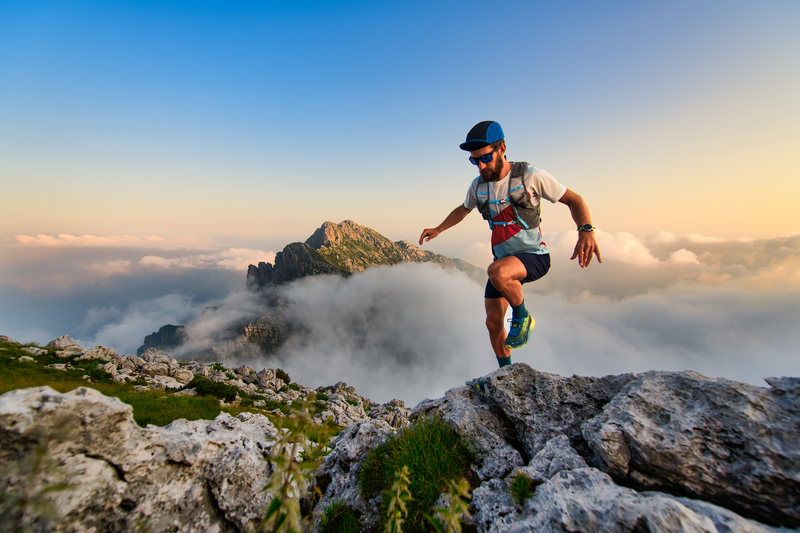
95% of researchers rate our articles as excellent or good
Learn more about the work of our research integrity team to safeguard the quality of each article we publish.
Find out more
EDITORIAL article
Front. Environ. Sci. , 08 August 2023
Sec. Environmental Economics and Management
Volume 11 - 2023 | https://doi.org/10.3389/fenvs.2023.1269419
This article is part of the Research Topic Fuzzy Mathematical Model and Optimization in Digital Green Innovation for Industry 5.0 View all 14 articles
Editorial on the Research Topic
Fuzzy mathematical model and optimization in digital green innovation for Industry 5.0
Since the implementation of the Paris Agreement, countries worldwide have made significant efforts to promote green development and transition towards a low-carbon economy. Among the various sectors, the global manufacturing industry stands out as the largest contributor, responsible for approximately 30% of total carbon emissions (Huang and Zhang, 2023). The manufacturing industry plays a critical role in determining the success or failure of global climate change mitigation efforts through its actions aimed at reducing carbon emissions. As the world embraces the rapid advancement of artificial intelligence, its impact on the industrial landscape cannot be underestimated. The integration of intelligent manufacturing applications and the new generation of artificial intelligence is unlocking tremendous value from data, which serves as a core element of the digital economy. This convergence has the potential to revolutionize the way manufacturing processes are optimized and managed, leading to more sustainable and environmentally friendly practices (Singh et al., 2023). Digitization technology plays a pivotal role in empowering green manufacturing by providing a crucial pathway for reducing emissions within the manufacturing industry.
Digital technology plays a significant role in optimizing various aspects of the manufacturing process, including product design, production efficiency, material waste reduction, and energy emissions minimization. The integration of intelligent transformation and green upgrading emphasizes leveraging digital technology to enhance energy, resource, and environmental management within the manufacturing industry (Yin et al., 2022). This approach enables the manufacturing industry to explore new economic growth opportunities in the field of green and low-carbon initiatives. Green innovation, propelled by artificial intelligence and big data, not only facilitates the digital transformation and green upgrading of the manufacturing sector, but also brings forth various benefits, including environmental preservation, climate mitigation, and economic impact (Dong et al., 2023). Digital green innovation is the integration of information, computation, communication, and connectivity technologies to reshape digital resources and incorporate digital technology into green innovation. This integration leads to the development of new products, enhancements in production processes, changes in organizational structures, and the creation and modification of business models. All of these advancements have a profound positive impact on the environment (Yin and Yu, 2022).
The introduction of the artificial intelligence era has sparked considerable interest in the field of intelligent manufacturing, particularly in relation to fuzzy set theory. This interest is observed both in academic and industrial sectors. The components within the digital green innovation system of the manufacturing industry are intricately interconnected, giving rise to an integrated entity that is characterized by, complexity, non-linearity, and hysteresis (Mahmood et al., 2023; Singh et al., 2023). However, traditional precise mathematical methods have certain limitations when it comes to expressing this system in a fuzzy manner (Garg et al., 2022). Therefore, it is crucial to utilize a dynamic analysis tool that is capable of capturing these characteristics. Fuzzy set theory has emerged as a valuable tool in addressing this challenge and provides valuable insights into the complex landscape of digital green innovation management. Consequently, the application of fuzzy system models plays a pivotal role in addressing the challenges associated with digitization and green innovation management, thereby promoting the advancement of intelligent manufacturing and green manufacturing (Dong et al., 2022).
This Research Topic significantly contributes to the integration of fuzzy mathematics and digital green innovation within the context of Industry 5.0. The research focuses on two main areas: the use of fuzzy mathematics models in environmental economics and management, and the exploration of digital green innovation. In terms of environmental economics and management, the research examines the intersection between fuzzy mathematics-based tools such as artificial intelligence, big data, and the internet of things, and their impact on the green economy. The goal is to understand how these tools can be harnessed to promote sustainable practices and enhance environmental management. Regarding environmental management, the research centers on the application of fuzzy mathematics-based digital technologies in fostering green intelligent manufacturing through digital green innovation management. It highlights the critical role that fuzzy mathematics plays in facilitating the green development of the manufacturing industry. Through this research, we aim to the gap between fuzzy mathematics and digital green innovation, ultimately contributing to the advancement of Industry 5.0 and the promotion of sustainable practices in the manufacturing sector.
The Research Topic reveals the international experiences in the application of fuzzy mathematics and digital green innovation management. Within Industry 5.0, the advancement of intelligent manufacturing and the transition to green manufacturing are facilitated by the implementation of digital green innovation technologies grounded in fuzzy mathematics theory. The Research Topic of papers pertaining to this research theme extensively examines and highlights the paramount significance of fuzzy mathematics and its derivative models in enabling digital and green innovations. These approaches are essential for effectively navigating the challenges and opportunities presented by the era of artificial intelligence and the imperative of carbon reduction.
In terms of environmental economics. Zhang et al. delved into the correlation between digital transformation and sustainable development within enterprises. It was posited that digital transformation could mitigate challenges such as knowledge circulation barriers, brain drain among technical personnel, industry monopolies, disadvantages faced by female board members, and the aging workforce, consequently injecting fresh momentum into the sustainable development of enterprises. Mingkai et al. examined the influencing factors impacting the harmonized development of green innovation and the digital economy using a fuzzy set qualitative comparative analysis method. The researchers found that a single influencing factor alone is not sufficient as a prerequisite for achieving a high level of coordination between green innovation and the digital economy. Consequently, the authors put forth four pathways to enhance the level of coordination between these two domains: human capital + research and development intensity + openness, excluding joint driving; research and development intensity + openness, excluding dual driving; human capital + government subsidy, excluding dual driving; and government subsidy direction. Zhao et al. revealed the influence of the digital economy on carbon emissions intensity. The authors assert the presence of a long-term equilibrium relationship between the two variables. Specifically, the digital economy exhibits a negative long-term impact on carbon emissions intensity, whereas carbon emissions intensity does not exert a negative impact on the digital economy. Ning et al. conducted an analysis of the influence of environmental liability insurance on green innovation within enterprises. Moreover, it examined the driving role of environmental liability insurance in fostering green innovation through the lens of green governance. Environmental liability insurance plays a crucial role in enhancing the capacity for green innovation by alleviating financing constraints and diminishing agency costs. Zhu et al. used the Entropy-CoCoSo method to assess the level of business environment for inter-provincial economic development. In the proposed Entropy-CoCoSo evaluation framework, the weight of each indicator is determined using the entropy method, and the comprehensive evaluation of the business environment for each province is achieved through a combination of compromise solution. Ashraf et al. proposed a complex intuitional fuzzy ELECTREE method to evaluate the impact of industrial pollution on the environment. This study focuses on four common criteria: air pollution, water pollution, land degradation, and waste generation. The proposed method is effective as it allows for the use of multiple criteria for multi-attribute decision-making and also allows us to visualize the ranking of members in a two-dimensional manner. Yang et al. developed a bipolar complex fuzzy set methodology to analyze the impact of various pollution on the environment and evaluate the most harmful types of pollutants. The authors believe that air pollution and water pollution are currently key factors affecting environmental safety.
In terms of environmental management, Hou et al. formulated the concept of digital green and proposed a novel Maclaurin Symmetric Mean operator based on interaction operational laws for T-spherical fuzzy information to assess digital green technologies. The authors believe that the concept of digital green refers to the use of digital technologies, techniques, and procedures in the field of ecological or sustainable protection. Khan et al. developed an aggregation operator to solve the green supplier selection problem with q-rung orthopair fuzzy hypersoft information. Although fuzzy sets can handle incomplete information in various real-world problems, they cannot handle all types of uncertainty, such as incomplete data and uncertain data. The structure of q-rung orthopair fuzzy hypersoft sets is a very effective processing tool. Ashraf et al. extended the evaluation based on the distance from the average solution approach under Pythagorean fuzzy Z-numbers. In this study, Pythagorean fuzzy Z-number arithmetic aggregation operators are proposed to assist manufacturing producers while incorporating uncertainty and reliability. Junjie et al. revealed the impact mechanism of social entrepreneurship opportunity recognition and resource mobilization on growth performance. Social entrepreneurship, as a way for businesses to fulfill their social responsibilities, is becoming one of the important means to address social responsibility conflicts. The more ties in the entrepreneurial social network, the stronger the impact of social entrepreneurship opportunity recognition on the growth performance of business start-ups. Guang-lin and Tao investigated the correlation between management capability and the processes of digital transformation and green technology innovation. The findings indicate a notable and positive relationship between management capability and both green technology innovation and digital transformation. In addition, internal control was identified as a significant and positive moderating factor within this relationship. Sun screened the factors that influence green innovation risk within the global value chain. The characteristic root method of group decision-making was employed to establish an evaluation index system for assessing the risks associated with manufacturing green innovation. The identified factors include the global proportion of investment in green R&D personnel, the global proportion of investment in green R&D funding, the stability of global manufacturing green R&D, the challenges associated with international transfer of green technologies, and international protection of green technology patents.
Therefore, these articles successfully address the gap in digital green innovation management by utilizing the principles of fuzzy mathematics. They offer a comprehensive understanding of how digital green innovation is distributed within Industry 5.0’s environmental economy and management in the age of artificial intelligence. Moreover, they present a holistic perspective on environmental economy and environmental management, highlighting the interconnectedness and interdependence of these facets.
The Research Topic introduces the concept of “digital green innovation”, which refers to the use of digital technology in implementing green innovation practices. These papers offer valuable insights into the relationship between fuzzy mathematical theory and digital green development. The ultimate goal is to assist industries in adapting to the complexities of the AI era and the inevitable shift towards decarbonization. Furthermore, this research explores various avenues within the realms of digital innovation and green innovation, systematically expanding upon different directions. It investigates the reasons and phases of digital green innovation, aiming to shed light on the crucial aspects of this process. Moving forward, there is a pressing need for further exploration new industrial intelligent technologies that are applicable to large-scale green complex industrial systems. The development of such large-scale modeling technology is expected to have a transformative impact in tackling highly intricate problems within the domain of industrial intelligence and greening.
SY: Conceptualization, Writing–original draft, Writing–review and editing, Conceptualization, Writing–original draft, Writing–review and editing. DP: Investigation, Resources, Writing–review and editing, Investigation, Resources, Writing–review and editing. KU: Resources, Writing–review and editing, Resources, Writing–review and editing. HG: Resources, Writing–review and editing, Resources, Writing–review and editing.
This research was funded by Top Young Talents Scientific Research Project of Higher Education in Hebei Province grant number (BJ2021084).
The guest editors appreciate all reviewers, editors, and the Editor-in-Chief for their continuous assistance in making this Research Topic a success.
The authors declare that the research was conducted in the absence of any commercial or financial relationships that could be construed as a potential conflict of interest.
The author(s) declared that they were an editorial board member of Frontiers, at the time of submission. This had no impact on the peer review process and the final decision.
All claims expressed in this article are solely those of the authors and do not necessarily represent those of their affiliated organizations, or those of the publisher, the editors and the reviewers. Any product that may be evaluated in this article, or claim that may be made by its manufacturer, is not guaranteed or endorsed by the publisher.
Dong, T., Yin, S., and Zhang, N. (2022). New energy-driven construction industry: digital green innovation investment project selection of photovoltaic building materials enterprises using an integrated fuzzy decision approach. Systems 11 (1), 11. doi:10.3390/systems11010011
Dong, T., Yin, S., and Zhang, N. (2023). The interaction mechanism and dynamic evolution of digital green innovation in the integrated green building supply chain. Systems 11 (3), 122. doi:10.3390/systems11030122
Garg, H., Ahmad, A., Ullah, K., Mahmood, T., and Ali, Z. (2022). Algorithm for multiple attribute decision-making using T-spherical fuzzy Maclaurin symmetric mean operator. Iran. J. Fuzzy Syst. 19 (6), 111–124. doi:10.22111/IJFS.2022.7215
Huang, Y., and Zhang, Y. (2023). Digitalization, positioning in global value chain and carbon emissions embodied in exports: evidence from global manufacturing production-based emissions. Ecol. Econ. 205, 107674. doi:10.1016/j.ecolecon.2022.107674
Mahmood, T., Rehman, U. U., and Naeem, M. (2023). Prioritization of strategies of digital transformation of supply chain employing bipolar complex fuzzy linguistic aggregation operators. IEEE Access 11, 3402–3415. doi:10.1109/access.2023.3234117
Singh, A., Madaan, G., Hr, S., and Kumar, A. (2023). Smart manufacturing systems: a futuristics roadmap towards application of industry 4.0 technologies. Int. J. Comput. Integr. Manuf. 36 (3), 411–428. doi:10.1080/0951192x.2022.2090607
Yin, S., and Yu, Y. (2022). An adoption-implementation framework of digital green knowledge to improve the performance of digital green innovation practices for industry 5.0. J. Clean. Prod. 363, 132608. doi:10.1016/j.jclepro.2022.132608
Yin, S., Zhang, N., Ullah, K., and Gao, S. (2022). Enhancing digital innovation for the sustainable transformation of manufacturing industry: a pressure-state-response system framework to perceptions of digital green innovation and its performance for green and intelligent manufacturing. Systems 10 (3), 72. doi:10.3390/systems10030072
Keywords: environmental economy, environmental management, digital green innovation, fuzzy mathematics, Industry 5.0, sustainable development
Citation: Yin S, Pamucar D, Ullah K and Garg H (2023) Editorial: Fuzzy mathematical model and optimization in digital green innovation for Industry 5.0. Front. Environ. Sci. 11:1269419. doi: 10.3389/fenvs.2023.1269419
Received: 30 July 2023; Accepted: 03 August 2023;
Published: 08 August 2023.
Edited and reviewed by:
Xingwei Li, Sichuan Agricultural University, ChinaCopyright © 2023 Yin, Pamucar, Ullah and Garg. This is an open-access article distributed under the terms of the Creative Commons Attribution License (CC BY). The use, distribution or reproduction in other forums is permitted, provided the original author(s) and the copyright owner(s) are credited and that the original publication in this journal is cited, in accordance with accepted academic practice. No use, distribution or reproduction is permitted which does not comply with these terms.
*Correspondence: Shi Yin, c2h5c2hpMDMxNEAxNjMuY29t
†ORCID: Shi Yin, orcid.org/0000-0001-6885-7412
Disclaimer: All claims expressed in this article are solely those of the authors and do not necessarily represent those of their affiliated organizations, or those of the publisher, the editors and the reviewers. Any product that may be evaluated in this article or claim that may be made by its manufacturer is not guaranteed or endorsed by the publisher.
Research integrity at Frontiers
Learn more about the work of our research integrity team to safeguard the quality of each article we publish.