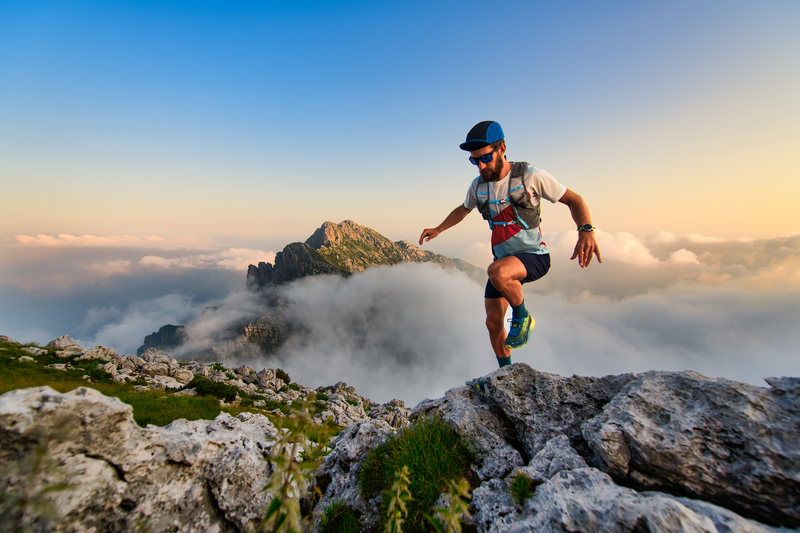
95% of researchers rate our articles as excellent or good
Learn more about the work of our research integrity team to safeguard the quality of each article we publish.
Find out more
ORIGINAL RESEARCH article
Front. Environ. Sci. , 03 August 2023
Sec. Environmental Economics and Management
Volume 11 - 2023 | https://doi.org/10.3389/fenvs.2023.1208497
This article is part of the Research Topic Fuzzy Mathematical Model and Optimization in Digital Green Innovation for Industry 5.0 View all 14 articles
As there are a large number of risk factors affecting green innovation under the global value chain, the screening of risk indicators is a key link in developing green innovation activities. Through the analysis of the influencing factors of current green innovation risk, the primary index of green innovation risk is established. The group decision characteristic root method is used to identify the key importance of primary indicators of green innovation risk and construct the global value chain’s green innovation risk index system, which provides a scientific method and new ideas for measuring and evaluating green innovation risk in the global value chain. The empirical results of this paper are as follows: 1) green R&D risk measurement indicators under the global value chain include the proportion of global green R&D personnel investment, the proportion of global green R&D capital investment, the stability of global green R&D in manufacturing, the ease of international transfer of green technology, and the international protection of green technology patents; 2) Green manufacturing risk measurement indicators under the global value chain have seven risk factors: the proportion of global green manufacturing personnel investment, the proportion of global green manufacturing capital investment, the global green manufacturing product production scale, the global green manufacturing product quality performance level, the global outsourcing scale of green products, the global green manufacturing technology transformation degree of manufacturing industry, and the global green manufacturing cost increase; 3) green marketing risk measurement indicators under the global value chain have seven risk factors: the proportion of global green marketing personnel investment, the proportion of global green marketing capital investment, the international demand level for green products, the international market competition intensity of green products, the possession of green products to existing international marketing channels, the commercialization and internationalization level of green technology, and the intensity of green technical barriers to trade in the international community; and 4) green service risk measurement indicators under the global value chain have five risk factors: the proportion of global green service personnel investment, the proportion of global green service capital investment, the 1coverage of global green service outlets, the globalization level of global green product supply chain, and the global after-sales technical service capability of green products.
Since the 1980s, the global economy has witnessed rapid advancements in production and the globalization of trade. This phenomenon of globalization has emerged as a significant characteristic of the modern economy, facilitating the accelerated international movement of production factors such as capital, goods, and technology. As a result, it effectively stimulates the optimal allocation of resources on a global scale., but also changes original production, consumption, and management methods and creates new market opportunities. The realization of globalization is governed and organized through global value chains (Sun Y. Y. et al, 2020). Within the global value chain’s division of labor system, China’s manufacturing industry currently finds itself at nearly the bottom of the global value chain, facing the unfavorable situation of being “low-end locked” (Sun Y. Y. et al, 2020). In order for China‘s manufacturing industry to break out of the low-end lock of the global value chain, the country needs to actively engage in the international division of labor by integrating itself into the global value chain, improve its own innovation ability and green market entry ability through green innovation, transition to the high value-added link in the global value chain, and complete the value promotion in the global value chain to enhance its competitiveness in the international market. The word “innovation” itself is related to risk. While green innovation in manufacturing has the potential to create economic, social, and ecological value (Dong et al, 2023a), it is important to recognize that it is also a high-risk endeavor. All technological innovation activities accompanied by risks (PIETROBELLI and RABELLOTTI, 2011). A series of current uncertain risk factors have become the biggest obstacles to improving green innovation in the manufacturing industry. The value of green innovation must be created and protected through risk management (Luo and Jia, 2023). At the same time, green innovation ability also directly determines the international market competitiveness of the company itself. Only with strong green R&D ability can a company develop and produce green products that meet the needs of the international market, to continuously improve the international competitiveness of the company and remain invincible in the face of international competition (Wang et al, 2022). Therefore, measuring and identifying the level of green innovation risk is essential for improving companies’ ability to develop green innovation, enhance market competitiveness, and achieve sustainable development. Correctly identifying and analyzing green innovation risk is key to the success of innovation activities (Xiao et al, 2022).
Global value chain is made up of four important links: R&D, production, marketing, and service (Zhang et al, 2020). However, not all links create the same amount of value in the global value chain’s value creation process. As the famous “smile curve” shows, the value created by R&D, marketing and service links is higher, while the value created by manufacturing links is lower (Wu and Fan, 2021). From the perspective of current development of the world economy, the global value chain not only brings opportunities for products to enter the international market (Ye et al, 2021), it also plays a crucial role in integrating green resources throughout various stages of the global value chain. This integration enhances the green innovation capabilities of the manufacturing industry and contributes to the advancement of green industries (Sui et al, 2015). Therefore, taking into account the influence of the global value chain on the green innovation process, this study divides the green innovation process of the manufacturing industry within the global value chain into four main stages: global green innovation R&D, global green innovation manufacturing, global green innovation marketing, and global green innovation service. The risks faced by green innovation in the global value chain of the manufacturing industry are the products of the combined effects of global value chain, green economic development needs, and innovation risks (Ren et al, 2021). Based on the four important stages of R&D, the linear process of R&D, manufacturing, The process of green innovation in the manufacturing industry within the global value chain encompasses various stages such as manufacturing, marketing and service of green innovation in the manufacturing industry includes the risk factors of green innovation in manufacturing industry under global value chain include global green innovation R&D risk, global green innovation manufacturing risk, global green innovation marketing risk, and global green innovation service risk (Ye, 2021), as shown in Figure 1.
FIGURE 1. Green innovation risk classification of the manufacturing industry under the global value chain.
The evaluation index design of intellectual property rights of high-tech companies should follow the principles of being comprehensive, scientific, operable, and quantifiable. In this study, we adopted the grounded theory research method to identify the risks associated with green innovation in the manufacturing industry within the global value chain. Furthermore, we explored the factors that influence these risks, using them as the theoretical foundation for our analysis. Based on the existing research results, from the four dimensions of global green R&D risk, global green manufacturing risk, global green marketing risk, and global green service risk, the global value chain green innovation risk index system was initially constructed, including four secondary indicators and 31 tertiary indicators. See Table 1 for details.
Academic research on green innovation risk indicators under the global value chain is in its exploratory stage. The existing research mostly uses fuzzy analytic hierarchy process (AHP) and matter-element methods to comprehensively analyze relevant indicators and obtain evaluation results. Due to the large number of green innovation risk indicators, secondary indicators cannot be accurately discarded to retain effective information unless the weight of each indicator under the criterion layer can be accurately identified. In 1996, Professor Yuanhua Qiu proposed the group decision characteristic root method, which is a new characteristic root method that combines the experience and wisdom of scholars in related fields to judge and make decisions on multiple evaluated objects. It is a mathematical decision-making method, which is a derivative branch of the analytic hierarchy process proposed by T. L. Saaty, but the group decision eigenvalue method is superior to the analytic hierarchy process. It avoids the inconsistency of the objectives of the analytic hierarchy process judgment matrix of the (Meltzer, 2014). Therefore, this paper adopts the group decision feature root method (GEM) to solve this problem. Group Decision Eigenroot Method (GEM) is a new feature root method for expert group decision-making system (G) to judge and make decisions on multiple evaluation targets. Using GEM only requires experts to score each index, before transposing the score matrix into matrix F. In elaborating the shortcomings of the expert weighting method, the group decision feature root method can compensate for these shortcomings. In group decision-making, the most commonly used method is the comprehensive weighting method. The weight selected by this method has many shortcomings, however. These include complex operation, overly strong subjectivity, and insensitivity to results. The group decision eigenvalue method does a good job of solving these shortcomings. By using an ideal expert, that the issue of the expert‘s evaluation result of the evaluation object being untrue because of too strong subjectivity or insensitivity to the result is overcome (Quan, 2017). Compared with AHP, not only can GEM overcome the inconsistency of the judgment matrix, but it also needs no consideration of the weight of experts. Thus, the object of evaluation can be properly evaluated using a simple calculation. (Xiao et al, 2009).
The definition of ideal expert S*: The expert with the smallest angle between the score vector and the score vector of each expert in the group is called the ideal expert of the group. Ideal expert scoring vector X* is the vector satisfying the maximum value of the function
Finding the ideal expert S * is the first step in this method. In the decision-making system G (S1, S2, …, Sm), m experts and n evaluated objects are selected, that is (A1,A2,…,An). The score of the i’th expert Si to the j’th evaluated target Aj is recorded as x.
The decision-making level of scoring experts is affected by many factors, such as personal professional aspects and personal emotions. Professional influence includes knowledge, experience, and personal comprehensive ability. Aspects of personal emotions include personal preferences, physical status, mental status, and emotions at the time. Based on the above factors, there is no ideal expert decision in reality (Wang and Tan, 1999). However, in the study, we assume that the ideal expert exists and is S*, and thus score each index. The result is the result of the expert‘s score on the index, which is the estimated value of the evaluated object. The score vector of the ideal expert is x*=(x1*,x2*,…xn*)T∈En, The ideal expert has the smallest angle between the scoring vector of the evaluated object and the scoring vector of other experts. When making group decisions, people habitually find more authoritative experts to participate each time, so they think that ' ideal experts ' are experts with high consistency with the expert group‘s understanding of the evaluated object. The conclusion of the ideal expert on the evaluated object is completely consistent with the conclusion of the other experts of the expert group on the evaluated object, and the difference between the expert conclusions is the smallest. Following the above definition, x* is an n-ary column vector. It is obtained by formula
Where x* is the total score of the decision system G for the selected index.
Index solving theorem.
Theorem 1.
Theorem 2. Suppose A is an m×n matrix and B is an n×m matrix. Then AB and BA have the same (including multiplicity) non-zero eigenvalues.
Theorem 3.
This method only needs all the experts involved in the decision-making to directly score the green innovation risk indicators of each evaluated manufacturing industry, and the scoring matrix obtained is then transposed and multiplied by matrix F. Therefore, the optimal decision conclusion is the eigenvector corresponding to the maximum eigenvalue of F. The scoring result of the ideal expert to the evaluated object obtained by the group decision characteristic root is the ranking of multiple evaluated objects.
When the maximum eigenvalue of the judgment matrix is a single root, the eigenvector corresponding to the maximum eigenvalue is the optimal solution. If the maximum characteristic root of the judgment matrix is a multiple root, it is necessary to find the two maximum characteristic roots at the same time, calculate the corresponding feature vector and determine the corresponding manufacturing green innovation risk index, and then rank the two risk indicators. Other risk indicators are based on the feature vector of the manufacturing green innovation risk index corresponding to the second largest characteristic root or deleting the ranking score in the existing judgment matrix. The above steps should be repeated until the single root of the largest characteristic root is found.
Under the guidance of the principle of index selection, GEM is used to screen the importance of manufacturing green innovation risk measurement indicators under the global value chain. The index portion combines the overall risk of manufacturing green innovation and invites 15 experts to form an expert group. The 15 experts come from universities, government low-carbon management functional departments and Chinese manufacturing enterprise management departments, green product R&D departments, green manufacturing departments, green marketing departments, and green service departments. Qualitative evaluation is made by scoring the questionnaire (see Appendix A questionnaire). The design of the questionnaire is based on the Likert five-point scale, and the importance of the green innovation risk measurement index is divided into five levels. From 1 to 5, they represent “very unimportant,” “unimportant,” “important,” “very important,” and “most important” (see Table 2).
The scores of experts on green innovation risk indicators are obtained after collecting the questionnaires. The expert scores of green innovation risk indicators after statistics are shown in Table 3.
The expert scoring results of the above green innovation risk indicators are then processed as follows:
Using MATLAB software to calculate the maximum eigenvalue, the results show that the maximum eigenvalue is a single root. The eigenvalue of maximum
On the basis of summarizing previous studies, this study selected 0.027 as the standard value of index screening, that is, the index with the feature vector less than 0.027 after eliminating the standardized results, and the index with the feature vector greater than 0.027 after retaining the standardized results. According to the results in Table 4, the standardized results of the six risk factors of C17, C24, C28, C31, C39, and C310 are respectively 0.0269439, 0.0242547, 0.0260108, 0.0203634, 0.0226152, and 0.025095. All are less than the standard value of 0.027 for index screening so these indicators are removed. The values of the standardized results of the remaining indicators are greater than the standard value of 0.027, so these indicators are retained. After the above screening indicators, the retained indicators are C11, C12, C13, C14, C15, C16, C21, C22, C23, C25, C26, C27, C32, C33, C34, C35, C36, C37, C38, C41, C42, C43, C44, and C45.
These relate to the proportion of global green R&D personnel investment in the manufacturing industry, the proportion of global green R&D investment in the manufacturing industry, the stability of global green R&D in the manufacturing industry, the ease of international transfer of green technology in the manufacturing industry, the international protection of green technology patents in the manufacturing industry, the proportion of global green manufacturing personnel investment in the manufacturing industry, the proportion of global green manufacturing personnel investment in the manufacturing industry, the proportion of global green manufacturing capital investment in the manufacturing industry, the production scale of global green manufacturing products in the manufacturing industry, the quality and performance level of global green manufacturing products in the manufacturing industry, the global outsourcing scale of green products in the manufacturing industry, the technological transformation degree of global green manufacturing in the manufacturing industry, the increase of global green manufacturing costs in the manufacturing industry, the proportion of global green marketing personnel investment in the manufacturing industry, the proportion of global green marketing capital investment in the manufacturing industry, and the international demand level of green products.
Global green innovation R&D risk refers to the various risks that may occur during the green innovation research and development stage on a global scale. At this stage, these risks can include personnel-related risks, financial, technical, and policy risks (Wang and Wang, 2020). As a highly professional activity, the R&D process of green innovation requires personnel with extensive knowledge and experience, as well as sufficient funding to support the innovation process. Insufficient R&D personnel and capital investment can have a negative impact on the success rate of green innovation. Furthermore, R&D risk stems from the immaturity of green innovation technology. The uncertainty and substitutability of the innovation R&D life cycle will lead to irreparable loss of innovation benefits (Hou et al, 2019). The international transfer of green innovation introduces uncertainties that can hinder the progress of green innovation. The uncertainty arises from factors such as differences in technological capabilities, regulatory frameworks, and market conditions between countries. Additionally, the effectiveness of international intellectual property rights’ protection plays a pivotal role. If intellectual property rights are not adequately protected, innovations can be easily imitated by competitors, jeopardizing the benefits derived from the innovation and thereby increasing the risk associated with green innovation.
Global green innovation manufacturing risk primarily refers to the potential failure of innovation caused by uncertainties and changes within the system during the manufacturing process (Deng, 2017). To achieve mass production of green products, it is crucial to have an adequate number of skilled manufacturing personnel and sufficient funding (Lu, 2015). Green innovation in the manufacturing industry introduces new requirements for processes, equipment, and raw materials. Global outsourcing is an effective approach to address challenges such as limited production capabilities and low value added in the manufacturing sector. However, changes in the scale of global outsourcing can also introduce risks. Additionally, the competition for orders among enterprises in the international market can be influenced by the scale of subcontracting. At the same time, the government or international organization’s production constraints on companies will increase the cost burden in the production process.
In the global green innovation marketing stage, if there is no sufficient guarantee of marketing personnel and funds, the risk of the entire marketing activity will increase. Uncertainty in the international market also has a greater impact at this stage. If the international market changes the demand for manufacturing green products (processes, services), the degree of recognition is not high, the international market share of manufacturing green products (processes, services) is too small, the international market competition is fierce, and it is difficult to use existing international marketing channels (Dong et al, 2023b). Therefore, a high degree of possession of existing marketing channels is an important way to achieve success in new product marketing. In addition, the globalization of the green supply chain in manufacturing and the presence of green technical barriers to trade in developed countries also influence green marketing activities. (Mao and Huo, 2002).
Human capital and capital investment are essential resources for service activities in manufacturing enterprises, and they have a direct impact on the efficiency and effectiveness of innovative product services (Xiao et al, 2014). The coverage of a service network is a critical factor in balancing the service capabilities of the manufacturing industry. Establishing a comprehensive service network can enhance service efficiency, reduce service costs, and support the successful promotion of product innovation activities (Jie and Zhu, 2021). Simultaneously, the adoption of “green supply chain management” by large multinational enterprises can pose challenges for Chinese enterprises and become a new threshold for their development. After-sales technical service, also known as “after-sales technical support,” plays a crucial role in enhancing customer satisfaction and loyalty. It involves providing assistance, guidance, and troubleshooting for customers using the manufactured products. Overall, the availability of skilled human resources, sufficient capital investment, a well-established service network, and effective after-sales technical service are all significant factors in ensuring the success of innovative product services in the manufacturing sector. involves installations and configuarations, use of instructions, and troubleshooting for products (processes, services) sold, as well as serving as a platform for information queries and customer information acquisition, consulting and technical training (Jiang, 2013). Helping to win high customer satisfaction plays a huge role in improving the market share of products (processes, services).
On the basis of referring to the existing relevant research at home and abroad, considering the particularity of the green innovation process in the manufacturing industry, following the scientific, effective, comparable, and operable principles of index selection, through the previous global value chain manufacturing green innovation risk identification, risk factor analysis, and 15 experts ' scoring of risk factor indicators, the group decision eigenvalue method is used to screen out the indicators with a score lower than 0.027. A total of 25 risk factors that have a significant impact on green innovation activities of the global value chain manufacturing industry and that can lead to risk consequences were identified. The risk indicators after screening are shown in Table 5.
The risk measurement index of green R&D under the global value chain encompasses six key risk factors. The first is proportion of global green R&D personnel investment. This factor refers to the allocation of human resources towards green research and development activities on a global scale. The higher the proportion of investment in skilled personnel focused on green R&D initiatives, the lower the associated risks. The second, proportion of global green R&D capital investment, pertains to the allocation of financial resources towards global green R&D efforts. A higher proportion of capital investment in green R&D indicates a lower level of risk. Stability of global green R&D in the manufacturing industry, the third factor, evaluates the stability and consistency of green R&D efforts within the manufacturing industry on a global scale. A stable and consistent approach to green R&D lowers the associated risks. Fourth is ease of international transfer of green technology, which considers the ease with which green technology can be transferred internationally. Effortless international transfer of green technology reduces the risks involved in green R&D activities. Next, international protection of green technology patents focuses on the level of international protection available for green technology patents. Strong protection measures minimize the risks associated with intellectual property theft and infringement. These risk factors collectively contribute to measuring the potential risks involved in green R&D activities under the global value chain. By assessing and managing these risks effectively, companies can enhance their green innovation capabilities and navigate the challenges associated with global green R&D initiatives.
The risk measurement index of green manufacturing under the global value chain includes seven risk factors: the proportion of global green manufacturing personnel input, the proportion of global green manufacturing capital investment, the scale of global green manufacturing products, the quality and performance level of global green manufacturing products, the scale of global outsourcing of green products, the degree of technological transformation of global green manufacturing in manufacturing industry, and the increase in global green manufacturing costs.
The green marketing risk measurement index under the global value chain comprises seven significant risk factors. Proportion of global green marketing personnel investment refers to the allocation of human resources dedicated to green marketing activities on a global scale. A higher proportion of investment in skilled personnel for green marketing signifies a lower level of risk. The second factor, proportion of global green marketing capital investment, pertains to the allocation of financial resources towards global green marketing efforts. A higher proportion of capital investment in green marketing indicates a lower level of risk. Third, international demand level of green products, assesses the level of demand for green products in international markets. Higher demand for green products signifies lower marketing risks associated with their promotion and acceptance. The fourth factor, international market competition intensity of green products, evaluates the level of competition in international markets specifically for green products. Higher competition intensity may indicate higher marketing risks due to the need to differentiate and stand out in the market. The next factor, degree of green products’ integration into existing international marketing channels, considers the extent to which green products can be integrated into existing international marketing channels. Greater integration implies lower marketing risks, as established channels can facilitate product distribution and customer reach. The sixth factor, level of green technology commercialization and internationalization, focuses on the extent to which green technologies can be successfully commercialized and internationalized. Higher levels of commercialization and internationalization reduce marketing risks associated with new technology adoption and market penetration. Finally, intensity of green technical trade barriers in the international community assesses the presence and intensity of trade barriers specific to green products and technologies in the global market. Higher barriers pose greater marketing risks, such as restricted market access or added compliance requirements. By considering and managing these risk factors effectively, companies can better evaluate the risks associated with green marketing activities in the global value chain. This enables them to develop appropriate strategies to overcome challenges and leverage opportunities in promoting green products internationally.
The green service risk measurement index under the global value chain consists of five key risk factors. The first risk factor is proportion of global green service personnel investment and refers to the allocation of human resources towards green service activities on a global scale. A higher proportion of investment in skilled personnel dedicated to green services indicates a lower level of risk. The second factor, proportion of global green service capital investment, pertains to the allocation of financial resources towards global green service initiatives. A higher proportion of capital investment in green services suggests a lower level of risk. Next, coverage of global green service outlets assesses the geographic coverage and distribution of green service outlets worldwide. A wider coverage of outlets decreases the risk associated with fulfilling customer demands and providing timely services. The fourth factor, globalization level of the global green product supply chain, evaluates the extent to which the global green product supply chain is integrated and interconnected internationally. A higher level of globalization reduces risks related to supply chain disruptions, ensuring smooth delivery of green services. The final factor, global after-sales technical service capability of green products, focuses on the ability to provide after-sales technical services for green products on a global scale. A strong global after-sales technical service capability minimizes risks associated with product malfunctioning, customer dissatisfaction, and warranty claims. Stability of global green technology application in the manufacturing industry. These risk factors collectively form the basis of assessing the potential risks involved in green service activities under the global value chain. By understanding and managing these risks effectively, companies can enhance their green service capabilities, ensure customer satisfaction, and establish a strong presence in the global market.
Due to the particularity of green innovation, the problem of innovation risk is particularly important (Wang and Liu, 2020). The design of risk indicators and the identification of important indicators needs to be more rigorous. The use of group decision characteristic root method can solve the problem of screening multiple indicators and improve the scientific methodology, independence, and effectiveness of risk indicators. The results show that the selected indicators meet the research requirements and provide a scientific basis for the measurement and evaluation of green innovation risk under the global value chain. Four major findings emerged. First, the risk measurement index of green R&D under the global value chain was found to include six risk factors: the proportion of global green R&D personnel investment, the proportion of global green R&D capital investment, the stability of global green R&D in manufacturing industry, the ease of international transfer of green technology, and the international protection of green technology patents. Second, the green manufacturing risk measurement index under the global value chain was found to include seven risk factors: the proportion of global green manufacturing personnel investment, the proportion of global green manufacturing capital investment, the global green manufacturing product production scale, the global green manufacturing product quality performance level, the global outsourcing scale of green products, the global green manufacturing technology transformation degree of the manufacturing industry, and the global green manufacturing cost increase. Third, the green marketing risk measurement index under the global value chain was found to include seven risk factors: the proportion of global green marketing personnel investment, the proportion of global green marketing capital investment, the international demand level for green products, the international market competition intensity of green products, the degree of green products occupying the existing international marketing channels, the level of green technology commercialization and internationalization, and the intensity of green technical barriers to trade in the international community. Finally, the green service risk measurement index under the global value chain was found to include six risk factors: the proportion of global green service personnel investment, stability of global green technology application in the manufacturing industry, the proportion of global green service capital investment, the coverage of the global green service network, the globalization level of global green product supply chain, and the global after-sales technical service capability of green products.
This research has important theoretical value and practical significance. In the early stage, the grounded theory research method was used to identify the green innovation risk of manufacturing enterprises and screened out the extension of the risk influencing factors, as well as risk measurement indicators that could accurately, objectively, and truly reflect the actual situation of green innovation risk in the manufacturing industry under the global value chain, with as few indicators as possible. This research, which contains the most comprehensive content to date, provides a theoretical basis for the next step in green innovation risk measurement modeling in the manufacturing industry under the global value chain. The limitation of this study is that there is no empirical study of specific manufacturing enterprises, so the author’s next research content is to conduct empirical analysis of specific manufacturing enterprises to test the applicability of risk indicators.
The raw data supporting the conclusion of this article will be made available by the authors, without undue reservation.
Ethical review and approval was not required for the study on human participants in accordance with the local legislation and institutional requirements. Written informed consent for participation was not required for this study in accordance with the national legislation and the institutional requirements. Written informed consent was obtained from the individual(s) for the publication of any potentially identifiable images or data included in this article.
The author confirms being the sole contributor of this work and has approved it for publication.
2021 Research Start-up Fund of Suihua University (SQ21006); Key topics of the 14th Five-Year Plan for Education Science of Heilongjiang Province in 2022 (GJB1422388).
The author declares that the research was conducted in the absence of any commercial or financial relationships that could be construed as a potential conflict of interest.
All claims expressed in this article are solely those of the authors and do not necessarily represent those of their affiliated organizations, or those of the publisher, the editors and the reviewers. Any product that may be evaluated in this article, or claim that may be made by its manufacturer, is not guaranteed or endorsed by the publisher.
Deng, T. (2017). Japan 's fiscal policy of developing low-carbon economy and its application to the green manufacturing system in Hunan Province. Industry Technol. Forum 16 (08), 118–119.
Dong, T., Yin, S., and Zhang, N. (2023b). New energy-driven construction industry: Digital green innovation investment project selection of photovoltaic building materials enterprises using an integrated fuzzy decision approach. Systems 11 (1), 11. doi:10.3390/systems11010011
Dong, T., Yin, S., and Zhang, N. (2023a). The interaction mechanism and dynamic evolution of digital green innovation in the integrated green building supply chain. Systems 11 (3), 122. doi:10.3390/systems11030122
Hou, J., Song, H., and Li, L. (2019). Non-R and D investment, knowledge accumulation and green innovation growth of China 's manufacturing industry. J. Syst. Manag. 28 (01), 67–76+85.
Jiang, G. (2013). On after-sales technical support and after-sales technical support and customer satisfaction relationship. Silicon Val. 01, 159–181.
Jiang, X. (2007). Research on the construction and countermeasures of independent innovation capability system of national defense industry enterprises. Harbin, China: Harbin Engineering University.
Jie, X., and Zhu, Q. (2021). How to solve the problem of " harmonious symbiosis " in enterprise green innovation practice. Manag. world 37 (01), 128–149+9.
Lu, X. (2015). Collaborative mode of enterprise low carbon innovation based on urban green transformation. Sci. Technol. Prog. Countermeas. (04), 67–71.
Luo, Y., and Jia, N. (2023). Management ability, green innovation and sustainable development of enterprises - - based on the panel data of listed manufacturing companies in China. Bus. Account. 2023 (03), 64–70.
Mao, Q., and Huo, B. (2002). Technology innovation risk and assessment. Quantitative Tech. Econ. Res. 2 (16), 1–5.
Meltzer, J. (2014). A carbon tax as a driver of green technology innovation and the implications for international trade. Energy Law J. 35, 45.
Pietrobelli, C., and Rabellotti, R. (2011). Global value chains meet innovation systems: Are there learning opportunities for developing countries? World Dev. 39 (7), 1261–1269. doi:10.1016/j.worlddev.2010.05.013
Quan, H. (2017). Research on technological innovation efficiency and its influencing factors of Chinese industrial enterprises under environmental constraints. Harbin, China: Harbin Engineering University.
Ren, J., Zhang, F., and Cheng, J. (2021). Analysis of green innovation index model of manufacturing industry based on WSR methodology. Account. Mon. 2021 (08), 133–140.
Sui, J., Bi, K., Yang, Z., and Liu, G. (2015). Research on the influence mechanism of multinational technology transfer on the green innovation performance of China 's manufacturing green innovation system. China soft Sci. (01), 118–129.
Sun, Y. Y., Bi, K. X., and Yin, S. (2020a). Measuring and integrating risk management into green innovation practices for green manufacturing under the global value chain. Sustainability 12 (2), 545. doi:10.3390/su12020545
Sun, Y. Y., Wu, L., and Yin, S. (2020b). Green innovation risk identification of the manufacturing industry under global value chain based on grounded theory. Sustainability 12, 10270. doi:10.3390/su122410270
Tan, L. (2015). Research on the construction and evaluation of intellectual property management system for military-civilian enterprises. Harbin, China: Harbin Engineering University.
Wang, J., and Liu, J. (2020). The impact of ambidextrous green innovation on the competitive advantage of Chinese manufacturing enterprises: The moderating effect of technological turbulence. Sci. Technol. Manag. Res. 40 (09), 196–204.
Wang, M. (2017). Research on risk management of harbin M bridge project. Harbin, China: Harbin Polytechnic Institute.
Wang, M., and Tan, W. (1999). A note on group decision characteristic root method. J. Jiangxi Normal Univ. ( Nat. Sci. Ed. ) (03), 34–37.
Wang, X., Yang, Y., and Wang, L. (2022). Contingent information disclosure: A new explanation of external financing on the smooth failure of corporate green innovation. Manag. Rev. 34 (08), 123–133.
Wang, X., and Yin, H. (2013). Improvement and application of interval Shapley value method for fuzzy cooperative games. Comput. Eng. Appl. 49 (15), 60–64+79.
Wang, X., and Wang, L. (2020). Performance gap and enterprise green innovation - - contingency thinking based on the decision-making practice of " poor thinking. J. Shanghai Univ. Finance Econ. 22 (01), 18–33.
Wu, J., and Fan, H. (2021). Research on the driving mode of enterprise green innovation based on configuration perspective. Res. Dev. Manag. 33 (04), 41–53.
Xiao, J., Zeng, P., and Ren, G. (2022). How to improve the green transformation performance of manufacturing industry - configuration research based on TOE framework. Sci. Res. 40 (12), 2162–2172.
Xiao, T., Liu, H., and Fan, Y. (2014). Research on the influencing factors of service innovation in manufacturing enterprises. Chin. J. Manag. 04, 591–598.
Xiao, Z., Zhang, Y., Shechter, D., Ahn, S. H., Fabrizio, L. A., Erdjument-Bromage, H., et al. (2009). WSTF regulates the H2A.X DNA damage response via a novel tyrosine kinase activity. Account. Mon. 457 (24), 57–62. doi:10.1038/nature07668
Ye, C. (2021). Financing constraints, government subsidies and enterprise green innovation. statistics Decis. 37 (21), 184–188.
Ye, J., Zhang, Y., and Wan, Y. (2021). Top management team risk preference, failure re-innovation behavior and re-innovation performance - - an empirical study based on listed companies in China 's pharmaceutical manufacturing industry. Stat. Res. 38 (08), 59–67.
Keywords: global value chain, green innovation, risk identification, group decision characteristic root, index screening
Citation: Sun Y (2023) Green innovation risk index screening under the global value chain based on the group decision characteristic root method. Front. Environ. Sci. 11:1208497. doi: 10.3389/fenvs.2023.1208497
Received: 19 April 2023; Accepted: 04 July 2023;
Published: 03 August 2023.
Edited by:
Shi Yin, Hebei Agricultural University, ChinaCopyright © 2023 Sun. This is an open-access article distributed under the terms of the Creative Commons Attribution License (CC BY). The use, distribution or reproduction in other forums is permitted, provided the original author(s) and the copyright owner(s) are credited and that the original publication in this journal is cited, in accordance with accepted academic practice. No use, distribution or reproduction is permitted which does not comply with these terms.
*Correspondence: Yingying Sun, c3VueWluZ3lpbmcyMDE5QDEyNi5jb20=
Disclaimer: All claims expressed in this article are solely those of the authors and do not necessarily represent those of their affiliated organizations, or those of the publisher, the editors and the reviewers. Any product that may be evaluated in this article or claim that may be made by its manufacturer is not guaranteed or endorsed by the publisher.
Research integrity at Frontiers
Learn more about the work of our research integrity team to safeguard the quality of each article we publish.