- 1CMA Cloud-Precipitation Physics and Weather Modification Key Laboratory, Beijing, China
- 2Weather Modification Centre, Beijing, China
- 3State Key Laboratory of Atmospheric Boundary Layer Physics and Atmospheric Chemistry, Institute of Atmospheric Physics, Chinese Academy of Sciences, Beijing, China
- 4Atmospheric Science and Global Change Division, Pacific Northwest National Laboratory, Richland, WA, United States
Aerosols affect development of clouds and precipitation by serving as cloud condensation nuclei (CCN) and ice nuclei (IN). Considering the dramatically changing ambient aerosol concentration, it is important to examine the potential “side effect” of aerosol pollution on precipitation enhancement by weather modification. In this study, the cloud seeding was performed on a precipitation event in Beijing in the summer of 2008, which is simulated by the NSSL two-moment cloud scheme of the Weather Research and Forecasting (WRF) model. Sensitivity tests were conducted by modifying the ambient aerosol concentration and the ice crystal seeding amount to investigate the cloud seeding efficacy in different CCN concentration scenarios. There was a slight difference in the precipitation distribution between the simulations with two ambient CCN concentrations: the northern precipitation center in polluted scenario was weaker and the southern center was stronger. Compared with normal CCN scenario, the cloud liquid water mass and ice crystal mass in the severe pollution scenario is larger, and the total contents of snow and graupel were not sensitive to the CCN concentration. With the same amount of man-made ice crystals seeding, the precipitation enhancement was quite different under different CCN conditions. The higher the CCN concentration usually leads to stronger precipitation suppression. As CCN concentration increase, the deposition growth of snow, auto-conversion and accretion of ice crystals to snow were weakened, as well as the conversion of melting snow and graupel into rainwater.
1 Introduction
It is well known that aerosols affect the cloud optical properties, the macrophysical and microphysical characteristics of clouds, and precipitation, by serving as cloud condensation nuclei (CCN) and ice nuclei (IN) (Levin et al., 1996; Xiao and Yin, 2011; Yang et al., 2011). Studies on the effect of aerosols on clouds and precipitation have been highly focused, and many papers have been published on this topic (Teller and Levin, 2006; Zhang et al., 2013; Fan et al., 2016; Zhou et al., 2020). The air pollution events increase aerosol particles that can be activated to become CCN, resulting in the formation of many small cloud particles in the non-precipitation clouds. Higher CCN concentrations tend to increase cloud number concentration, decrease the cloud droplet size, and narrow the droplet spectrum (Warner and Twomey, 1967; Pruppacher and Klett, 1997), which suppresses the precipitation formation (Rosenfeld, 2000; Li, et al., 2014) and extends the cloud lifetime. The collision efficiency of cloud droplet particles with a radius of 10 μm was 10%, and when the droplet size was 30 μm, the efficiency was greatly increased to 80% (Pinsky et al., 2000), indicating significantly reduced precipitation formation by aerosol pollution (Khain et al., 2005). The increase of aerosol concentration can alter ice particle riming efficiencies (Hindman et al., 1994; Borys et al., 2003), suggesting that aerosol-related effects are not limited to warm-cloud processes. For example, increased sulfate-based aerosol concentrations suppress the formation of larger cloud droplets and reduce the riming of cloud droplets by ice hydrometeors (Borys et al., 2003). Additionally, Rosenfeld et al. (2001) found that aerosols can inhibit precipitation, while Wurzler et al. (2000) and Yin et al. (2002) found that giant CCNs can increase precipitation by enhancing collision efficiency. There is a high-level uncertainty regarding the precipitation suppression by aerosols (Seifert and Beheng, 2006; Yue, et al., 2011). Givati and Rosenfeld (2004) noted that urban pollution could reduce annual precipitation in California by 15%–25%, and Rosenfeld et al. (2007) noted that precipitation on Mount Hua was reduced by 30%–50% in hazy weather conditions.
The precipitation suppression by aerosols also depends on local effects. Aerosols may enhance convective precipitation in the south and inhibit stratus precipitation in the north (Shi, et al., 2015). Fan et al. (2016) found that urbanization in Houston (i.e., the joint effect of both urban land and anthropogenic aerosols) notably enhanced the storm intensity by 75% in maximum vertical velocity and precipitation intensity by up to 45%. Previous studies have also shown that aerosols can affect precipitation from hail clouds and warm clouds and increase precipitation from robust convective clouds (Li et al., 2021). Continental aerosols were shown to increase convection largely at the tropical cyclone periphery, which led to its weakening prior to landfall (Khain et al., 2010).
The weather modification modifies the cloud and precipitation processes through cloud seeding with hygroscopic particles, silver iodide or coolant seeding agents, aiming at to enhance the precipitation (Cotton, 1986; Bruintjes, 1999; Rosenfeld, et al., 2010; Zheng and Guo, 2012). The ambient aerosols can affect the development of clouds and precipitation by acting as CCN and IN, which in turn affects the effects of cloud seeding (Rangno and Hobbs, 1995; Fang, et al., 2022). It has also been found that inappropriate cloud seeding slows down the precipitating particle generation (Xiao, et al., 2012; Chen, et al., 2020), and the specific outcome of cloud seeding will presumably also depend on the properties of the ambient aerosol as well as on the turbulence characteristics of the cloud. Namely, the aerosol-related effects on the precipitation processes may lead to unintentional weather modifications, especially in the case of increasing atmospheric pollution.
Most cloud microphysics schemes in the current numerical weather forecast models neglect the prognostic ambient aerosol concentration when calculating CCN and IN activation rates. Additionally, the current cloud microphysics schemes struggle to accurately represent precipitating particle size distribution (Shan et al., 2014; 2021; 2022), such as the incapability of the gamma function to represent the wide and truncated raindrop size distributions (Shan et al., 2020), and the underestimated solid-phase particle sedimentation and rimming rates (Lin et al., 2021; 2023). Thus, such a deficiency limits their application for cloud-seeding studies related to the interaction between the ambient aerosols and seeding aerosols (Flossmann, et al., 2019). Benefiting from the well-developed double-moment cloud microphysics scheme by National Severe Storms Laboratory (NSSL), physical processes related to the cloud development and precipitation generation are included in the mesoscale atmospheric model [e.g., Weather Research and Forecast (WRF)], as well as the treatments of prognostic aerosols. The objective of this study is to examine weather ambient CCNs counteract seeding agents of IN, especially under high-aerosol conditions. If so, how much seeding effect will be offset?
In this paper, to perform a rain enhancement seeding simulation, artificial ice crystal seeding was added to the NSSL double-moment cloud scheme in the WRF. This study was carried out on a precipitation event in Beijing in the summer of 2008. Sensitivity simulations were conducted by modifying the aerosol CCN concentration and seeding amounts to study the seeding effects under normal and severely polluted aerosol conditions. The remainder of this article is organized as follows. Section 2 presents model setups and describes the precipitation event used in this study. Section 3 exhibits the responses of clouds and precipitation to aerosol influences. Section 4 discusses influences of ambient aerosols on cloud seeding results. Section 5 summarizes the conclusions and further considerations.
2 Model setups and case description
2.1 Description of experimental setups
In this study, the WRF V3.6 model with the NSSL cloud microphysics scheme with prognostic aerosol processes was used (Mansell, et al., 2010). The NSSL scheme predicts number concentrations and mass mixing ratios of cloud droplets, rain drops, ice crystals, snow, graupel, and hail. The NSSL scheme is a relatively reasonable scheme of a heavy precipitation event, since it includes the graupel category [, e.g., high-density frozen drops (includes small hail) and low-density graupel (from rimed ice crystals/snow] that are usully neglected by cloud microphysics schemes. Furthermore, CCN concentration is predicted with a bulk activation spectrum approximating small aerosols. The NSSL scheme matches the objective of this study related to aerosol effects on cloud physics and cloud seeding of summer precipitation case. It calculates the cloud drop number increase (
where
Other physics schemes include the RRTM longwave radiation scheme (Mlawer, et al., 1997), Dudhia shortwave radiation scheme (Dudhia, 1989), Noah land surface process scheme (Tewari, et al., 2004), and YSU boundary layer scheme (Hong, et al., 2005). The WRF model is run on two nested domains with grid spacings of 27 and 9 km and mesh sizes of 135 × 146 and 178 × 163, respectively. The coarse domain covers the most part of east China, latitude from 21 N to 54 N, longitude from 90 E to 135 E, which guarantees that our simulation covers the whole life of the precipitation system. Both domains use 35 unevenly spaced vertical computational levels. The initial and lateral boundary conditions are from the NCEP/DOE reanalysis data. The simulation period is from 0200 UTC on 16 June 2008 to 0800 UTC on 17 June 2008, for a total of 30 h.
2.2 Case description
On 16 June 2008, due to the weak cold air in front of the high-altitude trough and southern warm air flow, there was a thunderstorm event with strong precipitation occurered in the Beijing area. From the 500 hPa geopotential height field at 08:00 on June 16 (Figure 1A), the trough of low pressure in the Hetao area affected Beijing, Beijing was at the front of this trough, and the high-altitude trough moved from west to east and brought strong precipitation.
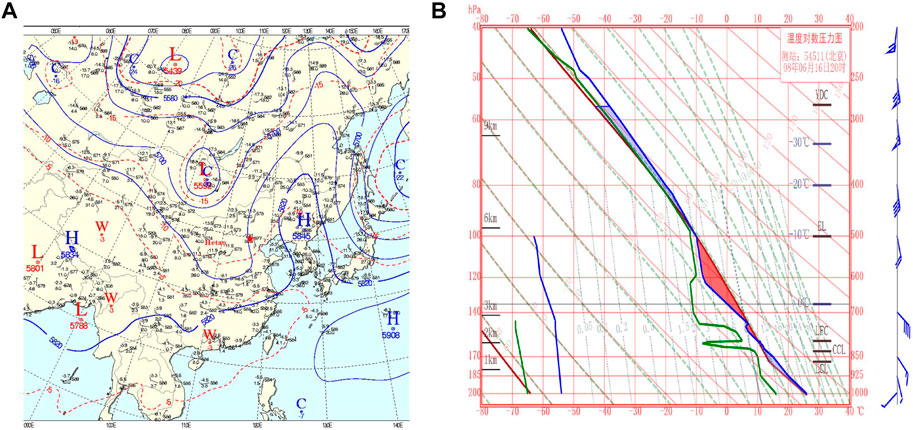
FIGURE 1. Observation of the 500 hPa geopotential height field (A) and T log-P diagram (B) at 08:00 on June16,2008. In (A), 500 hPa geopotential height field in blue line (unit: dagpm), temperature in red line (unit:°C) and wind field in green vector (unit: m s-1). Red star denotes Beijing.
According to sounding measurements in Beijing at 08:00 on the 16th, the dew point difference of the temperature below the 650 hPa level was significantly lower than that above 650 hPa (Figure 1B). The humidity in the lower atmosphere was much higher than that in the upper atmosphere, and the atmospheric layer was potentially unstable. At 20:00, the ground temperature rose by approximately 5 °C, reaching 26 °C, and the wind direction at the lower level changed from a clockwise to a counterclockwise direction. The CAPE value of the middle-level layer was relatively large, and there was convective effective potential energy, which promoted the development of convection and precipitation.
3 Sensitivity experiments of aerosol influences on clouds and precipitation
The effect of aerosols on the physical processes of clouds and precipitation was simulated by two experiments with different ambient CCN concentrations. According to the airborne CCN observation, the CCN varies greatly. The measurements in Hebei province (south to Beijing city) during summer of 2005 exhibit the lowest CCN concentrations as 584 cm-3, 808 cm-3 and 2,431 cm-3 at super-saturations of 0.1%, 0.3% and 0.5%, and the largest values are 9,495 cm-3, 16,332 cm-3 and 21,812 cm-3, respectively (Shi and Duan, 2007). The CCN concentration around Beijing city is larger than 1,500 cm-3 in most observations, and in pollution days CCN concentration can reach 6,000 cm-3 (Chen et al., 2015). So, this study sets 1,500 cm-3 as the baseline value around Beijing city to represent the benchmark condition, and 8,000 cm-3 is used to represent the severe pollution condition. Two experiments were designed: one with a CCN concentration of 1,500 cm-3, and the other with a CCN concentration of 8,000 cm-3 (Table 1).
3.1 Aerosol effects on precipitation
Experiments with these two CCN concentrations are carried out with the same initial input data and model configuration. The daily precipitation distributions of the two experiments are shown in Figure 2. The CCN sensitivity simulations show that there is no significant change in the precipitation intensity magnitudes when the concentration of aerosols changes but the locations of the precipitation centers change.
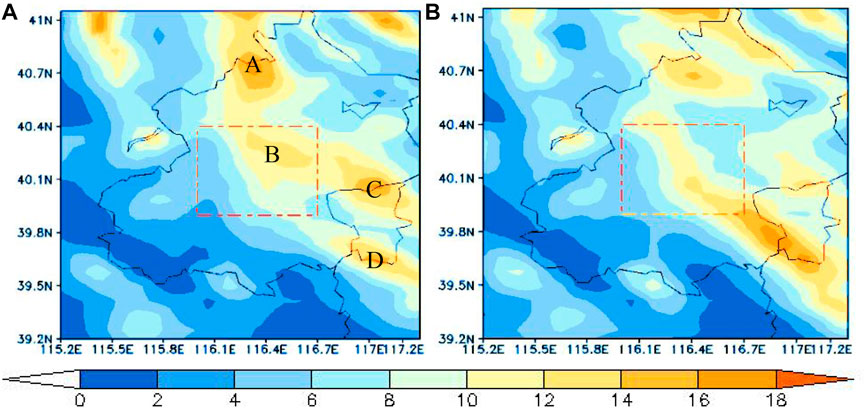
FIGURE 2. The 24 h precipitation (unit: mm) at 08:00 on 17 June 2008. (A): CCN1500, (B): CCN8000, the red rectangle is the seeding area in the next section.
There are four main rainfall centers in the CCN1500 experiment, A, B, C and D in Beijing district and its nearby, from north to south, and their 24 h precipitation amounts range from 12 mm to 16 mm. Compared to that of CCN1500, the northern precipitation centers in the CCN8000 are weaker, the rainfall amounts in centers A, B and C decrease by as much as 4 mm (29%), which are 14 mm or 16 mm in the CCN1500 test, and the southern center is stronger, with 13% more precipitation in the southern center D.
3.2 Aerosol influences on cloud condensates
The daily rainfall rate differences of these two simulations are caused by the cloud condensates evolution differences which are related to the ambient CCN concentration change. Figure 3 shows the horizontal distribution of the cloud-water mass content (qc), updraft and horizontal wind field under two CCN concentrations at the 650 hPa layer at two time points on the 17th, at 01:00 (Figures 3A,C) and 01:40 (Figures 3B,D), when the clouds develop deeply and strong rainfall occurs around Beijing. In general, as the aerosol concentration increases, the cloud water ratio content increases significantly in convective cloud centers, and there are more areas with qc larger than 0.4 g/kg. For north-east centers, the updraft change with CCN is not obvious, but the qc is much less In CCN1500 than that in CCN800. There is slight differences in cloud cover area between two CCN tests. The pattern of the cloud band in these two tests remain the same. At 01:00 on the 17th, the maximum cloud water content of both simulations is approximately 0.4 g/kg, as shown in Figures 3A,C. Forty minutes later, at 01:40, cloud systems continue to move towards northwest, and still keep organized as a northeast‒southwest band, with several high qc centers. The qc centers on the 650 hPa level in the Beijing area merge into one large convective center with rich qc, and it brings precipitation for Beijing.
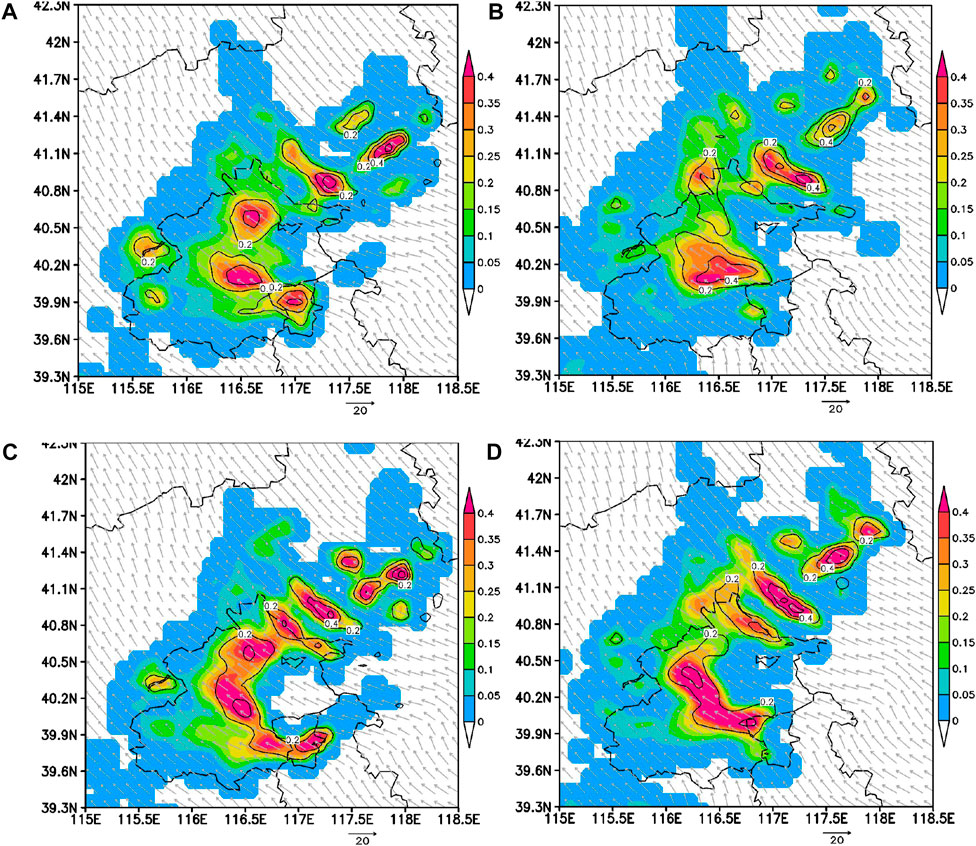
FIGURE 3. Horizontal distributions of the simulated cloud water mass content (shaded, unit: g kg-1), vertical velocity (black line, unit: m s-1) and horizontal wind field (vector) at the 650 hPa level on June 17. (A) at 01:00 for the CCN1500; (B) at 01:40 for CCN1500; (C) at 01:00 for CCN8000; and (D) at 01:40 for CCN8000.
Vertical sections along the 116.5 °E longitude shows the vertical distributions of the cloud condensates simulated by CCN1500 and CCN8000 at 01:00 on the 17th (Figure 4). The temperature at the cloud base is approximately 10 °C, and the 0 °C layer is near 700 hPa. Cloud water is widespread below the 0 °C layer, and the warm cloud water is rich in both simulations. The CCN increase results in larger qc in the CCN8000 (Figure 3B), leading to the cloud layer with qc larger than 0.5 g/kg thicker than that of the CCN1500. The ice crystal mass contents (qi) of both simulations mainly concentrate at 500 hPa and 700 hPa heights with a temperature range between 0 °C and −15°C. In contrast to the qc, qi significantly decreases from 0.08 g/kg in the CCN1500 to 0.04 g/kg in the CCN8000.
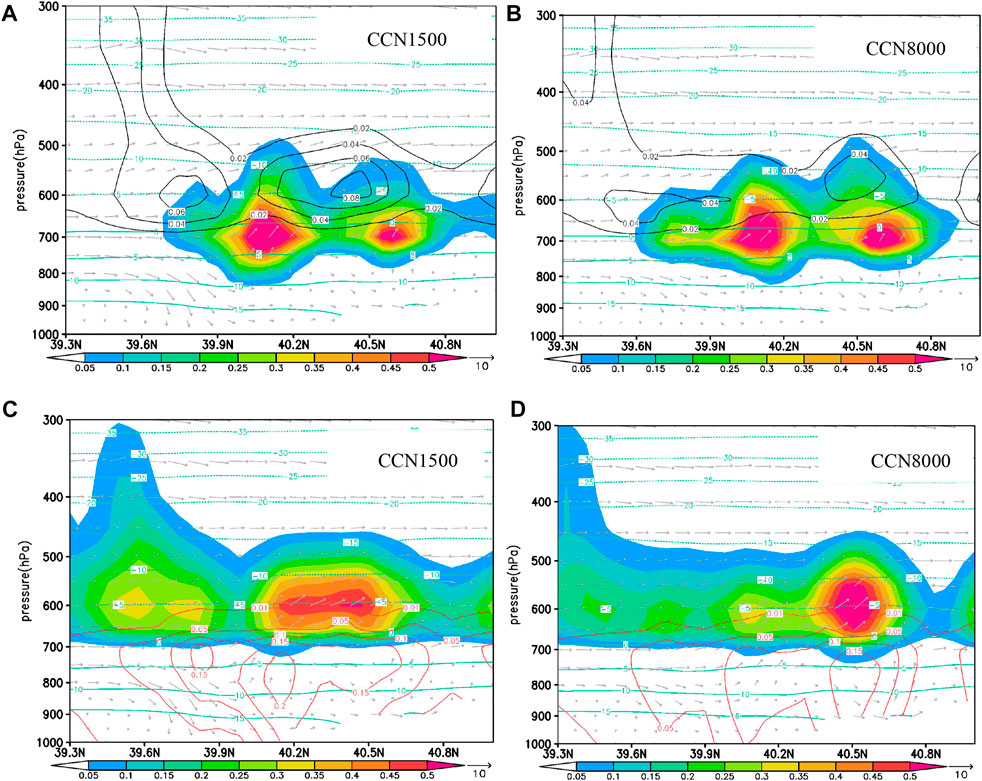
FIGURE 4. Vertical cross sections of hydrometeor mixing ratio of qc, qi, qs and qg, qr along 116.5°E at 01:00 on March 17, where green line: temperature (unit:°C), gray vector: wind field, mixing ratio unit: g/kg.(A) CCN1500, qc (shaded), qi (black line); (B) CCN8000, qc (shaded), qi (black line); (C) CCN1500, qs and qg (shaded), qr (red line); (D) CCN8000, qs and qg (shaded), qr (red line).
For precipitation particles, the CCN influence is not as obvious as that for cloud droplets and ice crystals. The total contents of snow and graupel are mainly distributed between the 500 and 700 hPa layers, with almost no difference in their distribution heights between these two simulations. Excluding a small area in the CCN8000 where the maximum mass content of snow (qs) and graupel (qg) is larger than that in the CCN1500, the summation of the qs and qg with more CCN is smaller than that with less CCN. The rainwater content (qr) in CCN8000 is slightly less than that in the CCN1500.
4 Aerosol influences on cloud seeding results
4.1 Seeding experiment designment
Previous weather modification experiments suggest that the artificial glaciogenic seeding should be carried out in the developing and mature stages of cloud-precipitation system and focus on rich supercooled water areas in cold cloud regime. Liu et al. (2016) compared two cloud seeding methods: 1) directly adding ice crystals and 2) seeding silver iodide particles that nucleate and grow to ice crystals, and concluded that in some cases, the seeding effect of the two methods did not show significant difference. In this research, artificial ice crystal seeding is added to the ice crystals in the NSSL two-moment cloud scheme.
The cloud system of this precipitation event moves cross Beijing in the southeast‒northwest direction, causing precipitation with different intensities along the way. In our simulations, the artificial ice seeding was performed at 01:00 on the 17th in an area with relatively high cloud water contents and low ice crystal concentrations. The seeding area is 39.9 °N - 40.4 °N, 116 °E − 116.7 °E (dashed red box in Figure 3), and the seeding altitude are between layers of 500 hPa and 600 hPa.Tthe research design in this paper includes seeding simulations of 1×104/kg, 1×105/kg and 1×106/kg (as in Table 2) artificial ice crystals to study the seeding effects and water substance responses under a normal atmospheric background and a heavily polluted atmospheric background (see Table 2).
4.2 CCN influence on seeding effects
All seeding experiments show both precipitation increase and the precipitation decrease that are represented by the shaded contours and cyan dotted contours in Figure 5, respectively. As shown by the distribution of the accumulated precipitation changes within the seeding period (80 min), it is obvious that the increases and decreases in precipitation depend on the amounts of seeding ice numbers and ambient CCN concentrations. When the seeding ice crystal number is 104 kg-1, a large area with precipitation enhancement appears in the CCN1500-Se4 and the CCN8000-Se4, and only a very small precipitation suppression area appears whose accumulated precipitation decrease is no more than 0.2 mm. With larger ice crystal seeding amounts, the areas with increased rainfall rates decrease obviously, meanwhile the accumulated precipitation increases, and the maximum increment can reach 0.8 mm in CCN1500-Se6.
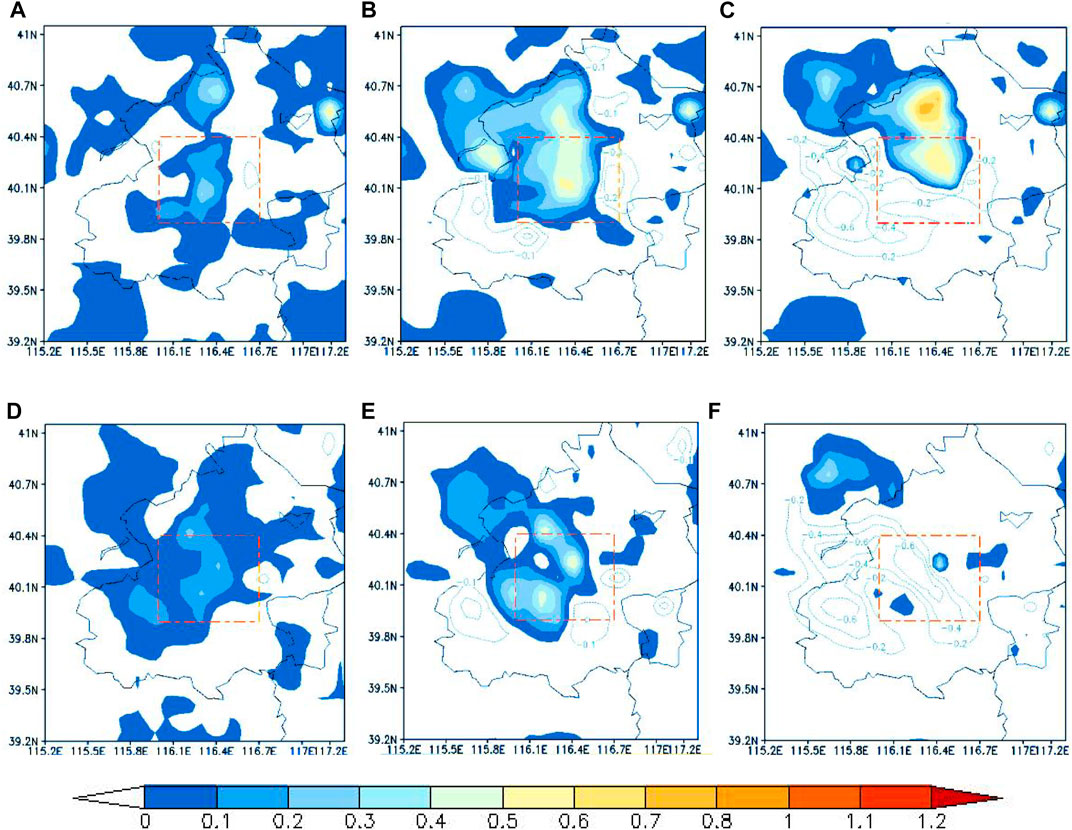
FIGURE 5. Positive changes (shaded areas) and negative changes (cyan dotted contours) in accumulated precipitation between seeding and non-seeding simulations at a time of 02:20, 80 min after seeding (unit: mm). The red rectangle is the seeding area. (A) CCN1500-Se4, (B) CCN1500-Se5, (C) CCN1500-Se6, (D) CCN8000-Se4, (E) CCN8000-Se5, and (F) CCN8000-Se6.
In the severe pollution scenario, the distribution area with precipitation enhancement decreases, especially in the case with the greatest ice crystal seeding amount (i.e., CCN8000-Se6) in which the precipitation enhancement area decreases to less than half of that in the CCN1500-Se6 case. The intensity of precipitation enhancement also significantly decreases to 0.4 mm, which is only half of that of CCN1500-Se6. Moreover, the center of precipitation enhancement moves further downwind.
In addition to the ambient CCN concentrations, ice crystal seeding effects greatly depend on the seeding amount. With a seeding ice number of 1×106 kg-1, the precipitation suppression areas are so large that they almost reach or surpass the precipitation enhancement areas, and the precipitation reduction becomes stronger, especially for CCN8000-Se6, indicating obvious negative seeding results. Therefore, in the simulation with severe pollution, the seeding results in less precipitation enhancement and more precipitation suppression. Namely, the seeding effect changes from enhancement in CCN1500-Se6 to suppression in CCN8000-Se6.
In the simulations with both normal and severe pollution scenarios, the seeding with ice crystal number of 1×106 kg-1 (i.e., CCN1500-Se6 and CCN8000-Se6) almost doubles qi compared with the seeding with 1×105 kg-1. The mass content of ice crystal slightly increases with ambient CCN concentration as shown by the vertical distributions of the regional averaged mass contents of the water condensates from simulations with CCN1500 and CCN8000 and with seeding ice crystal numbers of 1×105 and 1×106 kg-1 (Figure 6).
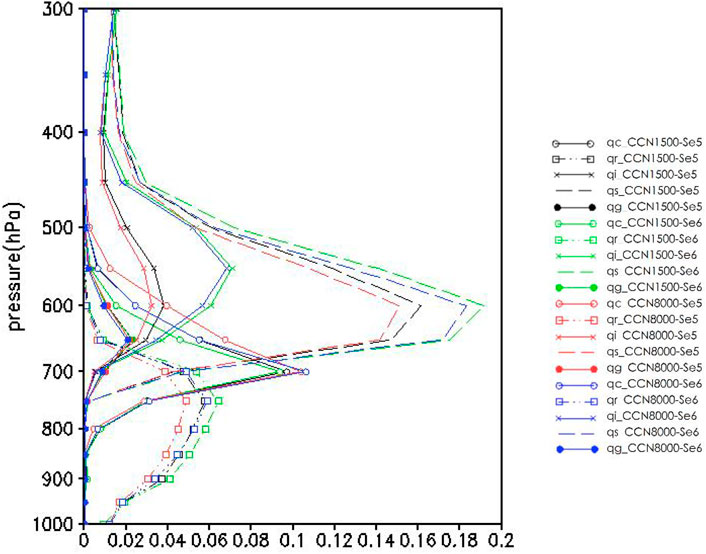
FIGURE 6. Vertical distribution of regional average particles under different aerosol concentrations 80 min after seeding, unit: g/kg, black line: CCN1500-Se5, green line: CCN1500-Se6, red line: CCN8000-Se5, blue line: CCN8000-Se6.
In all simulation tests, the snow mass contents are the largest among all cloud condensates and are even two times of graupel mass contents. The snow mass contents between the 550 hPa and 680 hPa layers obviously increase for all four seeding experiments. With larger seeding amounts, the snow mass content is greater; moreover, with the same seeding amount, the mass content of snow in CCN1500 scenario is larger than that in CCN8000 scenario. The graupel mass content is less sensitive to the ambient CCN concentration and ice crystal seeding amount than the snow mass content. The rainwater mass content has the same distribution pattern as the snow mass. In the case of CCN1500-Se6, there is more rainwater than the other simulations indicating the best seeding effect among all the seeding test simulations.
4.3 Precipitation particle producing rates
The vertical distributions of the main source microphysical processes leading to the formation of ice crystals, rain and snow particles (Table 3) in the main precipitation area (39.2–41 °N, 115.2–117.3 °E) within 40 min of seeding (Figure 7) illustrate that ambient CCNs affect almost all main source microphysical processes of ice crystals, snow and rain and but does not change their height distribution.
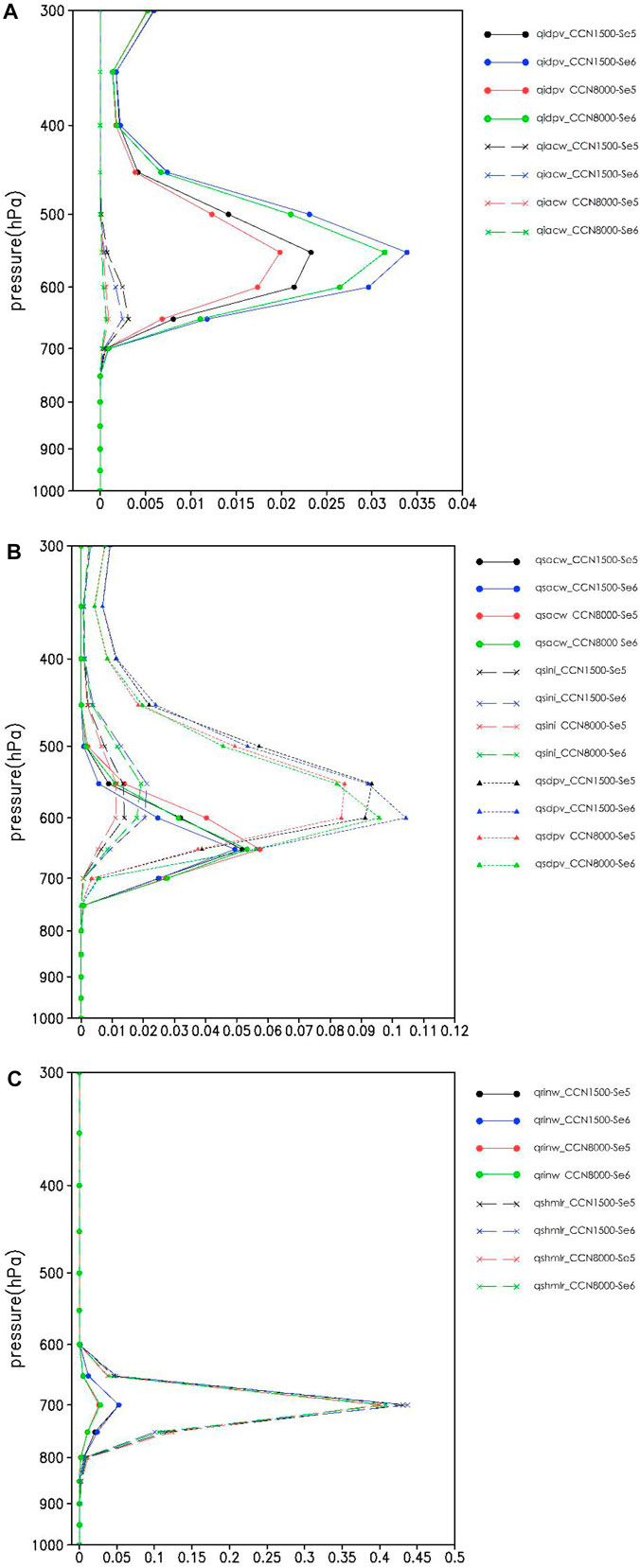
FIGURE 7. The vertical distributions of the main source microphysical process rates of the mass contents of ice (A), snow (B) and rain (C) 40 min after seeding (unit: 10–6 g/kg/s).
In the seeding area, the artificial ice crystals increased the growth of natural ice crystals in the 500–600 hPa layers mainly through the deposition of water vapor, while aggregations of supercooled cloud water only contributes about 10% of the ice mass increase. Higher seeding amounts result in larger ice conversion rates.
In all simulations, the growth of snow is mainly through the water vapor deposition process, followed by the riming of cloud droplets. The auto-conversion and accretion of ice crystals are the lowest growth rates. As there is more cloud water in the CCN8000 tests, the snow particles collect more cloud water than those of the CCN1500 tests. On the other hand, when the aerosol concentration increases, the deposition growth process of ice crystals is inhibited, and the conversion rate of the auto-conversion of ice crystals to snow and the collection process of ice crystals by snow are also reduced.
When the seeding amount is the same but the ambient aerosol concentration increases, the cloud system exhibit larger cloud water contents and smaller cloud drop sizes and the auto-conversion and accretion rates in the seeding cloud decrease slightly (Figure 7C). As the rainwater mainly comes from the melting of snow and graupel particles, and the graupel contents are almost 10% of the snow mass contents, the large amount of melting snow that becomes rain in the CCN1500-Se6 results in the highest rain content and the most obvious precipitation enhancement among all seeding tests.
5 Discussions and conclusion
In this paper, the WRF V3.6 model with the NSSL cloud microphysics scheme considering the prognostic CCN is used to simulate a precipitation event in Beijing on 16 June 2008. Two ambient CCN concentrations of 1,500 #/cm3 and 8,000 #/cm3 are designed to represent normal ambient CCN concentration and severe pollution CCN concentration, respectively. By increasing the ice crystal concentration, man-made ice crystal seeding with different ice amounts are performed to analyze the influence of aerosols on the effect of weather modification through ice crystal seeding. The main conclusions are as follows.
(1) The precipitation strength is affected by the ambient CCN. Compared with CCN1500, the rainfall rate at the northern center in CCN8000 is obviously suppressed (by up to 29%), while the rainfall rate at the southern center is 13% larger than that of CCN1500 test.
(2) Aerosols can affect the development of clouds and precipitation, which in turn affects the efficacy of cloud seeding. When the aerosol concentration is increased and the seeding amounts are higher, such as 1×106/kg, the precipitation enhancement by cloud seeding tends to decrease, and the increment of accumulated precipitation decreases from 0.8 mm in CCN1500-Se6 to 0.4 mm in CCN8000-Se6.
(3) The seeding ice crystals grow mainly through the vapor deposition, snow growth also mainly depends on the vapor deposition process, and rainwater mainly comes from the melting of snow and graupel particles. With increased aerosol concentration, the deposition growth process of ice crystals is inhibited, the auto-conversion and accretion of ice crystals to snow get weaker, and the contribution of melting snow and graupel to rain water is also weaker, leading to a weaker precipitation enhancement by ice seeding in the higher CCN test.
This study examines the possible “side effect” of ambient aerosols on the precipitation enhancement by ice crystal seeding. The numerical simulation sensitivity tests of precipitation enhancement in this paper are based on the seeding method that directly adds man-made ice crystals to natural cloud. The follow-up studies may also examine AgI seeding method to verify the influence of aerosols on the cloud seeding effect.
Data availability statement
The original contributions presented in the study are included in the article/Supplementary Material, further inquiries can be directed to the corresponding authors.
Author contributions
XL conceptualized and completed the literature research and analysis. YS and YSha completed the model simulation, analysis, methodology, and manuscript writing. All authors contributed to the article and approved the submitted version.
Funding
This research was supported by the Special Innovation and Development Program of the China Meteorological Administration (CXFZ 2022J032) and the National Natural Science Foundation of China (NSFC) (42175109 and 41275148).
Conflict of interest
The authors declare that the research was conducted in the absence of any commercial or financial relationships that could be construed as a potential conflict of interest.
Publisher’s note
All claims expressed in this article are solely those of the authors and do not necessarily represent those of their affiliated organizations, or those of the publisher, the editors and the reviewers. Any product that may be evaluated in this article, or claim that may be made by its manufacturer, is not guaranteed or endorsed by the publisher.
References
Borys, R. D., Wetzel, M. A., Cohn, S. A., and Brown, W. O. J. (2003). Mountain top and radar measurements of anthropogenic aerosol effects on snow growth and snowfall rate. Geophys. Res. Lett. 30, 1538. doi:10.1029/2002GL016855
Bruintjes, R. T. (1999). A review of cloud seeding experiments to enhance precipitation and some new prospects. Bull. Am. Meteor. Soc. 80, 805–820. doi:10.1175/1520-0477(1999)080<0805:arocse>2.0.co;2
Chen, S., Xue, L., and Yau, M.-K. (2020). Impact of aerosols and turbulence on cloud droplet growth: An in-cloud seeding case study using a parcel–DNS (direct numerical simulation) approach. Chem. Phys. 20, 10111–10124. doi:10.5194/acp-20-10111-2020
Chen, W. D., Fu, D. H., Miao, S. G., and Zhang, Y. Z. (2015). Impacts of aerosols from Beijing and the surrounding areas on urban precipitation. I Chin. Sci. Bull. 60, 2124–2135. doi:10.1360/N972015-00217
Cotton, W. R. (1986). Testing, implementation, and evolution of seeding concepts—a review. Meteorol. Monogr. 21, 139–150. doi:10.1175/0065-9401-21.43.139
Dudhia, J. (1989). Numerical study of convection observed during the winter monsoon experiment using a mesoscale two-dimensional model. J. Atmos. Sci. 46 (20), 3077–3107. doi:10.1175/1520-0469(1989)046<3077:NSOCOD>2.0.CO;2
Fan, J., Wang, Y., Rosenfeld, D., and Liu, X. (2016). Review of aerosol–cloud interactions: Mechanisms, significance, and challenges. J. Atmos. Sci. 73, 4221–4252. doi:10.1175/jas-d-16-0037.1
Fang, W., Lou, X. F., Zhang, X., and Fu, Y. (2022). Numerical simulations of cloud number concentration and ice nuclei influence on cloud processes and seeding effects. Atmosphere 13, 1792. doi:10.3390/atmos13111792
Flossmann, A. I., Manton, M., Abshaev, A., Bruintjes, R., Yao, Z., Prabhakaran, T., et al. (2019). Review of advances in precipitation enhancement research. Bull. Am. Meteorol. Soc. 100, 1465–1480. doi:10.1175/bams-d-18-0160.1
Givati, A., and Rosenfeld, D. (2004). Quantifying precipitation suppression due to air pollution. J. Appl. Meteorology 43, 1038–1056. doi:10.1175/1520-0450(2004)043<1038:qpsdta>2.0.co;2
Hindman, E. E., Campbell, M. A., and Borys, R. D. (1994). A ten-winter record of cloud-droplet physical and chemical properties at a mountaintop site in Colorado. Appl. Meteor. 33, 797–807. doi:10.1175/1520-0450(1994)033<0797:atwroc>2.0.co;2
Hong, S. Y., Noh, Y., and Dudhia, J. (2005). A new vertical diffusion package with an explicit treatment of entrainment processes. Mon. Weather Rev. 134 (9), 2318–2341. doi:10.1175/MWR3199.1
Khain, A., Lynn, B., and Dudhia, J. (2010). Aerosol effects on intensity of landfalling hurricanes as seen from simulations with the WRF model with spectral bin microphysics. J. Atmos. Sci. 67, 365–384. doi:10.1175/2009jas3210.1
Khain, A., Rosenfeld, D., and Pokrovsky, A. (2005). Aerosol impact on the dynamics and microphysics of deep convective clouds. Q. J. R. Meteorological Soc. 131, 2639–2663. doi:10.1256/qj.04.62
Li, J. X., Yin, Y., Li, P. R., and Xu, F. (2014). Advances in research on mechanism and observation of impacts of aerosol on cloud and precipitation (in Chinese). J. Meteorological Sci. 34 (5), 581–590. doi:10.3969/2013jms.0067
Li, X., Zhang, Q., Fan, J., and Zhang, F. (2021). Notable contributions of aerosols to the predictability of hail precipitation. Geophys. Res. Lett. 48, e2020GL091712. doi:10.1029/2020
Lin, L., Fu, Q., Liu, X., Shan, Y., Giangrande, S. E., Elsaesser, G. S., et al. (2021). Improved convective ice microphysics parameterization in the NCAR CAM model. J. Geophys. Res. Atmos. 126, e2020JD034157. doi:10.1029/2020JD034157
Lin, L., Liu, X., Fu, Q., and Shan, Y. (2023). Climate impacts of convective cloud microphysics in NCAR CAM5. . J. Clim., 1–48. doi:10.1175/JCLI-D-22-0136.1
Liu, W. G., Tao, Y., and Dang, J., (2016). Seeding modeling study of two precipitation processes over northern China in the spring of 2014. Chin. J. Atmos. Sci. (in Chinese) 40 (4), 669–688. doi:10.3878/j.issn.1006-9895.1508.15138
Mansell, E. R., Ziegler, C. L., and Bruning, E. C. (2010). Simulated electrification of a small thunderstorm with two-moment bulk microphysics. Journal of the Atmospheric Sciences 67 (1), 171–194. doi:10.1175/2009JAS2965.1
Mlawer, E. J., Brown, P. D., Iacono, M. J., and Clough, S. A. (1997). Radiative transfer for inhomogeneous atmospheres: RRTM, a validated correlated-k model for the longwave. J. Geophys. Res. 102, 16663–16682. doi:10.1029/97JD00237
Pinsky, M., Khain, A., and Shapiro, M. (2000). Collision efficiency of drops in a wide range of Reynolds numbers: Effects of pressure on spectrum evolution. J. Atmos. Sci. 58, 742–764. doi:10.1175/1520-0469(2001)058<0742:ceodia>2.0.co;2
Pruppacher, H. R., and Klett, J. D. (1997). Microphysics of clouds and precipitation. 2nd Edition. Dordrecht: Kluwer Academic, 954.
Rangno, A. L., and Hobbs, P. V. (1995). A new look at the Israeli cloud seeding experiments. J. Appl. Meteorol. 34, 1169–1193. doi:10.1175/1520-0450(1995)034<1169:anlati>2.0.co;2
Rosenfeld, D., Axisa, D., Woodley, W. L., and Lahav, R. (2010). A quest for effective hygroscopic cloud seeding. J. Appl. Meteor. Climatol. 49, 1548–1562. doi:10.1175/2010jamc2307.1
Rosenfeld, D., Dai, J., Yu, X., Yao, Z., Xu, X., Yang, X., et al. (2007). Inverse relations between amounts of air pollution and orographic precipitation. Science 315, 1396–1398. doi:10.1126/science.1137949
Rosenfeld, D., Rudich, Y., and Lahav, R. (2001). Desert dust suppressing precipitation: A possible desertification feedback loop. Proc Natl Acad Sci U. S. A. 98 (11), 5975–5980. doi:10.1073/pnas.101122798
Rosenfeld, D., 2000, Suppression of rain and snow by urban and industrial air pollution, Science, 287(5459):1793–1796. doi:10.1126/science.287.5459.1793
Seifert, A., and Beheng, K. D. (2006). A two-moment cloud microphysics parameterization for mixed-phase clouds. Part 2: Maritime vs. continental deep convective storms. Meteorology & Atmospheric Physics 92 (1-2), 67–82. doi:10.1007/s00703-005-0113-3
Shan, Y., Liu, X., Lin, L., Ke, Z., and Lu, Z. (2021). An improved representation of aerosol wet removal by deep convection and impacts on simulated aerosol vertical profiles. Journal of Geophysical Research Atmospheres 126, e2020JD034173. doi:10.1029/2020JD034173
Shan, Y., Shi, H., Fan, J., Lin, L., Gao, L., He, C., et al. (2022). Revealing bias of cloud radiative effect in WRF simulation: Bias quantification and source attribution. Journal of Geophysical Research Atmospheres 127, e2021JD036319. doi:10.1029/2021JD036319
Shan, Y., Wilcox, E. M., Gao, L., Lin, L., Mitchell, D. L., Yin, Y., et al. (2020). Evaluating errors in gamma-function representations of the raindrop size distribution: A method for determining the optimal parameter set for use in bulk microphysics schemes. Journal of the Atmospheric Sciences 77 (2), 513–529. doi:10.1175/jas-d-18-0259.1
Shan, Y., Yin, Y., and Xiao, H. (2014). A sensitivity experiment of forecasting precipitation with double-moment microphysics schemes in WRF model. Journal of the Meteorological Sciences 34 (1), 1–9.
Shi, L. X., and Duan, Y. (2007). Observation of cloud condensation nuclei in north China. ACTA Meteorologica SINICA 65 (4), 644–652. doi:10.3321/j.issn:0577-6619
Shi, R., Wang, T. J., Li, S., Zhuang, B. L., Jiang, Z. Q., Liao, J. B., et al. (2015). The spatial and temporal characteristics of aerosol-cloud-precipitation interactions during summer in east asia (in Chinese). Chinese Journal of Atmospheric Sciences 39 (1), 12–22.
Teller, A., and Levin, Z. (2006). The effects of aerosols on precipitation and dimensions of subtropical clouds: A sensitivity study using a numerical cloud model. Atmos. Chem. Phys. 6, 67–80. doi:10.5194/acp-6-67-2006
Tewari, M., Chen, F., and Wang, W., (2004). Implementation and verification of the unified NOAH land surface model in the WRF model[C] Conference on Weather Analysis and Forecasting/Conference on Numerical Weather Prediction.:11–15.
Warner, J., and Twomey, S. (1967). The production of cloud nuclei by cane fires and the effect on cloud droplet concentration. J Atmos Sci 24, 704–706. doi:10.1175/1520-0469(1967)024<0704:tpocnb>2.0.co;2
Wurzler, S., Reisin, T. G., and Levin, Z. (2000). Modification of mineral dust particles by cloud processing and subsequent effects on drop size distributions. J. Geophys. Res. 105 (D4), 4501–4512. doi:10.1029/1999JD900980
Xiao, H., Yang, H. L., Hong, Y. C., Guo, C., Tang, Q., and Li, A. (2012). Numerical simulation of the impacts of ice nucleus spectra on cloud seeding effects in convective storm clouds (In Chinese). Clim. Environ. Res. 17, 833–847.
Xiao, H., and Yin, Y. (2011). A numerical study of polluted aerosol effects on precipitation in shanxi province (in Chinese). Chinese Journal of Atmospheric Sciences 35 (2), 235–246. doi:10.3878/j.issn.1006-9895.2011.02.04
Yang, H. L., Xiao, H., and Hong, Y. C. (2011). Progress in impacts of aerosol on cloud properties and precipitation (in Chinese). Climatic and Environmental Research 16 (4), 525–542.
Yin, Y., Wurzler, S., and Levin, Z. (2002). Effects on precipitation and cloud optical properties. J. Geophys. Res. 107 (D23), 4724.
Yue, Z. G., Liu, X. D., and Liang, Gu. (2011). Numerical simulation of influence of aerosols on different cloud precipitation types in beijing area (in Chinese). Plateau Meteorology 30 (5), 1356–1367.
Zhang, X. Y., Sun, J. Y., Wang, Y. Q., Zhang, Y., Cao, G., Zhang, Q., et al. (2013). Factors contributing to haze and fog in China (in Chinese). Chin Sci Bull (Chin Ver). 58, 1178–1187. doi:10.1360/972013-150
Zheng, G. G., and Guo, X. L. (2012). Status and development of sciences and technology for Chinese weather modification (in Chinese). Chinese Engineering Science 14 (9), 20–27.
Zhou, S. Y., Yang, J., Wang, W. C., Zhao, C. F., Gong, D., and Shi, P. (2020). An observational study of the effects of aerosols on diurnal variation of heavy rainfall and associated clouds over Beijing–Tianjin–Hebei. Chem. Phys. 20, 5211–5229. doi:10.5194/acp-20-5211-2020
Keywords: cloud condensation nuclei, rain enhancement, cloud microphyisics, meso-scale model, aerosol
Citation: Lou X, Shi Y and Shan Y (2023) A numerical simulation of CCN impacts on weather modification efficiency. Front. Environ. Sci. 11:1181207. doi: 10.3389/fenvs.2023.1181207
Received: 07 March 2023; Accepted: 13 April 2023;
Published: 09 May 2023.
Edited by:
Ashish Sharma, University of Illinois at Urbana-Champaign, United StatesReviewed by:
Nannan Qin, Fudan University, ChinaChenghao Tan, Chinese Academy of Sciences (CAS), China
Chunsong Lu, Nanjing University of Information Science and Technology, China
Copyright © 2023 Lou, Shi and Shan. This is an open-access article distributed under the terms of the Creative Commons Attribution License (CC BY). The use, distribution or reproduction in other forums is permitted, provided the original author(s) and the copyright owner(s) are credited and that the original publication in this journal is cited, in accordance with accepted academic practice. No use, distribution or reproduction is permitted which does not comply with these terms.
*Correspondence: Yu Shi, c2hpeXVAbWFpbC5pYXAuYWMuY24=; Yunpeng Shan, WXVucGVuZy5TaGFuQHBubmwuZ292