- 1Centre for Digital Telecommunication Technologies, Saint Petersburg Electrotechnical University “LETI”, Saint Petersburg, Russia
- 2Institute of Environmental Sciences, Kazan Federal University, Kazan, Russia
- 3Institute for Fundamental Medicine and Biology, Kazan Federal University, Kazan, Russia
Increased anthropogenic pressure including intensification of agricultural activities leads to long-term decline of natural biotopes, with planted forests often considered as promising compensatory response, although reduced biodiversity and ecosystem stability represent their common drawbacks. Here we present a complex investigation of the impact of a large Grey Heron (Ardea cinerea L.) colony on soil biogeochemistry and vegetation in a planted Scots pine forest representing a natural in situ experiment on an engineered ecosystem. After settling around 2006, the colony expanded for 15 years, leading to the intensive deposition of nutrients with feces, food remains and feather thereby considerably altering the local soil biogeochemistry. Thus, lower pH levels around 4.5, 10- and 2-fold higher concentrations of phosphorous and nitrogen, as well as 1.2-fold discrepancies in K, Li, Mn, Zn and Co., respectively, compared to the surrounding control forest area could be observed. Unaltered total organic carbon (Corg) suggests repressed vegetation, as also reflected in the vegetation indices obtained by remote sensing. Moreover, reduced soil microbial diversity with considerable alternations in the relative abundance of Proteobacteria, Firmicutes, Acidobacteriota, Actinobacteriota, Verrucomicrobiota, Gemmatimonadota, Chujaibacter, Rhodanobacter, and Bacillus has been detected. The above alterations to the ecosystem also affected climate stress resilience of the trees indicated by their limited recovery from the major 2010 drought stress, in marked contrast to the surrounding forest (p = 3∙10−5). The complex interplay between geographical, geochemical, microbiological and dendrological characteristics, as well as their manifestation in the vegetation indices is explicitly reflected in the Bayesian network model. Using the Bayesian inference approach, we have confirmed the predictability of biodiversity patterns and trees growth dynamics given the concentrations of keynote soil biogeochemical alternations with correlations R > 0.8 between observations and predictions, indicating the capability of risk assessment that could be further employed for an informed forest management.
1 Introduction
In recent decades, engineered ecosystems characterized by considerable physical alterations of the local environments have attracted significant attention (Jones et al., 1994). Plantation ecosystems, including planted forests, are often viewed as a compensatory response to the continuous long-term decline of the total area covered by natural biotopes, mainly due to human interventions (Tilman and Lehman, 2001). However, typically limited plant composition represents their common drawback, leading to the reduction in the local soil microbiota diversity and stability (Chen et al., 2019), that in turn appears an essential driver of the flora diversification and vegetation productivity (Van Der Heijden et al., 2008). These complex symbiotic interactions largely govern stress resilience and adaptation capabilities of the entire ecosystem (Cardinale et al., 2012; Liang et al., 2016), especially under combined stress factors, imposing major challenges for the engineered ecosystems management. Therefore, a better understanding of the complex interplay between soil geochemistry, microbial biodiversity and flora vegetation dynamics that are inevitable components of the overall ecosystem stability and its adaptation capabilities is essential for overcoming the above challenges.
While the main focus of interest has been traditionally shifted towards anthropogenic effects, multiple studies of zoogenic and ornithogenic ecosystems largely focused on the unique and vulnerable habitats in the polar regions and on remote islands (Zmudczyńska-Skarbek et al., 2015; Zeglin et al., 2016; Rowe et al., 2017; Zmudczyńska-Skarbek and Balazy, 2017; Abakumov, 2018; Guo et al., 2018; Potapowicz et al., 2020; Abakumov et al., 2021; Nizamutdinov et al., 2021). Nevertheless, terrestrial ornithogenic ecosystems are also of considerable interest, since they often represent long-term natural testbeds of locally altered environments, and thus provide significant information on the adaptability of local flora and fauna, that can be studied without introducing any additional anthropogenic invasion.
Large bird colonies occupying relatively compact areas deposit excessive amounts of nutrients (Frederick and Powell, 1994; Ashworth et al., 2020), leading to considerable alterations in the soil geochemistry and microbiota, affecting the surrounding vegetation, and thus altogether considerably altering the local ecosystems (Sekercioglu, 2006; Whelan et al., 2015; Natusch et al., 2017; Lowney and Thomson, 2021; Grant et al., 2022; Hawke, 2022; Lowney and Thomson, 2022). For example, the impact of large birds like cormorants (Phalacrocorax carbo), Adélie penguins (Pygoscelis adeliae), Eurasian Crane (Grus grus) and Grey Heron (Ardea cinerea) has been intensively studied (Goc et al., 2005; Hobara et al., 2005; Kameda et al., 2006; Adamonytė et al., 2013; Klimaszyk et al., 2015; Klimaszyk and Rzymski, 2016; Guo et al., 2018; Matulevičiūtė et al., 2018; Veum et al., 2019; Al Shehhi and Muzaffar, 2021; Machač et al., 2022; Valkó et al., 2022). These birds form large colonies on coasts and forests leading to an intensive deposition of allochthonous substances in the local environment and consequent eutrophication, in turn altering the soil biogeochemistry, degrading the biodiversity and suppressing plants vegetation (Ishida, 1996; Anderson and Polis, 1999; Hobara et al., 2005; Kolb et al., 2012; Adamonytė et al., 2013). In turn, these colonies could be viewed as long-term natural testbeds often contributing to the local environment over decades, where the endpoint of this multi-year natural experiment could be observed and analyzed here and now.
Soil microbiomes have been proposed as sensitive indicators of global change and an integral part of biogeochemistry (Oyugi et al., 2006; Varin et al., 2012; Santamans et al., 2017). The structure of microbiome significantly depends on carbon and nitrogen (Ligeza and Smal, 2003; Harrow et al., 2006; Schaefer et al., 2014; Tytgat et al., 2016; Otero et al., 2018), moisture (Lavian et al., 2001), and phosphorus (Chong et al., 2009; Roesch et al., 2012; Kim et al., 2014). In turn, the forest floor quality and biogeochemistry deposition are among common consequences of the ornithogenic ecosystem alterations (Hobara et al., 2001; Ellis, 2005). Thus, the birds feaces, which are extremely rich in phosphorus (Osono, 2012; Zhu et al., 2014; Wurster et al., 2015; Domínguez et al., 2017; Telesford-Checkley et al., 2017; Otero et al., 2018), nitrogen (Legrand et al., 1998; Tomassen et al., 2005; Barrett et al., 2006; Aislabie et al., 2008) and ammonia (Mizutani and Wada, 1988; Zhu et al., 2011; Riddick et al., 2014; Crittenden et al., 2015; Croft et al., 2016), as well as organic matter (Huang et al., 2014; Chen et al., 2020), affect the soil and sediment microbiomes (Zhu et al., 2015; Shen et al., 2023). Moreover, they introduce significant concentrations of Mg, Ca, K and Zn (Ellis et al., 2006; Breuning-Madsen et al., 2010; García et al., 2011) into the affected soil, having significant impact on both microbial communities (Wang et al., 2015; Santamans et al., 2017; Minkina et al., 2022) and fungal diversity (Adamonytė et al., 2013; Kutorga et al., 2013) of the latter. These alterations initiate consequent considerable shifts in the soil macrofauna (Korobushkin and Saifutdinov, 2019) and formation of unique ornithophilic and ornithochorous vegetation (Bradbury et al., 2005; Azpiroz and Blake, 2016).
Conifer trees including Scots pines (Pinus sylvestris L.) are among the common nesting sites and thus are considerably affected by bird colonies (Kutorga et al., 2013; Żółkoś et al., 2013). While some investigations reported much better growth of pines at the sites with bird droppings (Tomassen et al., 2005), the long-term impact of bird feaces leads to the suppression of the trees and forest-specific plants growth, followed by their eventual replacement with ruderal and light-preferring plants due to both drastic changes in the soil biogeochemistry, as well as toxic properties of the bird feaces (Ishida, 1996; García et al., 2011). Finally, intensive vegetation of nitrophylic plants leads to the accumulation of dead biomass and diversity degradation (Jones et al., 1994; Mun, 1997; Moore, 2006).
Despite a large number of studies on the impact of bird colonies on soils and surrounding vegetation, many of them have limited focus either on soil geochemistry or on its microbiota or vegetation dynamics. In this work, we aimed at a better understanding of the complex interplay of various geographical, geochemical, microbiological, and dendrological characteristics, as well as their manifestation in the vegetation indices obtained by remote sensing observations in a Bayesian network statistical model representing the overall impact on the local environment.
We consider a regularly planted pine forest area as a prominent testbed, where the emergence and the following 15-year long expansion of a Grey Heron colony could be viewed as a nearly perfect in situ natural experiment, with surrounding unaffected forest of initially the same age, composition and diversity representing a relevant control. We analyze how long-term deposition of nutrients from the bird colony altered the local soil biogeochemistry both under the current nesting sites and in the surrounding area, leading to major alterations in the local microbiota. In turn, these conditions affected the trees and other surrounding flora growth, that has been particularly reflected in their increased vulnerability to combined biogeochemical and climate stresses, as well as reduced adaptational capabilities indicated by their limited recovery from the major 2010 drought. Finally, we also propose a statistical model reflecting the complex interplay between various factors from initial biogeochemical contributions by the bird colony to their effect on the vegetation dynamics and its reflection in the multispectral vegetation indices, and show how this model could be potentially employed in a couple of prediction scenarios.
2 Materials and methods
2.1 Study area, Grey Heron colony and sampling locations
The studied site is located near the southern edge of the Republic of Tatarstan, on the peninsula formed by the confluence of Volga, the largest and the longest river in Europe, and its largest tributary Kama, ashore of a smaller river Myosha (a tributary of Kama) at 55.46885°N, 49.34276°E. The site is represented by a regular forest area planted in 1983 consisting solely of Scots pines. As of summer 2022, the average height of the trees was 13 m, and the average trunk diameter reached 22 cm.
Being surrounded by two major streams near the point of their confluence altogether forming the largest artificial water reservoir in Europe, with vast areas covered by shallow waters, the location forms an extremely attractive habitat for birds due to large amounts of available food sources. The particular study site was occupied by a large grey heron colony since approximately 2006, consisting of 280 bird nests (as of summer 2022) located on the top of the Scots pine trees (Figure 1B). Following the decline and death of the trees supporting the initial nesting sites, the colony has expanded forming a consecutively widening circular area (Figure 1C), with the images taken at the time of analysis (15th June 2022) shown in Figure 1.
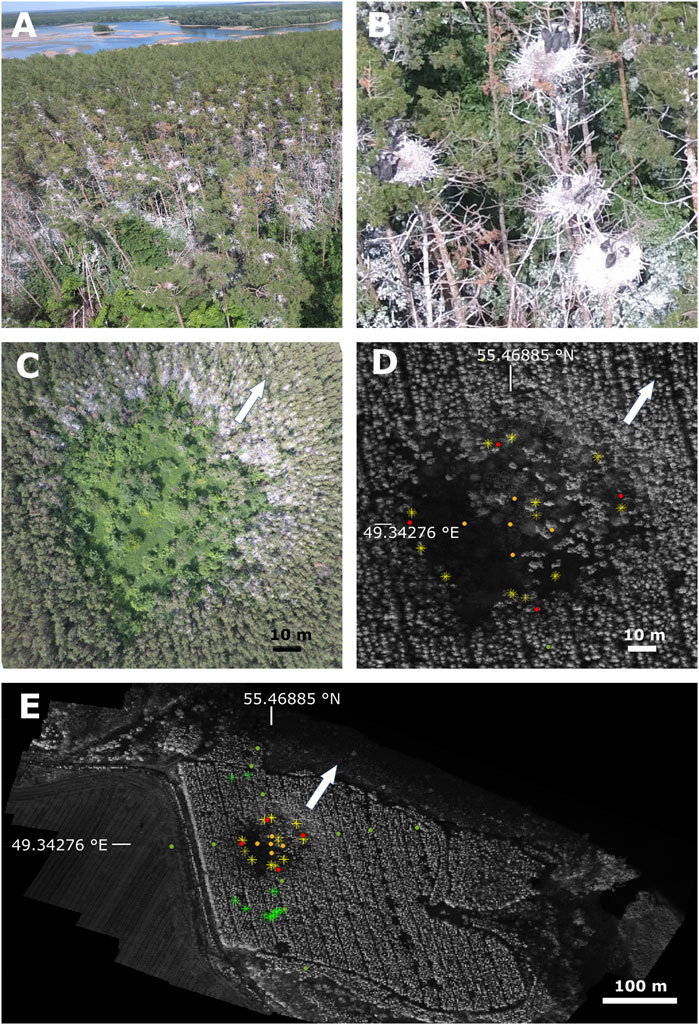
FIGURE 1. An aerial overview of the study area. The nests (around 280) are located on the tops of the trees (A), and two to four birds can be observed per nest (B) resulting in the colony size well above 500. (C) The colony overall view of the colony indicates a ring shape with no trees in the inner circle. (D, E) show the sampling points in the study area, arrow shows the direction of view shown in (A), coordinates correspond to the central sampling point within the inner circle. Full circles denote soil sampling points, including (i) four red bullets in the circular area nested by the Grey Heron colony, (ii) five orange bullets in the inner circle, and (iii) eleven green bullets in the outer area, the latter acting as relevant controls. Asterisks denote Scots Pine trees where tree-ring data have been collected, including yellow in the presumably affected area and green outside, the latter acting as relevant controls.
Soil samples were taken from a depth of 5–10 cm which is the most active zone for the plant roots. In total, 20 sampling sites arranged in a cross-shaped pattern with the crossover position coinciding with the center of the bird colony have been selected (Figures 1D, E). Five sampling sites were located within the inner circle (one at the center and four at the half distance between the center and the current nesting sites), four within the current nesting area, ten in the surrounding forest presumably unaffected by the bird colony, with one single site outside the forest area at the edge of an agricultural cropland (barley field).
The selected soil samples were transported at +4C and stored at −80C until being subjected to a complex biogeochemical analysis.
2.2 Chemical analysis
In the soil samples, the following geochemical properties have been investigated: pH, Corg, biogenic elements N, P, K, as well as 50 other chemical elements. Samples were dried in an oven at 105°C to constant mass. Soil pH was measured by adding 50 mL of distilled water to 10 g of dry soil, mixing over 3 min and keeping for another 5 min before recording pH with a pH meter (Hanna Instruments, Germany). The content of Corg was determined on Delta V Plus isotope mass spectrometer (ThermoFisher Scientific, Germany) with Flash HT attachment in constant flow mode. The other chemical elements were determined using inductively coupled plasma mass spectrometry (ICP-MS) on iCAP Qc (Thermo Fisher Scientific, Germany) and by CHNS/O elemental analysis on vario EL cube (Elementar, Germany) following standard protocols.
2.3 16S rRNA gene-based metagenomic analysis
Extraction and purification of soil DNA for metagenomic analysis was carried out using the Fast DNA®SPIN Kit for Soil (MP Biomedicals, Irvine, United States) and a Fast Prep®24 homogenizer (MP Biomedicals, United States) according to the manufacturer’s instructions.
A 16S rRNA sequencing library was constructed according to the 16S metagenomics sequencing library preparation protocol (Illumina, San Diego, CA, United States) targeting the V3 and V4 hypervariable regions of the 16S rRNA gene. The initial PCR was performed with template DNA using region-specific primers shown to have compatibility with Illumina index and sequencing adapters (forward primer: 5′). After purification of PCR products with AMPure XT magnetic beads, the second PCR was performed using primers from a Nextera XT Index Kit (Illumina). Subsequently, purified PCR products were visualized using gel electrophoresis and quantified with a Qubit dsDNA HS Assay Kit (Thermo Scientific, Germany) on a Qubit 2.0 fluorometer. The sample pool (4 nM) was denatured with 0.2 N NaOH, diluted further to 4 pM, and combined with 20% (v/v) denatured 4 pM PhiX, prepared following Illumina guidelines. Sequencing of 16S rRNA gene V3-V4 variable regions was performed on the Illumina MiSeq platform in 2 × 300bp mode. Reads were analyzed using the QIIME2 software, version 2022.8 (http://qiime2.org/) (Bolyen et al., 2019). Before filtering, there were 39515 read pairs per sample on average. Raw reads were processed using DADA2 algorithm implemented in QIIME (Callahan et al., 2016). After quality filtering, chimera and phiX sequences removal, we analyzed 11429 joined read pairs per sample on average. The taxonomy was assigned to the sequences using pre-trained on the latest SILVA 138 database 99% OTUs Naive Bayes classifier (Quast et al., 2012). To characterize the richness and evenness of the bacterial community, alpha diversity indices were calculated using Chao1, Shannon, Simpson, and Faith PD metrics.
Raw reads are deposited in the SRA under Project ID PRJNA933899 in the fastq format (https://www.ncbi.nlm.nih.gov/sra/PRJNA933899).
2.4 Multispectral remote sensing
Multispectral remote sensing has been performed using the Geoscan 401 Geodesy drone equipped with the five-band RedEdge-MicaSense Mx camera (pixel size 3.75 μm, resolution 1,280 × 960 (1.2 MP x 5 imagers), sensor size 4.8 mm × 3.6 mm, focal length 5.4 mm, output bit depth 12-bit) from 120 m height. Based on the multispectral images, ten different vegetation indices have been calculated, altogether providing 15 channels for further analysis, summarized in Supplementary Table S2 (Buschmann and Nagel, 1993; Gitelson et al., 1996; Rouse et al., 1974; Noe et al., 2001; Chuvieco et al., 2002; Haboudane et al., 2002; Huete et al., 2002; Haboudane et al., 2004; Ueno et al., 2006; Dogan, 2009; Yilmaz et al., 2014).
2.5 Tree-ring measurements
Scots pine trees, altogether n = 43, among them n = 22 located in the area surrounding the inner circle directly affected by the bird colony, and n = 21 located in the outer area, acting as a relevant control. Trees sampling was carried out according to the methodology adopted in earlier dendrochronological studies (Cook and Kairiukstis, 2013). The cores were extracted from the trees with a Pressler borer. Tree ring width (TRW) was measured on a Lintab-6 with the TSAPWin software package (Rinn, 2003). The quality of the cross-chronologies was assessed using the Cofecha software (Grissino-Mayer, 2001). The exact location of each studied tree was determined using a Garmin GPSMAR 62S GPS receiver as indicated in Figure 1.
2.6 Trend evaluation in tree-ring data
To evaluate trends in the tree ring width (TRW) data series, especially in the local time windows of length L, we followed the methodology described in (Bogachev et al., 2017) and performed linear regression analysis, and from the regression fits
Since the observed relative trend may be due to the natural variability of the data series, we compared the observational trends x with the probability distribution
where
Since the observational records obtained in this study were too short to evaluate their persistence properties, we have followed a recently reported consensus model for TRW data that is a long-term correlated series with Hurst exponent
We simulated 16 surrogate records of size
2.7 Statistical analysis
The considered geographical, biogeochemical, dendrological, and remote sensing based vegetation metrics have been first tested for the normality of observations at each of the three local areas (in the circular area surrounded by the nests directly affected by the bird colony, within the inner circle, and in the outer area) using Shapiro-Wilk statistical test with significance threshold at p = 0.05. Since in each considered category of the data, at least some of the essential metrics indicated significant deviations from the Gaussian distribution (see section 3.1 for more details), in the following we applied non-parametric methods. To reveal statistically significant discrepancies between these areas, we employed the one-way non-parametric ANOVA (Kruskal–Wallis) statistical test (or the Mann-Whitney U-test for pairwise comparisons) with significance threshold at p = 0.05.
2.8 Bayesian network
In order to combine the soil biogeochemistry, metagenomic and tree-ring data, taking into account that the number (n = 43) and location of the trees did not coincide with the soil sampling points (n = 20), weighted averages of the tree-ring data metrics for each sampling point has been calculated. Based on the assumption that the concentrations of locally introduced chemical substances and biological sediments decay approximately exponentially with increasing distance from the nests, that is a common assumption in the sediment distribution models (Van Dijk et al., 2002; Zhang and Wirtz, 2017), weights were taken inversely proportional to the logarithms of the distances between the locations of the sampling points and of the analyzed trees and renormalized for each sampling point, respectively.
For the analysis of interrelations between multiple factors affecting the ecosystem, we employed Spearman’s rank correlation analysis and reconstructed a non-parametric Bayesian network interaction model (Hanea et al., 2015), following a methodology similar to (Paprotny and Morales-Nápoles, 2017; Koot et al., 2023).
The practical algorithm of the Bayesian network reconstruction based on rank correlations included the following steps.
In the first step, only those factors that exhibited statistically significant discrepancies according to the one-way non-parametric ANOVA (Kruskal–Wallis) test at p < 0.05 were included in further analysis.
In the second step, we calculated pairwise non-parametric Spearman’s cross-correlation coefficients for all possible pairs of the remaining factors and represented them in the form of the cross-correlation matrix
In the third step, potentially plausible links have been selected by expert assessment. More specifically, either impossible or highly improbable causal affects, such as, for example, impacts of the current vegetation indices (observed at the time of the field investigations) on the trends in the tree growth dynamics (accumulated over many years prior to the time of the field investigations), have been excluded from the model by expert assessment due to the violation of the causality principle, while all potentially relevant links have been marked as feasible.
In the fourth step, to create a graph representing a Bayesian networks structure that is, by definition, implies a directed acyclic graph, the remaining loops were eliminated from the model. For that, a simple procedure which implied finding any existing loop and eliminating the “weakest” link within that loop, as indicated by the pairwise rank cross-correlation coefficient, was repeated until the resulting graph appeared fully acyclic.
3 Results
3.1 Statistical assessment
In each considered category of the data (geographical, biogeochemical, dendrological, and remote sensing based vegetation indices) significant deviations from the Gaussian distribution have been revealed according to Shapiro-Wilk statistical test with significance threshold at p = 0.05. These included the (by definition, one-sided) distribution of the distances from sample points to the colony center, concentrations of some essential chemicals, including Mn and Fe in the inner and nests areas, respectively, as well as the majority of vegetation indices obtained by multispectral remote sensing, relative trends in TRW data, the abundance data for the majority of considered bacterial taxons, and the Simpson’s biodiversity index in the outer area. Accordingly, in the following we refer to the results of non-parametric statistical methods, such as Kruskal–Wallis or Mann-Whitney tests, Spearman’s correlation coefficient, and non-parametric Bayesian network (NPBN) graphs.
3.2 Soil geochemistry
Our results indicate that the bird colony has dramatically altered the local soil geochemistry compared to the surrounding area that we consider presumably unaffected by sediments (feces, feathers, food remains, etc.), and the effect can be observed also in the center of the colony. Thus, the soil pH was 4.7 under the nests and about 5.3 in the center of the circle area representing the early location of the colony and currently surrounded by the nests compared to ∼6.0 at a reasonable distance of the colony (Figure 2). While the total organic carbon (Corg) did not differ significantly, concentrations of nitrogen (N) and potassium (K) were considerably higher in the current nesting area and decreased only slightly in inner circle area. Notably, in the inner area the concentration of phosphorous (P) was nearly 2-fold higher compared to the area where the birds are currently nesting, and up to10-fold higher compared to the outer area (Figure 2). The impact of nests on the concentration of the above biogenic elements is also reflected in the pronounced negative correlation between their concentrations and distance of the sampling points from the nests (Supplementary Figure S1). Among other chemical elements, in the inner area significant changes by 20%–25% has been observed for Li, Mn, Co. and Zn, while no significant differences between the current nesting area and the surrounding forest could be observed. No significant changes could be detected in the concentration of several other elements (Supplementary Figure S2), including Mg, Ca, Fe, Ni, As and Se, which were reported in previous works to be changed due to birds activity (Figure 2).
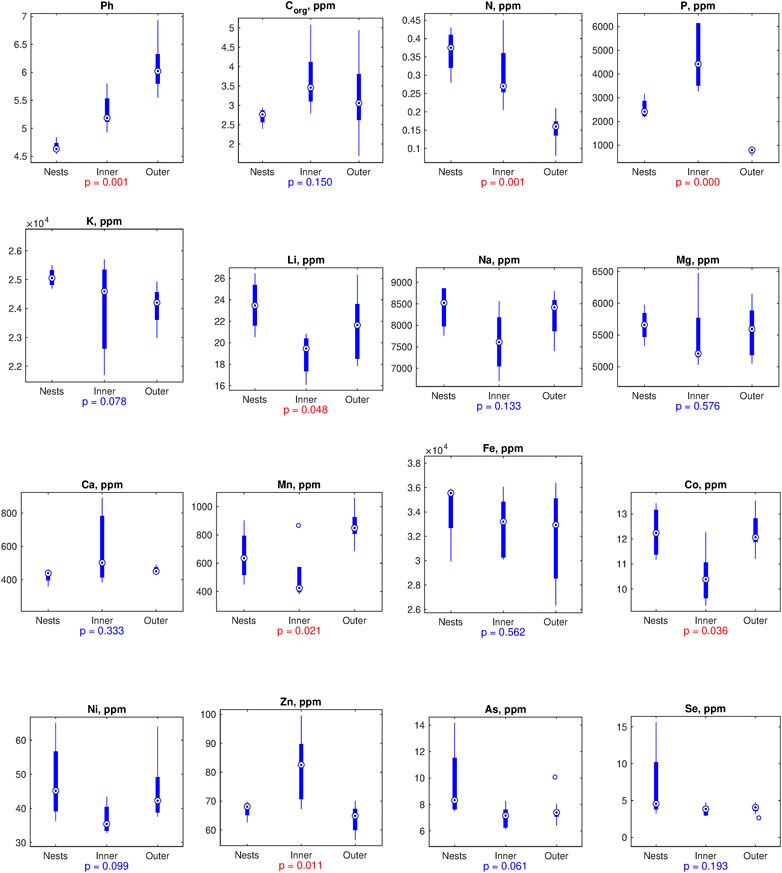
FIGURE 2. Boxplots represent relevant soil geochemical properties (Ph and chemical elements) in the samples collected in the central circle (inner), in the nested area (nests), and in the surrounding unaffected forest (outer). Significance of the differences according to one-way non-parametric ANOVA (Kruskal-Wallis test) is annotated by p-values, with p < 0.05 highlighted by red color.
3.3 Soil microbiota
A total of 750792 raw reads were obtained, which produced 217147 merged non-chimeric sequences after a series of treatments, averaging 11429 valid sequences per sample. Supplementary Figure S3 summarizes the bacterial diversity indices in 3 areas (Chao1, Faith PD, Shannon, Simpson, and number of observed features). While significant differences between the nesting area and the surrounding presumably unaffected forest area could be observed only for the Simpson’s index, all indices demonstrated similar tendency indicating reduced microbial diversity in the nesting area. Significant discrepancies between the relative abundance under the nests and outside the colony area are summarized in Supplementary Figures S4–S7; Supplementary Table S1. On the phyla level, significant discrepancies could be observed for Proteobacteria (33.8% and 21.7%), Actinobacteriota (26.5% and 33.3%), Firmicutes (8.7% and 1.3%), Acidobacteriota (7.3% and 12.9%), Planctomycetota (6.0% and 5.5%), Verrucomicrobiota (1.7% and 10.7%), Gemmatimonadota (0.5% and 3.8%), Myxococcota (0.1% and 1.6%), Patescibacteria (2.5% and 0.3%), Armatimonadota (0.5% and 0.1%), Methylomirabilota, Elusimicrobiota and Abditibacteriota (Figure 3). Nevertheless, the observed changes in the relative abundance of phyla were moderately correlated with the distance to either nests or colony center, with the highest impact on Acidobacteriota, Verrucomicrobiota, Gemmatimonadota and unidentified bacteria (Supplementary Figure S8).
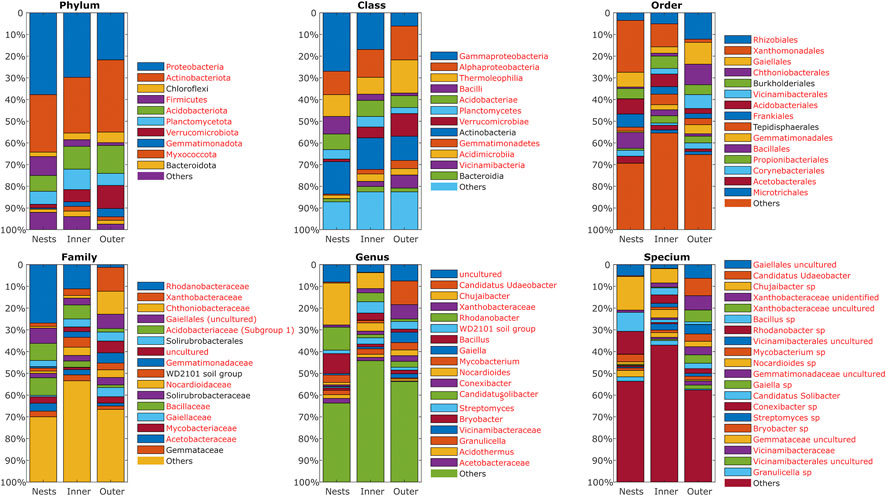
FIGURE 3. Comparison of microbiota structure from the soil samples in the central circle (inner), in the nested area (nests) and in the unaffected forest (outer).
At the class level, the most abundant classes with significant differences were Gammaproteobacteria (27.0% and 6.1%), Alphaproteobacteria (10.8% and 15.6%), Thermoleophilia (27.0% and 15.3%), Bacilli (8.1% and 1.2%), Verrucomicrobiae (1.2% and 10.5%), Actinobacteria (14.9% and 11.2%) (Supplementary Figure S5). Notably, in the nests area the relative abundance of dominant genera Chujaibacter (13.5%), Rhodanobacter (7.4%), Bacillus (6.4%), and uncultured bacteria of Gaiellales (5.6%) and Acidobacteriales (3.2%) were 7–10 fold higher compared to both inner and outer areas, while Gaiella (2.5%), Chloroflexi KD4-96 (2.5%), Candidatus_Udaeobacter from Chthoniobacteraceae (5.6%) and uncultured bacteria from Xanthobacteraceae (6.7%) and Gemmatimonadaceae (2.5%) were present only in the unaffected area (Supplementary Table S1).
3.4 Impact on vegetation
Multispectral channels and vegetation indices (summarized in Supplementary Table S2) were assessed for the entire analysis area (see Supplementary Figure S9). Circle areas with 8 m radius around the sampling sites were selected and median values were calculated for each channel and vegetation index, respectively. Figure 4 shows that among physical channels, only NIR indicated significant differences between the three studied areas (inner circle, current nesting area and surrounding forest). Among studied vegetation indices, most of them indicated U-shaped patterns, reflecting poor vegetation within the inner circle, suppressed conditions near the nesting sites, in contrast to the relatively good situation in the surrounding forest. While seven different indices reflecting qualitatively similar patterns indicated significant discrepancies between studied areas, for the overall model, we have chosen the MTVI2 index, since it provided with the smallest number of outliers in the statistical analysis (although due to pronounced correlations between multiple indices, see Supplementary Figure S10, selection of some alternative index would not affect the interpretation significantly). However, two specialized indices reflected a nearly reversed pattern, the Burn Area Index (BAI) commonly used to characterize marks of burns in wooden areas, as well as Iron Oxide (IO) index, a geological index commonly associated with the presence of iron oxide under the canopy, presumably reflecting considerable alterations in the soil geochemistry.
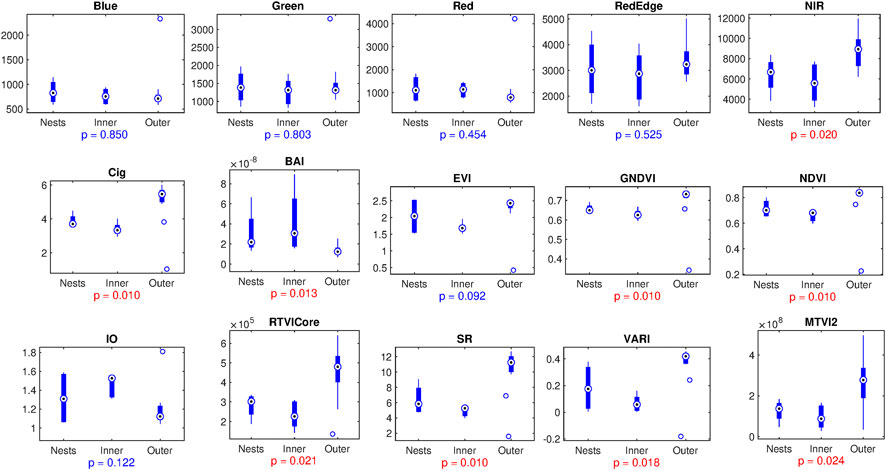
FIGURE 4. Boxplots representing spectral channels, as well as vegetation and geological indexes obtained by multispectral remote sensing in the central circle (inner), in the nested area (nests) and in the unaffected forest (outer). Significance of the differences according to one-way non-parametric ANOVA (Kruskal-Wallis test) is annotated by p-values, with p < 0.05 highlighted by red color.
3.5 Impact on pine trees growth dynamics
Figure 5A shows the TRW data series from 1986 until 2021 (total duration 36y) obtained from Scots pines located within the circle area directly affected by the bird colony (n = 22) and outside of this area (n = 21) as a relevant control (total n = 43). The figure shows that the TRW dynamics exhibits three different phases. In the first phase, the rapid growth rate of young trees is gradually declining approximately until 1995, when it is substituted by relatively flat rate growth in the second phase, approximately until 2005–2007, that coincides with the emergence and early expansion of the bird colony.
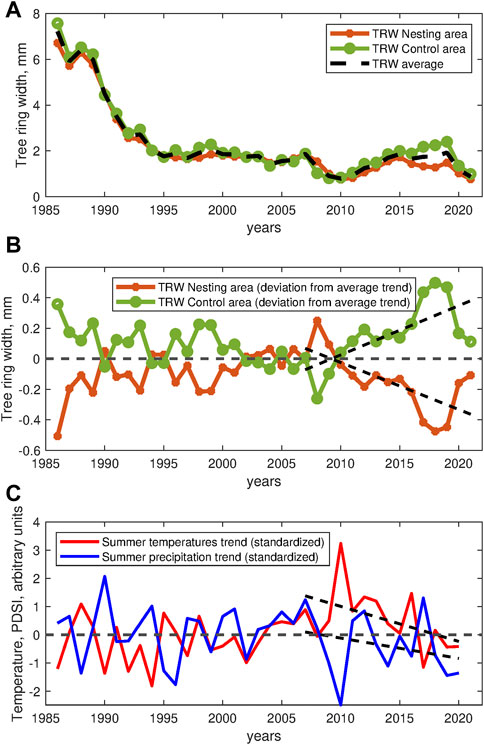
FIGURE 5. Tree ring width (TRW) data for the Scots Pine trees over 36-year time span (1986–2021) in the presumably impacted area (red curve) and outside (green curve), as denoted with in Figure 1 with asterisks, as well as their overall average (black dashed curve). (A) The averaged TRW data, (B) the TRW deviations from the overall average, (C) Standardized summer (July-September) trends for temperatures (red) and precipitation (blue).
Accordingly, the third phase represents a combined stress response pattern to the bird colony and to the hydroclimate anomaly associated with a major summer heatwave accompanied by a flash drought event in 2010, as indicated by the summer temperature and the Palmer Drought Severity Index (PDSI) extremes, see Figure 5C. We next focus on this particular stress response pattern and analyze the relative dynamics of the TRW series within the 15y window starting from 2007, that was the last year when the growth was nearly identical in both considered areas, until 2021. To highlight the discrepancies, we estimate the overall average trend and show deviations of the trends in the presumably affected and control areas from the average trend, see Figure 5B.
Starting from 2010, the temperature trend exhibits a temporary reversal, and PDSI stabilizes within the range corresponding to rather neutral conditions, until another drought emergies in 2019 (indicated by low PDSI, see Figure 5C). While the above changes in the hydroclimate conditions induced a recovery in the tree growth dynamics, this recovery appeared much more pronounced in the area unaffected by biogeochemical stress governed by the bird colony. To quantify the above effect, we calculated the relative trends
While statistically significant discrepancies between the relative TRW trends x in the area affected by the bird colony and in the surrounding areas have been observed (p = 3∙10−5, Mann-Whitney U-test), when compared to the null hypothesis that the observed trend remains within the natural variability of the long-term TRW fluctuations characterized by long-term correlations with H = 1, only two records in the affected area indicated a significant negative trend (and no positive trends could be observed), while three records in the surrounding area indicated positive trends (and no negative trends could be observed), respectively, see Supplementary Figure S11A.
4 Discussion
Increasing anthropogenic influence on the environment drastically changes the ecological profile of areas with high population density and/or intensive agricultural activity, that in turn requires real-time evaluation and prediction of the ecosystem status for a timely risk assessment and informed environmental management. While the engineered ecosystems partially compensate the decline of the natural biotopes, on the other hand, they are characterized by considerable alterations in the local environments and soil biogeochemistry (Jones et al., 1994; Tilman and Lehman, 2001).
In this research, we analyzed complex interrelations between the soil geochemistry, microbial biodiversity and flora vegetation dynamics in a planted Scots pine forest hosting a large colony of Grey Heron, see Figure 1 for location details. Being nested from approximately 2006, the colony consists of approximately 280 bird nests (as of summer 2022) located on the tops of mature Scots pines trees being surrounded by a relatively unaffected forest area. Given the initially uniform characteristics of the regularly planted forest area, the surrounding forest of the same age, composition and diversity, but barely affected by the bird colony, represents a relevant control. Thus, the investigated Grey Heron colony appears a prominent natural in situ experiment unfolding the changes in an engineered ecosystem in response to the excessive deposition of both biogenic elements (N, P) and toxic organics in the soil, as well as considerably reduced pH values compared to the presumably unaffected surrounding forest area. The above conditions are representative for both natural areas occupied with birds (Goc et al., 2005; Hobara et al., 2005; Kameda et al., 2006; Adamonytė et al., 2013; Klimaszyk et al., 2015; Klimaszyk and Rzymski, 2016; Guo et al., 2018; Matulevičiūtė et al., 2018; Veum et al., 2019; Al Shehhi and Muzaffar, 2021; Machač et al., 2022; Valkó et al., 2022) as well as agricultural environments (Bradbury et al., 2005; Minkina et al., 2022). Accordingly, considering the emergence of the bird colony around 2006 and its further expansion as an experimental input, one can currently observe an engineered ecosystem with accumulated alterations in soil biogeochemistry and consequent impact on the trees and other surrounding vegetation as an endpoint of a 15-year long natural experiment.
In order to select the keynote factors that govern the ecosystem changes, and thus could be used as indicators for predicting significant alterations in the environment, a cross-correlation matrix between them was obtained (Figure 6), that in turn allowed to design a non-parametric Bayesian network model (Figure 7) revealing the complex interplay between various ecosystem components, where the nodes are associated with particular geochemical, microbiological, dendrological and vegetation indicators, while the edges are characterized by their interrelations quantified by respective rank correlations R. In order to simplify the model by eliminating weak and often secondary effects, in its graphical representation we show only those correlations R exceeding an empirically chosen threshold 0.6.
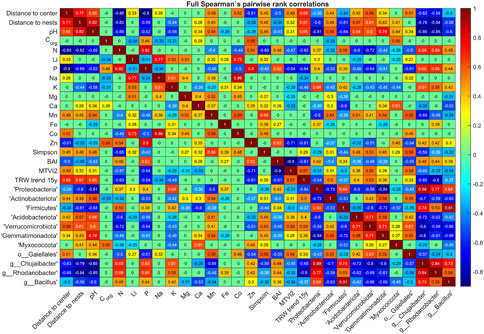
FIGURE 6. Spearman’s pairwise rank correlation coefficients between multiple factors: geographical (distance to either the center of the colony or the nesting sites from the sampling points), geochemical, microbiological, dendrological and observational characteristics exhibiting significant differences between at least two out of the three areas—the central circle (inner), the nested area (nests) and the unaffected surrounding forest (outer).
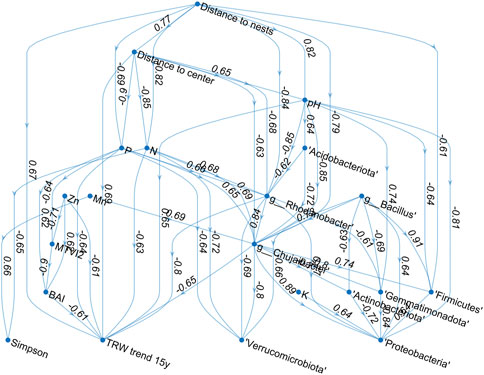
FIGURE 7. Graphical representation of the Bayesian network summarizing a combined model of complex interrelations between geographical, geochemical, microbiological and dendrological characteristics as well as their manifestation in the vegetation indices obtained by remote sensing observations.
Degradation of biodiversity and suppression of plants vegetation in areas occupied with large bird colonies has been reported by many authors (Hobara et al., 2001; 2005; Adamonytė et al., 2013). In the investigated Grey Heron colony, apparently because of high toxicity of the bird feces (Ishida, 1996; García et al., 2011) and extreme pH decrease to 4.5 (Figure 2), the death of trees under the nests was observed in the initial location of the colony with the maximum exposition time to excessive nutrients accumulation, followed by the formation of a consecutively widening circular area with the expansion of the bird colony on the surrounding trees (Figure 1C). This fact is also reflected in the positive correlations between the trees growth dynamics (TRW index) and pH (R = 0.65, Figure 6), indicating negative impact of the soil acidity on the pine trees growth. Notably, there are also positive correlations between the trees growth dynamics and microbial diversity represented by the Simpson’s index (R = 0.29), suggesting that the repression of trees growth is associated with the reduction of the soil microbiota, in agreement with recent literature data. As well, the overall vegetation characterized by the MTVI2 index is positively correlated with pH, while demonstrating a weaker association than for the pine trees growth (R = 0.31 vs. 0.57), since other types of vegetation appear to be less sensitive to soil acidity.
As well, because of the influence of the bird feces, feathers, food remains, etc., dramatic changes in the soil biogeochemistry have been observed. In particular, significant increase of biogenic elements like nitrogen (∼10 fold) and phosphorous (∼4 fold) in the soil could be observed, while the organic carbon (Corg) was even reduced compared to the inner and the outer areas presumably reflecting the repression of the vegetation (see Figure 2). Interestingly, while maximum concentrations of nitrogen have been observed in the area under the trees currently nested by the birds, phosphorous exhibited its maximum concentrations in the inner circle corresponding to the initial location of the colony, that could be attributed to its presumable sequestration in non-soluble organic matters, an effect that has been observed in earlier studies (Brenner et al., 2006; Turner et al., 2006).
Suppression of the vegetation, directly reflected in the measured vegetation indices MTVI2 and BAI obtained from multispectral remote sensing data, see Supplementary Figure S9 and Figure 4, is also in agreement with the tree-ring data indicating that the trees growth rates were considerably reduced in the inner circle and in the nesting areas. As one can see from Figure 5, there are pronounced discrepancies between the TRW dynamics in the affected and unaffected areas. Remarkably, in the year 2008 trees in the area affected by the bird colony exhibited higher growth rates than in the control areas, presumably due to the fertilizing effects of the bird manure containing excessive concentrations of nitrogen (N) and phosphorus (P). However, despite of the obvious discrepancy, the growth rate altogether reduced in both areas continuously from 2008 until 2010 that coincides with the gradual enhancement of summer temperatures reaching its apogee in a major heatwave in 2010 accompanied by a flash drought indicated by a gradual PDSI decline.
To further quantify the combined biogeochemical and climate stress resilience of the trees, we next consider the 10-year time span between 2010 and 2019 corresponding to the recovery from the prolonged drought prior and during the extreme 2010 heatwave. We find that about one-half of all TRW records in the control area indicated significantly positive trend, while only three records in the impacted area followed a significant recovery pattern, see Supplementary Figure S11B. Remarkably, five TRW records in the affected area demonstrated negative x estimates, including one statistically significant negative trend, indicating severely reduced ability to recover from the drought stress under concomitant impact from the biogeochemical alterations induced by the bird colony.
In addition, statistically significant differences between the inner circle (inner), the nesting area (nests), and the surrounding unaffected forest (outer) could be observed not only in the relative trend x, but also in the average TRW data over the same 15-year period, as well as over shorter time spans, in particular, since the point of divergence (2007–2019), as well as over a more recent 5-year time span (2015–2019), see Supplementary Figure S12. However, high correlations between all above metrics reveal their strong interconnection, and thus only the relative trend that exhibited the most pronounced and significant discrepancies between studied sites has been considered for further analysis (see Supplementary Figure S12).
Furthermore, despite of the excessive deposition of nitrogen and available phosphorous with bird feces (Osono, 2012; Wurster et al., 2015; Crittenden et al., 2015; Domínguez et al., 2017; Telesford-Checkley et al., 2017; Otero et al., 2018), reduced microbial diversity could be observed in the soils of the colony area (Supplementary Figure S3) (R = 0.45–0.46, see Figure 6). These experimental observations are reflected in the Bayesian network model reconstruction (Figure 7). The model reveals explicitly that the colony has a great impact on pH, nitrogen and phosphorous of the soil, which in turn affects all other indicators like trees growth rate (TRW), microbial diversity (Simpson’s index) and some given bacteria.
Besides of the extreme deposition of nitrogen and phosphorous, the bird colony areas are characterized with increased concentrations of K, Na, Mg, Ca, Fe and Zn (Ellis et al., 2006; Breuning-Madsen et al., 2010; García et al., 2011) in soils, that in turn also affects soil microbiota (Wang et al., 2015; Santamans et al., 2017; Minkina et al., 2022). In our investigation, the ∼1.2 fold increase in the concentration was observed only for K in the nested area (R = −0.44, although statistically insignificant) and Zn (R = −0.61) in the central area of the colony (Supplementary Figure S2). Therefore, these elements are not linked with the nests position in the network (Supplementary Figure S10), although they affect the microbiota structure. Of note, the concentration of Zn is linked with trees growth and vegetation indices BAI and MTVI2. Additionally, while the quantities of Li, Co. and Mn were significantly reduced in the inner area compared to the nests and outer areas, only Mn was found to significantly correlate with microbial diversity. Remarkably, the concentrations of Li, Co., Fe and Ca are positively correlated with the microbial diversity (R = 0.32…0.48), while concentations of Li and Co. decrease in the inner area of the colony (R = 0.33 and 0.52, respectively). Finally, geochemical anomalies are reflected in the BAI index that is positively associated with the concentrations of N, P, Zn and Fe (R = 0.27…0.62), while negatively correlated with pH (R = −0.43, although some associations appear relatively weak and thus are not shown in the network).
The relevance of Arsenic (As) and Samarium (Sm) in the nests area is questionable and their increase could be a secondary indicator of the accumulation of an unlocalized contamination. Arsenic has been reported earlier to accumulate in bird tissues and feces from the environment (Sánchez-Virosta et al., 2018; Eeva et al., 2020). Although Samarium is widely investigated for the development of both antimicrobial and antitumor agents (Morais et al., 2014; Kratochwil et al., 2021; Zahmatkesh et al., 2022), no data regarding its accumulation in bird tissues are available.
The above alterations in soil geochemistry, especially the dramatic contrast of pH (Green et al., 2012) accompanied by a major excess in nitrogen and phosphorous (Teixeira et al., 2013; Gao et al., 2021), apparently represented the main factors leading to the changes in soil microbiota structure, compared to presumably unaffected areas (Figure 7). The increased abundance of Proteobacteria and Firmicutes, as well as decrease in Acidobacteriota and Actinobacteriota fit with similar changes in soils occupied by bird colonies and increased nitrogen reported earlier (Teixeira et al., 2013; Gao et al., 2021), represented by terminal nodes in the reconstructed network (Figure 7). While the linkage of Verrucomicrobiota, Gemmatimonadota and Myxococcota with birds has not been described yet, the decrease of this normal soil flora in the colony area (Supplementary Figure S2) is significant (R = 0.34…0.59, Figure 6), and thus could reflect negative changes in geochemistry and structure of the soil, and could act as relevant biomarkers of the changes in the soil ecosystem (Supplementary Figure S8). Nevertheless, only Gemmatimonadota is included in the final network based on the desired correlation threshold.
Finally, four genera Chujaibacter, Rhodanobacter, Bacillus and uncultured bacteria of Gaiellales were the most abundant ones in soils under the nests (Figure 3). Among them, only Chujaibacter, Rhodanobacter, and Bacillus could serve as biomarkers, since they are linked with many other nodes in the network (Figure 7). Relatively high abundance of Bacillus in the soil could be a consequence of their introduction with bird feather (Sotnychuk et al., 2020), that is reflected in correlation with nests location (R = −0.56). By contrast, significant increase in the abundance of Chujaibacter, the bacterium reported to be efficient in sulfur transformation and carbohydrates degradation (Zhang et al., 2022), and Rhodanobacter, reported earlier as a resident of highly contaminated areas with low pH (Green et al., 2012) is observed in the inner area of colony (R = −0.63…–0.68, Figure 6). In turn, Gaiellales were shown to be dependent of organic and fatty acids content (Sun et al., 2022).
While detailed investigation of the interconnections among various factors governing the local environment is essential for the understanding of their complex interplay represented by the graph in Figure 7, it appears timely and costly. A more practical application scenario would be to employ the revealed association patterns for the risk assessment and prediction making in local environments based on selected measurements only. From the environmental management point of view, the typical question would be, how a given natural (such as an emergence of a bird colony) and/or anthropogenic (such as introduction of a bird farm or another agricultural activity) invasions could impact similar ecosystems? Answering these questions would require simulation of different scenarios leading to the projected concentrations of nutrients and other chemicals introduced by the invasion and estimating potential impacts on the soil microbial diversity and vegetation dynamics for these scenarios.
Figure 8 shows one test example containing a reduced cross-correlation matrix (panel A) and a corresponding NPBN graph (panel B) indicating the interrelation between the concentrations of the keynote chemicals in the soil, the diversity of the soil microbial community and the local tree growth dynamics. To test the potential predictability, we employed Bayesian inference with a bootstrap-based validation scheme according to (Koot et al., 2023) with the upper layer of the graph (pH and keynote chemicals) in Figure 8B representing the input variables, while predicting the variables in the lower layer of the same graph. Figure 8C shows the results of the regression analysis between the observational and the predicted soil microbial diversity and tree ring width trend metrics, both characterized by correlation coefficients R > 0.8. We believe that the above result indicates the capability of the putative evaluation and prediction of future trends in ecosystem adaptation and environmental alterations based on the proposed approach.
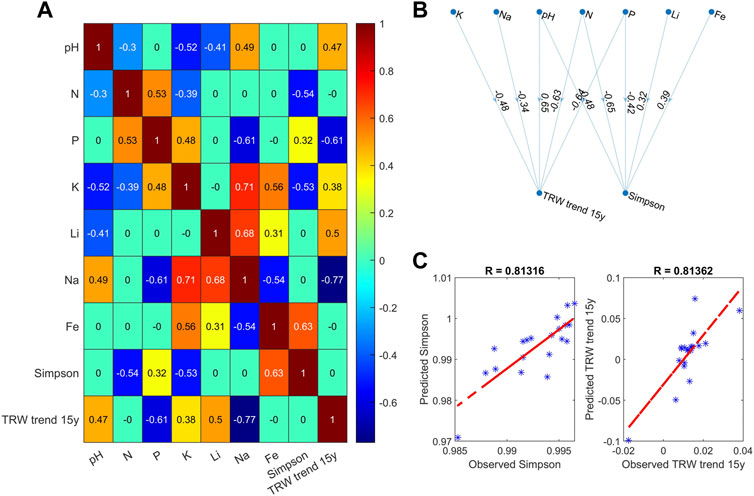
FIGURE 8. Graphical representation of the reduced model exemplifying the predictability of the Simpson’s biodiversity index and tree ring width dynamics for given concentrations of keynote soil chemicals: (A) matrix of Spearman’s correlation coefficients between model parameters; (B) non-parametric Bayesian network, with input parameters in the upper layer and predicted indicators in the lower layer; (C) linear regression analysis between observed and predicted Simpson’s index and TRW trend.
5 Conclusion
To summarize, this study investigated the complex impact of a major Grey Heron colony on a planted Scots pine forest viewing it as a natural in situ experiment on an engineered ecosystem with limited plant and microbial diversity, leading to its increased vulnerability to combined biogeochemical and climate stress. We have shown explicitly that the long-term deposition of nutrients from the bird colony starting from its initial location at the time of emergence around 2006 and further expansion over the surrounding area in the next 15 years considerably altered the local soil biogeochemistry. Among the most pronounced effects, pH reduced to 4.5, as well as nearly 10-fold higher concentrations of phosphorous and 2-fold higher concentrations of nitrogen in their maximum accumulation areas compared to the presumably unaffected surrounding forest area could be observed, while Corg did not change significantly. Moreover, contrasts in the soil microbial diversity with considerable discrepancies in the relative abundance of several bacterial genera (Chujaibacter, Rhodanobacter, Bacillus, uncultured bacteria of Gaiellales and Acidobacteriales) has been observed both in the inner area of the colony and under the nested trees, in turn altogether affecting the trees growth and other surrounding flora vegetation. The latter is reflected in MTVI2 and BAI vegetation indices obtained by remote sensing, and apparently unravels the increased vulnerability of the forest ecosystem to combined biogeochemical and climate stress, as indicated by the limited recovery of the affected trees from the major 2010 drought stress, in marked contrast to the surrounding forest in terms of the annual growth rate (p = 3∙10−5, Mann-Whitney U-test). Based on the results obtained, we proposed a combined non-parametric Bayesian network model reflecting the complex interplay between geographical, geochemical, microbiological and dendrological characteristics, as well as their manifestation in the vegetation indices obtained by remote sensing observations. Finally, based on the revealed interrelations, using the Bayesian inference approach with a bootstrap based validation scheme, we have shown that the NPBN is capable of predicting both Simpson’s diversity index and tree ring width trend from just a few input variables representing the concentrations of the keynote chemicals in the soil with correlation coefficient R between observations and model based predictions exceeding 0.8. The above result indicates the capability of the putative evaluation and prediction of environmental trends in the ecosystem status and could further facilitate an informed adjustment of the planted forest management by an early planning of possible intervention scenarios.
Data availability statement
The datasets presented in this study can be found in online repositories. The names of the repository/repositories and accession number(s) can be found below: https://www.ncbi.nlm.nih.gov/, PRJNA933899.
Author contributions
MB, DT, and AK contributed to the conceptualization and methodology of the study. DT, AG, RI, and BU performed field investigations. BG, MM, and VR performed laboratory analysis. MB, DK, MM, NP, SP, AMS, and AK contributed to data analysis and interpretation of the results. ANS and AK performed overall project management and resource coordination. All authors contributed to the article and approved the submitted version.
Funding
The authors would like to acknowledge the support of this work by the Russian Science Foundation (project No. 22-76-10042), https://rscf.ru/project/22-76-10042/. Metagenomic analysis was performed with support from the Ministry of Science and Higher Education in the framework of the state assignment in the sphere of research activities (project No. FZSM-2023-0013).
Acknowledgments
The authors would like to kindly acknowledge the opportunity to access the resources provided by the shared equipment use center at Kazan Federal University.
Conflict of interest
The authors declare that the research was conducted in the absence of any commercial or financial relationships that could be construed as a potential conflict of interest.
Publisher’s note
All claims expressed in this article are solely those of the authors and do not necessarily represent those of their affiliated organizations, or those of the publisher, the editors and the reviewers. Any product that may be evaluated in this article, or claim that may be made by its manufacturer, is not guaranteed or endorsed by the publisher.
Supplementary material
The Supplementary Material for this article can be found online at: https://www.frontiersin.org/articles/10.3389/fenvs.2023.1197657/full#supplementary-material
References
Abakumov, E. (2018). Content of available forms of nitrogen, potassium and phosphorus in ornithogenic and other soils of the Fildes Peninsula (King George Island, Western Antarctica). Biol. Commun. 63 (2), 109–116. doi:10.21638/spbu03.2018.203
Abakumov, E. V., Parnikoza, I. Y., Zhianski, M., Yaneva, R., Lupachev, A. V., Andreev, M. P., et al. (2021). Ornithogenic factor of soil formation in Antarctica: A review. Eurasian Soil Sci. 54 (4), 528–540. doi:10.1134/S1064229321040025
Adamonytė, G., Iršėnaitė, R., Motiejūnaitė, J., Taraškevičius, R., and Matulevičiūtė, D. (2013). Myxomycetes in a forest affected by great cormorant colony: A case study in western Lithuania. Fungal Divers. 59, 131–146. doi:10.1007/s13225-012-0203-8
Aislabie, J. M., Jordan, S., and Barker, G. M. (2008). Relation between soil classification and bacterial diversity in soils of the Ross Sea region, Antarctica. Geoderma 144 (1-2), 9–20. doi:10.1016/j.geoderma.2007.10.006
Al Shehhi, H., and Muzaffar, S. B. (2021). Impact of nesting Socotra Cormorants on terrestrial invertebrate communities. Insects 12 (7), 615. doi:10.3390/insects12070615
Anderson, W. B., and Polis, G. A. (1999). Nutrient fluxes from water to land: Seabirds affect plant nutrient status on gulf of California islands. Oecologia 118, 324–332. doi:10.1007/s004420050733
Ashworth, A. J., Chastain, J. P., and Moore, P. A. (2020). Nutrient characteristics of poultry manure and litter. Animal manure Prod. Charact. Environ. concerns, Manag. 67, 63–87. doi:10.2134/asaspecpub67.c5
Azpiroz, A. B., and Blake, J. G. (2016). Associations of grassland birds with vegetation structure in the Northern Campos of Uruguay. Condor Ornithol. Appl. 118 (1), 12–23. doi:10.1650/CONDOR-15-49.1
Barrett, J. E., Virginia, R. A., Wall, D. H., Cary, S. C., Adams, B. J., Hacker, A. L., et al. (2006). Co-variation in soil biodiversity and biogeochemistry in northern and southern Victoria Land, Antarctica. Antarct. Sci. 18 (4), 535–548. doi:10.1017/s0954102006000587
Bogachev, M. I., Gafurov, A. M., Iskandirov, P. Y., Kaplun, D. I., Kayumov, A. R., Lyanova, A. I., et al. (2023). Milti-scale detrended partial cross-correlation analysis of tree ring width and climate variations: Revealing heat and drought stress resilience factors in a forest ecosystem. doi:10.1101/2023.05.30.542825
Bogachev, M. I., Yuan, N., and Bunde, A. (2017). “Fractals and multifractals in geophysical time series,” in Fractals: Concepts and applications in geosciences (Florida, United States: CRC Press), 231–271. doi:10.1201/9781315152264
Bolyen, E., Rideout, J. R., Dillon, M. R., Bokulich, N. A., Abnet, C. C., Al-Ghalith, G. A., et al. (2019). Reproducible, interactive, scalable and extensible microbiome data science using QIIME 2. Nat. Biotechnol. 37 (8), 852–857. doi:10.1038/s41587-019-0209-9
Bradbury, R. B., Hill, R. A., Mason, D. C., Hinsley, S. A., Wilson, J. D., Balzter, H., et al. (2005). Modelling relationships between birds and vegetation structure using airborne LiDAR data: A review with case studies from agricultural and woodland environments. Ibis 147 (3), 443–452. doi:10.1111/j.1474-919x.2005.00438.x
Brenner, M., Hodell, D. A., Leyden, B. W., Curtis, J. H., Kenney, W. F., Gu, B., et al. (2006). Mechanisms for organic matter and phosphorus burial in sediments of a shallow, subtropical, macrophyte-dominated lake. J. Paleolimnol. 35, 129–148. doi:10.1007/s10933-005-7881-0
Breuning-Madsen, H., Ehlers-Koch, C., Gregersen, J., and Løjtnant, C. L. (2010). Influence of perennial colonies of piscivorous birds on soil nutrient contents in a temperate humid climate. Geografisk Tidsskrift-Danish J. Geogr. 110 (1), 25–35. doi:10.1080/00167223.2010.10669494
Büntgen, U., Allen, K., Anchukaitis, K. J., Arseneault, D., Boucher, É., Bräuning, A., et al. (2021). The influence of decision-making in tree ring-based climate reconstructions. Nat. Commun. 12, 3411. doi:10.1038/s41467-021-23627-6
Buschmann, C., and Nagel, E. (1993). In vivo spectroscopy and internal optics of leaves as basis for remote sensing of vegetation. Int. J. Remote Sens. 14 (4), 711–722. doi:10.1080/01431169308904370
Callahan, B. J., McMurdie, P. J., Rosen, M. J., Johnson, A. J. A., and Holmes, S. P. (2016). DADA2: High-resolution sample inference from Illumina amplicon data. Nat. methods 13 (7), 581–583. doi:10.1038/nmeth.3869
Cardinale, B. J., Duffy, J. E., Gonzalez, A., Hooper, D. U., Perrings, C., Venail, P., et al. (2012). Biodiversity loss and its impact on humanity. Nature 486 (7401), 59–67. doi:10.1038/nature11148
Chen, C., Chen, H. Y., Chen, X., and Huang, Z. (2019). Meta-analysis shows positive effects of plant diversity on microbial biomass and respiration. Nat. Commun. 10 (1), 1332. doi:10.1038/s41467-019-09258-y
Chen, Y., Shen, L., Huang, T., Chu, Z., and Xie, Z. (2020). Transformation of sulfur species in lake sediments at ardley island and fildes peninsula, king george island, antarctic peninsula. Sci. Total Environ. 703, 135591. doi:10.1016/j.scitotenv.2019.135591
Chong, C. W., Dunn, M. J., Convey, P., Tan, G. A., Wong, R. C., and Tan, I. K. (2009). Environmental influences on bacterial diversity of soils on Signy Island, maritime Antarctic. Polar Biol. 32, 1571–1582. doi:10.1007/s00300-009-0656-8
Chuvieco, E., Martin, M. P., and Palacios, A. (2002). Assessment of different spectral indices in the red-near-infrared spectral domain for burned land discrimination. Int. J. Remote Sens. 23 (23), 5103–5110. doi:10.1080/01431160210153129
Crittenden, P. D., Scrimgeour, C. M., Minnullina, G., Sutton, M. A., Tang, Y. S., and Theobald, M. R. (2015). Lichen response to ammonia deposition defines the footprint of a penguin rookery. Biogeochemistry 122, 295–311. doi:10.1007/s10533-014-0042-7
Croft, B., Wentworth, G. R., Martin, R. V., Leaitch, W. R., Murphy, J. G., Murphy, B. N., et al. (2016). Contribution of Arctic seabird-colony ammonia to atmospheric particles and cloud-albedo radiative effect. Nat. Commun. 7 (1), 13444. doi:10.1038/ncomms13444
Dogan, H. M. (2009). Mineral composite assessment of Kelkit River Basin in Turkey by means of remote sensing. J. Earth Syst. Sci. 118, 701–710. doi:10.1007/s12040-009-0059-9
Domínguez, M. T., Gutiérrez, E., González-Domínguez, B., Román, M., Ávila, J. M., Ramo, C., et al. (2017). Impacts of protected colonial birds on soil microbial communities: When protection leads to degradation. Soil Biol. Biochem. 105, 59–70. doi:10.1016/j.soilbio.2016.11.007
E. R. Cook, and L. A. Kairiukstis (Editors) (2013). Methods of dendrochronology: Applications in the environmental sciences (Berlin, Germany: Springer Science & Business Media).
Eeva, T., Raivikko, N., Espín, S., Sánchez-Virosta, P., Ruuskanen, S., Sorvari, J., et al. (2020). Bird feces as indicators of metal pollution: Pitfalls and solutions. Toxics 8 (4), 124. doi:10.3390/toxics8040124
Ellis, J. C., Fariña, J. M., and Witman, J. D. (2006). Nutrient transfer from sea to land: The case of gulls and cormorants in the gulf of Maine. J. Animal Ecol. 75 (2), 565–574. doi:10.1111/j.1365-2656.2006.01077.x
Ellis, J. (2005). Marine birds on land: A review of plant biomass, species richness, and community composition in seabird colonies. Plant Ecol. 181, 227–241. doi:10.1007/s11258-005-7147-y
Frederick, P. C., and Powell, G. V. (1994). “Nutrient transport by wading birds in the Everglades,” in Everglades: The ecosystem and its restoration (Florida, United: CRC Press), 571–584.
Gao, L., Liu, L., Du, C., and Hou, Q. (2021). Comparative analysis of fecal bacterial microbiota of six bird species. Front. veterinary Sci. Front. Vet. Sci. 8, 791287. doi:10.3389/fvets.2021.791287
García, L. V., Ramo, C., Aponte, C., Moreno, A., Domínguez, M. T., Gómez-Aparicio, L., et al. (2011). Protected wading bird species threaten relict centenarian cork oaks in a mediterranean biosphere reserve: A conservation management conflict. Biol. Conserv. 144 (2), 764–771. doi:10.1016/j.biocon.2010.11.007
Gitelson, A. A., Kaufman, Y. J., and Merzlyak, M. N. (1996). Use of a green channel in remote sensing of global vegetation from EOS-MODIS. Remote Sens. Environ. 58 (3), 289–298. doi:10.1016/S0034-4257(96)00072-7
Goc, M., Iliszko, L., and Stempniewicz, L. (2005). The largest European colony of great cormorant on the vistula spit (N Poland)—an impact of the forest ecosystem. Ecol. Quest. 6, 93–103.
Grant, M. L., Bond, A. L., and Lavers, J. L. (2022). The influence of seabirds on their breeding, roosting and nesting grounds: A systematic review and meta-analysis. J. Animal Ecol. 91 (6), 1266–1289. doi:10.1111/1365-2656.13699
Green, S. J., Prakash, O., Jasrotia, P., Overholt, W. A., Cardenas, E., Hubbard, D., et al. (2012). Denitrifying bacteria from the genus Rhodanobacter dominate bacterial communities in the highly contaminated subsurface of a nuclear legacy waste site. Appl. Environ. Microbiol. 78 (4), 1039–1047. doi:10.1128/AEM.06435-11
Grissino-Mayer, H. D. (2001). Evaluating crossdating accuracy: A manual and tutorial for the computer program COFECHA. Tree-Ring Res. 57 (2), 205–221.
Guo, Y., Wang, N., Li, G., Rosas, G., Zang, J., Ma, Y., et al. (2018). Direct and indirect effects of penguin feces on microbiomes in Antarctic ornithogenic soils. Front. Microbiol. 9, 552. doi:10.3389/fmicb.2018.00552
Haboudane, D., Miller, J. R., Pattey, E., Zarco-Tejada, P. J., and Strachan, I. B. (2004). Hyperspectral vegetation indices and novel algorithms for predicting green LAI of crop canopies: Modeling and validation in the context of precision agriculture. Remote Sens. Environ. 90 (3), 337–352. doi:10.1016/j.rse.2003.12.013
Haboudane, D., Miller, J. R., Tremblay, N., Zarco-Tejada, P. J., and Dextraze, L. (2002). Integrated narrow-band vegetation indices for prediction of crop chlorophyll content for application to precision agriculture. Remote Sens. Environ. 81 (2-3), 416–426. doi:10.1016/S0034-4257(02)00018-4
Hanea, A., Napoles, O. M., and Ababei, D. (2015). Non-parametric Bayesian networks: Improving theory and reviewing applications. Reliab. Eng. Syst. Saf. 144, 265–284. doi:10.1016/j.ress.2015.07.027
Harrow, G., Hawke, D. J., and Holdaway, R. N. (2006). Surface soil chemistry at an alpine procellariid breeding colony in New Zealand, and comparison with a lowland site. N. Z. J. Zoology 33 (2), 165–174. doi:10.1080/03014223.2006.9518441
Hawke, D. (2022). The biogeochemistry and ecological impact of Westland petrels (Procellaria westlandica) on terrestrial ecosystems. N. Z. J. Ecol. 46 (1), 1–16. doi:10.20417/nzjecol.46.3
Hobara, S., Koba, K., Osono, T., Tokuchi, N., Ishida, A., and Kameda, K. (2005). Nitrogen and phosphorus enrichment and balance in forests colonized by cormorants: Implications of the influence of soil adsorption. Plant Soil 268, 89–101. doi:10.1007/s11104-004-0231-6
Hobara, S., Osono, T., Koba, K., Tokuchi, N., Fujiwara, S., and Kameda, K. (2001). Forest floor quality and N transformations in a temperate forest affected by avian-derived N deposition. Water, Air, Soil Pollut. 130, 679–684. doi:10.1023/A:1013869115132
Huang, T., Sun, L., Wang, Y., Chu, Z., Qin, X., and Yang, L. (2014). Transport of nutrients and contaminants from ocean to island by emperor penguins from Amanda Bay, East Antarctic. Sci. Total Environ. 468, 578–583. doi:10.1016/j.scitotenv.2013.08.082
Huete, A., Didan, K., Miura, T., Rodriguez, E., Gao, X., and Ferreira, L. (2002). Overview of the radiometric and biophysical performance of the MODIS vegetation indices. Remote Sens. Environ. 83, 195–213. doi:10.1016/S0034-4257(02)00096-2
Ishida, A. (1996). Effects of the common cormorant, Phalacrocorax carbo, on evergreen forests in two nest sites at Lake Biwa, Japan. Ecol. Res. 11, 193–200. doi:10.1007/bf02347685
Jones, C. G., Lawton, J. H., and Shachak, M. (1994). Organisms as ecosystem engineers. Oikos 69 (3), 373–386. doi:10.2307/3545850
Kameda, K., Koba, K., Hobara, S., Osono, T., and Terai, M. (2006). “Pattern of natural 15 N abundance in lakeside forest ecosystem affected by cormorant-derived nitrogen,” in Limnology and aquatic birds: Proceedings of the fourth conference working group on aquatic birds of societas internationalis limnologiae (SIL), sackville, new brunswick, Canada (Heidelberg: Springer Netherlands), 69–86. August 3–7, 2003.
Kim, H. M., Jung, J. Y., Yergeau, E., Hwang, C. Y., Hinzman, L., Nam, S., et al. (2014). Bacterial community structure and soil properties of a subarctic tundra soil in Council, Alaska. FEMS Microbiol. Ecol. 89 (2), 465–475. doi:10.1111/1574-6941.12362
Klimaszyk, P., Brzeg, A., Rzymski, P., and Piotrowicz, R. (2015). Black spots for aquatic and terrestrial ecosystems: Impact of a perennial cormorant colony on the environment. Sci. Total Environ. 517, 222–231. doi:10.1016/j.scitotenv.2015.02.067
Klimaszyk, P., and Rzymski, P. (2016). The complexity of ecological impacts induced by great cormorants. Hydrobiologia 771, 13–30. doi:10.1007/s10750-015-2618-1
Kolb, G. S., Jerling, L., Essenberg, C., Palmborg, C., and Hambäck, P. A. (2012). The impact of nesting cormorants on plant and arthropod diversity. Ecography 35 (8), 726–740. doi:10.1111/j.1600-0587.2011.06808.x
Koot, P., Mendoza-Lugo, M. A., Paprotny, D., Morales-Nápoles, O., Ragno, E., and Worm, D. T. (2023). PyBanshee version (1.0): A Python implementation of the MATLAB toolbox BANSHEE for non-parametric bayesian networks with updated features. SoftwareX 21, 101279. doi:10.1016/j.softx.2022.101279
Korobushkin, D. I., and Saifutdinov, R. A. (2019). Influence of seabird colonies on soil macrofauna communities at the Black Sea coast forests. Russ. J. Ecol. 50, 567–573. doi:10.1134/S1067413619060080
Kratochwil, C., Giesel, F. L., Rathke, H., Fink, R., Dendl, K., Debus, J., et al. (2021). [153 Sm] Samarium-labeled FAPI-46 radioligand therapy in a patient with lung metastases of a sarcoma. Eur. J. Nucl. Med. Mol. Imaging 48, 3011–3013. doi:10.1007/s00259-021-05273-8
Kutorga, E., Irsenaite, R., Iznova, T., Kasparavicius, J., Markovskaja, S., and Motiejunaite, J. (2013). Species diversity and composition of fungal communities in a Scots pine forest affected by the great cormorant colony. Acta Mycol. 48 (2), 173–188. doi:10.5586/am.2013.019
Lavian, I. L., Vishnevetsky, S., Barness, G., and Steinberger, Y. (2001). Soil microbial community and bacterial functional diversity at machu picchu, king george island, Antarctica. Polar Biol. 24, 411–416. doi:10.1007/s003000100230
Legrand, M., Ducroz, F., Wagenbach, D., Mulvaney, R., and Hall, J. (1998). Ammonium in coastal Antarctic aerosol and snow: Role of polar ocean and penguin emissions. J. Geophys. Res. Atmos. 103 (D9), 11043–11056. doi:10.1029/97jd01976
Liang, J., Crowther, T. W., Picard, N., Wiser, S., Zhou, M., Alberti, G., et al. (2016). Positive biodiversity-productivity relationship predominant in global forests. Science 354 (6309), aaf8957. doi:10.1126/science.aaf8957
Ligeza, S., and Smal, H. (2003). Accumulation of nutrients in soils affected by perennial colonies of piscivorous birds with reference to biogeochemical cycles of elements. Chemosphere 52 (3), 595–602. doi:10.1016/S0045-6535(03)00241-8
Lowney, A. M., and Thomson, R. L. (2022). Ecological engineering across a spatial gradient: Sociable weaver colonies facilitate animal associations with increasing environmental harshness. J. Animal Ecol. 91 (7), 1385–1399. doi:10.1111/1365-2656.13688
Lowney, A. M., and Thomson, R. L. (2021). Ecological engineering across a temporal gradient: Sociable weaver colonies create year-round animal biodiversity hotspots. J. Animal Ecol. 90 (10), 2362–2376. doi:10.1111/1365-2656.13544
Ludescher, J., Bunde, A., Büntgen, U., and Schellnhuber, H.-J. (2020). Setting the tree-ring record straight. Clim. Dyn. 55, 3017–3024. doi:10.1007/s00382-020-05433-w
Machač, O., Ivinskis, P., Rimšaitė, J., Horňák, O., and Tuf, I. H. (2022). In the shadow of cormorants: Succession of avian colony affects selected groups of ground dwelling predatory arthropods. Forests 13 (2), 330. doi:10.3390/f13020330
Matulevičiūtė, D., Motiejūnaitė, J., Uogintas, D., Taraškevičius, R., Dagys, M., and Rašomavičius, V. (2018). Decline of a protected coastal pine forest under impact of a colony of great cormorants and the rate of vegetation change under ornithogenic influence. Silva Fenn. 52 (2), 131–146. doi:10.14214/sf.7699
Minkina, T., Sushkova, S., Delegan, Y., Bren, A., Mazanko, M., Kocharovskaya, Y., et al. (2022). Effect of chicken manure on soil microbial community diversity in poultry keeping areas. Environ. Geochem. Health 2022, 01447. doi:10.1007/s10653-022-01447-x
Mizutani, H., and Wada, E. (1988). Nitrogen and carbon isotope ratios in seabird rookeries and their ecological implications. Ecology 69 (2), 340–349. doi:10.2307/1940432
Moore, J. W. (2006). Animal ecosystem engineers in streams. BioScience 56 (3), 237–246. doi:10.1641/0006-3568(2006)056[0237:aeeis]2.0.co;2
Morais, D. S., Coelho, J., Ferraz, M. P., Gomes, P. S., Fernandes, M. H., Hussain, N. S., et al. (2014). Samarium doped glass-reinforced hydroxyapatite with enhanced osteoblastic performance and antibacterial properties for bone tissue regeneration. J. Mater. Chem. B 2 (35), 5872–5881. doi:10.1039/C4TB00484A
Mun, H. T. (1997). Effects of colony nesting of Adrea cinerea and Egretta alba modesta on soil properties and herb layer composition in a Pinus densiflora forest. Plant Soil 197, 55–59. doi:10.1023/a:1004292103610
Natusch, D. J. D., Lyons, J. A., Brown, G. P., and Shine, R. (2017). Biotic interactions mediate the influence of bird colonies on vegetation and soil chemistry at aggregation sites. Ecology 98 (2), 382–392. doi:10.1002/ecy.1642
Nizamutdinov, T., Andreev, M., and Abakumov, E. (2021). The role of the ornithogenic factor in soil formation on the Antarctic oasis territory Bunger Hills (East Antarctica). Eurasian J. Soil Sci. 10 (4), 308–319. doi:10.18393/ejss.962538
Noe, G. B., Childers, D. L., and Jones, R. D. (2001). Phosphorus biogeochemistry and the impact of phosphorus enrichment: Why is the everglades so unique? Ecosystems 4, 603–624. doi:10.1007/s10021-001-0032-1
Osono, T. (2012). Excess supply of nutrients, fungal community, and plant litter decomposition: A case study of avian-derived excreta deposition in conifer plantations. Environ. Change 2012, 173–196.
Otero, X. L., De La Peña-Lastra, S., Pérez-Alberti, A., Ferreira, T. O., and Huerta-Diaz, M. A. (2018). Seabird colonies as important global drivers in the nitrogen and phosphorus cycles. Nat. Commun. 9 (1), 246. doi:10.1038/s41467-017-02446-8
Oyugi, J. O., Qiu, H., and Safronetz, D. (2006). Global warming and the emergence of ancient pathogens in Canada’s arctic regions. Med. hypotheses 68 (3), 709. doi:10.1016/j.mehy.2006.09.006
Paprotny, D., and Morales-Nápoles, O. (2017). Estimating extreme river discharges in Europe through a Bayesian network. Hydrology Earth Syst. Sci. 21 (6), 2615–2636. doi:10.5194/hess-21-2615-2017
Potapowicz, J., Szumińska, D., Szopińska, M., Bialik, R. J., Machowiak, K., Chmiel, S., et al. (2020). Seashore sediment and water chemistry at the Admiralty Bay (King George Island, Maritime Antarctica)–geochemical analysis and correlations between the concentrations of chemical species. Mar. Pollut. Bull. 152, 110888. doi:10.1016/j.marpolbul.2020.110888
Quast, C., Pruesse, E., Yilmaz, P., Gerken, J., Schweer, T., Yarza, P., et al. (2012). The SILVA ribosomal RNA gene database project: Improved data processing and web-based tools. Nucleic acids Res. 41 (D1), D590–D596. doi:10.1093/nar/gks1219
Riddick, S. N., Blackall, T. D., Dragosits, U., Daunt, F., Braban, C. F., Tang, Y. S., et al. (2014). Measurement of ammonia emissions from tropical seabird colonies. Atmos. Environ. 89, 35–42. doi:10.1016/j.atmosenv.2014.02.012
Rinn, F. (2003). TSAP-Win. Time series analysis and presentation for dendrochronology and 409 related applications. User reference.
Roesch, L. F., Fulthorpe, R. R., Pereira, A. B., Pereira, C. K., Lemos, L. N., Barbosa, A. D., et al. (2012). Soil bacterial community abundance and diversity in ice-free areas of Keller Peninsula, Antarctica. Appl. Soil Ecol. 61, 7–15. doi:10.1016/j.apsoil.2012.04.009
Rouse, J. W., Haas, R. H., Schell, J. A., and Deering, D. W. (1974). Monitoring vegetation systems in the great plains with ERTS. NASA Spec. Publ. 351 (1), 309.
Rowe, J. A., Litton, C. M., Lepczyk, C. A., and Popp, B. N. (2017). Impacts of endangered seabirds on nutrient cycling in montane forest ecosystems of Hawai‘i. Pac. Sci. 71 (4), 495–509. doi:10.2984/71.4.7
Sánchez-Virosta, P., Espín, S., Ruiz, S., Salminen, J. P., García-Fernández, A. J., and Eeva, T. (2018). Experimental manipulation of dietary arsenic levels in great tit nestlings: Accumulation pattern and effects on growth, survival and plasma biochemistry. Environ. Pollut. 233, 764–773. doi:10.1016/j.envpol.2017.10.113
Santamans, A. C., Boluda, R., Picazo, A., Gil, C., Ramos-Miras, J., Tejedo, P., et al. (2017). Soil features in rookeries of Antarctic penguins reveal sea to land biotransport of chemical pollutants. PLoS One 12, e0181901. doi:10.1371/journal.pone.0181901
Schaefer, K., Lantuit, H., Romanovsky, V. E., Schuur, E. A., and Witt, R. (2014). The impact of the permafrost carbon feedback on global climate. Environ. Res. Lett. 9 (8), 085003. doi:10.1088/1748-9326/9/8/085003
Sekercioglu, C. H. (2006). Increasing awareness of avian ecological function. Trends Ecol. Evol. 21 (8), 464–471. doi:10.1016/j.tree.2006.05.007
Shen, L., Huang, T., Chen, Y., Chu, Z., and Xie, Z. (2023). Diverse transformations of sulfur in seabird-affected sediments revealed by microbial and stable isotope analyses. J. Oceanol. Limnol. 41 (1), 138–149. doi:10.1007/s00343-021-1173-z
Sotnychuk, N. M., Cutshaw, L. R., Tuhela, L., and Beckmann, C. (2020). Prevalence of feather-degrading Bacillus spp. on the plumage of birds in Australia. Emu-Austral Ornithol. 120 (1), 65–73. doi:10.1080/01584197.2019.1686995
Sun, L., Wang, Y., Ma, D., Wang, L., Zhang, X., Ding, Y., et al. (2022). Differential responses of the rhizosphere microbiome structure and soil metabolites in tea (Camellia sinensis) upon application of cow manure. BMC Microbiol. 22 (1), 55. doi:10.1186/s12866-022-02470-9
Teixeira, L. C., Yeargeau, E., Balieiro, F. C., Piccolo, M. C., Peixoto, R. S., Greer, C. W., et al. (2013). Plant and bird presence strongly influences the microbial communities in soils of Admiralty Bay, Maritime Antarctica. PLoS One 8 (6), e66109. doi:10.1371/journal.pone.0066109
Telesford-Checkley, J. M., Mora, M. A., Grant, W. E., Boellstorff, D. E., and Provin, T. L. (2017). Estimating the contribution of nitrogen and phosphorus to waterbodies by colonial nesting waterbirds. Sci. Total Environ. 574, 1335–1344. doi:10.1016/j.scitotenv.2016.08.043
Tilman, D., and Lehman, C. (2001). Human-caused environmental change: Impacts on plant diversity and evolution. Proc. Natl. Acad. Sci. 98 (10), 5433–5440. doi:10.1073/pnas.091093198
Tomassen, H. B., Smolders, A. J., Lamers, L. P., and Roelofs, J. G. (2005). How bird droppings can affect the vegetation composition of ombrotrophic bogs. Can. J. Bot. 83 (8), 1046–1056. doi:10.1139/b05-051
Turner, B. L., Newman, S., and Reddy, K. R. (2006). Overestimation of organic phosphorus in wetland soils by alkaline extraction and molybdate colorimetry. Environ. Sci. Technol. 40 (10), 3349–3354. doi:10.1021/es052442m
Tytgat, B., Verleyen, E., Sweetlove, M., D'hondt, S., Clercx, P., Van Ranst, E., et al. (2016). Bacterial community composition in relation to bedrock type and macrobiota in soils from the Sør Rondane Mountains, East Antarctica. FEMS Microbiol. Ecol. 92 (9), fiw126. doi:10.1093/femsec/fiw126
Ueno, Y., Hori, M., Noda, T., and Mukai, H. (2006). Effects of material inputs by the Grey Heron Ardea cinerea on forest-floor necrophagous insects and understory plants in the breeding colony. Ornithol. Sci. 5 (2), 199–209. doi:10.2326/1347-0558(2006)5[199:EOMIBT]2.0.CO;2
Valkó, O., Borza, S., Godó, L., Végvári, Z., and Deák, B. (2022). The Eurasian crane (Grus grus) as an ecosystem engineer in grasslands: Conservation values, ecosystem services, and disservices related to a large iconic bird species. Land Degrad. Dev. 33 (12), 2155–2165. doi:10.1002/ldr.4314
Van Der Heijden, M. G., Bardgett, R. D., and Van Straalen, N. M. (2008). The unseen majority: Soil microbes as drivers of plant diversity and productivity in terrestrial ecosystems. Ecol. Lett. 11 (3), 296–310. doi:10.1111/j.1461-0248.2007.01139.x
Van Dijk, A. I. J. M., Meesters, A. G. C. A., and Bruijnzeel, L. A. (2002). Exponential distribution theory and the interpretation of splash detachment and transport experiments. Soil Sci. Soc. Am. J. 66 (5), 1466–1474. doi:10.2136/sssaj2002.1466
Varin, T., Lovejoy, C., Jungblut, A. D., Vincent, W. F., and Corbeil, J. (2012). Metagenomic analysis of stress genes in microbial mat communities from Antarctica and the High Arctic. Appl. Environ. Microbiol. 78 (2), 549–559. doi:10.1128/AEM.06354-11
Veum, L. M., Dorr, B. S., Hanson-Dorr, K. C., Moore, R. J., and Rush, S. A. (2019). Double-crested cormorant colony effects on soil chemistry, vegetation structure and avian diversity. For. Ecol. Manag. 453, 117588. doi:10.1016/j.foreco.2019.117588
Wang, N. F., Zhang, T., Zhang, F., Wang, E. T., He, J. F., Ding, H., et al. (2015). Diversity and structure of soil bacterial communities in the Fildes Region (maritime Antarctica) as revealed by 454 pyrosequencing. Front. Microbiol. 6, 1188. doi:10.3389/fmicb.2015.01188
Whelan, C. J., Şekercioğlu, Ç. H., and Wenny, D. G. (2015). Why birds matter: From economic ornithology to ecosystem services. J. Ornithol. 156, 227–238. doi:10.1007/s10336-015-1229-y
Wurster, C. M., Munksgaard, N., Zwart, C., and Bird, M. (2015). The biogeochemistry of insectivorous cave guano: A case study from insular southeast asia. Biogeochemistry 124, 163–175. doi:10.1007/s10533-015-0089-0
Yilmaz, P., Parfrey, L. W., Yarza, P., Gerken, J., Pruesse, E., Quast, C., et al. (2014). The SILVA and “all-species living tree project (LTP)” taxonomic frameworks. Nucleic Acids Res. 42 (1), D643–D648. doi:10.1093/nar/gkt1209
Zahmatkesh, H., Mirpour, M., Zamani, H., and Rasti, B. (2022). Effect of samarium oxide nanoparticles fabricated by curcumin on efflux pump and virulence genes expression in MDR Pseudomonas aeruginosa and Staphylococcus aureus. J. Clust. Sci. 34, 1227–1235. doi:10.1007/s10876-022-02274-x
Zeglin, L. H., Wang, B., Waythomas, C., Rainey, F., and Talbot, S. L. (2016). Organic matter quantity and source affects microbial community structure and function following volcanic eruption on Kasatochi Island, Alaska. Environ. Microbiol. 18 (1), 146–158. doi:10.1111/1462-2920.12924
Zhang, W., and Wirtz, K. (2017). Mutual dependence between sedimentary organic carbon and infaunal macrobenthos resolved by mechanistic modeling. J. Geophys. Res. Biogeosciences 122 (10), 2509–2526. doi:10.1002/2017JG003909
Zhang, W., Wu, Y., Wu, J., Zheng, X., and Chen, Y. (2022). Enhanced removal of sulfur-containing organic pollutants from actual wastewater by biofilm reactor: Insights of sulfur transformation and bacterial metabolic traits. Environ. Pollut. 313, 120187. doi:10.1016/j.envpol.2022.120187
Zhu, R., Shi, Y., Ma, D., Wang, C., Xu, H., and Chu, H. (2015). Bacterial diversity is strongly associated with historical penguin activity in an Antarctic lake sediment profile. Sci. Rep. 5 (1), 17231. doi:10.1038/srep17231
Zhu, R., Sun, J., Liu, Y., Gong, Z., and Sun, L. (2011). Potential ammonia emissions from penguin guano, ornithogenic soils and seal colony soils in coastal Antarctica: Effects of freezing-thawing cycles and selected environmental variables. Antarct. Sci. 23 (1), 78–92. doi:10.1017/s0954102010000623
Zhu, R., Wang, Q., Ding, W., Wang, C., Hou, L., and Ma, D. (2014). Penguins significantly increased phosphine formation and phosphorus contribution in maritime Antarctic soils. Sci. Rep. 4 (1), 7055. doi:10.1038/srep07055
Zmudczyńska-Skarbek, K., and Balazy, P. (2017). Following the flow of ornithogenic nutrients through the Arctic marine coastal food webs. J. Mar. Syst. 168, 31–37. doi:10.1016/j.jmarsys.2016.12.006
Zmudczyńska-Skarbek, K., Balazy, P., and Kuklinski, P. (2015). An assessment of seabird influence on Arctic coastal benthic communities. J. Mar. Syst. 144, 48–56. doi:10.1016/j.jmarsys.2014.11.013
Keywords: Grey Heron, biogeochemistry, soil metagenome, tree ring width, vegetation indices
Citation: Bogachev MI, Tishin DV, Gafurov AM, Gareev BI, Imaev RG, Kaplun DI, Markelova MI, Pyko NS, Pyko SA, Romanova VA, Safonova AN, Sinitca AM, Usmanov BM and Kayumov AR (2023) The impact of Grey Heron (Ardea cinerea L.) colony on soil biogeochemistry and vegetation: a natural long-term in situ experiment in a planted pine forest. Front. Environ. Sci. 11:1197657. doi: 10.3389/fenvs.2023.1197657
Received: 31 March 2023; Accepted: 26 May 2023;
Published: 08 June 2023.
Edited by:
Paul V. Doskey, Michigan Technological University, United StatesReviewed by:
Tao Huang, Anhui University, ChinaScott Rush, Mississippi State University, United States
Copyright © 2023 Bogachev, Tishin, Gafurov, Gareev, Imaev, Kaplun, Markelova, Pyko, Pyko, Romanova, Safonova, Sinitca, Usmanov and Kayumov. This is an open-access article distributed under the terms of the Creative Commons Attribution License (CC BY). The use, distribution or reproduction in other forums is permitted, provided the original author(s) and the copyright owner(s) are credited and that the original publication in this journal is cited, in accordance with accepted academic practice. No use, distribution or reproduction is permitted which does not comply with these terms.
*Correspondence: Mikhail I. Bogachev, cm9nZXhAeWFuZGV4LmNvbQ==; Denis V. Tishin, a3BmdWVjb2xvZ3lAZ21haWwuY29t; Airat R. Kayumov, a2FpcmF0ckB5YW5kZXgucnU=