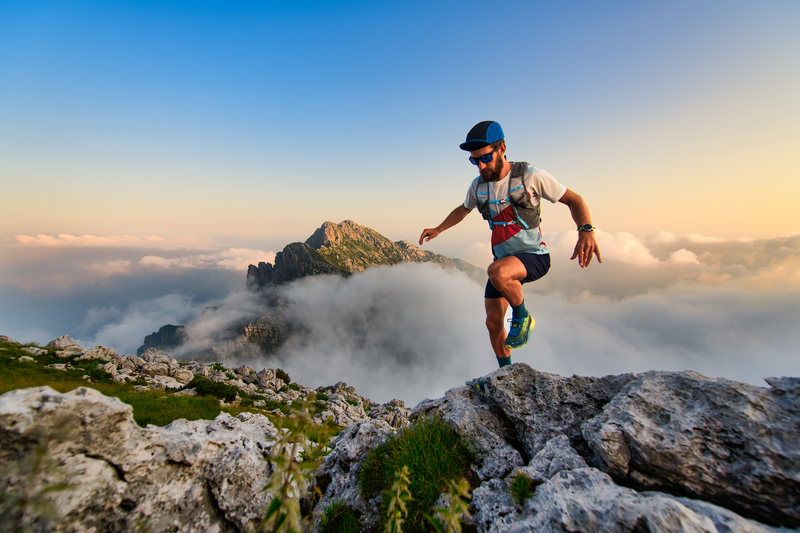
94% of researchers rate our articles as excellent or good
Learn more about the work of our research integrity team to safeguard the quality of each article we publish.
Find out more
EDITORIAL article
Front. Environ. Sci. , 28 June 2023
Sec. Environmental Economics and Management
Volume 11 - 2023 | https://doi.org/10.3389/fenvs.2023.1235845
This article is part of the Research Topic Fuzzy Decisions and Machine Learning Methods in Climate Change View all 5 articles
Editorial on the Research Topic
Fuzzy decisions and machine learning methods in climate change
Different factors can affect Fuzzy decisions and machine learning methods in climate change. Energy efficiency ensures that energy resources are used more effectively, which means energy savings. Less energy consumption reduces energy costs and ensures that energy sources can be used for a longer period. System quality is very important for ensuring Fuzzy decisions and machine learning methods in climate change. Accurate and reliable data is needed for Fuzzy decisions and machine learning methods in climate change. Energy consumption, energy costs and other performance indicators must be accurately measured and recorded. Quality systems reliably perform data collection, automation, and measurement, ensuring the precision and accuracy of data. To ensure Fuzzy Decisions and Machine Learning Methods in Climate Change, effective legal regulations should also be provided. Energy performance regulations help set energy efficiency standards and targets. These standards and targets encourage government and organizations to achieve a certain level of energy efficiency.
There are many variables that have an important role on the effectiveness of Fuzzy decisions and machine learning methods in climate change. In this context, to improve this performance, businesses need to make the necessary improvements for the development of these factors. However, these improvements also lead to an increase in the costs of the enterprises. In other words, if businesses carry out these improvement practices unplanned, this causes the costs to reach an uncontrollable level. Therefore, among these actions, the more important ones need to be determined. In this way, businesses will be able to use their limited budgets for more priority issues. This will also help increase productivity so that Fuzzy decisions and machine learning methods in climate change should be improved without having high amount costs. Additionally, energy efficiency can be improved by comparing the practices of countries that are successful in Fuzzy decisions and machine learning methods in climate change and Energy Efficiency. Fuzzy decisions and machine learning methods in climate change are especially suitable and important for developing countries as found in previous research (Candila et al, 2021; Saqib et al, 2021; Bhuiyan et al, 2022; Dinçer et al, 2022; Li et al, 2022).
The population growth rate in developing countries is quite high. In addition, these countries are acting to increase their economic growth. These issues cause the demand for energy to increase rapidly. Therefore, the high energy performance of countries will contribute to the reduction of energy consumption in developing countries. Thus, a radical increase in energy demand will be prevented. Consequently, extraordinary increases in energy demand can be mitigated by managing the energy performance. So, climate change is more important for developing countries than for other countries. In this context, developing countries are analyzed in this study. China has taken more accurate steps to manage the energy performance. Hence, it will also increase the economic performance of successful countries. These countries will be able to manage their energy demands and avoid extra costs.
Soam et al. proposed novel AHP Analyser. They used new decision-making tool for prioritizing climate change mitigation options and forest management.
Tryndina et al. analyzed the renewable energy incentives on the road to sustainable development during climate change. Then, developing countries aim to grow economically they can achieve their goals by implementing the energy strategies.
Barykin et al. investigated the relationship between Innovative analysis and climate change. It is evidence from developed European countries. In the study, which concluded that renewable energy reduces the ecological footprint, it was also stated that the energy consumption of developing countries is high.
Nyangarika et al. examined the energy stability and decarbonization in developing countries: Random Forest approach for forecasting of crude oil trade flows and macro indicators.
All authors listed have made a substantial, direct, and intellectual contribution to the work and approved it for publication.
The authors declare that the research was conducted in the absence of any commercial or financial relationships that could be construed as a potential conflict of interest.
All claims expressed in this article are solely those of the authors and do not necessarily represent those of their affiliated organizations, or those of the publisher, the editors and the reviewers. Any product that may be evaluated in this article, or claim that may be made by its manufacturer, is not guaranteed or endorsed by the publisher.
Bhuiyan, M. A., Zhang, Q., Khare, V., Mikhaylov, A., Pinter, G., and Huang, X. (2022). Renewable energy consumption and economic growth nexus—a systematic literature review. Front. Environ. Sci. 10, 878394. doi:10.3389/fenvs.2022.878394
Candila, V., Maximov, D., Mikhaylov, A., Moiseev, N., Senjyu, T., and Tryndina, N. (2021). On the relationship between oil and exchange rates of oil-exporting and oil-importing countries: From the great recession period to the COVID-19 era. Energies 14, 8046. doi:10.3390/en14238046
Dinçer, H., Yüksel, S., Mikhaylov, A., Barykin, S. E., Aksoy, T., and Hacioğlu, U. (2022). Analysis of environmental priorities for green project investments using an integrated q-rung orthopair Fuzzy modeling. IEEE Access 10, 50996–51007. doi:10.1109/ACCESS.2022.3174058
Li, J., Dinçer, H., Yüksel, S., Mikhaylov, A., and Barykin, S. E. (2022). Bipolar q-ROF hybrid decision making model with golden cut for analyzing the levelized cost of renewable energy alternatives. IEEE Access 10, 42507–42517. doi:10.1109/ACCESS.2022.3168315
Keywords: renewable energy, economic growth, emission, emerging economies, model
Citation: Yüksel S, Dinçer H and Mikhaylov A (2023) Editorial: Fuzzy decisions and machine learning methods in climate change. Front. Environ. Sci. 11:1235845. doi: 10.3389/fenvs.2023.1235845
Received: 06 June 2023; Accepted: 23 June 2023;
Published: 28 June 2023.
Edited by:
Xiaolei Sun, Chinese Academy of Sciences (CAS), ChinaReviewed by:
Yasir Ahmed Solangi, Jiangsu University, ChinaCopyright © 2023 Yüksel, Dinçer and Mikhaylov. This is an open-access article distributed under the terms of the Creative Commons Attribution License (CC BY). The use, distribution or reproduction in other forums is permitted, provided the original author(s) and the copyright owner(s) are credited and that the original publication in this journal is cited, in accordance with accepted academic practice. No use, distribution or reproduction is permitted which does not comply with these terms.
*Correspondence: Alexey Mikhaylov, YWxleGV5ZmFAeWEucnU=
Disclaimer: All claims expressed in this article are solely those of the authors and do not necessarily represent those of their affiliated organizations, or those of the publisher, the editors and the reviewers. Any product that may be evaluated in this article or claim that may be made by its manufacturer is not guaranteed or endorsed by the publisher.
Research integrity at Frontiers
Learn more about the work of our research integrity team to safeguard the quality of each article we publish.