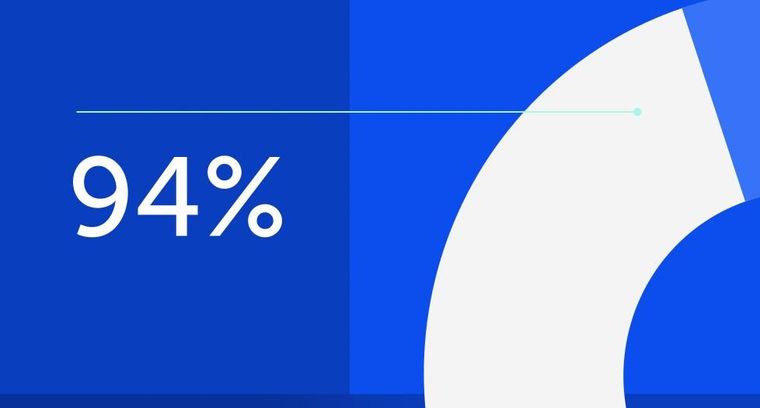
94% of researchers rate our articles as excellent or good
Learn more about the work of our research integrity team to safeguard the quality of each article we publish.
Find out more
ORIGINAL RESEARCH article
Front. Environ. Sci., 21 December 2023
Sec. Atmosphere and Climate
Volume 11 - 2023 | https://doi.org/10.3389/fenvs.2023.1294039
This article is part of the Research TopicCrucial Air Quality, Atmospheric Environment, and Climate Change in Low- and Middle-Income CountriesView all 8 articles
Air pollution has become an increasing concern in China. At the same time China has undergone a rapid shift from traditional government to E-government. Our study contributes to the existing literature by exploring the relationship between E-government and air pollution. This study investigates the impact of E-government on PM2.5 in 226 cities between 2012 and 2016. The results show that E-government has a significantly positive effect on air quality, the PM2.5 concentration will decrease by 6.71 percent with one percent increase in the E-government score. Furthermore, we find the impacts of E-government on air quality vary across cities. E-government has stronger effects of air quality improvements on more market-oriented cities and non-capital provincial cities, which illustrates although the government plays a leading role in the construction of E-government, its impact on the environment depends more on market dynamics. We also tested there exist three channels through which E-government affects air quality, namely, promoting innovation, improving total factor productivity, and optimizing industrial structure. This study provides important references for the green transformation in the context of E-government.
Information and communication technology (ICT) has rapidly advanced in recent years, promoting the development of E-government, or Electronic Government. E-government refers to the use of digital technologies and online platforms by government agencies to provide public services and interact with local citizens. Since E-government facilitates governance efficiency and transparency, the Chinese government gives priority to E-government buildup and elevates it as a national strategy (Yan and Lyu, 2023). In the past decade, China has carried out large-scale E-government projects throughout the country. According to the United Nations E-government report, all 193 member states have provided some form of online government services since 2014. China’s E-government development level continues to rise, with the E-government development index (EGDI) climbing from 0.7948 in 2020 to 0.8919 in 2022 (refer to Figure 1). The improvement of the level of E-government has become a significant phenomenon in the digital society.
At the same time, with the rapid economic development, air pollution has become a hot topic in recent years. China’s haze pollution, which is mainly composed of fine particulate matter PM2.5, ranks among the highest in the world (refer to Figure 2). PM2.5 is mainly produced by natural and man-made sources. Natural sources include wildfires and volcanic eruptions, while man-made sources are primarily derived from fossil fuel consumption. The burning of fossil fuels due to human activities is the main source of haze concentration in China. In 2013, China launched the toughest-ever clean air action plan to resolve serious and extensive air pollution issues. Despite the remarkable air quality improvement after the introduction of the Action Plan, air pollution in China is still severe and remains a big social concern. For example, 64 percent of 338 prefecture-level cities in China failed to meet the national standard of annual PM2.5 level in 2017 (Zhang et al., 2019).
FIGURE 2. The emission of major pollutants in exhaust gas from 2013 to 2022 (units: 10,000 tons). Source: Socioeconomic Data and Applications Center (SEDAC) at Columbia University.
Existing research suggests that the digital economy is expected to provide an opportunity for China to go green (Wu et al., 2023).The promotion of E-government helps to improve government efficiency and transparency, and strengthen the regulation of corporate emissions and emission reduction management, so it has a mitigating effect on air pollution. According to Dhaoui (Dhaoui, 2022), E-government promotes sustainable economic development and creates opportunities for innovation. Das (Das and Das, 2022)believes that the development of E-government has made it easier to start a new business and initiate innovative ventures. Taken all the above together, there is an inseparable correlation between economic growth and environmental pollution (Zhang and Ran, 2023).
Since E-government has the potential to enhance the efficiency of human activities, we attempt to answer the following questions. First, can E-government reduce haze pollution? Second, is there heterogeneity among different cities? Third, what are the possible mechanisms of the E-government affecting haze pollution?
This paper aims to contribute to existing research in the following ways. Firstly, we integrate the E-government and haze pollution into a unified research framework. Accordingly, this paper explores the impact of digital economic development on air quality from the perspective of E-government. The current researches on E-government primarily focuses on areas such as economic growth and innovation. To the best of our knowledge, there are limited literatures on the impact of E-government on air quality. Our objective is to address this academic gap, striving to contribute to the understanding of the relationship between E-government and the environment. We aim to summarize our research findings objectively and provide a reasonable interpretation of this phenomenon. Secondly, the panel data of E-government and air pollution from 226 cities in China are constructed. Based on the two-way fixed effect linear model, we find that E-government can improve air quality significantly. In particular the impacts are more effective in more market-oriented cities and non-capital provincial cities. It illustrates that even when the E-government policy is pushed, its impact on environment rolls out mainly by the free market. The findings may provide a new green development path for other regions and other countries, especially for less-developed cities. Finally, the possible channels through which E-government affects air quality are explored. This study suggests that E-government reduces air pollution by three channels, namely, promoting innovation, improving total factor productivity and optimizing industrial structure. These mechanism analyses provide useful references for understanding the relationship between E-government and air pollution.
The rest of this paper is structured as follows. The next section provides a review of the existing literature on the subject. The third section details the definitions of variables and sources of data. The fourth section presents the empirical analysis. Finally, the fifth section concludes and gives some suggestions.
With the emergence and development of E-government, more and more literatures begin to cover this field of E-government. E-government. In January 1998, former U. S. Vice President Al Gore introduced the concept of Digital Earth in his speech titled “Awareness of the Human Planet in the New Century” at the opening ceremony of the California Science Center. This marked the beginning of the emergence of digital-related concepts and practices on a global scale. The world we live in is transforming from an agricultural and industrial society to a digital society. This shift has not only changed the way people live but also transformed the mode of governance. By integrating fragmented information, data, and intelligent services, traditional government is gradually transitioning to E-government (Zhang and Kimathi, 2022).
E-government refers to the use of digital and information communication technologies to improve the quality and efficiency of government services; it is part of digital society and an important direction for government transformation. There are three aspects of E-government, namely, digital management, digital services, and digital governance. With the development of E-government, it also needs to address a series of issues such as information security, privacy protection, data management, etc. Previous empirical studies have quantitatively analyzed the impact of E-government on the transformation of government organization (Gao et al., 2013), public engagement (Khan and Krishnan, 2021), public service (Yan and Lyu, 2023), transparency (Hochstetter et al., 2023) and efficiency of government operation (Shao et al., 2023), etc. Krishnan and Majeed found that E-government can promote economic growth by reducing government corruption through empirical analysis (Krishnan et al., 2013; Majeed and Malik, 2016). From a sustainable development perspective, E-government can promote sustainable development by improving the quality of government supervision (Dhaoui, 2022; Zhang and Kimathi, 2022). Moreover, regional integration can help alleviate the effects of the post-Newcastle pneumonia pandemic and promote sustainable development to some extent through the implementation of strong E-government systems (Ullah et al., 2022).
The positive role of E-government in improving governance and promoting economic growth has been well-studied, however, the research about how E-government acts on the environment is scarce. This paper focuses on haze pollution and regards it as one of the major environmental concerns. The previous studies have involved the factors contributing to the haze pollution, such as transportation (Guo et al., 2014), China’s cleanup policy (Zhang et al., 2019) and industrial emissions (Huang et al., 2015). Some researchers have found that although the concentration of PM2.5 is affected by factors such as population, urbanization rate and planting rate, the influence of different countries varies according to the development path of different countries (Lim et al., 2020). For China, some scholars have found that there are obvious differences in the level of green development between the eastern region and the western region (Yang et al., 2023).When focusing on air pollution implementation policies, it was found that the effectiveness of implementation varied significantly from city to city (Jiang et al., 2020).
About water pollution, some scholars suggest that pollution levels vary significantly between the upper and lower reaches of the Yangtze River in China. This difference may be attributed to the fact that the Pigou tax, which aims to reduce negative externalities through taxation, is less effective in the lower reaches. Additionally, officials in the downstream area may face disincentives to address the issue, which leads to a greater accumulation of pollutants (Chen et al., 2018). Other scholars have studied pear-packing plants, it is found that increased haze pollution affects worker productivity and thus reduces economic growth (Chang et al., 2016). Economic growth and pollution have a negative correlation, China’s economic growth in the past 20 years has led to improved health, and pollution has acted as a counterbalance (Ebenstein et al., 2015). In general, there is little research on the direct impact of E-government on haze pollution. E-government is for the needs of citizens, it deepens integration and collaboration among reformed government levels, agencies, and organizations, thereby reducing resource depletion, personnel consumption, and environmental pollution. Additionally, E-government optimizes resource allocation. It relies on big data and Internet to pay attention to high-polluting industries, promotes multi-governance patterns of government, market, and society, and it enhances citizens’ decision-making rights and reduces haze pollution from various aspects. Therefore, this paper proposes the first hypothesis as follows.
Hypothesis 1. E-government can significantly reduce the level of haze pollution.
E-government can provide better public service and increase public satisfaction compared to traditional government methods. Through data integration, E-government can break down information barriers between different government levels and departments, creating an efficient government network that saves social costs. Almeida and Zouain (Almeida and Zouain, 2016) studied the impact of E-government on business and entrepreneurship in high-, middle-, and low-income countries. Shamim (Shamim, 2007) argues that the number of cell phone and internet users has a significant impact on economic growth. The factors affecting PM2.5 concentrations are divided into two categories: economic and environmental factors, with R&D having the highest impact (Wu et al., 2020). E-government has three modes of internal drive, external drive, and internal-external linkage (Yifan and Bei, 2021). Therefore, E-government can reduce haze pollution through three main channels:
One channel is by promoting innovation and entrepreneurship. Firstly, the E-government can achieve the modernization, efficiency, convenience, and transparency of government work by promoting digital transformation (Majeed and Malik, 2016). For example, through digital management and services, the government can respond more quickly and accurately to environmental pollution incidents, and carry out relevant regulations and processing. In addition, digital transformation can also provide more convenient services for enterprises and the public, reducing unnecessary traffic and personnel flow, and thus reducing the impact on air quality (Das and Das, 2022). Secondly, E-government can improve the level of environmental protection technology by promoting technological innovation. E-government can encourage enterprises and research institutions to conduct innovative research and development in the field of environmental protection technology. It promotes the application and popularization of new environmental protection technology, which will reduce pollutant emissions and thus improve air quality. Thirdly, E-government can promote the development and innovation of the environmental protection industry through data sharing and collaborative innovation among the government, enterprises, and the public. For example, the government can publicly share air quality data, encourage enterprises and research institutions to carry out environmental protection technology research and development. Also, E-government promotes the application based on data, and spurs the development and innovation of the environmental protection industry.
The second channel is by promoting green total factor productivity. China’s industrial activities with high investment and energy consumption have led to not only rapid GDP growth but also severe environmental pollution, especially the emission of PM2.5. Green total factor productivity refers to a production method that achieves sustainable development by improving resource utilization efficiency and reducing pollution emissions while achieving economic growth. E-government can promote the green total factor productivity of enterprises through digital technology and information sharing. For example, the government can monitor and assess the environmental pollution and resource consumption of enterprises in real-time through digital supervision and data sharing, thereby promoting enterprises to adopt more environmentally friendly and efficient production methods and reduce the impact on air quality. In addition, E-government can also encourage enterprises to adopt more environmentally friendly production methods and improve green total factor productivity through policy guidance and incentive measures. For example, the government can introduce relevant environmental protection policies, increase support for environmental protection technology research and application, and improve the market competitiveness of the environmental protection industry, thereby promoting enterprises to achieve green production.
Another channel is by optimizing industrial structure. Firstly, E-government can promote industrial upgrading and transformation through industrial planning and adjustments. The government can formulate relevant policies to guide enterprises to develop towards green, low-carbon industries. It encourages the development of new energy, environmental protection technology, and improves high-polluting and high-energy-consuming industries. These measures help to reduce the negative impact on air quality and promote sustainable development (Zhang et al., 2019). Secondly, the E-government can promote collaborative development and resource sharing among different industries through digital technology and data sharing, improving resource utilization efficiency. For example, the government can promote collaborative development among industries through digital platforms and data sharing, enabling effective treatment and reuse of waste and emissions, thereby reducing the impact on the environment and air quality. Thirdly, the E-government can promote the digital upgrading and intelligent transformation of traditional industries through digital technology and information services, improving production efficiency and reducing resource consumption. For example, the government can encourage enterprises to adopt digital technology to optimize production processes, reduce energy consumption and waste emissions, and lower the impact on air quality.
In summary, this paper proposes the second hypothesis.
Hypothesis 2. E-government can reduce the level of haze through three paths: innovation, improving green total factor productivity and optimizing industrial structure.
According to the theoretical analysis of the relationship between E-government and air pollution, the benchmark model is established as follows:
In the above model,
Haze pollution (PM2.5) is the explained variable in this paper, the data comes from the annual average of PM2.5 concentrations from the Socioeconomic Data and Applications Center (SEDAC) at Columbia University. PM2.5 is a relatively mainstream research variable in current society, and it is mainly fine particulate matter with a diameter less than or equal to 2.5 μm, which contains many toxic and harmful substances, and if it exists in the air for a long time, the pollution of human body and environment should not be underestimated. Therefore, its annual average value is used as an important indicator to measure haze pollution in this paper, which can be a more objective and accurate measure of the pollution status of an area.
E-government (score) is the key influencing variable. In this paper, the level of E-government is measured by using the total score of E-government data released by the EChinagov website, which has been widely used in previous studies. The total score of E-government is 100 points, determined by scoring and summing up the scores of Public Affairs, online service, interactive communication, user experience, and internationalization. This study focuses on E-government panel data of 226 cities from 2012 to 2016, including 32 cities with more developed economies such as provincial capitals and sub-provincial cities. We exclude municipalities directly under the central government due to data availability.
We use several control variables. One group of variables is meteorological condition, it contains wind speed (wind), temperature (temp), sunshine duration (sun), and precipitation (rain), these variables can affect the level of haze. We also use variables of infrastructure (km), represented by road mileage in each city; Foreign Direct Investment (FDI), represented by the actual use of foreign investment in the year as a percentage of regional GDP, it may influence the environmental governance decisions of the government that year. Urbanization rate (urb) is used, since the urbanization process will generate a large demand for energy consumption, which may lead to a corresponding increase in pollutant emissions (Maheshwari et al., 2020). Referring to Gan (Gan et al., 2020), the level of urbanization is measured by the ratio of the number of residents to the total number of urban residents at the end of the year. Trade openness (trade) is another control variable, since it can affect the pollutant emissions by introducing advanced foreign clean technology and environmental protection equipment. Referring to Lin (Lin, 2017), trade openness is measured by the ratio of total imports and exports to GDP.
This paper uses 226 cities from 2012 to 2016 as samples. We exclude cities lacking relevant data. The data of E-government meteorological condition are obtained from the daily value dataset of Chinese terrestrial climate data V3.0 processing. The rest of the data are obtained from China Statistical Yearbook, China County Statistical Yearbook, China City Statistical Yearbook, Guo-Tai-An database, National Bureau of Statistics, etc. All metrics are logarithmic. The valid samples are 1182, the descriptive statistics are shown in Table 1.
In this paper, we use a fixed-effects model to estimate the effect of E-government on haze pollution, as shown in Table 2. In our regressions, we control for meteorological variables that may affect air pollution, such as precipitation, sunshine duration, temperature, and wind speed. We control road mileage, which is one of the factors affecting PM2.5 concentration. We also control other variables that may affect PM2.5 concentration and E-government construction.
Except for adding control variables, we use year-fixed effects and solidly fixed effects. Standard errors are clustered according to the interaction term of city and year. In this way, standard errors can be estimated to accommodate the dependence of the year of the sample period and the arbitrary structure of the city.
The results in column (1) show the regression result with only the E-government and PM2.5. It can be seen that for 1 percent increase in the E-government score, the PM2.5 concentration decreased by 6.84 percent. This result is still slightly higher than we expected. Because it only fixed the influencing factors of PM25, but not the influencing factors of E-government.
Column (2) shows the results that we added Meteorological data more than column (1) had. It can be seen that for 1 percent increase in the E-government score, the PM2.5 concentration decreased by 3.82 percent.
Column (3) shows the results that we added infrastructure, Foreign Direct Investment, Urbanization rate, and Trade openness more than column (1) had. It can be seen that for 1 percent increase in the E-government score, the PM2.5 concentration decreased by 7.14 percent.
The estimates in column (4) report our main regression results, fixing the individual and year cross-product terms. It can be seen that the coefficient of E-government is significantly negative and remains robust across the remaining columns of different regression results, which can be interpreted in an economic sense: each 1 percent increase in E-government score is associated with a 6.71 percent decrease in haze pollution concentration, which is economically significant.
Overall, E-government significantly reduces haze pollution, which is significant in an economic sense.
To verify the reliability of the conclusions drawn above, a series of robustness tests are provided in Table 3.
Replace the explained variables. Since there is not only a single pollutant in air pollution, to verify that E-government will effectively reduce the level of air pollution, the first to third columns of the table below replaces the haze pollution PM2.5 with another pollutant such as PM10 and industrial smoke (FC) emissions, which are also major pollutants in the atmosphere, and the regression results are shown in the column (1)–(2) below. In the meantime, we also add Industrial wastewater discharge (WATER) as an extra variable to compare, the regression is shown in column (3). As can be seen, the coefficients before the key explanatory variables remain significantly negative, when E-government increases by 1 percent, PM10 comes down 0.2070 percent, FC comes down 0.2279 percent, and WATER comes down 0.1603 percent. This indicates that E-government does have an ameliorative effect on the improvement of pollution, and the regression results are still robust.
Change the clustering method. In the above regression results, our regression results are clustered on the intersection term of city and year. Now we switch the regression to the interaction terms clustered to province and year, to verify whether the results are still robust at a higher level of clustering. As we can see in column (4) below, each 1 percent increase in the E-government score is associated with 6.71 percent decrease in haze pollution concentration, the result is still robust.
Lagging all control variables by one period. Some control variables do not have a current period effect on haze pollution, such as wind speed, precipitation, etc. To alleviate the endogeneity problem caused by this, we lagged all control variables by one period for regression, as shown in column (5) below, and the results show that the results of the baseline regression are still significant by lagging all control variables by one period.
To exclude possible biases in estimation caused by endogeneity such as reverse causality and omission of explanatory variables, this paper uses the instrumental variables method for two-stage least squares regression, and the instrumental variables are selected as follows.
First, it is considered that the development of digital government cannot be separated from the interaction of information, whether it is the implementation of policies or the communication with enterprise departments. In the early days, people’s information communication was mainly through the post office system, so the post office reflected the level of information exchangeability of a region. For example, in remote areas, there are fewer post offices and the policy implementation cycle is longer. In contrast, places closer to government agencies are more densely populated, with more post offices distributed and information transmitted faster. In this sense, the number of post offices selected as an instrumental variable for the digital government development index satisfies the relevance requirement. At the same time, the historical number of post offices does not have a direct effect on the existing pollution relative to the speed of digital government development and information technology changes in recent years. In this sense, after controlling for other control variables, the selection of the number of historical post offices as an instrumental variable satisfies the exclusion requirement to some extent. Based on the above logic, the number of post offices per million people in 1984 is selected as the instrumental variable for the digital government index in this paper. Because the sample of this study is panel data, using only the number of post offices per million people in 1984 at the city level as the instrumental variable will be difficult to moderate because of the application of the fixed effects model. For this reason, drawing on the setting method of Nunn (Nunn and Qian, 2014), this paper constructs the interaction term between the number of post offices per million people in 1984 in each city respectively and the mean of digital government in that year as an instrumental variable (yj2), and the following Table 4 present the 2SLS regression results.
Second, considering that the above instrumental variables may have an unknown effect on air pollution, we use the mean value obtained by excluding the total value of the numerical government of the year, after excluding region i itself, as the instrumental variable (mean) of region i in that year. The specific construction formula is as follows.
Where i denotes city and k denotes the other city except i, and j represents the total number of the city samples in year t.
The reasons are: (1) The characteristics of individual i within the group, will be influenced by the mean or sum characteristics of other individuals within the group, the correlation condition of the instrumental variable is satisfied. (2) The average or sum characteristics of other individuals in the group do not directly affect the results of individual i, (PM2.5it) i.e., the homogeneity condition of the instrumental variable is satisfied. The instrumental variable consists of itself. The advantage of this method is that a valid instrumental variable can be constructed by using the information contained in the heteroskedasticity of the error without resorting to external variables, and the regression results are shown in columns (3)–(4) below.
We find that when regressing the number of post offices per million people in 1984 on the interaction term with the annual digital government means and constructing the digital government group means as the instrumental variable for the digital government construction index, the regression results are roughly as those obtained in the previous benchmark regression, with the development of digital government having a significant dampening effect on air pollution. Therefore, combining the regression results in Table 4, the core conclusion of this paper remains robust after considering endogeneity.
Since the data on the process of E-government construction and the concentration of haze pollution levels in each region of the samples vary widely, it is reasonable to suspect that the haze pollution control levels in different regions affected by E-government construction will also vary widely and that the level of development and policy levels of cities will vary widely. To distinguish the effects of differences among cities, this paper divides the sample into provincial capital cities and other cities in the province. Also we divide cities with high marketization levels and cities with low marketization levels.
Firstly, we consider the difference of whether the sample is the capital city of a province. Generally speaking, the capital city of a province is the economic and political center of a province. Directives or policies issued by the central government are also often run first by provincial capital cities. However, provincial capital cities may be less affected by heavy polluters because these enterprises generally migrate down to other cities in the province, and provincial capital cities may inherently pay more attention to pollution control than other cities in the province-so will E-government be more useful in other cities in the province? In this paper, the samples are divided into provincial capitals and other cities in the province for regression. As shown in the following table, the improvement of air pollution is greater in E-government in other cities in the province, while the improvement of air pollution in provincial capitals because of E-government is not significant. This might because provincial capitals pay more attention in pollution control, and the vast majority of air control policies issued by the central government to each province are provincial capitals. The provincial capitals are the face of each province and the center of political rights, so they will receive more attention and faster response to environmental management. For the other cities in the province, firstly, there will be more heavy industrial enterprises in the local area leading to a decrease in air quality, and secondly, not much attention is paid to environmental governance itself. However, after building the E-government platform, it can be seen that the coefficient in front of the key explanatory variable for other cities in the province is significantly negative, so it further indicates that E-government will improve air quality and has a greater impact on other cities in the province.
Secondly, we consider the differences in marketization levels. Market mechanism plays an important role in the accumulation of capital, land, talents, technology, and other factors required by enterprises for energy conservation and emission reduction. Specifically, when marketization is low, inter-regional market differentiation is serious, and inter-regional factor flow is blocked, which can easily lead to resource allocation imbalance, structural overcapacity, and factor market distortion. In particular, distortions in factor markets may lead to underpricing of energy. Low energy input costs will lead to a substantial increase in energy consumption, a decline in energy utilization efficiency, and serious environmental pollution (Zhang et al., 2017). In this paper, the samples are divided by year into highly market-oriented cities with an Index of marketization above the mean and low-market cities with an Index of marketization below the mean. As can be seen in Table 5, the regression results show that E-government has a more significant impact on the management of air pollution in high marketization cities. This effect is not significant in cities with below-average marketization. The reason for this phenomenon may be that in cities with a better market environment, the government has extra funds for air pollution control. Therefore, the direct or indirect effect of E-government on air pollution is more obvious. In cities where the marketization index is below average, the government may not have more money to manage air pollution, which is after all very expensive in terms of human and financial resources.
From the above regressions, we can see that E-government can significantly reduce the level of haze pollution, but what is the transmission mechanism of this? From the previous analysis, E-government may promote innovation development, improve green total factor productivity, and optimize the industrial structure to reduce the level of haze pollution. The first stage of the mechanical test is the regression of E-government on innovation index, green total factor productivity, and industrial structure.
First, E-government promotes innovation and entrepreneurship. The construction of digital government may encourage innovation and entrepreneurship by providing digital services and technical support, simplifying administrative procedures, and other means. This can help increase enterprises’ awareness and ability in environmental protection, promote the development of green technologies and environmental protection industries, and thus reduce environmental pollution. In this paper, the innovation index is chosen as the mediating effect, and the updated model of the patent data published by the China Intellectual Property Office is estimated concerning Yang (Yang et al., 2021), and the results are aggregated into provincial data to obtain the index of technological innovation, which is obtained from the Chinese Cities and Industries Innovation Report published by the Center for Industrial Development of Fudan University. This paper only presents the results of the first stage of the mechanism test, and the results of the second stage are elaborated to the reader by the theory. First of all, it can be seen from column (1) of Table 6 that E-government has a significant effect on the innovation index. We also add the Number of green inventions filed that year (sgreenpat) and the number of green utility model patents applied in that year (sgreenpatnew) as two other variables that represent innovation, the results of which are shown in columns (2)–(3) below. In the past, the application for a patent may go through a long waiting and approval process, while the establishment of E-government may make the patent application more efficient. E-government itself is also an important marker built on innovation, and innovation can drive E-government to have a significant impact on reducing the level of environmental pollution technologically, configurationally, and structurally.
E-government is moving towards information management, scientific management, and networked management through the use of emerging technologies and various innovative patents to reduce the level of environmental pollution. In this process, the development of innovation will improve the management of emission enterprises and the efficiency of pollution management. The Chinese government is an innovation-driven government, through which E-government can promote entrepreneurship, so that resources such as land, labor, and capital can realize their potential motivation for a cleaner environment, avoiding the waste of resources and reducing the intensity of pollution emissions. Innovation allows companies to reduce transaction costs, which not only reduces energy consumption, but also indirectly improves the efficiency of pollution emission treatment. Collectively, E-government on the one hand optimizes the pollution treatment technology of heavy polluters and also improves the innovation level of enterprises, which directly affects air quality; on the other hand, E-government also plays a key role in promoting industrial upgrading, which allows further reduction of air pollution.
Second, E-government improves Green Total Factor Productivity (GTFP). The construction of digital government may improve enterprise productivity and quality, and reduce pressure on the environment by promoting the sharing, collaboration, and optimization of public resources. For example, digital transportation, logistics, and supply chain management can reduce energy and resource waste, and reduce environmental pollution. Green total factor productivity responds to the dynamic changes of green technology, which is also the key to achieving energy saving and industrial win-win. This paper draws on Pastor to measure urban total factor productivity growth under a global covariance data envelopment analysis framework that integrates an over-efficient SBM model considering non-desired outputs and the Malmquist productivity index (Pastor and Lovell, 2005). As shown in column (4) in Table 6, E-government has a significant positive impact on green total factor productivity. At the same time, green total factor productivity can influence the transformation of China’s industrial development mode. Total factor productivity has become the core driver of China’s high industrial growth and pollution reduction, and green total factor productivity can influence the transformation of China’s industrial development mode by affecting environmental pollution, i.e., the higher the green TFP, the higher the industrial efficiency, and thus the reduction of environmental pollution.
Third, E-government encourages Industrial structure. The construction of digital government may encourage enterprises to transform from high-polluting industries to low-polluting industries by improving the market and investment environment, accelerating industrial structure adjustment and upgrading. This can reduce pollution emissions from high-polluting industries while promoting a low-carbon economy and green growth. The upgrade of industrial structure can reduce environmental pollution in cities. E-government improves government work efficiency and interconnection services between government and enterprises by improving the government’s Internet office, big data measurement and mobile interconnection. These efficiency improvements will also promote industry just enough to upgrade, and the industrial upgrade brought about by high value-added, high technology content and low energy consumption, low pollution industrial characteristics can reduce pollution emissions and improve environmental quality. The column 5 in Table 6 shows that E-government has a significant positive effect on industrial structure, and E-government makes pollutant emissions further reduced by optimizing the cooperative relationship between government and enterprises and by changing and optimizing the factor input structure and production and operation mode of polluting industries. From the direction of green economy development, the production mode of high-polluting industries will be curbed, so E-government can achieve the purpose of reducing pollution emissions by promoting industrial upgrading.
From Table 6, it can be seen that E-government has a significant positive effect on the innovation index, total factor green productivity, and industrial structure upgrading, and the economic significance is significant.
Based on the data from the Socioeconomic Data and Applications Center (SEDAC) at Columbia University and EChinagov website, this study investigates the impact of E-government on PM2.5 in 226 cities between 2012 and 2016 in China. Our main conclusions are as follows. First, we find that E-government has a significantly positive effect on air quality, for one percent increase in the E-government score, the PM2.5 concentration decreased by 6.71 percent. Our results are robust under several robustness checks, including the replacement of the key explanatory variables, shrinking the tail, adjustment of clustering method and so on. Second, we examine the roles of three impact mechanism variables (innovation, industrial structure and total factor productivity) in the causal relationship between E-government and air quality. It is tested that the E-government’s improvement effects on air quality are mainly by promoting innovation, improving total factor productivity and optimizing industrial structure. Third, our heterogeneity analysis shows a series of interesting conclusions when we divide the sample into different areas. The E-government plays a great role in reducing air pollution in more market-oriented cities and non-capital provincial cities. The empirical analysis shows that the increase of E-government development in the provincial capital cities will not cause a significant change in air pollution, while in non-capital cities it will be significantly reduced by 6.42 percent. Furthermore, we observe the air pollution reduced significantly by 8.76 percent in the samples that exceed the marketization average index. Which suggests that although the government plays a leading role in the construction of E-government, its impact on the environment depends more on market dynamics.
Here are some suggestions on how to improve air quality (pollution) by enhancing the development of E-government.
E-government provides a new way to spur the green transition for one country, so it should be prioritized and taken as a national strategy. More resources including monetary, human and technology resources should be pooled and put into the E-government construction. Government should use digital technology to build smart cities, and improve urban management efficiency and environmental protection level. For example, an intelligent traffic management system can be established to reduce the emission of pollutants from vehicles by optimizing traffic flow and reducing congestion. At the same time, an intelligent waste classification system can be established to promote waste classification, processing, and reuse, and reduce waste pollution to the environment.
E-government should be more used to monitor and evaluate environmental pollution and resource consumption of enterprises in real-time, as well as the air quality of cities. For example, an intelligent environmental monitoring system can be established to monitor and analyze environmental data of enterprises and cities in real-time using sensors, big data, and artificial intelligence technology. This can improve the efficiency and accuracy of government supervision, prompting enterprises to adopt more environmentally friendly and efficient production methods, and reduce the impact on air quality.
E-government should be more used to encourage enterprises to adopt more environmentally friendly production methods and improve the green total factor productivity through policy guidance and incentive measures. For example, relevant environmental protection policies can be introduced, and support for the research and development, and application of environmental protection technology can be increased to enhance the market competitiveness of the environmental protection industry, thereby promoting green production by enterprises. At the same time, incentive measures such as tax breaks, subsidies, and rewards can be introduced to encourage enterprises to adopt environmental protection measures and reduce the impact on air quality.
Finally E-government should be more used to promote the environmental awareness through education and public participation. For example, digital platforms and social media can be used to disseminate environmental knowledge to the public, and improve public environmental awareness and participation. At the same time, environmental education can be strengthened, and environmental awareness and environmental protection literacy can be fostered to promote comprehensive participation in environmental protection action.
The main shortcomings of this paper are as follows. Due to the availability of data, we set the time window of the study between 2011 and 2016, and the research did not include municipalities directly under the central government. However, as the development of E-government is an ongoing process, a sustained and comprehensive focus on the environmental implications is necessary for future analysis. In addition, in order to illustrate the level of E-government, we used a multi-dimensional evaluation index of E-government. Although it’s more comprehensive than single index, more measurement methods still remain to be included in the future study.
The datasets presented in this study can be found in online repositories. The names of the repository/repositories and accession number(s) can be found in the article/Supplementary material.
HH: Conceptualization, Writing–original draft. TW: Writing–review and editing. CH: Writing–review and editing. NZ: Writing–review and editing.
The author(s) declare financial support was received for the research, authorship, and/or publication of this article. Social Science Foundation of Jiangsu Province under Grant 22JD006. Postgraduate Research and Practice Innovation Program of Jiangsu Province under Grant KYCX23_1266.
The authors declare that the research was conducted in the absence of any commercial or financial relationships that could be construed as a potential conflict of interest.
All claims expressed in this article are solely those of the authors and do not necessarily represent those of their affiliated organizations, or those of the publisher, the editors and the reviewers. Any product that may be evaluated in this article, or claim that may be made by its manufacturer, is not guaranteed or endorsed by the publisher.
Almeida, G. de O., and Zouain, D. M. (2016). E-government impact on business and entrepreneurship in high-upper-middle- and lower-income countries from 2008 to 2014: a linear mixed model approach. Glob. Bus. Rev. 17, 743–758. doi:10.1177/0972150916645485
Chang, T., Zivin, J. G., Gross, T., and Neidell, M. (2016). Particulate pollution and the productivity of pear packers. Am. Econ. J. Econ. Policy 8, 141–169. doi:10.1257/pol.20150085
Chen, Z., Kahn, M. E., Liu, Y., and Wang, Z. (2018). The consequences of spatially differentiated water pollution regulation in China. J. Environ. Econ. Manage 88, 468–485. doi:10.1016/j.jeem.2018.01.010
Das, A., and Das, S. S. (2022). E-government and entrepreneurship: online government services and the ease of starting business. Inf. Syst. Front. 24, 1027–1039. doi:10.1007/s10796-021-10121-z
Dhaoui, I. (2022). E-government for sustainable development: evidence from MENA countries. J. Knowl. Econ. 13, 2070–2099. doi:10.1007/s13132-021-00791-0
Ebenstein, A., Fan, M., Greenstone, M., He, G., Yin, P., and Zhou, M. (2015). Growth, pollution, and life expectancy: China from 1991-2012. Pittsburgh: American Economic Review. doi:10.1257/aer.p20151094
Gan, T., Liang, W., Yang, H., and Liao, X. (2020). The effect of Economic Development on haze pollution (PM2.5) based on a spatial perspective: urbanization as a mediating variable. J. Clean. Prod. 266, 121880. doi:10.1016/j.jclepro.2020.121880
Gao, X., Song, Y., and Zhu, X. (2013). Integration and coordination: advancing China’s fragmented e-government to holistic governance. Gov. Inf. Q. 30, 173–181. doi:10.1016/j.giq.2012.12.003
Guo, S., Hu, M., Zamora, M. L., Peng, J., Shang, D., Zheng, J., et al. (2014). Elucidating severe urban haze formation in China. Proc. Natl. Acad. Sci. U. S. A. 111, 17373–17378. doi:10.1073/pnas.1419604111
Hochstetter, J., Vásquez, F., Diéguez, M., Bustamante, A., and Arango-López, J. (2023). Transparency and E-government in electronic public procurement as sustainable development. Sustain. Switz. 15, 4672. doi:10.3390/su15054672
Huang, R. J., Zhang, Y., Bozzetti, C., Ho, K. F., Cao, J. J., Han, Y., et al. (2015). High secondary aerosol contribution to particulate pollution during haze events in China. Nature 514, 218–222. doi:10.1038/nature13774
Jiang, B., Li, Y., and Yang, W. (2020). Evaluation and treatment analysis of air quality including particulate pollutants: a case study of Shandong province, China. Int. J. Environ. Res. Public Health 17, 9476. doi:10.3390/ijerph17249476
Khan, A., and Krishnan, S. (2021). Moderating effects of business-systems corruption on corruption in basic national institutions and electronic government maturity: insights from a dynamic panel data analysis. Int. J. Inf. Manage 59, 102349. doi:10.1016/j.ijinfomgt.2021.102349
Krishnan, S., Teo, T. S. H., and Lim, V. K. G. (2013). Examining the relationships among e-government maturity, corruption, economic prosperity and environmental degradation: a cross-country analysis. Inf. Manag. 50, 638–649. doi:10.1016/j.im.2013.07.003
Lim, C.-H., Ryu, J., Choi, Y., Jeon, S. W., and Lee, W.-K. (2020). Understanding global PM2.5 concentrations and their drivers in recent decades (1998–2016). Environ. Int. 144, 106011. doi:10.1016/j.envint.2020.106011
Lin, F. (2017). Trade openness and air pollution: city-level empirical evidence from China. China Econ. Rev. 45, 78–88. doi:10.1016/j.chieco.2017.07.001
Maheshwari, B., Pinto, U., Akbar, S., and Fahey, P. (2020). Is urbanisation also the culprit of climate change? – Evidence from Australian cities. Urban Clim. 31, 100581. doi:10.1016/j.uclim.2020.100581
Majeed, M. T., and Malik, A. (2016). E-government, financial development and economic growth. Pak. J. Appl. Econ. 26.
Nunn, N., and Qian, N. (2014). US food aid and civil conflict. Am. Econ. Rev. 104, 1630–1666. doi:10.1257/aer.104.6.1630
Pastor, J. T., and Lovell, C. A. K. (2005). A global Malmquist productivity index. Econ. Lett. 88, 266–271. doi:10.1016/j.econlet.2005.02.013
Shamim, F. (2007). The ICT environment, financial sector and economic growth: a cross-country analysis. J. Econ. Stud. 34, 352–370. doi:10.1108/01443580710817452
Shao, D., Ishengoma, F. R., Alexopoulos, C., Saxena, S., Nikiforova, A., and Matheus, R. (2023). Integration of IoT into e-government. Foresight 25, 734–750. doi:10.1108/FS-04-2022-0048
Ullah, A., Pinglu, C., Ullah, S., Qaisar, Z. H., and Qian, N. (2022). The dynamic nexus of E-Government, and sustainable development: moderating role of multi-dimensional regional integration index in Belt and Road partner countries. Technol. Soc. 68, 101903. doi:10.1016/j.techsoc.2022.101903
Wu, L., Wan, X., Jahanger, A., Li, M., Murshed, M., and Balsalobre-Lorente, D. (2023). Does the digital economy reduce air pollution in China? A perspective from industrial agglomeration. Energy Rep. 9, 3625–3641. doi:10.1016/j.egyr.2023.02.031
Wu, W., Zhang, M., and Ding, Y. (2020). Exploring the effect of economic and environment factors on PM2.5 concentration: a case study of the Beijing-Tianjin-Hebei region. J. Environ. Manage 268, 110703. doi:10.1016/j.jenvman.2020.110703
Yan, Y. C., and Lyu, S. J. (2023). Can e-government reduce local governments’ financial deficits? Analysis based on county-level data from China. Gov. Inf. Q. 40, 101812. doi:10.1016/j.giq.2023.101812
Yang, W., Hu, Y., Ding, Q., Gao, H., and Li, L. (2023). Comprehensive evaluation and comparative analysis of the green development level of provinces in eastern and western China. Sustain. Switz. 15, 3965. doi:10.3390/su15053965
Yang, X., Wu, H., Ren, S., Ran, Q., and Zhang, J. (2021). Does the development of the internet contribute to air pollution control in China? Mechanism discussion and empirical test. Struct. Change Econ. Dyn. 56, 207–224. doi:10.1016/j.strueco.2020.12.001
Yifan, G., and Bei, L. (2021). Influencing factors and multiple paths of construction ability of digital government: qualitative comparative analysis based on 31 Chinese provinces. Procedia Comput. Sci. 199, 1213–1220. doi:10.1016/j.procs.2022.01.154
Zhang, J., Zeng, W., Wang, J., Yang, F., and Jiang, H. (2017). Regional low-carbon economy efficiency in China: analysis based on the Super-SBM model with CO2 emissions. J. Clean. Prod. 163, 202–211. doi:10.1016/j.jclepro.2015.06.111
Zhang, Q., Zheng, Y., Tong, D., Shao, M., Wang, S., Zhang, Y., et al. (2019). Drivers of improved PM 2.5 air quality in China from 2013 to 2017. Proc. Natl. Acad. Sci. 116, 24463–24469. doi:10.1073/pnas.1907956116
Zhang, Y., and Kimathi, F. A. (2022). Exploring the stages of E-government development from public value perspective. Technol. Soc. 69, 101942. doi:10.1016/j.techsoc.2022.101942
Keywords: e-government, air quality, PM2.5, innovation, green development
Citation: Han H, Wu T, Hai C and Zhou N (2023) The impact of E-government on air quality: new evidence from China. Front. Environ. Sci. 11:1294039. doi: 10.3389/fenvs.2023.1294039
Received: 14 September 2023; Accepted: 11 December 2023;
Published: 21 December 2023.
Edited by:
Ravindra Khaiwal, Post Graduate Institute of Medical Education and Research (PGIMER), IndiaReviewed by:
Worradorn Phairuang, Kanazawa University, JapanCopyright © 2023 Han, Wu, Hai and Zhou. This is an open-access article distributed under the terms of the Creative Commons Attribution License (CC BY). The use, distribution or reproduction in other forums is permitted, provided the original author(s) and the copyright owner(s) are credited and that the original publication in this journal is cited, in accordance with accepted academic practice. No use, distribution or reproduction is permitted which does not comply with these terms.
*Correspondence: Chenxi Hai, MTAzNTM0MTc4MkBxcS5jb20=; Nianchi Zhou, MjQ5NzE3MzIyOUBxcS5jb20=
Disclaimer: All claims expressed in this article are solely those of the authors and do not necessarily represent those of their affiliated organizations, or those of the publisher, the editors and the reviewers. Any product that may be evaluated in this article or claim that may be made by its manufacturer is not guaranteed or endorsed by the publisher.
Research integrity at Frontiers
Learn more about the work of our research integrity team to safeguard the quality of each article we publish.