). Ecological risk assessment of cities on the Tibetan Plateau based on land use/land cover changes – case study of Delingha City. Ecol. Indic. 101, 185–191. doi:10.1016/j.ecolind.2018.12.050
Kaiser, G., Burkhard, B., Römer, H., Sangkaew, S., Graterol, R., Haitook, T., et al. (2013). Mapping tsunami impacts on land cover and related ecosystem service supply in Phang Nga, Thailand. Nat. Hazards Earth Syst. Sci. 13 (12), 3095–3111. doi:10.5194/nhess-13-3095-2013
Kang, P., Chen, W., Hou, Y., and Li, Y. (2018). Linking ecosystem services and ecosystem health to ecological risk assessment: a case study of the Beijing-Tianjin-Hebei urban agglomeration. Sci. Total Environ. 636, 1442–1454. doi:10.1016/j.scitotenv.2018.04.427
Keith, D. A., Rodríguez, J. P., Brooks, T. M., Burgman, M. A., Barrow, E. G., Bland, L., et al. (2015). The IUCN red list of ecosystems: motivations, challenges, and applications. Conserv. Lett. 8 (3), 214–226. doi:10.1111/conl.12167
Krupke, C. H., Hunt, G. J., Eitzer, B. D., Andino, G., and Given, K. (2012). Multiple routes of pesticide exposure for honey bees living near agricultural fields. PLoS one 7 (1), e29268. doi:10.1371/journal.pone.0029268
Kwon, O.-S., Kim, J.-H., and Ra, J.-H. (2021). Landscape ecological analysis of green network in urban area using circuit theory and least-cost path. Land 10 (8), 847. doi:10.3390/land10080847
Landis, W. G. (2003a). The frontiers in ecological risk assessment at expanding spatial and temporal scales. Hum. Ecol. Risk Assess. An Int. J. 9 (6), 1415–1424. doi:10.1080/10807030390250912
Landis, W. G. (2003b). Twenty years before and hence; ecological risk assessment at multiple scales with multiple stressors and multiple endpoints. Hum. Ecol. Risk Assess. 9, 1317. doi:10.1080/10807030390248500
Landis, W. G., Durda, J. L., Brooks, M. L., Chapman, P. M., Menzie, C. A., Stahl, R. G., et al. (2013). Ecological risk assessment in the context of global climate change. Environ. Toxicol. Chem. 32 (1), 79–92. doi:10.1002/etc.2047
Li, Z.-T., Li, M., and Xia, B.-C. (2020). Spatio-temporal dynamics of ecological security pattern of the Pearl River Delta urban agglomeration based on LUCC simulation. Ecol. Indic. 114, 106319. doi:10.1016/j.ecolind.2020.106319
Liang, Y., and Song, W. (2022). Integrating potential ecosystem services losses into ecological risk assessment of land use changes: a case study on the Qinghai-Tibet Plateau. J. Environ. Manag. 318, 115607. doi:10.1016/j.jenvman.2022.115607
Liu, D., Chen, H., Zhang, H., Geng, T., and Shi, Q. (2020). Spatiotemporal evolution of landscape ecological risk based on geomorphological regionalization during 1980–2017: a case study of Shaanxi Province, China. Sustainability 12 (3), 941. doi:10.3390/su12030941
Liu, R., Li, L., Guo, L., Jiao, L., Wang, Y., Cao, L., et al. (2022). Multi-scenario simulation of ecological risk assessment based on ecosystem service values in the Beijing-Tianjin-Hebei region. Environ. Monit. Assess. 194 (6), 434. doi:10.1007/s10661-022-10086-9
Lozoya, J. P., Sarda, R., and Jiménez, J. A. (2011). A methodological framework for multi-hazard risk assessment in beaches. Environ. Sci. policy 14 (6), 685–696. doi:10.1016/j.envsci.2011.05.002
Luttik, R., Arnold, G., Boesten, J. J. T. I., Cresswell, J., Hart, A., Pistorius, J., et al. (2012). Scientific Opinion on the science behind the development of a risk assessment of Plant Protection Products on bees (Apis mellifera, Bombus spp. and solitary bees). EFSA J. 10 (5), 2668. Available at: http://www.efsa.europa.eu/efsajournal. doi:10.2903/j.efsa.2012.2668
Maddock, A., Plessis, M. A. d.J. B., and Conservation, (1999). Can species data only be appropriately used to conserve biodiversity? Biodivers. Conserv. 8, 603–615. doi:10.1023/a:1008819015819
Maltby, L. (2013). Ecosystem services and the protection, restoration, and management of ecosystems exposed to chemical stressors. Environ. Toxicol. Chem. 32 (5), 974–983. doi:10.1002/etc.2212
Maltby, L., van den Brink, P. J., Faber, J. H., and Marshall, S. (2018). Advantages and challenges associated with implementing an ecosystem services approach to ecological risk assessment for chemicals. Sci. Total Environ. 621, 1342–1351. doi:10.1016/j.scitotenv.2017.10.094
Meixner, M. D. (2010). A historical review of managed honey bee populations in Europe and the United States and the factors that may affect them. J. Invertebr. pathology 103, S80–S95. doi:10.1016/j.jip.2009.06.011
Mensah, S., Veldtman, R., Assogbadjo, A. E., Ham, C., Kakaï, R. G., and Seifert, T. (2017). Ecosystem service importance and use vary with socio-environmental factors: a study from household-surveys in local communities of South Africa. Ecosyst. Serv. 23, 1–8. doi:10.1016/j.ecoser.2016.10.018
Mommaerts, V., Reynders, S., Boulet, J., Besard, L., Sterk, G., and Smagghe, G. (2010). Risk assessment for side-effects of neonicotinoids against bumblebees with and without impairing foraging behavior. Ecotoxicology 19 (1), 207–215. doi:10.1007/s10646-009-0406-2
Mongeon, P., and Paul-Hus, A. J. S. (2016). The journal coverage of Web of Science and Scopus: a comparative analysis. J. Cover. Web Sci. Scopus a Comp. analysis 106 (1), 213–228. doi:10.1007/s11192-015-1765-5
Moraes, R., and Molander, S. (2004). A procedure for ecological tiered assessment of risks (PETAR). Hum. Ecol. Risk Assess. 10 (2), 349–371. doi:10.1080/10807030490438427
Munns, W. R., Helm, R. C., Adams, W. J., Clements, W. H., Cramer, M. A., Curry, M., et al. (2009). Translating ecological risk to ecosystem service loss. Integr. Environ. Assess. Manag. An Int. J. 5 (4), 500–514. doi:10.1897/ieam_2009-009.1
Munns, W. R., Poulsen, V., Gala, W. R., Marshall, S. J., Rea, A. W., Sorensen, M. T., et al. (2017). Ecosystem services in risk assessment and management. Integr. Environ. Assess. Manag. 13 (1), 62–73. doi:10.1002/ieam.1835
Munns, W. R., and Rea, A. W. (2015). Ecosystem services: value is in the eye of the beholder. Integr. Environ. Assess. Manag. 11 (2), 332–333. doi:10.1002/ieam.1620
Munns, W. R., Rea, A. W., Suter, G. W., Martin, L., Blake-Hedges, L., Crk, T., et al. (2016). Ecosystem services as assessment endpoints for ecological risk assessment. Integr. Environ. Assess. Manag. 12 (3), 522–528. doi:10.1002/ieam.1707
Ng, J. Y., Dhawan, T., Dogadova, E., Taghi-Zada, Z., Vacca, A., Fajardo, R.-G., et al. (2022). A comprehensive search string informed by an operational definition of complementary, alternative, and integrative medicine for systematic bibliographic database search strategies. BMC Complementary Med. Ther. 22 (1), 200–208. doi:10.1186/s12906-022-03683-1
Nienstedt, K. M., Brock, T. C., van Wensem, J., Montforts, M., Hart, A., Aagaard, A., et al. (2012). Development of a framework based on an ecosystem services approach for deriving specific protection goals for environmental risk assessment of pesticides. Sci. Total Environ. 415, 31–38. doi:10.1016/j.scitotenv.2011.05.057
Noss, R. F., and Peters, R. L. (1995). Endangered ecosystems: a status report on America’s vanishing habitat and wildlife[M]. Defenders of Wildlife.
Pan, N., Du, Q., Guan, Q., Tan, Z., Sun, Y., and Wang, Q. (2022). Ecological security assessment and pattern construction in arid and semi-arid areas: a case study of the Hexi Region, NW China. Ecol. Indic. 138, 108797. doi:10.1016/j.ecolind.2022.108797
Pártl, A., Vačkář, D., Loučková, B., and Krkoška Lorencová, E. (2017). A spatial analysis of integrated risk: vulnerability of ecosystem services provisioning to different hazards in the Czech Republic. Nat. Hazards 89 (3), 1185–1204. doi:10.1007/s11069-017-3015-z
Peng, J., Dang, W., Liu, Y., Zong, M., and Hu, X. (2015). Review on landscape ecological risk assessment. Acta Geogr. Sin. 70, 664–677. doi:10.11821/dlxb201504013
Peng, J., Liu, Y., Li, T., and Wu, J. (2017). Regional ecosystem health response to rural land use change: a case study in Lijiang City, China. Ecol. Indic. 72, 399–410. doi:10.1016/j.ecolind.2016.08.024
Plant Protection Products and their Residues (2012). Scientific Opinion on the science behind the development of a risk assessment of Plant Protection Products on bees (Apis mellifera, Bombus spp. and solitary bees). Efsa J. 10 (5), 2668. doi:10.2903/j.efsa.2012.2668
Plant Protection Products and their Residues (2014). Scientific opinion addressing the state of the science on risk assessment of plant protection products for non-target terrestrial plants. EFSA J. 12 (7), 3800. doi:10.2903/j.efsa.2014.3800
Plant Protection Products and their Residues (2015). Scientific Opinion addressing the state of the science on risk assessment of plant protection products for non-target arthropods. Efsa J. 13 (2), 3996. doi:10.2903/j.efsa.2015.3996
Potts, S. G., Biesmeijer, J. C., Kremen, C., Neumann, P., Schweiger, O., and Kunin, W. E. (2010). Global pollinator declines: trends, impacts and drivers. Trends Ecol. Evol. 25 (6), 345–353. doi:10.1016/j.tree.2010.01.007
Pressey, R. J. C. b. (2004). Conservation planning and biodiversity: assembling the best data for the job. Conservation Plan. Biodivers. assembling best data job 18 (6), 1677–1681. doi:10.1111/j.1523-1739.2004.00434.x
Qu, C., Li, B., Wu, H., Wang, S., and Li, F. (2016). Probabilistic ecological risk assessment of heavy metals in sediments from China’s major aquatic bodies. Stoch. Environ. Res. Risk Assess. 30 (1), 271–282. doi:10.1007/s00477-015-1087-4
Rahman, M. S., Saha, N., and Molla, A. H. (2014). Potential ecological risk assessment of heavy metal contamination in sediment and water body around Dhaka export processing zone, Bangladesh. Environ. Earth Sci. 71 (5), 2293–2308. doi:10.1007/s12665-013-2631-5
Rapport, D., Böhm, G., Buckingham, D., Cairns, J., Costanza, R., Karr, J., et al. (1999). Ecosystem health: the concept, the ISEH, and the important tasks ahead. Ecosyst. health 5 (2), 82–90. doi:10.1046/j.1526-0992.1999.09913.x
R.Core Team (2022). R: a language and environment for statistical computing. Vienna, Austria: R Foundation for Statistical Computing.
Renn, O. (2008). Concepts of risk: an interdisciplinary review part 1: disciplinary risk concepts. GAIA-Ecological Perspect. Sci. Soc. 17 (1), 50–66. doi:10.14512/gaia.17.1.13
Rodríguez, J. P., Rodríguez-Clark, K. M., Baillie, J. E., Ash, N., Benson, J., Boucher, T., et al. (2011). Establishing IUCN red list criteria for threatened ecosystems: IUCN red list criteria for ecosystems. Establishing IUCN red list criteria Threat. Ecosyst. 25 (1), 21–29. doi:10.1111/j.1523-1739.2010.01598.x
Sandrock, C., Tanadini, L. G., Pettis, J. S., Biesmeijer, J. C., Potts, S. G., and Neumann, P. (2014). Sublethal neonicotinoid insecticide exposure reduces solitary bee reproductive success. Agric. For. entomology 16 (2), 119–128. doi:10.1111/afe.12041
Schäfer, R. B., Bundschuh, M., Rouch, D. A., Szöcs, E., Von Der Ohe, P. C., Pettigrove, V., et al. (2012). Effects of pesticide toxicity, salinity and other environmental variables on selected ecosystem functions in streams and the relevance for ecosystem services. Sci. Total Environ. 415, 69–78. doi:10.1016/j.scitotenv.2011.05.063
Serveiss, V. B. (2002). Applying ecological risk principles to watershed assessment and management. Environ. Manag. 29, 145–154. doi:10.1007/s00267-001-0025-z
Shirvani, Z., Abdi, O., and Buchroithner, M. (2019). A synergetic analysis of Sentinel-1 and-2 for mapping historical landslides using object-oriented Random Forest in the Hyrcanian forests. Remote Sens. 11 (19), 2300. doi:10.3390/rs11192300
Skinner, D. J., Rocks, S. A., Pollard, S. J., and Drew, G. H. (2014). Identifying uncertainty in environmental risk assessments: the development of a novel typology and its implications for risk characterization. Hum. Ecol. Risk Assess. An Int. J. 20 (3), 607–640. doi:10.1080/10807039.2013.779899
Sommers, W. T., Marcy, S. K., van der Schalie, W. H., Lessard, G., Ouigley, T. M., Lackey, R. T., et al. (1999). Ecological assessments in ecosystem management. Ln: ecological risk assessment in the federal government. Washington, DC: National Science and Technology Council.
Sperotto, A., Molina, J.-L., Torresan, S., Critto, A., and Marcomini, A. (2017). Reviewing Bayesian Networks potentials for climate change impacts assessment and management: a multi-risk perspective. J. Environ. Manag. 202, 320–331. doi:10.1016/j.jenvman.2017.07.044
Streissl, F., Egsmose, M., and Tarazona, J. V. (2018). Linking pesticide marketing authorisations with environmental impact assessments through realistic landscape risk assessment paradigms. Ecotoxicology 27, 980–991. doi:10.1007/s10646-018-1962-0
Su, X., Li, X., and Kang, Y. (2019). A bibliometric analysis of research on intangible cultural heritage using CiteSpace. Sage Open 9 (2), 215824401984011. doi:10.1177/2158244019840119
Sugiyama, M., and Sugiyama, T. (2010). Interpretation of CBD COP10 decision on geoengineering. SERC Discussion.
Syberg, K., Backhaus, T., Banta, G., Bruce, P., Gustavsson, M., Munns, W. R., et al. (2017). Toward a conceptual approach for assessing risks from chemical mixtures and other stressors to coastal ecosystem services. Integr. Environ. Assess. Manag. 13 (2), 376–386. doi:10.1002/ieam.1849
Thomsen, M., Faber, J. H., and Sorensen, P. B. (2012). Soil ecosystem health and services–Evaluation of ecological indicators susceptible to chemical stressors. Ecol. Indic. 16, 67–75. doi:10.1016/j.ecolind.2011.05.012
Tittensor, D. P., Walpole, M., Hill, S. L., Boyce, D. G., Britten, G. L., Burgess, N. D., et al. (2014). A mid-term analysis of progress toward international biodiversity targets. A mid-term analysis Prog. toward Int. Biodivers. targets 346 (6206), 241–244. doi:10.1126/science.1257484
Tredennick, A. T., Hooker, G., Ellner, S. P., and Adler, P. B. (2021). A practical guide to selecting models for exploration, inference, and prediction in ecology. Ecology 102 (6), e03336. doi:10.1002/ecy.3336
Tuholske, C., Tane, Z., López-Carr, D., Roberts, D., and Cassels, S. (2017). Thirty years of land use/cover change in the Caribbean: assessing the relationship between urbanization and mangrove loss in Roatán, Honduras. Appl. Geogr. 88, 84–93. doi:10.1016/j.apgeog.2017.08.018
Turner, B., Janetos, A. C., Verbug, P. H., and Murray, A. T. (2013). Land system architecture: using land systems to adapt and mitigate global environmental change. Richland, WA (United States): Pacific Northwest National Lab.
Väänänen, K., Leppänen, M. T., Chen, X., and Akkanen, J. (2018). Metal bioavailability in ecological risk assessment of freshwater ecosystems: from science to environmental management. Ecotoxicol. Environ. Saf. 147, 430–446. doi:10.1016/j.ecoenv.2017.08.064
Van den Brink, P. J. (2008). Ecological risk assessment: from book-keeping to chemical stress ecology. Washington, D.C.: ACS Publications.
Van Eck, N., and Waltman, L. (2010). Software survey: VOSviewer, a computer program for bibliometric mapping. scientometrics 84 (2), 523–538. doi:10.1007/s11192-009-0146-3
Wang, B., Ding, M., Li, S., Liu, L., and Ai, J. (2020a). Assessment of landscape ecological risk for a cross-border basin: a case study of the Koshi River Basin, central Himalayas. Ecol. Indic. 117, 106621. doi:10.1016/j.ecolind.2020.106621
Wang, H., Liu, X., Zhao, C., Chang, Y., Liu, Y., and Zang, F. (2021a). Spatial-temporal pattern analysis of landscape ecological risk assessment based on land use/land cover change in Baishuijiang National nature reserve in Gansu Province, China. Ecol. Indic. 124, 107454. doi:10.1016/j.ecolind.2021.107454
Wang, K., Zheng, H., Zhao, X., Sang, Z., Yan, W., Cai, Z., et al. (2023). Landscape ecological risk assessment of the Hailar River basin based on ecosystem services in China. Ecol. Indic. 147, 109795. doi:10.1016/j.ecolind.2022.109795
Wang, M.-E., Ding, S.-K., Guo, G.-L., Li, X.-Z., Jiang, R., Zhang, Y., et al. (2020b). Advances in ecological risk assessment of soil in contaminated sites. Ying yong sheng tai xue bao = J. Appl. Ecol./ Zhongguo sheng tai xue xue hui, Zhongguo ke xue yuan Shenyang ying yong sheng tai yan jiu suo zhu ban 31, 3946–3958. doi:10.13287/j.1001-9332.202011.035
Wang, S., Tan, X., and Fan, F. (2022). Landscape ecological risk assessment and impact factor analysis of the qinghai–Tibetan plateau. Remote Sens. 14 (19), 4726. doi:10.3390/rs14194726
Wang, X., Che, L., Zhou, L., and Xu, J. (2021b). Spatio-temporal dynamic simulation of land use and ecological risk in the Yangtze River Delta Urban Agglomeration, China. Chin. Geogr. Sci. 31, 829–847. doi:10.1007/s11769-021-1229-1
Watanabe, M. E. (1994). Pollination worries rise as honey bees decline. Science 265 (5176), 1170. doi:10.1126/science.265.5176.1170
Wei, S., Pan, J., and Liu, X. (2020). Landscape ecological safety assessment and landscape pattern optimization in arid inland river basin: take Ganzhou District as an example. Hum. Ecol. Risk Assess. An Int. J. 26 (3), 782–806. doi:10.1080/10807039.2018.1536521
West, J. M., Julius, S. H., Kareiva, P., Enquist, C., Lawler, J. J., Petersen, B., et al. (2009). US natural resources and climate change: concepts and approaches for management adaptation. Environ. Manag. 44, 1001–1021. doi:10.1007/s00267-009-9345-1
Wong, C. P., Jiang, B., Kinzig, A. P., Lee, K. N., and Ouyang, Z. (2015). Linking ecosystem characteristics to final ecosystem services for public policy. Ecol. Lett. 18 (1), 108–118. doi:10.1111/ele.12389
Wu, F., Wang, X., Cai, Y., and Li, C. (2016). Spatiotemporal analysis of precipitation trends under climate change in the upper reach of Mekong River basin. Quat. Int. 392, 137–146. doi:10.1016/j.quaint.2013.05.049
Xiao, D., and Chen, W. (2002). On the basic concepts and contents of ecological security. Ying Yong Sheng Tai Xue Bao 13 (3), 354–358.
Xie, H., Wen, J., Chen, Q., and Wu, Q. (2021). Evaluating the landscape ecological risk based on GIS: a case-study in the poyang lake region of China. Land Degrad. Dev. 32 (9), 2762–2774. doi:10.1002/ldr.3951
Xing, L., Hu, M., and Wang, Y. (2020). Integrating ecosystem services value and uncertainty into regional ecological risk assessment: a case study of Hubei Province, Central China. Sci. Total Environ. 740, 140126. doi:10.1016/j.scitotenv.2020.140126
Xu, X., Yang, G., Tan, Y., Zhuang, Q., Li, H., Wan, R., et al. (2016). Ecological risk assessment of ecosystem services in the Taihu Lake Basin of China from 1985 to 2020. Sci. Total Environ. 554-555, 7–16. doi:10.1016/j.scitotenv.2016.02.120
Yan, J., Li, G., Qi, G., Qiao, H., Nie, Z., Huang, C., et al. (2021). Landscape ecological risk assessment of farming-pastoral ecotone in China based on terrain gradients. Hum. Ecol. Risk Assess. An Int. J. 27 (8), 2124–2141. doi:10.1080/10807039.2021.1956300
Yue, Z., Fei, Z., Mei, Z., Xiao-hang, L., Yan, R., and Juan, W. (2016). Landscape ecological risk assessment and its spatio-temporal variations in Ebinur Lake region of inland arid area. Yingyong Shengtai Xuebao 27 (1), 233–242.
Zhang, J., Cenci, J., Becue, V., Koutra, S., and Ioakimidis, C. S. J. S. (2020a). Recent evolution of research on industrial heritage in Western Europe and China based on bibliometric analysis. Sustainability 12 (13), 5348. doi:10.3390/su12135348
Zhang, T., Du, Z., Yang, J., Yao, X., Ou, C., Niu, B., et al. (2021). Land cover mapping and ecological risk assessment in the context of recent ecological migration. Remote Sens. 13 (7), 1381. doi:10.3390/rs13071381
Zhang, W., Chang, W. J., Zhu, Z. C., and Hui, Z. (2020b). Landscape ecological risk assessment of Chinese coastal cities based on land use change. Available at: http://www.efsa.europa.eu/efsajournal. Appl. Geogr. 10 (5), 2668. doi:10.2903/j.efsa.2012.2668
Keywords: ecosystem service, ecological risk assessment, thematic evolution, landscape ecology, social-ecological systems, land use, ecological risk management
Citation: Qian L, Zeng X, Ding Y and Peng L (2023) Mapping the knowledge of ecosystem service-based ecological risk assessment: scientometric analysis in CiteSpace, VOSviewer, and SciMAT. Front. Environ. Sci. 11:1326425. doi: 10.3389/fenvs.2023.1326425
Received: 23 October 2023; Accepted: 13 November 2023;
Published: 30 November 2023.
Edited by:
Yalçın Tepe, Giresun University, TürkiyeCopyright © 2023 Qian, Zeng, Ding and Peng. This is an open-access article distributed under the terms of the Creative Commons Attribution License (CC BY). The use, distribution or reproduction in other forums is permitted, provided the original author(s) and the copyright owner(s) are credited and that the original publication in this journal is cited, in accordance with accepted academic practice. No use, distribution or reproduction is permitted which does not comply with these terms.
*Correspondence: Li Peng, cGVuZ2xpQG5qZnUuZWR1LmNu
Disclaimer: All claims expressed in this article are solely those of the authors and do not necessarily represent those of their affiliated organizations, or those of the publisher, the editors and the reviewers. Any product that may be evaluated in this article or claim that may be made by its manufacturer is not guaranteed or endorsed by the publisher.
Research integrity at Frontiers
Learn more about the work of our research integrity team to safeguard the quality of each article we publish.