- 1College of Transport and Communications, Shanghai Maritime University, Shanghai, China
- 2School of Economics and Management, Tongji University, Shanghai, China
- 3Naval Medical Center, Naval Medical University, Shanghai, China
The garbage classification recycling policy is proposed to curb the waste of recyclable and land resources to reduce the environmental pollution caused by garbage. This paper establishes a tripartite evolutionary game model with governments, recycling companies, and citizens as stakeholders to discuss their corresponding strategic behaviors. Through the stability analysis, we draw a conclusion that only when governments choose to regulate the classification, while both recycling companies and citizens take an active part in the classification, can the environmental benefit be maximized. In addition, the government and recycling companies are advised to increase the rate at which the evolutionary game model converges to a steady state by reducing their own operating costs during the implementation of garbage classification. On this basis, we also recommend an appropriate increase in the benefits given to citizens, which will have a significantly positive impact on citizens and even also on the government and the recycling companies themselves.
1 Introduction
Based on the fast-developing modernization and increasing population, the amount of waste generated globally will increase from 2 billion tons in 2016 to 3.5 billion tons in the next 30 years (Wang et al., 2019). Increasing municipal garbage poses a significant threat to city sustainability, especially in developing countries (Kuang et al., 2021). From 2013 to 2017, the average growth rate was 9.48%, making China the country with the largest waste output (Wei, 2015). Because the implementation of garbage classification recycling is still in the early stage, phenomena such as residents’ low awareness of garbage classification, the vaguely defined concept of circulation, incomplete separation of wet and dry waste, and insufficient dissemination of relevant knowledge still abound, although a harmless garbage disposal rate in China has been on a significant upward trend in recent years, which is from 52.1% to 99% (National Bureau of Statistics, 2020); it treats just symptoms but not the root cause. Hence, the effect of improving environmental quality is negligible.
Nowadays, the implementation of the waste classification policy has gradually become the top priority to improve environmental quality, resource recycling, and social value realization and has also been closely followed by scholars around the world. Constructing the first three-way evolutionary game model with the government, farmers, and village collectives as stakeholders, Teng et al. (2022) explored the game relationship between the interested parties. By analyzing the replication dynamics equations and stability points, the impact of the decisions made by village collectives on the whole game process and other interested parties in the rural garbage classification process is investigated, which promotes the improvement of the overall operational efficiency of waste separation in rural China. Kang et al. (2020) proposed a concept for an automated waste sorting system based on deep learning—a branch of the machine learning field, aimed at improving the efficiency of front-end waste recycling. Subsequently, Chen et al. (2022) developed a deep learning-based waste sorting system that can be used for automatic identification and recycling of household waste. In addition, a lightweight waste classification model, garbage classification network, called GCNet, was proposed to solve the problems of low accuracy and poor timeliness in the current waste classification process. Peng et al. (2021) constructed a multi-agent decision function, interaction model, and recursive bivariate probit model by using the AnyLogic platform to discuss the influence of residents’ behavior, participation, and enthusiasm on garbage classification. To sum up, numerous studies from multiple perspectives on the implementation of garbage classification show that there is a growing awareness of the urgency of implementing the policy.
However, inappropriate management policies from the government will not only cause a waste of resources and degrade people’s quality of life (Padilla and Trujillo, 2018) but also impose heavy financial burdens on municipal governments (Hu et al., 2019), which will bring serious barriers and threats to sustainable development and human health (Chen et al., 2021; Liu et al., 2021; Xu et al., 2021; Teng et al., 2022). Therefore, the government’s reward and punishment strategy will play a crucial part in the ultimate effectiveness of implementing garbage sorting. In addition, although the influence of the residents’ behavior on garbage classification has been studied in previous studies, those papers have mostly taken this as the starting point, while as the intermediate link, there has been little discussion on recycling companies. In this paper, we not only combine the government policy with the actions of recycling companies and citizens but also consider the potential impact of the recycling companies’ behavior on the citizens and how it will ultimately affect the recycling company itself (Liu and Teng, 2023). As a breakthrough, the influence on the implementation of garbage classification caused by recycling companies' cost is also given.
In terms of the research tool, considering that the theory of evolutionary game is an efficient instrument used to analyze the interaction scenarios of decisions between two and more stakeholders, this article builds a tripartite evolutionary game model with the government, recycling companies, and citizens as stakeholders. It is a quantitative research method which can be used for environmental impact assessment, thus being applied to verify the rationality of decisions made by interested parties (Bond et al., 2016). Based on this, the government might choose to regulate or to ignore in the game process. Recycling companies and citizens also have two strategies: to comply with garbage classification guidelines or not. Before constructing the model, we propose the following five questions and analyze the solutions around them in the later part of the paper, which in turn fulfills the purpose of this research.
(1) What are the premises that need to be met in order to construct a tripartite evolutionary game model?
(2) How to construct the payment matrix based on the different combinations of decisions of the three stakeholders?
(3) How to achieve each stakeholders’ analysis of replication dynamics? What is the corresponding trilateral replication of dynamic equations?
(4) How to give the corresponding evolutionary stability strategy based on the equilibrium in the model?
(5) How should each stakeholder choose the most appropriate evolutionary stability strategy to maximize their own interests?
In response to the aforementioned questions, we establish a tripartite evolutionary game model among the governments, recycling companies, and citizens and establish the payment matrix. In this paper, the dynamic equation of ternary replication is calculated, and the stability evolution process is analyzed. Finally, through numerical simulation, the impact of each parameter in the model on the equilibrium is explored.
The relevant literature is reviewed and collated in the second part of this paper. In the third part, a tripartite evolutionary game model is presented and analyzed. In the next part, simulation analysis and parameter sensitivity analysis are carried out. The final part summarizes and puts forward the direction for future research.
2 Literature review
In this section, we have reviewed and organized the relevant literatures according to the following categories: 1) the government’s strategy and influence in the implementation of garbage classification; 2) garbage sorting behavior of citizens; 3) the strategy of recycling companies in the implementation of garbage classification; 4) application of the evolutionary game in garbage classification.
2.1 The governments’ strategy and influence in the implementation of garbage classification
Over the past 20 years, China has done its best to try to promote household garbage separation and recycling in a wide variety of ways (Liu et al., 2019). In response, numerous national laws and regulations are being introduced, and pilot demonstration work has been carried out in some cities since 2000, involving cities such as Beijing, Shanghai, Guangzhou, Shenzhen, Hangzhou, and Nanjing (Xiao et al., 2020). According to Zhang et al. (2017), even in cities such as Beijing, which was the first to introduce the concept of waste classification, there is still a lack of accuracy in the separation rate, which ranges from 16.92% to 34.56%, the reasons for which include the lack of participation by residents, the low profitability of mid-level recycling companies, the poor operability of government policies due to their lack of systematization, and the inadequacy of measures related to waste recycling. Wertz (1976) first proposed problems related to garbage recycling and classification by urban residents. For this work, scholars such as Fullerton and Kinnaman (1996), Linderhof et al. (2001), and Callan and Thomas (2006) further explored the direct impacts of government incentives and sanctions on residents’ garbage classification. Iyer and Kashyap (2007) found that government incentives are heavily influenced by time and that the stronger the government incentive, the weaker the effect produced by the policy. In addition, the efficiency of sustainable urban waste management may be affected by the inconsistency between residents’ participation behavior and waste segregation management. In particular, waste segregation management mainly consists of publicity and education, supervision and management, and assessment and reward and punishment of segregation management. To address this issue, Cui et al. (2021) constructed a model and comprehensive evaluation index system for compulsory urban household waste sorting management by combining a waste sorting management system with a subject–object–process model to quantify the holistic synergy between waste sorting regulation and citizens’ participation behavior, which in turn also provides substantial and targeted measures for the enforcement of future efforts for waste classification regulation. Zhao et al. (2021) suggested that an effective way to facilitate people’s waste separation behavior is to improve waste collection facilities within communities. In addition, financial incentive is also an effective means of promoting municipal waste separation (Wadehra et al., 2018). At the same time, enhancing credibility, timely empathy for the difficulties in the implementation of waste separation, and mutually beneficial behavior are also crucial indicators to promote the effective implementation of policies on garbage classification management. Public education can provide citizens with a deeper understanding of the concept and value of waste separation, thus facilitating the promotion of waste separation management (Hua et al., 2021).
2.2 Garbage sorting behavior of citizens
The rapid development of urbanization in China has brought about the increasing amount of household waste, which directly affects the citizens’ living space. This indirectly implies that to reduce the production of municipal solid waste and the need for garbage disposal and recycling, household waste-classification activities by the residents are of great significance (Tang et al., 2022). According to Liu et al. (2022), nowadays, two-thirds of China’s cities are surrounded by garbage, and a quarter of them almost have little room for landfill. In these dire times, a top–down revolution in waste classification is imperative, and the willingness of citizens to participate is the key to the success of this revolution. Liu et al. (2022) combined the Theory of Planned Behavior (TPB) and Value-Belief-Norm (NAM) theory, proposing a “motivation-intention-behavior” theoretical model to systematically elaborate the antecedents of domestic garbage classification behavior. The results showed that citizens’ behavior of garbage classification is positively influenced by their intention to separate waste, which is positively influenced by a number of factors, including attitude (ATT), subjective norm (SN), and perceived behavioral control (PBC). In addition, the attitude and perceived intention to separate waste are significantly positively influenced by policy effectiveness (PPE). Wang et al. (2021) investigated the key factors influencing urban citizens’ willingness to sort waste and developed an extended model of planned behavior by combining the expectancy theory (ET) and the norm activation model (NAM). The results showed that expectation (Exp) was a significant positive predictor of willingness to separate waste, and that citizens’ expectation of garbage classification was positively influenced by attitude (AT), perceived behavioral control (PBC), and subjective norms (SN), with subjective norms having the most significant effect on expectation. Bai et al. (2022) found that most citizens were willing to pay for garbage classification, but the amount they were willing to pay for that was in the lower range. In addition, they used an ordered probit model to identify the factors influencing citizens’ willingness to pay for waste sorting. These factors include, in addition to individual characteristics, the frequency of littering, the level of literacy of people, and the level of external publicity. Kuang et al. (2021) conducted a randomized survey of major Chinese cities around citizens’ participation in urban waste classification, which revealed that there is a bias between citizens’ willingness to segregate waste and their waste segregation behavior due to the influence of the environment and their differential perceptions, as the probability of occurrence of waste segregation behavior does not exactly increase with the increase in willingness to separate waste. It is worth noting that citizens who are frequently concerned about the state of urban environmental pollution, familiar with urban waste sorting, or live in communities with well-established garbage sorting facilities are more likely to participate in this activity. Tang et al. (2022) suggested that gender and literacy levels are likely to have an impact on the probability of participation in waste segregation. In addition, the same effect can be attributed to the publicity of waste separation, the planning of related facilities, and the attitude of residents toward environmental protection. Zhao et al. (2021) found that rural residents were significantly less willing to separate their waste than urban residents. This is because rural residents differ significantly from urban residents in terms of the average number of meals per month in the household, the average monthly household food expenditure, household cleanliness, and household crowding, which all have a direct impact on the willingness to separate household waste.
To sum up, the research related to citizens’ garbage sorting behavior has been quite mature up to now, whose objects mainly concentrate on the influencing factors of residents’ garbage sorting behavior and willingness.
2.3 The strategy of recycling companies in the implementation of garbage classification
This paper assumes that the responsibilities of recycling companies include garbage collection, which involves packing the garbage residents put in the garbage collection point and transporting it to the garbage disposal center, and garbage disposal. Therefore, we reviewed the literature related to the recycling and disposal aspects of waste separation. For China, as the leader of developing countries, the excessive consumption of resources and the rapid growth of urban solid waste caused by industrialization and urbanization are increasingly prominent (Mo et al., 2009; Meng et al., 2015; Dong et al., 2016). As resource consumption increases dramatically in China, waste sorting and resource recycling are considered to be the most efficient ways to solve the problem of “waste city” (Dong et al., 2013; Tong and Tao, 2016). Using ML algorithms, Ziouzios et al. (2020) proposed a cloud-based automated machine classification method for recycling plants. They trained an efficient mobile network method able to classify five different types of garbage, which can be put into use on the cloud server in real-time. Adedeji et al. (2019) proposed a smart waste material classification algorithm, i.e., established with the 50-layer residual net pretrain (ResNet-50) CNN method, i.e., ML algorithm, and serves as the extractor, and SVM, i.e., employed for classifying the waste into distinct types or groups like metal, glass, plastic, and paper. Huang et al. (2020) proposed a new combinational classification algorithm depending on three pretrained CNN methods (NASNetLarge, VGG19, and DenseNet169) to process the ImageNet database and attain higher classification performance. Gan et al. (2020) proposed a novel approach to waste recycling classification, which relies on DL techniques and CNN for the construction of classification algorithms and intelligent simultaneous interpretation of waste, resulting in significant improvements in both the speed and accuracy of waste image detection. Narayan (2021) developed a mobile application called DeepWaste by improving DL techniques, which is characterized by its ease of use and immediacy and is able to provide users with a variety of waste sorting services such as recycling and composting. The research used various CNN frameworks for waste detection and classification. Wang et al. (2020) used the application of DL technology in environmental protection as an entry point to implement the problem of identifying and classifying household waste with the help of the CNN VGG16 model.
We can see that most of the existing research studies use algorithms or build a model to establish a new garbage collection and classification system which can be used by departments responsible for recycling and processing garbage. However, there are few studies regarding this kind of department as a stakeholder to analyze how the evolution of its behavior strategy will affect the implementation of garbage classification.
2.4 Application of evolutionary game theory in garbage classification
Evolutionary game theory has a wide range of applications. On the basis of bounded rationality, all stakeholders tend to constantly imitate and adjust to improve strategies (Chen and Hu, 2018). However, there is still a lack of research on the application of the evolutionary game theory in garbage classification. Teng et al. (2022) first constructed the tripartite evolutionary game model of governments, farmers, and village collectives and introduced rural waste charging as a parameter into the model to discuss the game relationship between the three interested parties. Through the analysis of replicated dynamic equations and stability points, combined with realistic numerical simulations, the decision-making characteristics of the tripartite behavior and the evolutionary trend of each stakeholder’s behavior were assessed, and the impact of the behavioral decisions of the village collectives on the overall game process and each other stakeholder during rural waste separation was investigated, facilitating the improvement of the overall operational efficiency of rural waste separation in China. Thus, it is clear that although there is relatively little research in the existing literature in this area, the application of evolutionary games in the implementation of garbage classification is predictable.
The aforementioned research system provides theoretical support for the research of this paper. Based on evolutionary game theory, this paper considers the dynamic competition among the governments, recycling companies, and citizens in the process of garbage classification and establishes a dynamic game among the three participants, which provides policy and theoretical support for the government to promote the implementation of garbage sorting, and also provides business strategies for recycling companies.
3 Model and assumptions
The evolutionary game assumes that the players are bounded rationally (Long et al., 2019). In the initial stage of the game, it is difficult for each player to make a perfect strategy, which maximizes its interests. However, participants can adjust their strategies by working with others and learning from previous experiences. In this way, the final decision made by various stakeholders can maximize their interests. This paper expounds the game relationship among the governments who work as the supervisor, the garbage recycling companies, and the citizens who are practitioners of garbage classification and points out the influence of governments’ supervision and the cost control of the recycling companies on the promotion of garbage classification.
3.1 Assumptions
By analyzing the evolutionary game relationship among multiple stakeholders, we posit the following assumptions:
(1) In the evolutionary game system, each stakeholder takes profit maximization as the ultimate goal, and the information used for communication is open and transparent. Each stakeholder can continuously adjust its strategy according to the behavior of other stakeholders in order to maximize their own interests.
(2) In the garbage classification system, there are only three game players: governments, recycling companies, and citizens. The responsibilities of the recycling companies include garbage disposal and garbage collection, which involves packing the garbage that residents put in the garbage collection point and transporting it to the garbage disposal center.
(3) The dynamic game between governments and recycling companies:
Recycling companies are an important node in the implementation of garbage classification, and their purpose is to further process the garbage put out by citizens, to reduce environmental pollution, and save recyclable and land resources while gaining economic benefits. However, the overall cost of implementing garbage classification is too high, making it difficult for recycling companies to make a profit in a short period of time. Faced with the high cost of equipping and maintaining more elaborate equipment, recycling companies are less motivated to respond to the garbage classification policy. Therefore, the governments’ supervision will be crucial, and the willingness of the governments to adopt active strategies including fines and subsidies will also have an important impact on the recycling companies.
Recycling companies that strictly follow the garbage classification standard will receive a welfare subsidy
If the recycling company does not follow the standard, it will be fined
(4) The dynamic game between governments and citizens:
As the most basic unit and the initiator of garbage classification, citizens play a decisive role in how much benefit can garbage classification produce. However, it will consume a lot of time and energy
(5) The dynamic game between recycling companies and citizens:
Recycling companies play an important role in maintaining the orderly operation of garbage classification, and to a great extent, their environmental awareness affects the behavioral orientation of citizens. If recycling companies choose the active strategy, there will be a positive influence on the behavior of the citizens, thus increasing the output efficiency of environmental benefits produced by garbage sorting behavior such as saving time that is put in garbage classification and promoting the overall efficiency of garbage classification. The potential benefits are
(6) The total cost of recycling companies includes the basic cost for operation and later garbage disposal cost. The basic cost of recycling companies that comply with the garbage classification standard is slightly greater than that of those with the opposite attitude. However, their later garbage disposal cost is much less. Therefore, the total cost required by recycling companies to implement garbage classification is far less than that required by those who do not implement garbage classification, namely,
(7) The subsidy provided by the governments to the recycling companies and citizens depends on how much the long-term benefits the two game players have created for the environment. The welfare provided by the recycling companies to citizens is determined from the quantity of the positive impact that the citizens’ behavior has on them.
(8) In terms of environmental benefits, the three game players are the community of interests, which means the environmental benefits generated by one side will be shared by the three parties.
Table 1 presents the parameters and connotations:
3.2 Combination of game strategies for the three players
The evolutionary game described in this paper involves three stakeholders: governments, recycling companies, and citizens. As the supervisor, governments can select from two strategies: supervision or non-supervision. The following strategies can be selected by recycling companies: act strictly in accordance with the regulation of garbage classification (hereafter referred to as “compliance”) or work on the principle of their own convenience (“incompliance”). Accordingly, as practitioners, citizens also face two choices: implement garbage classification (“participation”) or not (“non-participation”). The following are eight types of game combination among the governments, recycling companies, and citizens: (supervision, compliance, and participation); (supervision, compliance, and non-participation); (supervision, non-compliance, and participation); (supervision, non-compliance, and non-participation); (non-supervision, compliance, and participation); (non-supervision, compliance, and non-participation); (non-supervision, non-compliance, and participation); (non-supervision, non-compliance, and non-participation), as shown in Figure 1. The figure shows the behavior decisions and interest interactions of each stakeholder under different choices.
3.3 Equilibrium analysis of evolutionary game
3.3.1 The expected earnings of each player
By calculating, this paper lists the payment matrix of the tripartite evolutionary game when the governments select Supervision and Non-supervision. The functions given in Table 2 represent the income of the governments, recycling companies, and citizens. In the initial stage of the tripartite evolutionary game, we suppose that if the possibility of the governments selecting supervision
In this paper,
Supposing that
Similarly,
3.3.2 The replicator dynamics analysis of each game player
The replicator dynamics equation is a model used to describe the dynamic mechanism of game players' strategy adjustment. is an ordinary differential equation that describes the frequency or frequency of a specific strategy used in a group.
The replicator dynamics equation of the possibility
where
The replicator dynamics equation of the probability
where
The replicator dynamics equation of the proportion
where
Having obtained the tripartite replication dynamic equation, we need to calculate the stable equilibrium of the dynamically evolving system. One equilibrium point
In addition, the other eight potential equilibrium points that can be easily obtained are as follows:
3.3.3 The stability analysis of the equilibrium strategy
Analysis of the Jacobian matrix is an effective way to obtain the stability of equilibrium (Friedman et al., 1991). Therefore, this paper takes the analysis of the Jacobian matrix as the starting point to analyze the stability of the equilibrium strategy. The Jacobian matrix of the aforementioned replicated dynamic system is
The specific calculation process and results of the Jacobian matrix are shown in Supplementary Appendix C. According to existing theories, we find that only when the values of the Jacobian matrix are all negative is the equilibrium point an ESS. Otherwise, this point is an unstable point. We explore the eight surrounding locations since this model is in tight Nash equilibrium, substituting each point into the Jacobian matrix in the aforementioned equations, and the positive and negative properties of each aforementioned eigenvalue are discussed. Based on the assumptions, the positive and negative characteristics of eigenvalues at the local equilibrium points are judged. As shown in Table 3, which also shows the local stability of the equilibrium point, the following conclusions can be drawn:
(1) When the public reputation is greater than the sum of the basic cost of supervision and the subsidies given to recycling companies, the governments select Supervision. Otherwise, they will choose the other option.
(2) The governments choose Supervision when the public reputation is greater than the sum of the basic cost and the subsidy paid to the recycling companies. Otherwise, the governments will select the “Non-supervision” strategy.
(3) When the impact of the recycling companies’ behavior on citizens creates less hidden benefits for themselves than the subsidy given to citizens, the recycling companies choose Incompliance. Otherwise, the recycling company will choose the other strategy.
(4) When the time citizens spent on garbage classification is more than the subsidies they receive from recycling companies and the environmental benefits, citizens will select “Participation,” or they will choose to implement garbage classification.
(5) When recycling companies and citizens both choose the active strategy, only when the governments also choose to supervise can a stable game state be achieved, i.e., all parties in the game achieve their respective maximum utility.
According to the properties of the ESS and the stability theorem of differential equations, ESS points are robust to small perturbations (Jiang et al., 2019). More precisely, when
Proposition 1.
For governments,
(1) When
(2) When
However, the stability strategy choice of governments needs further analysis. To obtain an evolutionary stability strategy, the condition
Proof.
(1) When
(2) When
a: When
b: When
Then, the incremental stability of recycling companies’ behavior strategy is analyzed.
Proposition 2.
As for recycling companies,
(1) When
(2) When
These two solutions are stability states of
Proof.
(1) When
(2) When
a: When
b: When
Finally, the stability of citizens’ behavior strategy is analyzed, and the following propositions are proposed.
Proposition 3.
As for citizens,
(1) When
(2) When
However, the stability strategy choice of citizens needs further analysis. To obtain an evolutionary stability strategy, the condition
Proof.
(1) If
(2) If
a: When
b: When
In the evolutionary process, the probability
Based on the derivative property of the stable point, the aforementioned three-party replication dynamics equation of each stakeholder can be rewritten as
The model diagram of the system dynamics of a three-party evolutionary game is established, as shown in Figure 2:
4 Simulation of the tripartite evolutionary game
In this section, we simulate the tripartite evolutionary game model through numerical simulation to obtain the evolutionary trend of each stakeholder’s behavior under the current situation. Zhejiang Province is one of the first provinces to carry out waste separation in China. With rich experience and a relatively complete waste separation system, Zhejiang is quite worthy of being learned from. Therefore, some of the data required for the simulation in this paper are selected from Zhejiang Province. The parametric data are collected from news reports and previous research findings related to this field. In order to fit the actual situation as closely as possible, the parameter values are strictly proportional. The evolution and stabilization path of the three-party game model under practical conditions is then analyzed through simulations via MATLAB. The specific source of each parameter is shown in Supplementary Appendix D. Based on this, we can set the initial values of the parameters involved in the model as
4.1 Evolutionary path
Since the initial probabilities of the three individuals choosing Supervision (
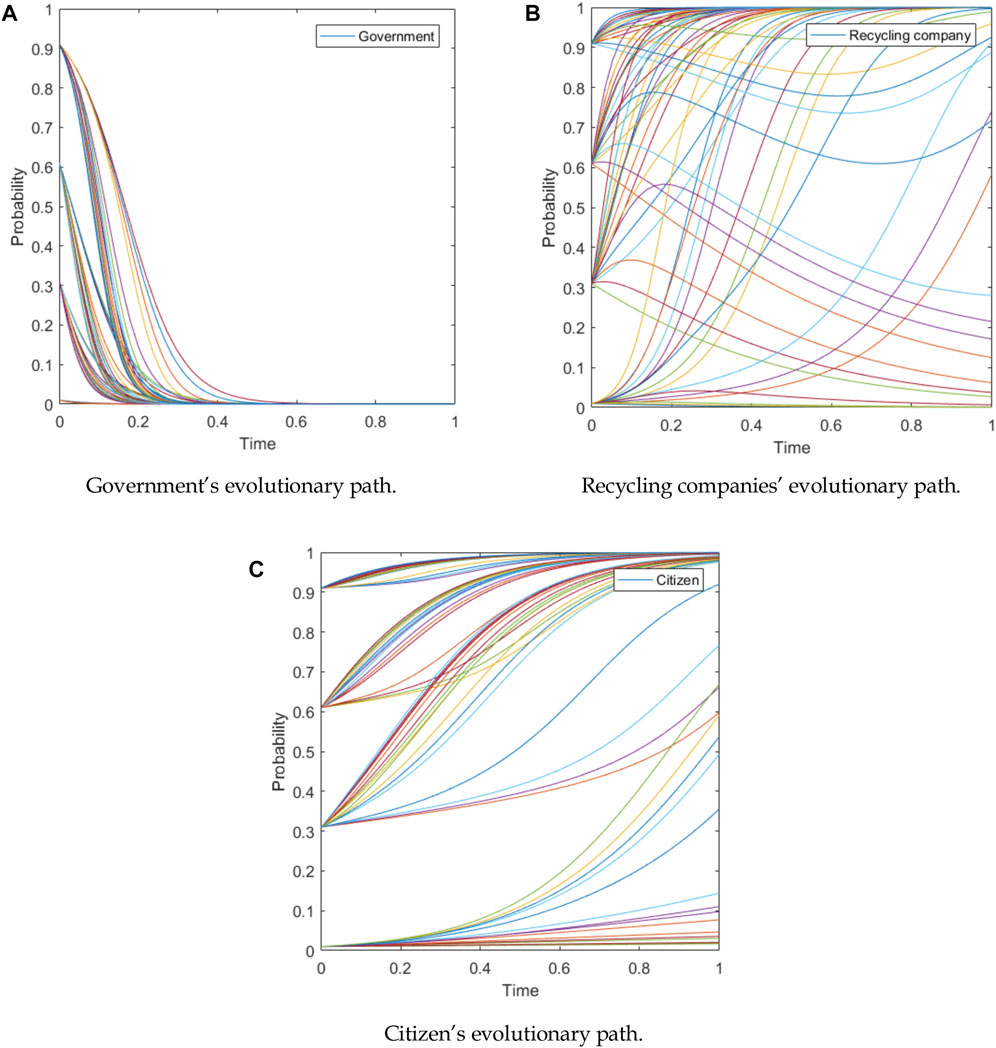
FIGURE 3. Equilibrium evolution with different initial probabilities. (A) Government’s evolutionary path. (B) Recycling companies’ evolutionary path. (C) Citizen’s evolutionary path.
According to the aforementioned simulation results, we find that different initial probabilities will affect the evolution of equilibrium. Considering the actual situation, the government does not strictly supervise the behavior of both recycling companies and citizens; the cost of garbage classification is much higher than that of non-intervention; and furthermore, citizens will choose the corresponding resistance strategy in consideration of their own interest. In order to better simulate the actual situation, this paper sets the initial selection probability of the governments, recycling companies, and citizens as 0.8, 0.6, and 0.3, respectively, specifically because this is in line with the current situation of government supervision to obtain environmental benefits. However, recycling companies do not actively implement garbage classification due to the high operating costs and more high-quality staff required for the refined process of garbage collecting and processing. On the other hand, citizens tend to not implement garbage classification because they cannot receive timely subsidies and welfare equivalent to the time and cost consumed by garbage classification.
4.1.1 Equilibrium evolution under (0,1,1)
From the aforementioned analysis, only if the conditions
4.1.2 Equilibrium evolution under (0,0,1)
Similarly, only when
4.1.3 Equilibrium evolution under (1,1,1)
Simulation is carried out in the case of (1,1,1), i.e.,
The aforementioned three scenarios are a state of equilibria that can be achieved by the governments, recycling companies, and citizens when they choose the appropriate combination of strategies. As stakeholders in an evolutionary game system, each party will continuously adjust their own strategy in different situations by taking the strategies of the other two parties into consideration until their own interests are maximized.
4.2 Sensitivity analysis
In this subsection, we choose governance cost, recycling companies’ cost, and subsidies citizens receive from recycling companies as the main parameters of sensitivity analysis.
4.2.1 Sensitivity analysis of governance cost
From the perspective of a subjective initiative, we believe that when all stakeholders actively participate in the garbage classification work, an optimal balance is reached, i.e.,
Under this premise, combined with the analysis of the replication dynamic equation, we find that the government’s behavior decisions will be directly affected by the government’s regulatory cost
As seen from Fig. 10, we find that as the cost of government regulation increases, the evolutionary game system will reach equilibrium at an increasingly slower rate. When the amount reaches a certain threshold, it shifts to a different equilibrium state (0,1,1), where the government chooses not to regulate. This suggests that the greater the cost of regulation, the less aggressive the government will be in regulating garbage classification. When the cost required for regulating the action of implementing garbage classification reaches a certain amount, the government will no longer choose to regulate. Figure 11 shows that the optimal cost of regulation should be between 18.5 and 25.5. Reducing the cost of regulation too much could make it less effective than it should be, and investing too much money in regulation might lead to a waste of funds and strain the state’s finances.
4.2.2 Sensitivity analysis of recycling companies’ cost
Similarly, in order to analyze the sensitivity of recycling companies’ cost, the simulation is performed under the “best equilibrium steady state,” i.e.,
As shown in Figure 8, we find the rate at which the evolutionary game converges to a steady state gradually as the recycling companies’ cost of implementing garbage classification decreases. As profit-oriented companies, recycling companies are more likely to implement aggressive strategies when the cost of intervention is smaller. On the other hand, when recycling companies spend a certain small amount of money on implementing garbage classification, the subsidies they give to citizens will increase accordingly, making the citizens more motivated to sort their waste and thus speeding up the model toward equilibrium.
4.2.3 Sensitivity analysis of subsidies citizens receive from recycling companies
Furthermore, under the best equilibrium steady state, this paper discusses the sensitivity of subsidies that citizens receive from recycling companies. In the game model, all the parameters remain unchanged, except that
As can be seen in Figure 15, the higher the welfare given to citizens, the faster the evolutionary game model reaches equilibrium. This indicates that the welfare package will stimulate citizens to implement garbage classification, making them more willing to cooperate with the recycling companies, which, in the long run, will also significantly reduce the workload and burden of the recycling companies, and in turn, increase their motivation and efficiency. In this way, both parties are actively involved in garbage classification. Therefore, the pressure on the government to regulate will be reduced.
5 Conclusion
In order to curb the further waste of recyclable and land resources, and thus reduce the environment pollution caused by garbage, garbage classification is gaining increasing attention as well as the public recognition of the numerous environmental benefits that it brings. However, the implementation of garbage classification has not achieved the expected results due to the government’s inappropriate management policies and the lack of integration with the recycling companies and citizens, resulting in the continued waste of resources and the increasing financial burden on the governments. Therefore, in order to find the best strategy to maximize the benefits of the governments, recycling companies, and citizens, this paper develops a tripartite evolutionary game model based on the aforementioned three stakeholders. The results of our study are as follows:
(1) Environmental benefits can be maximized when government supervision, recycling companies, and citizens are actively engaged in garbage classification. However, recycling companies do not actively implement garbage classification due to the high operating costs and more high-quality staff required for the refined process of garbage collecting and processing. On the other hand, citizens tend to not implement garbage classification because they cannot receive timely subsidies and welfare equivalent to the time and cost consumed by garbage classification.
(2) The government’s regulation is necessary to promote the implementation of garbage classification. The government’s subsidies for recycling companies will have a significant positive impact on the cooperation of recycling companies in the implementation of garbage classification. In other words, the government’s attitude directly influences whether recycling companies are willing to take the active strategy. Therefore, to achieve the ideal effect, the government, first of all, needs to keep its basic regulatory costs within a certain range, which is considered to range from 18.5 to 25.5. Specifically, the government can improve the utilization of funds by developing detailed systems and methods of financial fund management and assessment of rewards and penalties. In addition, internal structures can be overhauled to reduce and eliminate waste, such as overly lenient office expenses, lack of restraint in hospitality, and other non-regular official expenses.
(3) Recycling companies can maximize the benefits of the three parties by controlling their own costs within a reasonable range with reference to their own situations such as strengthening the company’s appraisal system to improve the professionalism and efficiency of employees or adopting quality operational facilities to reduce the number of cost increases caused by depreciation or replacement of equipment. This will be quite important because only if the recycling companies reduce their own operating costs as much as possible in the premise of compromising the effectiveness of implementation of garbage classification will it be possible to increase the benefits distributed to citizens, which will have a direct impact on the choices and attitudes of citizens.
The basic research in our study can be extended to other fields. The model in the paper has cross-domain extension, and it is applicable to most evolutionary game models with two or three game players. Although this paper focuses on a tripartite evolutionary game among the governments, recycling companies, and citizens, there are still some limitations. First, we assume that the information involved in the whole game system is completely open and transparent. One party can be informed in time whether others choose to implement garbage classification. Likewise, the other two parties can be informed in time whether the government chooses to regulate or not. In addition, the suggestions given to the game players in this paper are biased toward the general direction, and the specific scope is still vague. Finally, the whole process of the implementation of garbage classification involves many other stakeholders, which are not considered in this paper. The aforementioned point can be used as a direction for future research (Zheng and Ma, 2022; Zhou et al., 2022).
Data availability statement
The original contributions presented in the study are included in the article/Supplementary Material; further inquiries can be directed to the corresponding author.
Author contributions
LX: writing and calculating. HT: investigating. LnL: data interpretation and analysis. LyL: funding. All authors contributed to the article and approved the submitted version.
Acknowledgments
The authors acknowledge the University of Toronto, York University, for providing the laboratory facilities. The authors acknowledge the Shanghai Philosophy and Social Science Planning Project for providing fund support.
Conflict of interest
The authors declare that the research was conducted in the absence of any commercial or financial relationships that could be construed as a potential conflict of interest.
Publisher’s note
All claims expressed in this article are solely those of the authors and do not necessarily represent those of their affiliated organizations, or those of the publisher, the editors, and the reviewers. Any product that may be evaluated in this article, or claim that may be made by its manufacturer, is not guaranteed or endorsed by the publisher.
Supplementary material
The Supplementary Material for this article can be found online at: https://www.frontiersin.org/articles/10.3389/fenvs.2023.1185932/full#supplementary-material
References
Adedeji, O., and Wang, Z. (2019). Intelligent waste classification system using deep learning convolutional neural network. Procedia Manuf. 35, 607–612.
Bond, A., Pope, J., and Morrison-Saunders, A. (2016). A game theory perspective on environmental assessment: What games are played and what does this tell us about decision making rationality and legitimacy? Environ. Impact Assess. 57, 187–194.
Callan, S. J., and Thomas, J. M. (2006). Analyzing demand for disposal and recycling services: A systems approach. East. Econ. J. 32, 221–240.
Chen, J., Xiong, W., and Xu, L. (2021). Evolutionary game analysis on supply side of the implement shore-to-ship electricity. Ocean Coast. Manag. 215, 105926.
Chen, W., and Hu, Z. H. (2018). Using evolutionary game theory to study governments and manufacturers’ behavioral strategies under various carbon taxes and subsidies. J. Clean. Prod. 201, 123–141.
Chen, Z., Yang, J., Chen, L., et al. (2022). Garbage classification system based on improved ShuffleNet v2[J]. Resour. Conservation Recycl. 178, 106090.
Cui, T., Su, X., and Zhang, Y. (2021). Study on compulsory classification management and behavior synergy of municipal solid waste. Sustainability 13 (11), 6265.
Dong, L., Fujita, T., and Dai, M. (2016). Towards preventative eco-industrial development: An industrial and urban symbiosis case in one typical industrial city in China. J. Clean. Prod. 114, 387–400.
Dong, L., Fujita, T., and Zhang, H. (2013). Promoting low-carbon city through industrial symbiosis: A case in China by applying HPIMO model. Energy Policy 61, 864–873.
Friedman, D., Econometrica, S., and May, N. (1991). Evolutionary games in economics published by: The econometric society. Econometrica 59, 637–666.
Fullerton, D., and Kinnaman, T. C. (1996). Household responses to pricing garbage by the bag. Univ. Tex. A. T. Austin Cent. Appl. Res. Econ. 86, 971–984.
Gan, B., and Zhang, C. “Research on the algorithm of urban waste classification and recycling based on deep learning technology,” in Proceedings of the International Conference on Computer Vision, Image and Deep Learning (CVIDL), Chongqing, China, July 2020, 232–236.
Hua, Y., Dong, F., and Goodman, J. (2021). How to leverage the role of social capital in pro-environmental behavior: A case study of residents’ express waste recycling behavior in China. J. Clean. Prod. 280 (8), 124376.
Huang, G., He, J., and Xu, Z. (2020). A combination model based on transfer learning for waste classification. Concurrency Comput. Pract. Exp. 32 (19), 1–12.
Iyer, E. S., and Kashyap, R. K. (2007). Consumer recycling: Role of incentives, information, and social class. J. Consumer Behav. 6, 32–47.
Jiang, K., You, D., and Merrill, R. (2019). Implementation of a multi-agent environmental regulation strategy under Chinese fiscal decentralization: An evolutionary game theoretical approach. J. Clean. Prod. 214, 902–915.
Kang, Z., Yang, J., and Li, G. (2020). An automatic garbage classification system based on deep learning. IEEE Access 99, 1.
Kuang, Y., and Lin, B. (2021). Public participation and city sustainability: Evidence from urban garbage classification in China. Sustain. Cities Soc. 67 (6), 102741.
Linderhof, V., Kooreman, P., and Allers, M. (2001). Weight-based pricing in the collection of household waste: The oostzaan case. Resour. Energy Econ. 23, 359–371.
Liu, J., and Teng, Y. (2023). Evolution game analysis on behavioral strategies of multiple stakeholders in construction waste resource industry chain. Environ. Sci. Pollut. Res. 30, 19030–19046.
Liu, J., Yi, Y., and Li, C. (2021). A model for analyzing compensation for the treatment costs of construction waste. Sustain. Energy Technol. Assessments 46, 101214.
Liu, Q., Xu, Q., and Shen, X. (2022). The mechanism of household waste sorting behaviour-A study of jiaxing, China. Int. J. Environ. Res. Public Health 19 (4), 2447.
Liu, X., Wang, Z., and Li, W. (2019). Mechanisms of public education influencing waste classification willingness of urban residents. Resour. Conserv. Recycl. 149, 381–390.
Long, R., Yang, J., and Chen, H. (2019). Co-evolutionary simulation study of multiple stakeholders in the take-out waste recycling industry chain. J. Environ. Manag. 231, 701–713.
Meng, X., Wen, Z., and Qian, Y. (2015). Evaluation of cleaner production technology integration for the Chinese herbal medicine industry using carbon flow analysis. J. Clean. Prod. 163, 310–319.
Mo, H., Wen, Z., and Chen, J. (2009). China’s recyclable resources recycling system and policy: A case study in suzhou. Resour. Conservation Recycl. 53 (7), 409–419.
Narayan, Y. “DeepWaste: Applying deep learning to waste classification for a sustainable planet,” in Proceedings of the NeurIPS 2020 Workshop on Tackling Climate Change with Machine Learning, Virtual-only Conference, December 2021.
National Bureau of Statistics, (2020). National data. https://data.stats.gov.cn/(Accessed February 5, 2021).
Peng, H., Shen, N., and Ying, H. (2021). Factor analysis and policy simulation of domestic waste classification behavior based on a multiagent study—taking shanghai's garbage classification as an example. Environ. Impact Assess. Rev. 89, 106598.
Tang, D., Shi, L., and Huang, X. (2022). Influencing factors on the household-waste-classification behavior of urban residents: A case study in Shanghai. Int. J. Environ. Res. Public Health 19 (11), 6528.
Teng, Y., Lin, P. W., and Chen, X. L. (2022). An analysis of the behavioral decisions of governments, village collectives, and farmers under rural waste sorting. Environ. Impact Assess. Rev. 95, 106780.
Tong, X., and Tao, D. (2016). The rise and fall of a waste city in the construction of an urban circular economic system: The changing landscape of waste in beijing. Resour. Conservation Recycl. 107, 10–17.
Wadehra, S., and Mishra, A. (2018). Encouraging urban households to segregate the waste they generate: Insights from a field experiment in Delhi, India. Resour. Conservation Recycl. 134, 239–247.
Wang, H., Gui, H., and Ren, C. (2021). Factors influencing urban residents’ intention of garbage sorting in China: An extended TPB by integrating expectancy theory and norm activation model. Sustainability 13 (23), 12985.
Wang, H. “Garbage recognition and classification system based on convolutional neural network vgg16,” in Proceedings of the 3rd International Conference on Advanced Electronic Materials Computers and Software Engineering (AEMCSE), Shenzhen, China, April 2020, 252–255.
Wang, S., Wang, J., and Zhao, S. (2019). Information publicity and resident’s waste separation behavior: An empirical study based on the norm activation model. Waste Manag. 87, 33–42.
Wei, J. (2015). Research on overall governance on living garbage under the guidance of the urban and rural integration development. China Popul. Resour. Environ. 25, 171–176.
Wertz, K. L. (1976). Economic factors influencing households’ production of refuse. J. Environ. Economics& Manag. 2 (4), 263–272.
Xiao, S., Dong, H., and Geng, Y. (2020). Policy impacts on municipal solid waste management in Shanghai: A system dynamics model analysis. J. Clean. Prod. 262, 121366.
Xu, L., Di, Z., and Chen, J. (2021). Evolutionary game of inland shipping pollution control under government co-supervision. Mar. Pollut. Bull. 171, 112730.
Zhang, H., Liu, J., and Wen, Z. (2017). College students’ municipal solid waste source separation behavior and its influential factors: A case study in beijing, China. J. Clean. Prod. 164, 444–454.
Zhao, L., and Chen, H. (2021). Exploring the effect of family life and neighbourhood on the willingness of household waste sorting. Sustainability 13 (24), 13653.
Zheng, H., and Ma, W. (2022). Impact of ICT adoption on cosmetic expenditure of Chinese residents: An exploration accounting for urban-rural differentials. Appl. Econ. Lett. 29 (14), 1286–1291.
Zhou, Y., Xu, Y., and He, H. (2022). System dynamics simulation of the regulatory evolution game of residential waste separation behavior. J. Syst. Sci. 30 (2), 6.
Keywords: city governance, dynamic equation, evolutionary game, garbage classification recycling, environmental psychology
Citation: Xu L, Tang H, Liu L and Liu L (2023) An equilibrium analysis on the tripartite evolutionary game of garbage classification recycling. Front. Environ. Sci. 11:1185932. doi: 10.3389/fenvs.2023.1185932
Received: 28 March 2023; Accepted: 23 May 2023;
Published: 07 June 2023.
Edited by:
Shigeyuki Hamori, Kobe University, JapanReviewed by:
Jingkuang Liu, Guangzhou University, ChinaHanliang Fu, Xi'an University of Architecture and Technology, China
Copyright © 2023 Xu, Tang, Liu and Liu. This is an open-access article distributed under the terms of the Creative Commons Attribution License (CC BY). The use, distribution or reproduction in other forums is permitted, provided the original author(s) and the copyright owner(s) are credited and that the original publication in this journal is cited, in accordance with accepted academic practice. No use, distribution or reproduction is permitted which does not comply with these terms.
*Correspondence: Lin Liu, earnestliu@126.com; Liying Liu, llyhys@163.com