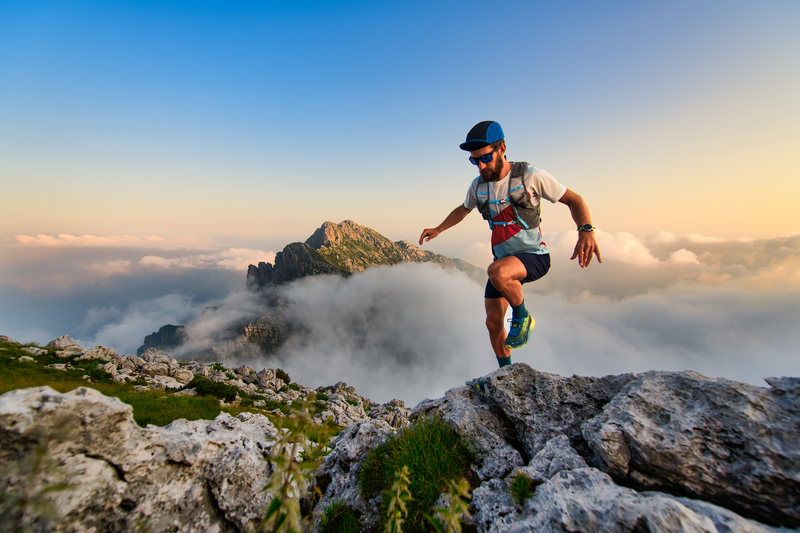
94% of researchers rate our articles as excellent or good
Learn more about the work of our research integrity team to safeguard the quality of each article we publish.
Find out more
ORIGINAL RESEARCH article
Front. Environ. Sci. , 17 October 2023
Sec. Land Use Dynamics
Volume 11 - 2023 | https://doi.org/10.3389/fenvs.2023.1261295
This article is part of the Research Topic Realization of Ecological Product Value, Land Use Change and Environment View all 11 articles
Vegetation cover is a crucial indicator of biodiversity and ecological processes, but there are still uncertainties about the factors driving changes in vegetation. In this study, we conducted a comprehensive analysis of vegetation cover changes in Sichuan Province from 2000 to 2020 using Formation Vegetation Cover (FVC) derived from MODIS13Q1 data. Our results revealed a consistent increase in vegetation FVC, rising from 0.506 to 0.624 over the 21-year period, with an annual growth rate of 0.0028. The turning point in this growth occurred in 2006. Of significance, the expansion of vegetation covered a substantial portion, accounting for 84.76%, while the decrease constituted 13%. Elevation proved to be an effective explanatory factor, with a coefficient of 0.417, indicating its role in explaining vegetation cover changes. It is important to note that FVC trends and averages exhibited distinct patterns concerning elevation, land use, population density, topography, and soil type, while their correlation with meteorological factors was relatively weak. Concurrently, the increase in construction and urban development had a negative impact on vegetation cover.
Surface vegetation, a cornerstone of Earth’s terrestrial ecosystem, serves as a vital indicator of ecosystem health and interconnects various natural elements like the atmosphere and soil (Du et al., 2015; Kong et al., 2018; Zhao et al., 2018). In recent decades, global changes have led to significant shifts in vegetation cover at regional and continental scales (Kotharkar et al., 2016; Pang et al., 2017). This has spurred a critical need to comprehensively study long-term changes in vegetation cover to better understand spatial and temporal dynamics in terrestrial ecosystems. Such understanding is crucial for maintaining ecosystem equilibrium in the face of environmental changes over the past century (Fang et al., 2018). Remote sensing offers a valuable means to monitor vegetation cover dynamics with high precision and frequency across different spatial and temporal scales (Piao et al., 2003; Jalonen et al., 2014). Formation Vegetation Cover (FVC) is adept at tracking changes in ground-level vegetation (Zhang et al., 2003). For instance, Du et al. (2015) noted a consistent increase in vegetation activity in Xinjiang during spring, summer, and autumn over the past 3 decades. Zhao et al. (2019) further supported this observation, documenting a yearly rise in vegetation cover from 2000 to 2014. Notably, He’s work highlighted the significant impact of farmland-to-forest initiatives on vegetation dynamics. Additionally, a strong correlation was found between cumulative afforestation efforts and vegetation cover in the Yan’an and Yulin regions from 2000 to 2013. In a separate study, Peng et al. (2019) conducted a geographic analysis of natural factors influencing vegetation dynamics in Sichuan from 2000 to 2015. This research revealed key factors driving vegetation growth, enriching our understanding of the interaction between natural factors and the mechanisms behind vegetation change. It is worth noting that many of these studies relied on the GIMMS dataset, which, despite its long availability, has limitations due to its coarse resolution. This limitation may result in the loss of critical spatial details at the regional level. Furthermore, acquiring detailed Landsat satellite imagery at a large scale demands substantial computational resources and time, which led to the selection of the MODIS dataset with higher resolution. The computation of vegetation cover was carried out using the Google Earth Engine (GEE) platform, a powerful cloud-based computational facility designed for processing and analyzing extensive spatial datasets (Mutanga, O. and Kumar, 2019). This platform enables efficient monitoring of vegetation cover changes through high-resolution imagery, despite its limited temporal scope (Kumar, L. and Mutanga, O., 2018).
Moreover, it is imperative to acknowledge that the intricate causative linkages between influential factors and the dynamics of vegetation cover exhibit a multifaceted and non-linear character (Zhang et al., 2018). Preceding scholarly inquiries have conventionally resorted to correlation and residual analyses in their pursuit of unraveling the driving forces governing vegetation changes (Zhao et al., 2021; Jiang et al., 2022). However, these conventional approaches often fall short in elucidating the nuanced, non-linear interdependencies among multiple impact factors, notably those entwined with anthropogenic influences and climate fluctuations. In response to these inherent limitations, non-linear methodologies have been employed as an indispensable toolset to disentangle the intricate tapestry of driving mechanisms underlying changes in vegetation cover (Jiang et al., 2022). Foremost among these methodologies are Geodetectors, which represent spatially-focused analytical methods adept at discerning the spatial variances inherent in these dynamics and unveiling their causative underpinnings (Wang et al., 2017). Notably, a multitude of studies have effectively applied Geodetectors methodologies across various scales and encompassing a diverse array of influential factors, yielding comprehensive insights into the driving forces steering alterations in vegetation cover (Huo et al., 2021). Hence, predicated on the intrinsic spatial heterogeneity characterizing geographical phenomena, Geodetectors models emerge as a compelling and nuanced approach, well-suited to furnishing cogent explanations for the manifold transformations in vegetation cover.
Sichuan Province, situated in the upper reaches of the Yangtze River, holds strategic significance as a vital water source and ecological protector within the Yangtze River Basin. Given its climatic diversity and substantial variations in topography across different regions, Sichuan Province exhibits notable disparities in surface vegetation cover and ecological conditions. This study utilizes remote sensing data from MODIS, processed through the Google Earth Engine (GEE) platform. Employing various analytical methods, including the image element dichotomous model, coefficient of variation analysis, and Geodetectors, it aims to identify complex patterns and temporal changes in vegetation cover across Sichuan Province. The study also explores the primary factors driving these changes. The results are significant for advancing ecological restoration efforts in the region and providing guidance for the preservation of the local environment.
Located in the southwest region of inland China, Sichuan Province spans a vast area of 485,000 square kilometers and is situated between 26°03′-34°19′N latitude and 97°21′-108°12′E longitude, predominantly covering the upper reaches of the Yangtze River (Figure 1). The province’s landscape exhibits significant variations from east to west, characterized by a complex and diverse topography. Residing in the transitional zone between the Qinghai-Tibetan Plateau, representing the initial terrain step of the Chinese mainland, and the middle and lower reaches of the Yangtze River Plain, constituting the third step, Sichuan Province boasts an extensive range of elevations, featuring a prominent west-to-east elevation gradient. Its terrain comprises mountains, hills, plains, basins, and plateaus. Notably, Sichuan Province experiences three major climatic zones. In central Sichuan, the Sichuan Basin is characterized by a humid central subtropical climate. Meanwhile, the mountains in southwest Sichuan exhibit a semi-humid subtropical climate, while northwest Sichuan is characterized by an alpine plateau climate (Wang, H, X and Liu, C, M.; 2000). Furthermore, the province is home to numerous rivers that primarily form part of the extensive Yangtze River system, with major tributaries including the Yalong, Min, and Dadu Rivers. Lastly, the natural vegetation of Sichuan Province encompasses eight vegetation types, 18 phyla groups, and 48 group groups, contributing to its relatively rich biological resources and unique ecological value.
The data sources and processing of the article are shown in Table 1.
This model operates on the idea that an image’s surface can be divided into two parts–one with vegetation and one without (Figure 2) (He et al., 2023; Xu et al., 2023). It suggests that the spectral information captured by remote sensors is a blend of these two parts, with their importance determined by their relative surface areas within the image. For example, vegetation cover can be seen as the weight assigned to the vegetation component based on its area relative to the total image area. (Kalisa et al., 2019; Li et al., 2023). Its calculation formula is
The Enhanced Vegetation Index (EVI), which adjusts for the absorption of red light by residual aerosols by comparing red and blue light transmission through the aerosol, offers a more accurate representation of vegetation changes (Wu et al., 2020).
The coefficient of variation CV, also known as the coefficient of dispersion, is a normalised measure of the degree of dispersion of a probability distribution (Deng et al., 2017; Kinjal C and Ankit T, 2023). The coefficient is a dimensionless quantity that does not need to be referenced to the mean of the data, so when comparing the stability of vegetation cover, the ratio of the standard deviation to the mean (relative value) can be used for comparison (He et al., 2023). It is calculated as:
where s is the standard deviation and μ is the mean.
The Theil-Sen Median and Mann-Kendall tests are non-parametric statistical methods introduced (Hirsch and Slack., 1984). The Theil-Sen Median is adept at identifying trends and quantifying changes in a time series but does not independently determine the statistical significance of these trends. In contrast, the Mann-Kendall test is employed to assess the statistical significance of temporal trends in a time series, and the Kendall test specifically evaluates the significance of these trends. The combination of these two non-parametric methods has become a common approach in remote sensing for analyzing trends in time series data (De Jong et al., 2011; Gocic and Trajkovic, 2013).
The Theil-Sen median method, also known as Sen slope estimation, is a robust, non-parametric statistical approach to trend analysis (Xie et al., 2022; Tabari et al., 2011; Xiang et al., 2023). The method is computationally efficient, insensitive to measurement error and niche data, and suitable for trend analysis of long time series data (Yue et al., 2002; Li et al., 2020). Its calculation formula is:
If β is greater than zero, it indicates an increasing trend in vegetation cover, and vice versa for a decreasing trend.
The Mann-Kendall (MK) test is a non-parametric time series trend test (Wang et al., 2019; He et al., 2022) that does not require measurements to follow a normal distribution, is unaffected by missing values and outliers, and is suitable for testing whether long time series data are indeed significant (Wang et al., 2010; Li et al., 2020; Guo and Bryan, 2022). However, it is not applicable to detect sequences with multiple mutation sites. The procedure is as follows: for a sequence
where sgn () is the sign function, calculated as:
The trend test is performed with the test statistic Z. The calculation of the Z-value is as follows:
where var is computed by the formula
where n is the number of data in the sequence; m is the number of nodes (repeating data groups) in the sequence.
Again, a bilateral trend test was used to find the critical value Z1-α/2 in the normal distribution table at the given significance level. if |Z| = Z1-α/2, the original hypothesis was accepted, i.e., the trend was not significant, and if |Z|>Z1-α/2, the original hypothesis was rejected, i.e., the trend was considered significant. This paper refers to the trend classification of Yuan et al. (2013), where if the significance level is α = 0.05, the critical value is Z1-α/2 = ±1.96, then the absolute value of Z is greater than 1.96, indicating that the trend has passed the significance test at the 95% confidence level. The method of distinguishing the significance of the trends is shown in Table 2.
The Pettitt test, a statistical technique employed for identifying the inflection point within a time series, discerns pronounced shifts in the trend exhibited by the sequence over an extended temporal span (Radu et al., 2022; Zhao et al., 2023). A notable advantage intrinsic to this method lies in its independence from the constraint of adhering to specific probability distributions within the sample sequence (Carla et al., 2023). A Pettitt mutation test yielding a statistic K value below the 0.05 significance threshold serves as compelling evidence of a substantively significant mutation point within the sequence under consideration (Karen et al., 2019).
If moment
If the statistic
Geographical detectors can either test for spatial heterogeneity of a single variable or detect possible causal relationships between two variables by testing them (Song et al., 2020; Zhang et al., 2020). It consists of four main components: factor detection, interaction detection, risk zone detection and ecological detection (Wang et al., 2016; Wang et al., 2017; Huang et al., 2023). In this paper, the interaction detection tool and the Geodetector interaction detection tool were selected to analyse the influence of vegetation cover drivers in Sichuan Province.
Factor detection: detection of the spatial heterogeneity of Y (FVC); and detection of how much of the spatial heterogeneity of attribute Y is explained by a given factor X (each detection factor) (Lei et al., 2023). Using the q-value metric, the expressions are:
where:
Interaction detection: different detection factors were used for two-by-two interactions on FVC. The type of interaction between factors was determined by comparing the interaction q-values with the single factor q-values, and the interaction was judged as shown in Table 3.
Correlation analysis refers to the analysis of the variable elements relevant to each influence factor, and calculates the correlation coefficient between each influence factor and FVC on a frame-by-frame basis to measure the closeness of the correlation of each factor (Liu et al., 2020). Its calculation formula is:
Where: R_XY is the correlation coefficient between the two variables, between 1 and -1, where 1 indicates a perfectly positive correlation between the variables, 0 indicates no correlation, and −1 indicates a perfectly negative correlation; xi is the meteorological factor in year i;
This study aimed to examine the inter-annual variation trend of vegetation fractional cover (FVC) across Sichuan Province spanning from 2000 to 2020. The investigation revealed a consistent upward trend in FVC, as evidenced by an average growth rate of 0.0028/a over the past 21 years. Strikingly, the mean value of FVC reached its nadir at 0.5061 in 2001, subsequently exhibiting a gradual increase over time and reaching its highest point of 0.6244 in 2020, representing an overall growth of 81.05% during the 21-year period under investigation.
Figure 3 effectively depicts the trends of vegetation cover changes observed within the study area. The graphic indicates that 84.76% of the region exhibited significant or slight improvement in vegetation cover from 2000 to 2020, while 13.23% of the total area experienced various degrees of degradation. Notably, these degraded areas were primarily concentrated in Chengdu City, Yibin City, and other scattered regions, which can be attributed to the rapid urban expansion that has occurred within these regions. As a result, there has been a conversion of land for construction purposes, leading to a corresponding reduction in vegetated areas. However, it is important to acknowledge the significant strides that have been made towards improving vegetation cover within Sichuan Province, which can be attributed to various efforts such as land restoration, afforestation, and the implementation of ecological construction projects. These positive trends are also attributable to relevant policies aimed at promoting ecological conservation and restoration, including the conversion of cultivated land back to forest and grassland.
The average value of vegetation FVC in Sichuan Province during 2000–2020 is 0.60. The spatial distribution of vegetation cover in Sichuan Province shows the characteristics of high in the east and low in the west, high in the south and low in the north, with the eastern region dominated by the Sichuan Basin, with low elevation and extensive deciduous forests; the southern region dominated by the Mountainous areas, with low elevation and extensive deciduous forests.
The coefficient of variation, a measure of spatial variability, ranged from 0 to 0.93 across Sichuan Province’s study area. Based on the prevailing situation of vegetation cover fluctuations, the coefficient of variation was categorized into five distinct classes (refer to Figure 4; Table 4): low fluctuation (<0.05), relatively low variability (0.05≤CV < 0.10), medium variability (0.10≤CV < 0.15), relatively high variability (0.15≤CV < 0.2), and high variability (≥0.2). The mean coefficient of variation determined for the province was 0.1167 (<0.15), indicating a relatively steady rate of variation. However, considerable spatial differences were noted in the degree of variation. High and relatively high variability accounted for 10% of the region and were predominantly evident in districts and counties around Shiqu County and Chengdu City. The low vegetation cover and high variability observed in these regions may be attributed to rapid socio-economic development in the past 2 decades, leading to extensive land use changes and recurrent human activities. Conversely, areas with lower variation accounted for 10% of the total area and were mainly concentrated in Batang County, Derong County, Ganzi County, and Shiqu County. These regions are characterized by high mountain plateaus with moderately low vegetation cover, high altitudes, and colder climatic conditions. Overall, the spatial variability of vegetation cover in Sichuan Province exhibits a complex pattern that reflects the combined influence of geographical and anthropogenic factors on vegetation dynamics across various regions within the province.
FIGURE 4. Spatial distribution of vegetation cover and stability in Sichuan Province from 2000 to 2020.
Figure 5 provides additional insights by indicating that the inception of vegetation cover mutation was observed as early as 2006. The findings suggest a bifurcation in the temporal evolution of vegetation mutation, broadly categorized into two distinct phases. The initial phase spans from 2000 to 2010, predominantly manifesting in the southeastern sector of the nation and the western expanse of the Sichuan Plateau. Subsequently, the second phase, commencing from 2010 onward, is characterized by its prevalence in the northern and southwestern regions of the country, as well as within the transitional zone bridging the Sichuan Basin and the Yunnan-Guizhou Plateau.
In order to probe the impact of various geographical factors on vegetation coverage dynamics in Sichuan Province, a total of 11 pertinent variables were selected for investigation across 4,862 sampling points in the province through Geodetector detection.
Geodetector analysis were employed to calculate and scrutinize the q-values of each geographic factor (Figure 6), subsequently appraising the impact of these factors on vegetation FVC in Sichuan Province. It has been observed that elevation, soil type, and landform type exhibit q-values exceeding 0.3, consequently indicating their remarkable contribution towards the elucidation of changes in vegetation cover and their identification as chief driving forces. Notably, elevation boasts the highest q-value of 0.417, signifying its unparalleled influence over vegetation cover variations. Coupled with this, other geographic factors, including vegetation type, temperature, population density, GDP, precipitation, and land use type, have demonstrated significant explanatory power with q-values greater than 0.05, although they are considered secondary drivers. Conversely, slope and slope direction manifest weaker explanatory capabilities, indicative of their minimal impact on the study area’s vegetation cover changes. In general, geological landforms, comprising of elevation, soil type, and landform type, have exerted the most substantial impact on the integration of vegetation cover changes. Moreover, a thorough investigation of vegetation cover dynamics based on plateau and plain areas reveals the provision of additional insights into vegetation cover dynamics from an elevation perspective through slope, slope orientation, precipitation, and temperature. The relatively low explanatory power of population density, GDP, and land use type may be attributed to frequent ecological engineering measures implemented in the study area, the overall positive trend in vegetation cover growth observed in Sichuan Province, and significant improvements in vegetation cover recovery. Ultimately, the study highlights the preeminent role played by geological landforms, particularly elevation, in shaping vegetation cover dynamics in Sichuan Province, whilst other factors such as vegetation type, temperature, precipitation, and land use contribute collectively to the overall understanding of vegetation cover changes.
The detection of spatial changes in vegetation cover relating to diverse drivers imparts insights into whether the explanatory power of the individual drivers is increasing, decreasing or fluctuating. The Geodetector tool effectively discloses the interplay of detection factors responsible for vegetation cover changes. Figure 7 illustrates the results of the factor interaction detection, revealing a mutually reinforcing and non-linear relationship between the influence of detection factors on vegetation cover in Sichuan Province. Specifically, 1) among natural factors, there is a two-factor amplification of slope with altitude and land use type, and a non-linear amplification with other interacting factors. Additionally, there is a non-linear amplification of slope direction with all other interacting factors. 2) Among human factors, there is a two-factor enhancement of GDP with vegetation type and population density, and a non-linear enhancement with other interaction factors. Moreover, there is a non-linear enhancement of land use type with slope direction, and a two-factor enhancement with other interaction factors.
FIGURE 7. Interactive explanatory power of detection factors in Sichuan Province from 2000 to 2020 (q).
All interactions between factors exhibited q-values surpassing those of individual factors, indicating that the super positioning of multiple factors results in an increased explanatory power of vegetation cover. Notably, crucial drivers displaying a heightened influence on the spatial distribution of vegetation cover in Sichuan Province encompass elevation ∩ vegetation type (q = 0.540), elevation ∩ GDP (q = 0.539), elevation ∩ population density (q = 0.506), and elevation ∩ land use type (q = 0.501). Notably, elevation, as the foremost driver, possesses the highest explanatory power for vegetation cover’s spatial distribution in Sichuan Province when interacting with other detection factors. The human activity factor’s explanatory power is markedly elevated after interplaying with geological and geomorphological factors.
Figure 8 illustrates the discernible trend and mean of FVC for each landform, indicating the highest growth rate of 0.0187/a in the Plain category, while Extremely undulating mountainous hills display the lowest growth rate at 0.0046/a. Hills cover boasts the highest mean FVC value of 0.6865, whereas Extremely undulating mountainous hills demonstrate the lowest mean FVC value of 0.1547. Moreover, the FVC trends and means across every soil type disclose that Man-Made soils exhibit the largest trend with a growth rate of 0.0165/a, whereas High-Mountain-Soils showcase the smallest trend at 0.0076/a. The mean FVC value is notably higher for Iron bauxite soils, registering a value of 0.7642, while High-Mountain-Soils record the lowest mean FVC value of 0.3686.
FIGURE 8. Relationship between vegetation FVC trend, mean value, landform type and soil type in Sichuan Province.
The majority of areas revealed non-significant correlation coefficients between vegetation FVC and both temperature and air temperature, with an increased proportion of areas displaying negative correlation between vegetation FVC and air temperature and precipitation compared to those exhibiting positive correlation (as portrayed in Figure 9; Table 5). Additionally, the relevance of this article is classified by reference to Xie [0]. Notably, the spatial range of the temperature correlation coefficient spans from −0.948 to 0.940, wherein 40.20% and 59.80% of the area correspondingly displays positive and negative correlation with vegetation FVC. Furthermore, 1.52% and 2.55% of the area exhibit significant positive and negative correlation, respectively, and are more widely dispersed across the study area than those for precipitation. In contrast, the spatial extent of the precipitation correlation coefficient ranges from −0.926 to 1, with 41.06% and 58.94% of the area reflecting positive and negative correlation, respectively, and only 0.79% and 1.30% of the area exhibiting significant positive and negative correlation, respectively.
FIGURE 9. Classification of vegetation FVC and climatic factors correlation coefficient in Sichuan Province from 2000 to 2020.
TABLE 5. Distribution of area associated with FVC interannual variation of vegetation and climatic factors in Sichuan Province/%.
The analysis involved computing the mean vegetation cover and its changing trend within elevation zones at 100-m intervals (Figure 10). The results reveal a bifurcated trend in the evolution of Formation Vegetation Cover (FVC). In the initial stage, spanning from 0 to 3,300 m, the trend exhibits fluctuations followed by an increase with rising altitude. Subsequently, in the second stage, commencing above 3,300 m, the FVC change trend reaches its zenith growth rate at 3,300 m, registering a rate of 0.0138/a. Beyond this altitude, the trend experiences a substantial deceleration, gradually approaching stability. The average FVC value demonstrates an ascent followed by a descent, with its peak at 0.8165 occurring at an elevation close to 1,000 m above sea level. As elevation increases, the average FVC steadily diminishes, ultimately converging toward zero.
FIGURE 10. Relationship between vegetation FVC trend, mean value, elevation and population density in Sichuan Province.
Examining the FVC trends and means at all population density levels depicted in Figure 10, it is discernible that the most significant trend appears within densely populated areas, exhibiting a growth rate of 0.0142/a. Conversely, sparsely populated areas display the lowest growth rate of 0.094/a. Moreover, the mean FVC value is notably higher for moderately populated areas, registering a value of 0.7527, whilst extremely sparse regions reflect the lowest mean FVC value of 0.4396.
Upon scrutinizing the alterations in land use types that have transpired in Sichuan Province, as illustrated in Table 6, a clear preponderance of forest and construction lands is discernible. Between 2000 and 2020, the areas for forest land, water sources, construction land, and idle land have substantially augmented, while cultivated land and grassland exhibit noteworthy decline. Notably, arable land and grassland are the primary land conversion categories for forest land, accounting for a transferred area of 21,357.74 km2 and 36,173.38 km2, respectively. Furthermore, the chief conversion category for construction land is arable land, with an area of transferred land amounting to 3,731.06 km2.
The influence of land cover on vegetation FVC is perceptible in the composite trend of FVC variation resulting from land use conversion, as presented in Table 7. The conducted investigation unveils that the overall growth rate of vegetation cover for watersheds, unused, and construction lands in 2020 is considerably inferior in comparison to the growth rate of grassland, forest land, and cropland. Remarkably, the area that has reverted back to forest and grassland in Sichuan Province over the course of 21 years attains 28,359.30 km2, correlating with a total growth rate of 317.62/a. Furthermore, the vegetation cover of unused land has rapidly expanded to 16,375.22 km2, corresponding to a total growth rate of 183.40/a, whilst urban expansion encompasses an area of 5,664.73 km2 with a total growth rate of 63.45/a.
TABLE 7. Proportion of vegetation cover change area of land use type in Sichuan Province from 2000 to 2020.
Between 2000 and 2020, a marked increase in vegetation cover throughout Sichuan Province has been observed, consistently supported by the recent findings of Xu et al. (2022) and Zhu et al. (2022). The spatial distribution of vegetation exhibits an uneven pattern, with higher values concentrated in the eastern and southern regions, while lower values are found predominantly in the western and northern provinces. Nevertheless, overall vegetation cover has shown a relatively stable trend, with more pronounced fluctuations detected in the western Sichuan Plateau and Chengdu City. Areas experiencing degradation are concentrated primarily in Chengdu, Yibin, and scattered locations in their adjacent regions. Notably, Chengdu, as a provincial capital and an economic center, is densely populated, with a significant proportion of available land (94.2%) converted into urban areas. The dominant vegetation type in Chengdu consists of cultivated vegetation, including food crops, fruit forests, and other economically-driven artificial forests. However, human activities significantly influence vegetation cover in this region (Li et al., 2019). The rapid growth of urban areas and population has led to the conversion of a significant portion of land into urban development, consequently reducing vegetation coverage (Ma et al., 2023). This aligns with findings from previous research.
The trends and mean values of FVC change exhibit distinct patterns in relation to elevation, land use, population density, geomorphological type, and soil type (Jiang et al., 2021). Notably, there is a negative correlation between vegetation FVC and both temperature and air temperature, with a relatively weak direct correlation (Li et al., 2012; Xu et al., 2018; Du et al., 2019). It is worth mentioning that as afforestation policies have evolved, the correlation between vegetation growth and climate has diminished (Huang et al., 2020). Wu et al. (2020) have observed that changes in the Enhanced Vegetation Index are minimally correlated with meteorological factors but strongly associated with artificial ecological engineering, signifying that ecological engineering initiatives are the predominant drivers of such variations. The year 2006 marks a pivotal juncture when sudden shifts in vegetation cover occurred. Prior to this year, thermal factors played a substantial role, but post-2006, active interventions like afforestation and terrain modification diminished the influence of vegetation. In studies of vegetation change, it is crucial to monitor human activities that impact vegetation growth to varying degrees (Zhang et al., 2017; Al-bukhari et al., 2018). Additionally, alterations in land use patterns serve as a tangible reflection of human activity. Over the course of 21 years, the area dedicated to returning farmland to forests and grasslands in Sichuan Province expanded to 28,359.30 km2 translating to a notable annual growth rate of 317.62/a. This trend underscores the effectiveness of policies promoting land conversion to forests and grasslands, afforestation endeavors, and desert management in fostering increased vegetation cover within Sichuan Province.
To summarize, the vegetation fractional cover (FVC) in Sichuan Province is influenced by a complex interplay of multiple factors. This study employed geographic probes and correlation analysis to reveal an overall increasing trend in vegetation cover within the province. Notably, elevation, landform type, and soil type were identified as dominant factors that promoted vegetation growth and exerted significant effects on vegetation FVC. The study also introduced a dichotomous image model based on MOD13Q1 data, which proved to be a relatively simple, intuitive, and efficient approach for analyzing vegetation patterns.
This study employed the higher-resolution MODIS dataset, which spans from 2000 onwards. While the GIMMS dataset offers long-term data, it has limitations due to its coarser analysis rate. Alternatively, Landsat satellite imagery, which provides more detailed information on a larger scale, demands substantial computational resources and time. To attain detailed, long-term surface data and detect significant vegetation cover changes over the past decades, we utilized the Google Earth Engine (GEE) platform. Moreover, the image element dichotomous model is a remote sensing estimation model. It is not particularly sensitive to the radiatively corrected images within its remote sensing data. However, it exhibits some limitations when applied solely to the distribution of two components: photosynthetic vegetation end-elements and bare soil, especially in specific regions like desertified areas. To enhance our understanding of the distinct driving mechanisms in different regions of Sichuan Province, future research could consider utilizing the image element trichotomous model. This advanced approach would allow for a more comprehensive study of vegetation, addressing uncertainties in specific regions that may arise from the dichotomous model. Ultimately, it would enable a more precise analysis of vegetation cover trends.
Utilizing MOD13Q1 data, DEM data, and additional relevant data sources, this study sought to investigate the spatial and temporal dynamics of vegetation cover in Sichuan Province from 2000 to 2020. Further, it explored the driving forces behind these changes by employing the Geodetector model and correlation analysis. The key findings of this research are as follows:
(1) The vegetation Formation Vegetation Cover (FVC) in Sichuan Province displayed a clear pattern from 2000 to 2020, with higher values in the eastern and southern regions and lower values in the western and northern areas. The overall coefficient of variation (CV) was 0.1167, indicating relatively stable changes (CV < 0.15), with the earliest abrupt change occurring in 2006. Approximately 20% of the region exhibited significant fluctuations, primarily concentrated in areas like Shiqu County, Chengdu City, and its neighboring districts and counties. Conversely, about 10% of the area experienced minor fluctuations, mainly in places such as Batang County, Derong County, Ganzi County, and Shiqu County. Regarding the temporal dimension, 84.76% of the vegetation area showed improvement, while 13% experienced degradation. The degradation was primarily observed in Chengdu City, Yibin City, and sporadically in the surrounding areas.
(2) Within the study area, the q-values of elevation, landform type, and soil type were observed to exceed 0.3, suggesting that they predominantly account for changes in vegetation cover. Conversely, the explanatory power of slope and slope direction was insignificant, with q-values less than 0.05, indicating that their influence on vegetation is minimal. Notably, the various factors displayed significant interactions, with q-values indicating interactions surpassing those associated with individual factors. For instance, the most influential interaction was noted between altitude ∩ vegetation type, yielding an impressive explanatory power of 0.540.
(3) The analysis revealed the existence of correlations between each factor and vegetation fractional cover (FVC) across Sichuan Province. Notably, distinct characteristics were observed in relation to the trend and mean of FVC changes and elevation, land use, population density, landform type, and soil type. Additionally, the correlation coefficients of both temperature and air temperature with vegetation FVC exhibited negative correlations, albeit displaying low spatial correlation. These factors were observed to significantly influence the ecological interaction cycle. Intriguingly, shifts in land use were found to be indicative of human activities, with rapid urban expansion increasingly encroaching upon areas previously occupied by vegetation cover, resulting in a corresponding decrease in FVC.
Publicly available datasets were analyzed in this study. This data can be found here: https://search.earthdata.nasa.gov/search.
LN: Conceptualization, Investigation, Writing–original draft, Writing–review and editing. WP: Conceptualization, Writing–review and editing. YY: Conceptualization, Data curation, Investigation, Writing–review and editing. JX: Formal Analysis, Methodology, Supervision, Writing–review and editing. YW: Writing–review and editing.
The author(s) declare that no financial support was received for the research, authorship, and/or publication of this article.
The authors declare that the research was conducted in the absence of any commercial or financial relationships that could be construed as a potential conflict of interest.
All claims expressed in this article are solely those of the authors and do not necessarily represent those of their affiliated organizations, or those of the publisher, the editors and the reviewers. Any product that may be evaluated in this article, or claim that may be made by its manufacturer, is not guaranteed or endorsed by the publisher.
Al-bukhari, A., Hallett, S., and Brewer, T. (2018). A review of potential methods for monitoring rangeland degradation in Libya. Pastoralism 8, 13. doi:10.1186/s13570-018-0118-4
Carla, L. F. D. S., Demetrius, D. D. S., Michel, C. M., Jackson, M. R., Igor, S. D. S. R., Rafael, P. C. L., et al. (2023). Trend analysis and identification of possible periods of change in the occurrence of extreme streamflow events in a tropical basin. J. S. Am. Earth Sci. 128, 2023. 104485. doi:10.1016/j.jsames.2023.104485
De, Jong, R., De, Bruin, S., De, Wit, A., Schaepman, M. E., and Dent, D. L. (2011). Analysis of monotonic greening and browning trends from global NDVI time-series. Remote Sens. Environ. 115 (2), 692–702. doi:10.1016/j.rse.2010.10.011
Deng, X. Y., Liu, Y., Liu, Z. F., and Yao, J. Q. (2017). Spatial and temporal dynamics of evapotranspiration in the arid zone of northwest China. Acta Ecol. Sin. 37 (9), 2994–3008. doi:10.5846/stxb201601270190
Du, J. Q., Qing, F., Fang, S. F., Wu, J. H., He, P., and Quan, Z. J. (2019). Effects of rapid urbanization on vegetation cover in the metropolises of China over the last four decades. Ecol. Indic. 107, 105458. doi:10.1016/j.ecolind.2019.105458
Du, J. Q., Shu, J. M., Yin, J. Q., Yuan, X. J., Ahati, J. H., Xiong, S. S., et al. (2015). Analysis on spatio-temporal trends and drivers in vegetation growth during recent decades in Xinjiang, China. Int. J. Appl. Earth Obs. Geoinf. 38, 216–228. doi:10.1016/j.jag.2015.01.006
Fang, W., Huang, S. Z., Huang, Q., Huang, G. H., Meng, E., and Luan, J. K. (2018). Reference evapotranspiration forecasting based on local meteorological and global climate information screened by partial mutual information. J. Hydrol. 561, 764–779. doi:10.1016/j.jhydrol.2018.04.038
Gocic, M., and Trajkovic, S. (2013). Analysis of changes in meteorological variables using Mann-Kendall and Sen's slope estimator statistical tests in Serbia. Glob. PlanetaryChange 100, 172–182. doi:10.1016/j.gloplacha.2012.10.014
Guo, F. Z., and Rasmussen., B. (2022). Predictive maintenance for residential air conditioning systems with smart thermostat data using modified Mann-Kendall tests. Appl. Therm. Eng. 222, 119955. 119955. doi:10.1016/j.applthermaleng.2022.119955
He, X., Zhang, F., Cai, Y., Tan, M. L., and Chan, N. W. (2023). Spatio-temporal changes in fractional vegetation cover and the driving forces during 2001-2020 in the northern slopes of the Tianshan Mountains, China. Environ. Sci. Pollut. Res. Int. 30 (30), 75511–75531. doi:10.1007/s11356-023-27702-x
He, Y. H. Z., Wang, L., Niu, Z., and Nath, B. W. (2022). Vegetation recovery and recent degradation in different karst landforms of southwest China over the past two decades using GEE satellite archives. Ecol. Inf. 68, 101555. doi:10.1016/j.ecoinf.2022.101555
Hirsch, R. M., and Slack, J. R. (1984). A nonparametric trend test for seasonal data withserial dependence. Water Resour. Res. 20 (6), 727–732. doi:10.1029/wr020i006p00727
Hu, X. L., Zhang, S. Y., Zhang, J., and Aamir, S. (2023). Efficient CUSUM control charts for monitoring the multivariate coefficient of variation. Comput. Industrial Eng. 179, 109159. 109159. doi:10.1016/j.cie.2023.109159
Huang, C. L., Yang, Q. K., Guo, Y. H., Zhang, Y. Q., and Guo, L. N. (2020). The pattern, change and driven factors of vegetation cover in the Qin Mountains region. Sci. Rep. 10, 20591. doi:10.1038/s41598-020-75845-5
Huang, S., Yu, L., Cai, D., hu, J., Liu, Z., Zhang, Z., et al. (2023). Predictors of surgery choices in women with early-stage breast cancer in China: a retrospective study. Ecol. Indic. 23, 23–28. 16718. doi:10.1186/s12885-023-10510-4
Huo, H., and Sun, C. P. (2021). Spatiotemporal variation and influencing factors of vegetation dynamics based on Geodetector: a case study of the northwestern Yunnan Plateau, China. Ecol. Indic. 130, 108005. doi:10.1016/j.ecolind.2021.108005
Jalonen, J., Järvelä, J., Koivusalo, H., and Hyyppä, H. (2014). Deriving floodplain topography and vegetation characteristics for hydraulic engineering applications by means of terrestrial laser scanning. J. Hydraulic Eng. 140, 04014056. doi:10.1061/(ASCE)HY.1943-7900.0000928
Jiang, H., Xu, X., Zhang, T., Xia, H., Huang, Y., and Qiao, S. (2022). The relative roles of climate variation and human activities in vegetation dynamics in coastal China from 2000 to 2019. Remote Sens.-Basel. 14, 2485. doi:10.3390/rs14102485
Jiang, H. P., Sun, Z. C., Guo, H. D., Weng, Q. H., Du, W. J., Xing, Q., et al. (2021). An assessment of urbanization sustainability in China between 1990 and 2015 using land use efficiency indicators. npj Urban Sustain 1, 34. doi:10.1038/s42949-021-00032-y
Kalisa, W., Igbawua, T., Henchiri, M., Ail, S., Zhang, S., Bai, Y., et al. (2019). Assessment of climate impact on vegetation dynamics over East Africa from 1982 to 2015. Sci. Rep. 9, 16865. doi:10.1038/s41598-019-53150-0
Karen, R., Ryberg, , Glenn, A., Hodgkins, , Robert, W., and Dudley, (2019). Change points in annual peak streamflows: method comparisons and historical change points in the United States. J. Hydrology 583, 124307. 124307. doi:10.1016/j.jhydrol.2019.124307
Kinjal, C., and Ankit, T. (2023). Neural network systems with an integrated coefficient of variation-based feature selection for stock price and trend prediction. Expert Syst. Appl. 219, 2023. 119527. doi:10.1016/j.eswa.2023.119527
Kong, D. X., Miao, C. Y., Borthwick, A. G. L., Lei, X. H., and Li, H. (2018). Spatiotemporal variations in vegetation cover on the Loess Plateau, China, between 1982 and 2013: possible causes and potential impacts. Environ. Sci. Pollut. Res. 25, 13633–13644. doi:10.1007/s11356-018-1480-x
Kotharkar, R., and Surawar, M. (2016). Land use, land cover, and population density impact on the formation of canopy urban heat islands through traverse survey in the nagpur urban area, India. J. Urban Plan. Development-asce 142, 04015003. doi:10.1061/(ASCE)UP.1943-5444.0000277
Kumar, L., and Mutanga, O. (2018). Google earth engine applications since inception: usage, trends, and potential. Remote Sens. 10, 1509–1515. doi:10.3390/rs10101509
Li, A., Wu, J., and Huang, J. (2012). Distinguishing between human-induced and climate-driven vegetation changes: a critical application of RESTREND in inner Mongolia. Landsc. Ecol. 27, 969–982. doi:10.1007/s10980-012-9751-2
Li, H. H., and Song, W. (2022). Spatial transformation of changes in global cultivated land. Sci. Total Environ. 859, 160194. 160194. doi:10.1016/j.scitotenv.2022.160194
Li, J. K., Yang, Y. T., Zhang, H. R., Huang, A. W., and Gao, Y. M. (2019). Spatial and temporal evolution characteristics of vegetation NPP and analysis of natural and anthropogenic factors in the Qinba Mountains in the past 15 years. Acta Ecol. Sin. 39 (22), 8504–8515. doi:10.5846/stxb201807231575
Li, X., Hai, Q., Zhu, Z., Zhang, D., Shao, Y., Zhao, Y., et al. (2023). Spatial and temporal changes in vegetation cover in the three north protection forest project area supported by GEE cloud platform. Forests 14, 295. doi:10.3390/f14020295
Li, Y. L., Wang, X. Q., Chen, Y. Z., and Wang, M. M. (2020). Land surface temperature variations and their relationship to fractional vegetation coverage in subtropical regions: a case study in Fujian Province, China. Int. J. Remote Sens. 41 (6), 2081–2097. doi:10.1080/01431161.2019.1685714
Liu, H., Jiao, F. S., Yin, J. Q., Li, T. Y., Gong, H. B., Wang, Z. Y., et al. (2020). Nonlinear relationship of vegetation greening with nature and human factors and its forecast-a case study of Southwest China. Ecol. Indic. 111, 106009. doi:10.1016/j.ecolind.2019.106009
Ma, B. X., He, C. X., Jing, J. L., Wang, Y. F., Liu, B., and He, H. C. (2023). Attribution of vegetation dynamics in Southwest China from 1982 to 2019. Acta Geogr. Sin. 78 (3), 714–728. doi:10.11821/dlxb202303013
Mutanga, O., and Kumar, L. (2019). Google earth engine applications since inception: usage, trends, and potential. Remote Sens. 11, 1509–1514. doi:10.3390/rs10101509
Pang, G. J., Wang, X. J., and Yang, M. X. (2017). Using the NDVI to identify variations in, and responses of, vegetation to climate change on the Tibetan Plateau from 1982 to 2012. Quatern. Int. 444, 87–96. doi:10.1016/j.quaint.2016.08.038
Peng, W. F., Zhang, D. M., Luo, Y. M., Tao, S., and Xu, X. L. (2019). Geographical detection of natural factors on NDVI changes of vegetation in Sichuan. Acta Geogr. Sin. 74 (9), 1758–1776. doi:10.11821/dlxb201909005
Piao, S., Fang, J. Y., Zhao, L. M., Guo, Q. H., Henderson, M., Ji, W., et al. (2003). Interannual variations of monthly and seasonal normalized difference vegetation index (NDVI) in China from 1982 to 1999. J. Geophys. Res. 108, 1–13. doi:10.1029/2002JD002848
Radu, G. P., Cristian, V. P., Bogdan, R., Dragoș, A., Mirea, , Vasile, D., et al. (2022). Insights into the Phaeozems pedogenesis using total elemental composition analysis. A case study north-eastern Romania, Geoderma 409, 115604. doi:10.1016/j.geoderma.2021.115604
Song, Y. Z., Wang, J. F., Ge, Y., and Xu, C. D. (2020). An optimal parameters-based geographical detector model enhances geographic characteristics of explanatory variables for spatial heterogeneity analysis: cases with different types of spatial data. GIS Sci. Remote Sens. 57 (5), 593–610. doi:10.1080/15481603.2020.1760434
Wang, H. X., and Liu, C. M. (2000). The meaning of crop water use efficiency and progress of research. Adv. Water Sci. 11 (1), 99–104.
Wang, J. B., Zhao, J., Li, C. H., Zhu, Y., Kang, C. Y., and Gao, C. (2019). Spatial and temporal patterns of anthropogenic impacts on vegetation cover in China from 2001-2015. Acta Geogr. Sin. 74 (3), 504–519. doi:10.11821/dlxb201903008
Wang, J. F., and Xu, C. D. (2017). Instrumental networking and social network building: how horizontal networking and upward networking create social capital. Acta Geogr. Sin. 72, 116–134. doi:10.3724/sp.j.1041.2017.00116
Wang, J. F., Zhang, T. L., and Fu, B. J. (2016). A measure of spatial stratified heterogeneity. Ecol. Indic. 67, 250–256. doi:10.1016/j.ecolind.2016.02.052
Wang, J., Li, X., Christakos, G., Liao, Y., Zhang, T., Gu, X., et al. (2010). Geographical detectors-based health risk assessment and its application in the neural tube defects study of the heshun region, China. Int. J. Geogr. Inf. Sci. 24 (1), 107–127. doi:10.1080/13658810802443457
Wu, Y. D., Ma, Y., Wu, H. R., Xiao, Y., and Li, H. (2020). Spatial and temporal evolution characteristics and driving forces of vegetation index in Sichuan Province based on MODIS-EVI index. Soil Water Conservation Res. 27 (05), 230–236+243+2. doi:10.13869/j.cnki.rswc.2020.05.031
Xiang, J. Y., Peng, W. F., Tao, S., Yin, Y., and Liu, H. S. (2023). Effectiveness and influencing factors of vegetation restoration in Sichuan Province, 2000-2019. Acta Ecol. Sin. 43 (04), 1596–1609. doi:10.5846/stxb202106071505
Xie, H., Tong, X. J., Li, J., Zhang, J. R., Liu, P. R., and Yu, P. Y. (2022). Changes of NDVl and EVI and their responses to climatic variables in the Yellow River Basin during the growing season of 2000 2018. Acla Ecol. Sin. 42 (11). doi:10.5846/stxb202104271108
Xu, Q. L., Li, W. H., Liu, J., and Wang, X. (2023). A geographical similarity-based sampling method of non-fire point data for spatial prediction of forest fires. For. Ecosyst. 10, 100104. doi:10.1016/j.fecs.2023.100104
Xu, S. Q., Yu, Z. B., Yang, C. G., Ji, X. B., and Zhang, K. (2018). Trends in evapotranspiration and their responses to climate change and vegetation greening over the upper reaches of the Y ellow River Basin. Agric. For. Meteorol. 263, 118–129. doi:10.1016/j.agrformet.2018.08.010
Xu, Y., Huang, W. T., Dou, S. Q., Guo, Z. D., Li, X. Y., Zheng, Z. M., et al. (2022). Response of vegetation NDVI to climate change and human activities in Southwest China from 2000 to 2020. Environ. Sci. 43 (06), 3230–3240. doi:10.13227/j.hjkx.202108107
Yuan, L. H., Jiang, W. G., Shen, W. M., Liu, Y. H., Wang, W. J., Tao, L. L., et al. (2013). Spatial and temporal variation of vegetation cover in the Yellow River Basin from 2000 to 2010. Acta Ecol. Sin. 33 (24), 7798–7806. doi:10.5846/stxb201305281212
Yuan, L., Cao, J., Wang, D., Yu, D., Liu, G., and Qian, Z. C. (2023). Regional disparities and influencing factors of high quality medical resources distribution in China. Int. J. Equity Health 22, 8. doi:10.1186/s12939-023-01825-6
Yue, S., Pilon, P., and Cavadias, G. (2002). Power of the Mann-Kendall and Spearman’s rho tests for detecting monotonic trends in hydrological series. J. Hydrology 259 (1/4), 254–271. doi:10.1016/S0022-1694(01)00594-7
Zhang, K., Lü, Y., Fu, B., and Li, T. (2018). The effects of restoration on vegetation trends: spatiotemporal variability and influencing factors. Earth Env. Sci. T R. SO 109, 473–481. doi:10.1017/S1755691018000518
Zhang, K., Lv, Y. H., Fu, B. J., Yin, L. X., and Yu, D. D. (2020). Impacts of vegetation cover changes on ecosystem services and their thresholds in the Loess Plateau. Acta Geogr. Sin. 75 (5), 949–960. doi:10.11821/dlxb202005005
Zhang, L., Karthikeyan, R., Bai, Z. K., and Srinivasan, R. (2017). Analysis of streamflow responses to climate variability and land use change in the Loess Plateau region of China. CATENA 154, 1–11. doi:10.1016/j.catena.2017.02.012
Zhang, Y. X., Li, X. B., and Chen, Y. H. (2003). A review of multi-scale remote sensing and field measurement methods for grassland vegetation cover. Adv. Earth Sci. 18 (1), 1673–4831. doi:10.3321/j.issn:1001-8166.2003.01.012
Zhao, A. Z., Zhang, A. B., Liu, J. H., Feng, L. L., and Zhao, Y. L. (2019). Assessing the effects of drought and “Grain for Green” program on vegetation dynamics in China’s Loess Plateau from 2000 to 2014. CATENA 175, 446–455. doi:10.1016/j.catena.2019.01.013
Zhao, J. Y., Li, J. J., Zuo, L. L., Liu, G. H., and Su, X. K. (2023). Interaction dynamics of multiple ecosystem services and abrupt changes of landscape patterns linked with watershed ecosystem regime shifts. Ecol. Indic. 150, 110263. 110263. doi:10.1016/j.ecolind.2023.110263
Zhao, L., Dai, A., and Dong, B. (2018). Changes in global vegetation activity and its driving factors during 1982–2013. Agric. For. Meteorol. 249, 198–209. doi:10.1016/j.agrformet.2017.11.013
Zhao, Y., Feng, Q., and Lu, A. (2021). Spatiotemporal variation in vegetation coverage and its driving factors in the Guanzhong Basin, NW China. Ecol. Inf. 64, 101371. doi:10.1016/j.ecoinf.2021.101371
Keywords: GeoDetectors, human activities, sichuan province, spatial and temporal dynamics, vegetation cover
Citation: Ning L, Peng W, Yu Y, Xiang J and Wang Y (2023) Quantifying vegetation change and driving mechanism analysis in Sichuan from 2000 to 2020. Front. Environ. Sci. 11:1261295. doi: 10.3389/fenvs.2023.1261295
Received: 19 July 2023; Accepted: 06 October 2023;
Published: 17 October 2023.
Edited by:
Hualin Xie, Jiangxi University of Finance and Economics, ChinaReviewed by:
Bruna Almeida, NOVA University of Lisbon, PortugalCopyright © 2023 Ning, Peng, Yu, Xiang and Wang. This is an open-access article distributed under the terms of the Creative Commons Attribution License (CC BY). The use, distribution or reproduction in other forums is permitted, provided the original author(s) and the copyright owner(s) are credited and that the original publication in this journal is cited, in accordance with accepted academic practice. No use, distribution or reproduction is permitted which does not comply with these terms.
*Correspondence: Wenfu Peng, cHdmemhAMTI2LmNvbQ==
Disclaimer: All claims expressed in this article are solely those of the authors and do not necessarily represent those of their affiliated organizations, or those of the publisher, the editors and the reviewers. Any product that may be evaluated in this article or claim that may be made by its manufacturer is not guaranteed or endorsed by the publisher.
Research integrity at Frontiers
Learn more about the work of our research integrity team to safeguard the quality of each article we publish.