- 1GeneMind Biosciences Company Limited, Shenzhen, China
- 2Shenzhen Academy of Environmental Sciences, Shenzhen, China
Algal genomics research contributes to a deeper understanding of algal evolution and provides useful genomics inferences correlated with various functions. Published algal genome sequences are very limited owing to genome assembly challenges. Because genome data of freshwater algae are rapidly increasing with the recent boom in next-generation sequencing and bioinformatics, an interface to store, interlink, and display these data is needed. To provide a substantial genomic resource specifically for freshwater algae, we developed the Freshwater Algae Database (FWAlgaeDB), a user-friendly, constantly updated online repository for integrating genomic data and annotation information. This database, which includes information on 204 freshwater algae, allows easy access to gene repertoires and gene clusters of interest and facilitates potential applications. Three functional modules are integrated into FWAlgaeDB: a Basic Local Alignment Search Tool tool for similarity analyses, a Search tool for rapid data retrieval, and a Download function for data downloads. This database tool is freely available at http://www.fwalagedb.com/#/home. To demonstrate the utility of FWAlgaeDB, we also individually mapped metagenomic sequencing reads of 10 water samples to FWAlgaeDB and Nt algae databases we constructed to obtain taxonomic composition information. According to the mapping results, FWAlgaeDB may be a better choice for identifying algal species in freshwater samples, with fewer potential false positives because of its focus on freshwater algal species. FWAlgaeDB can therefore serve as an open-access, sustained platform to provide genomic data and molecular analysis tools specifically for freshwater algae.
1 Introduction
Algae are a group of aquatic organisms with diverse taxonomic, morphological, and genetic characteristics. Numerous species have been shown to play beneficial roles in carbon fixation and global productivity and have applications in renewable energy, aquaculture, and pharmaceutical production (Field et al., 1998; Hannon et al., 2010; Dewi et al., 2018; Sarker et al., 2018). Nevertheless, many potential applications and products derived from major algal species still await discovery. By providing proper genomic inferences correlated with various proteins and products from algae, the complete genomes of algae help generate new paradigms, ranging from sequences to functions. For example, comparative genomics opens the door to unknown function prediction by association with known functions of proteins (Gabaldón, 2008; Labarre et al., 2021).
Algal taxonomy initially relied on morphological traits to infer evolutionary trees and delineate taxa, and it was mainly based on nature or degree of similarities and/or differences. Genomic information, including whole genome features and marker genes, provides novel, promising taxonomic markers for algal taxonomy. Molecular sequence analysis supports the existence of approximately nine major phyla, including Cyanobacteria, Chlorophyta, Bacillariophyta, Ochrophyta, Euglenophyta, Cryptophyta, Dinophyta, Rhodophyta, and Streptophyta (Sahoo and Seckbach, 2015; Khan et al., 2020).
Although the value of algal genomes cannot be overstated, the number of published algal genome sequences is minuscule compared with a large number of species. The diversity of genome sizes, ranging from tiny (e.g., Ostreococcus sp. SAG12, genome size ∼2.76 Mb) (Benites et al., 2019) to giant (e.g., Prorocentrum micans, genome size ∼250 Gb) (Hou and Lin, 2009), and complex sequence characteristics have impeded algal genomics research to a certain extent (Khan et al., 2020). In recent decades, freshwater algae have attracted increasing attention from researchers for use in water quality monitoring, biodiversity estimation, and removal of heavy metals from wastewater (Shamshad et al., 2016). Along with the expansion in next-generation sequencing and bioinformatics, the amount of available genome data from freshwater algae is rapidly increasing. The storage, interlinking, and display of these data in an interface are therefore important.
Several public databases include information on freshwater algae, but these repositories have various limitations. GenBank (www.ncbi.nlm.nih.gov/genbank/) houses valuable algal genetic resources, including genomes, genes, and genome annotations, and has indisputably contributed to algal research. This database focuses mainly on genetic information, however, it contains little coverage of living environments and microscopic images of algae. In addition, GenBank has no specific filtering options for freshwater algae, which is cumbersome for researchers only interested in these organisms. Another database, JGI-PhycoCosm (https://phycocosm.jgi.doe.gov/phycocosm/home), integrates genome sequences and annotations of 136 algal genomes across the eukaryotic tree of life but does not include a large branch of algae–cyanobacteria. In addition, algae and other species with sequenced genomes are classified in JGI-PhycoCosm according to major eukaryotic clades without consideration of marine vs. freshwater habitats. JGI-Phytozome (https://phytozome-next.jgi.doe.gov/) focuses on plant genome information and comparative genomics studies and only includes a few algal species belonging to Archaeplastida. Finally, AlgaeBase (https://www.algaebase.org/) and Algae-Hub (https://www.algaehub.cn/#/home_page), which exist as online sources for taxonomic and distributional data on all algae, lack genome and annotation information.
The above-mentioned databases have been developed to serve as specialized repositories for algae, but an integrated resource of genome data and biological information for freshwater algae has not yet been developed. FWAlgaeDB, specially designed for freshwater algae, provides a more convenient academic platform and tool for use by freshwater algal scientists. This platform was developed by comprehensively collecting taxonomic classifications, distributional information, and available genome sequences of 204 freshwater algae and then re-annotating genes using a sequence similarity search against six public gene-function databases. FWAlgaeDB is an open-access, sustained platform with regular data updates. We anticipate that FWAlgaeDB will benefit researchers exploring the evolutionary history, biodiversity, and potential functions of freshwater algae.
2 Methods
2.1 Collecting the data
All genomic sequences in FWAlgaeDB were downloaded from the public NCBI database (https://www.ncbi.nlm.nih.gov/). Other information, such as taxonomy, algal images, living environment, geographic location, and references, was collected from NCBI, FACHB-collection (http://algae.ihb.ac.cn/english/), AlgaeBase (https://www.algaebase.org/), and Algae-Hub (https://www.algaehub.cn/#/home_page) databases and related publications. All copyrighted images were used in accordance with the Creative Commons Attribution-NonCommercial-NoDerivatives 4.0 International License; the images are to be used only for scientific research, without any commercial purposes.
2.2 Supplementing and improving the dataset
Algal gene prediction and function annotation were performed by Shanghai Origingene Bio-pharm Technology Co. In brief, Prodigal v2.6.3 (Hyatt et al., 2010) and GeneMark-ES (Lomsadze et al., 2005) were used for gene prediction in prokaryotic and eukaryotic genomes, respectively. The predicted genes were aligned against the NCBI Nt database (Sayers et al., 2010) using Basic Local Alignment Search Tool (BLAST) with an E-value cut-off of 1.0 × 10−5. Next, genes were functionally annotated against data deposited in public databases, including Nr (Deng et al., 2006), Swiss-Prot (Yip et al., 2008), GO (Ashburner et al., 2000), KEGG (Kanehisa et al., 2004), COG (Tatusov et al., 2000), and KOG (Koonin et al., 2004), using BLASTx according to the same criterion used for BLASTn.
2.3 Constructing the database
The FWAlgae database was developed with the following software: Java (jdk1.8) (https://www.oracle.com/java/) for the construction of the underlying data structure, Vue (2.0) (https://v2.vuejs.org/) for user pages and UI interaction logic, and Spring Cloud (2.1.9) (https://spring.io/projects/spring-cloud) for submitted data access and other service interfaces. All data in FWAlgaeDB were deposited and managed using Mysql (5.7.18) (https://www.mysql.com/). Feign (2.1.3) (https://spring.io/projects/spring-cloud-openfeign) was used to invoke the service interface. The BLAST tool (2.12.0) (https://blast.ncbi.nlm.nih.gov/Blast.cgi) was integrated into FWAlgaeDB for sequence similarity analysis.
2.4 FWAlgaeDB validation
Ten water samples were collected from the Shenzhen Reservoir (23°34′14″N 114°08′48″E) in Shenzhen, China. We used a 0.45-μm porous polycarbonate membrane (Collins, Shanghai, China) for water sample filtering and a FastDNA SPIN kit for soil (MP Biomedicals, Santa Ana, CA, United States) for DNA extraction. A library was constructed using 1 μg DNA and subjected to shotgun metagenomic sequencing on the GenoLab M sequencing platform (GeneMind Biosciences) operated in 100-cycle, single-end, high-output sequencing mode.
For taxonomic analysis, Kraken 2 was used with exact k-mer matches to achieve high accuracy and fast classification speeds (https://ccb.jhu.edu/software/kraken2/). The databases to be aligned were constructed using freshwater algal genomic data from FWAlgaeDB or algal genomic resources from the Nt database. We extracted all algal genomic information in the Nt database to form a new Nt algal database mainly derived from 10 phyla (Cyanobacteria, Chlorophyta, Bacillariophyta, Haptophyta, Rhodophyta, Dinophyta, Glaucophyta, Cryptophyta, Euglenophyta, and Ochrophyta). We subsequently compared the results of species identification between FWAlgaeDB and the Nt algae database. Kraken 2 was used to assign a taxonomic label to short DNA sequencing reads (confidence: 0.9) according to the internal k-mer (k = 35 bp) to the lowest common ancestor mapping algorithm. Relative abundance was then calculated on the basis of the proportion of fragments assigned to each taxon by Kraken 2.
3 Results
3.1 FWAlgaeDB overview
FWAlgaeDB, which was developed as a comprehensive, open-access hub for freshwater algae, integrates genomic datasets (FWAlgae pool), functional annotation files (Annotation), BLAST analysis, and search and download components (Figure 1). FWAlgaeDB currently contains 204 genomes from 204 species belonging to seven phyla. The database is hosted by the Shenzhen Academy of Environmental Sciences (SZAES) and GeneMind Biosciences, Ltd. SZAES updates the database every 6 months by adding new genomes (freshwater cyanobacteria and eukaryotic algae) directly downloaded from public databases (e.g., NCBI and JGI) or derived from checked, high-throughput sequencing data of isolated freshwater algae. GeneMind supports bioinformatics analysis of updated genomes, including gene prediction and functional annotations, and performs website technical maintenance.
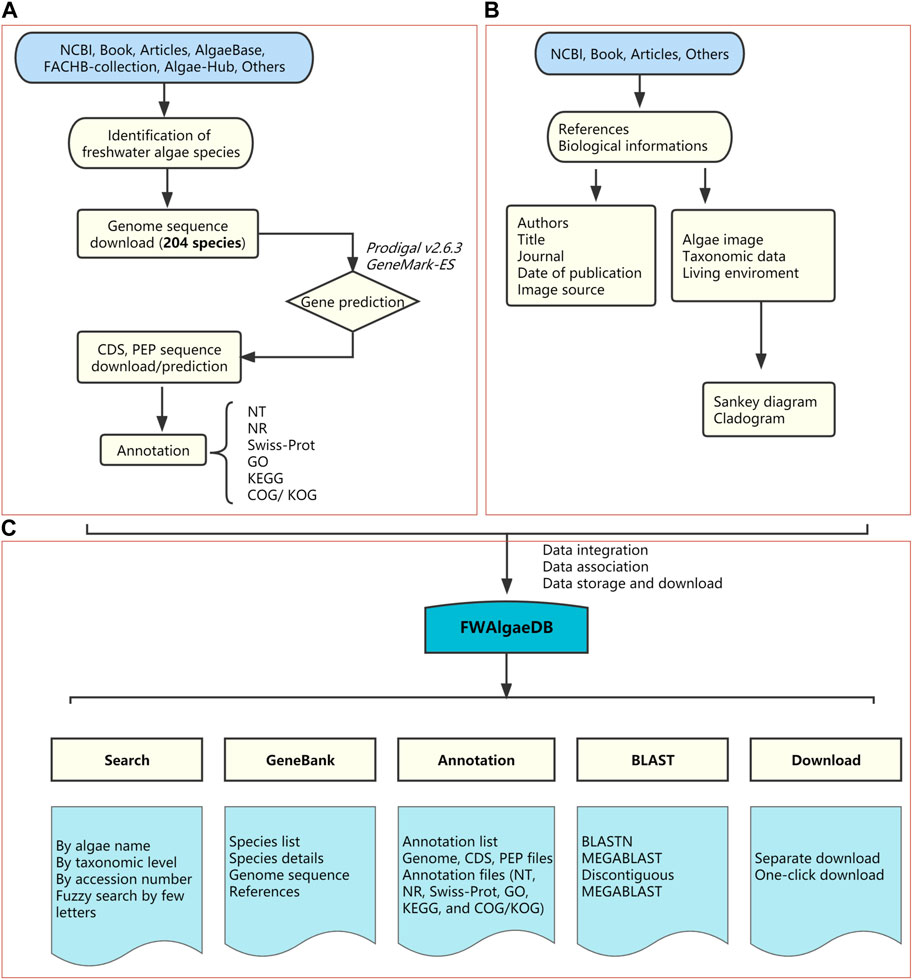
FIGURE 1. Schematic diagram of FWAlgaeDB construction. (A) Data sources and data preprocessing. (B) Collection of biological information and phylogenetic analysis. (C) Database construction and fundamental components.
3.2 Search function
FWAlgaeDB incorporates an intelligent search module to help researchers rapidly retrieve data of interest. To allow flexibility, a variety of search methods are available. To search at the taxonomic level (phylum, class, order, family, or genus), users can input taxonomic terms (e.g., Cyanobacteria, Ochrophyta, Chlorella, and Pseudanabaena) and receive genomic information on all species at the corresponding level. Researchers can jump to a specific page to browse or download relevant data. FWAlgaeDB uses a fuzzy matching algorithm, which lists results based on potential relevance even if search strings or spellings are incomplete. Researchers can also obtain species information by searching by scientific name, database number, or NCBI taxonomy ID. All of the above resources are freely available and downloadable.
3.3 FWAlgae pool
The FWAlgae pool section is an open-access repository containing taxonomic information and genome sequences for more than 200 species of freshwater algae. Genera and species of freshwater algae are listed alphabetically in the browser interface. Each species is linked to a specific page that displays detailed information, including algal images, taxonomy (according to the NCBI Taxonomy Database), accession number, living environment, and literature sources. On this page, researchers can download the genome sequence of the corresponding species in FASTA format. In addition, clicking Algae Name leads directly to the Annotation download interface.
A Sankey diagram is used to visualize the taxonomic abundance and distribution of algal species across different phylogenetic levels, ranging from kingdom to genus (Figure 2). Cyanobacteria, Chlorophyta, and Bacillariophyta are dominant at the phylum level.
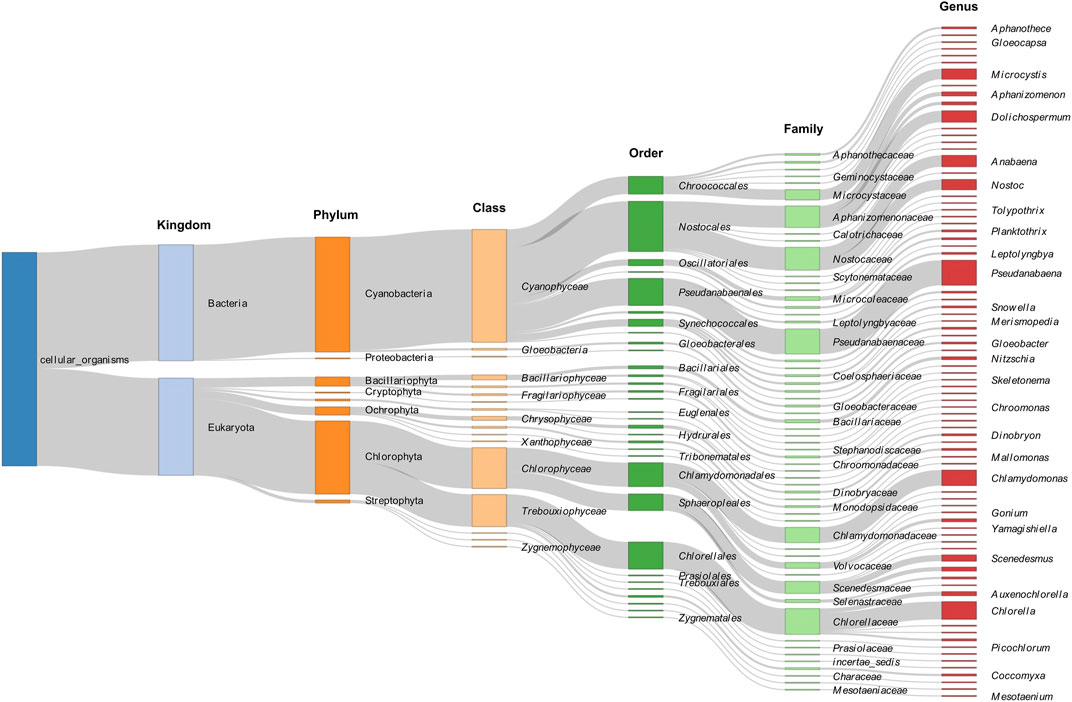
FIGURE 2. A Sankey diagram for visualization of the taxonomic abundance and distribution of algal species across different phylogenetic levels, ranging from kingdom to genus.
3.4 BLAST tool
The BLASTN/BLASTP tool was integrated into FWAlgaeDB to allow researchers to align a query sequence (nucleotide or amino acid sequence) against the whole database and obtain a BLAST report (Figure 3). Users can paste FASTA sequences in the operational interface or upload files to quickly search FWAlgaeDB for matches to the query genome/protein sequence. We adopted an E-value (Expect value) threshold for BLAST quality filtration to obtain significant alignments. The output data, a list of hits with corresponding E-values, are sorted by E-value by default, with the smallest E-value corresponding to the closest match. Advanced command-line parameters can be used to refine the search. BLASTN, MEGABLAST, and Discontiguous MEGABLAST are all available.
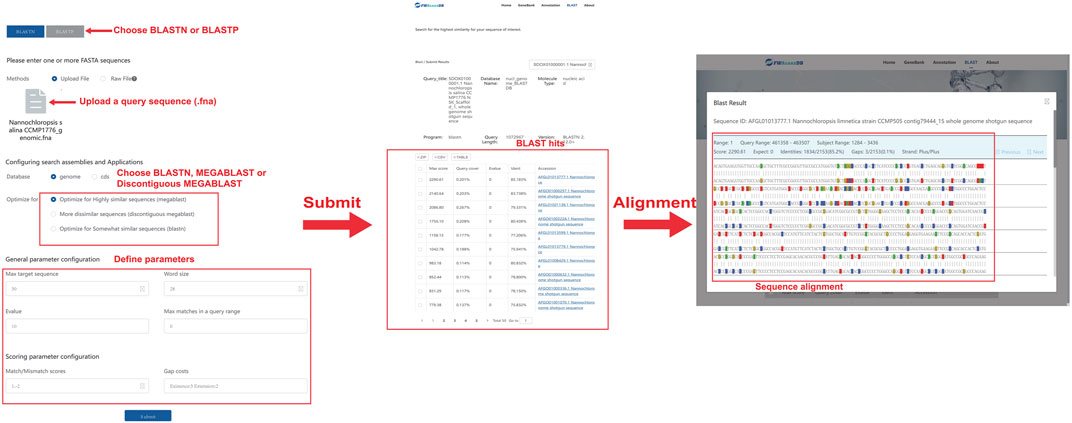
FIGURE 3. Operational interface and results of BLAST searching using Nannochloropsis salina CCMP1776 as an example.
3.5 Annotation files and download
The annotation interface is presented in tabular form, and users can collectively or individually select Genome, CDS, Protein, and different database annotations for downloading. Coding sequences (.ffn) and corresponding amino acid sequences (.faa) of 46 prokaryotic and 74 eukaryotic algal species have been predicted. The predicted data are listed as CDS and Protein items in the Annotation table and can be downloaded freely. We have also performed gene classification and functional annotations via BLAST against six public databases. Correspondingly, we annotated algal species with defined CDS and protein sequences from the NCBI database (65 prokaryotic and 19 eukaryotic algal species) against the six databases to determine gene classification and putative functions. To better understand algal genomic information, we collected and annotated major gene families, including ABC transporter permease, cytochrome p450, transposase, major facilitator superfamily, and algal toxin gene clusters, of the 204 algal species, located in “Gene Family”. The search tool embedded on this page also supports accurate searching by species name or database ID.
3.6 FWAlgaeDB validation via reservoir samples
To verify the validity of our database, we compared taxonomic compositions determined by mapping the sequence reads of ten water samples to FWAlgaeDB and Nt algae databases. Standard quality filtering of metagenomic sequences obtained using the GenoLab M platform yielded 28.17 M–75.23 M reads per sample. A comparison of the algal species identified using the two databases is shown in Figure 4. Compared with the number of species obtained using FWAlgaeDB, many more species were identified in the Nt database; in the latter case, however, identifications were more complicated, and freshwater algal species need to be further distinguished (Figure 4A). The large amount of data in the Nt database may be a distraction for researchers and obscure important information. Regarding relative abundance, the top five species in the two groups were different. Of note, several well-recognized marine species (marked in orange in the figure) were detected in the Nt database, an inexplicable result given that our samples were collected from a freshwater reservoir. The presence of these potential false positives suggests that FWAlgaeDB is a better choice for identifying algal species in freshwater samples. Further analysis, such as algal isolation and culture or PCR, are required to verify these species identifications. Figure 4B displays the abundance of the overlapping species detected in both databases. Significant differences in abundance are evident in this heat map, thus indicating the importance of database selection for use in species identification.
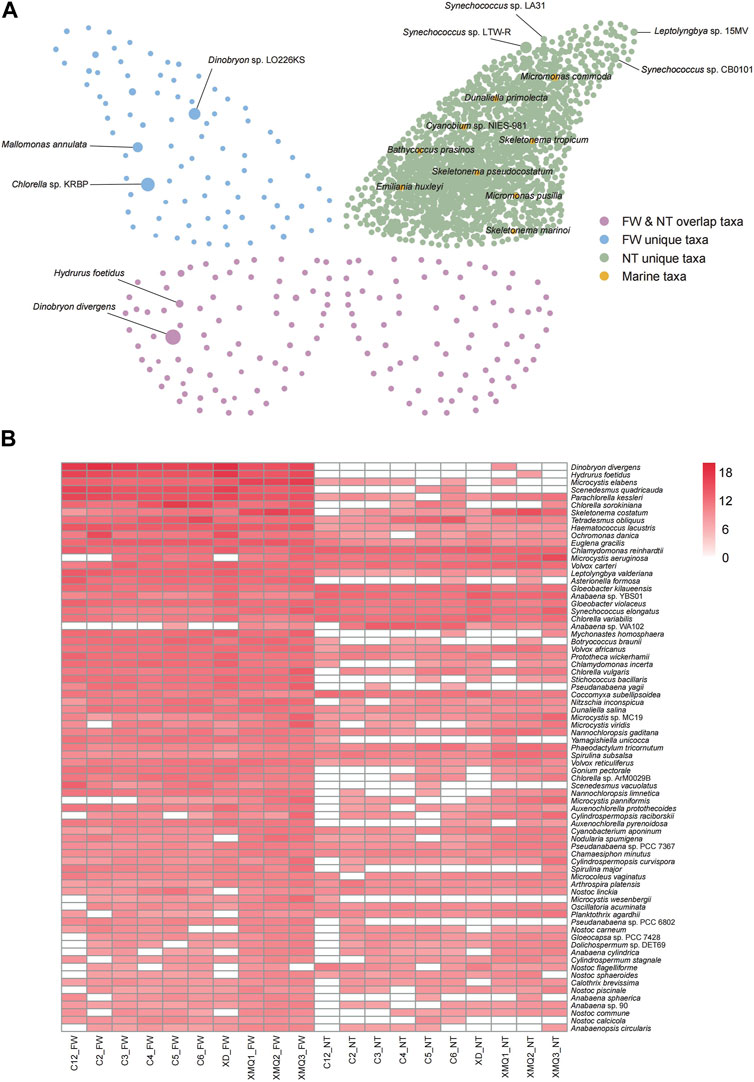
FIGURE 4. Comparison of algal species identified in 10 water samples using FWAlgaeDB and Nt algal databases. (A) Network plot showing the algal species identified in the two databases. Each node in the graph represents a species; the larger the node, the higher the abundance of the species. The upper left wing of the butterfly represents the unique species identified in the FWAlgaeDB, and the upper right wing of the butterfly indicates the unique species identified in the Nt database. The lower part of the butterfly (left and right) represents taxa that overlapped between the two databases. (B) Heat map displaying the abundance of overlapped species identified in both databases. Species abundance is represented by color (the darker the color, the higher the abundance), as indicated in the legend. The value was calculated by the following formula: lg (data*1e9+1).
4 Discussion
Analysis of algal genomes provides valuable insights, not only into algal identification, classification, and evolution, but also environmental adaptation mechanisms, sewage treatment applications, and genetic manipulation of bioeconomically relevant species. Nelson et al. combined high-throughput cultivation, genome sequencing, and bioinformatics strategies to infer that membrane and viral proteins shared among marine microalgae may contribute to the adaptive strategy of algal halotolerance (Nelson et al., 2021). Zhang et al. sequenced and assembled a high-quality genome sequence of an Antarctic psychrophilic green alga, Chlamydomonas sp. ICE-L, and found that massively expanded gene families involved in various processes, such as unsaturated fatty acid biosynthesis, DNA repair, and photoprotection, may support its extremophilic lifestyle (Zhang et al., 2020). Increasing attention has been paid to algae in waste management, as these organisms are considered to be effective for removal of heavy metal contamination from soil or water (Marella et al., 2020; Pande et al., 2022). The recent availability of genomic and omic datasets from diverse microalgal species have a remarkable potential to guide genetic engineering strategies in industrial strain improvement programs (B-Béres et al., 2022). Comparative genomics analysis of Chlorococcum sp. FFG039, a water surface-floating Chlorophyta, has revealed several gene families involved in biofilm formation, which are thus attractive targets for future reverse genomics studies aiming to elucidate water surface-floating mechanisms (Maeda et al., 2019).
With the development of genome sequencing technology and bioinformatics tools for data assembly and annotation, the amount of published algal genomic data has greatly increased. These data facilitate the study of the algal diversity of environmental samples, novel gene recognition, and gene functional comparison. Through integration of entire genome sequences, a genomic database can serve as a supplement to “DNA barcoding” libraries based on 16 S and ITS ribosomal RNA and aid taxonomic identification in the complex microbiomes or environments that are difficult to study. DNA barcoding enables the efficient recognition of all species using information from one or a few gene regions, whereas genomic data describe all gene functions and interactions in a single species. Both strategies are valuable approaches for obtaining genetic information and studying taxonomic diversity.
Given the above considerations, an integrative platform of algal genomes can help scientists easily apply genomic data for biological discovery through rapid retrieval and analysis of information related to algal genomes. We note, however, that the existing algal genome database still needs improvement, and some potential omissions exist in public databases. Regarding genomic data, GenBank and JGI provide no-cost access to high-throughput genomic data on algae. The former includes no images or distributional information on algae, whereas the latter currently focuses only on eukaryotic algal genomes, and both lack specific filtering options for freshwater algae. Researchers thus require information about freshwater species before downloading a genome of interest. Our taxonomic composition analysis of 10 water samples uncovered potential false positives when using the all-inclusive GenBank database (Figure 4). Regarding biological characteristics of algae, AlgaeBase, Algae-Hub, and FACHB-collection (http://algae.ihb.ac.cn/english/) provide free access to authoritative taxonomic, distributional, nomenclatural, and culture information. Although these valuable online resources for algae unquestionably contribute to phycological research and development, the lack of genomic data and analysis tools in these databases limits their use.
With its integration of genomic and biological information on 204 species of freshwater algae, FWAlgaeDB is a user-friendly platform for freshwater algae research. FWAlgaeDB includes options for basic biological information querying, genome downloading, sequence alignment, and species inference and should attract future interest in freshwater algal research. In particular, FWAlgaeDB provides gene prediction and multiple functional annotation files for each species in the pool, thereby greatly supplementing the insufficient annotations in GenBank, which is mainly focused on sequence characterizations, including coding regions and mRNA features. These genomic data will help researchers understand the evolutionary, structural, functional, and developmental aspects of freshwater algae at the genomic level.
FWAlgaeDB, however, has inherent limitations. First, the volume of FWAlgaeDB is samll, currently containing 204 freshwater algal species. This number pales in comparison to the enormous number of algae species although an increase has been made in contrast to the existing algal database, such as JGI. FWAlgaeDB is therefore designed as a continuously updated platform to cover more freshwater algae species. Second, ten reservoir samples used to verify the validity of this database do not adequately reflect all types of freshwater, including rivers, lakes, streams, etc. So, a larger number of samples and more diverse sample types (such as sediment samples) will be evaluated.
5 Conclusion
To our knowledge, FWAlgaeDB is the first database specifically designed for freshwater algae that integrates a large amount of genome data from different sources, predicts coding genes, and re-annotates functions. This database should be valuable for data retrieval/download and BLAST-based similarity alignments. A dramatic expansion in algal genomics in the next few years will require more genomic resources and efficient analysis tools. We plan to continuously update FWAlgaeDB with newly released data from public databases as well as our laboratory.
Data availability statement
The metagenomic sequencing data are available in the CNGB Sequence Archive (https://db.cngb.org/cnsa/) under project accession number CNP0003000.
Author contributions
LS and JF: directing the project. JL, QL, YoL, and QY: conceptualization, investigation, and writing, and editing. TS, WL, YiL, SW, and ZX: sample collection. YaL and ML: DNA extraction, library construction, and sequencing. MW, XZ, WY, and LeZ: bioinformatics analysis. HJ, YoL, and LiZ: database design. All authors contributed to the article and approved the submitted version.
Funding
This work was supported by the Shenzhen Science and Technology Program (No. KCXFZ20201221173007020).
Acknowledgments
The authors thank the members of the GeneMind team who contributed to the development of GenoLab M. We thank Liwen Bianji (Edanz) (www.liwenbianji.cn/ac) for editing the English text of a draft of this manuscript.
Conflict of interest
Authors JL, XZ, YoL, MW, WY, YaL, HJ, LeZ, ML, LiZ, QY, and LS were employed by the company GeneMind Biosciences Company Limited.
The remaining authors declare that the research was conducted in the absence of any commercial or financial relationships that could be construed as a potential conflict of interest.
Publisher’s note
All claims expressed in this article are solely those of the authors and do not necessarily represent those of their affiliated organizations, or those of the publisher, the editors and the reviewers. Any product that may be evaluated in this article, or claim that may be made by its manufacturer, is not guaranteed or endorsed by the publisher.
References
Ashburner, M., Ball, C. A., Blake, J. A., Botstein, D., Butler, H., Cherry, J. M., et al. (2000). Gene ontology: Tool for the unification of biology. Nat. Genet. 25 (1), 25–29. doi:10.1038/75556
B-Béres, V., Stenger-Kovács, C., Buczkó, K., Padisák, J., Selmeczy, G. B., Lengyel, E., et al. (2022). Ecosystem services provided by freshwater and marine diatoms. Hydrobiologia 850, 2707–2733. doi:10.1007/s10750-022-04984-9
Benites, L. F., Poulton, N., Labadie, K., Sieracki, M. E., Grimsley, N., and Piganeau, G. (2019). Single cell ecogenomics reveals mating types of individual cells and ssDNA viral infections in the smallest photosynthetic eukaryotes. Philos. Trans. R. Soc. B 374 (1786), 20190089. doi:10.1098/rstb.2019.0089
Deng, Y., Li, J., Wu, S., Zhu, Y., Chen, Y., and He, F. (2006). Integrated nr database in protein annotation system and its localization. Comput. Eng. 32 (5), 71–74. doi:10.3969/j.issn.1000-3428.2006.05.026
Dewi, I. C., Falaise, C., Hellio, C., Bourgougnon, N., and Mouget, J. L. (2018). “Anticancer, antiviral, antibacterial, and antifungal properties in microalgae,” in Microalgae in health and disease prevention (Cambridge: Academic Press), 235–261.
Field, C. B., Behrenfeld, M. J., Randerson, J. T., and Falkowski, P. (1998). Primary production of the biosphere: Integrating terrestrial and oceanic components. Science 281 (5374), 237–240. doi:10.1126/science.281.5374.237
Gabaldón, T. (2008). Comparative genomics-based prediction of protein function. Genomics Protoc. 387, 387–401. doi:10.1007/978-1-59745-188-8_26
Hannon, M., Gimpel, J., Tran, M., Rasala, B., and Mayfield, S. (2010). Biofuels from algae: Challenges and potential. Biofuels 1 (5), 763–784. doi:10.4155/bfs.10.44
Hou, Y., and Lin, S. (2009). Distinct gene number-genome size relationships for eukaryotes and non-eukaryotes: Gene content estimation for dinoflagellate genomes. PloS One 4 (9), e6978. doi:10.1371/journal.pone.0006978
Hyatt, D., Chen, G.-L., LoCascio, P. F., Land, M. L., Larimer, F. W., and Hauser, L. J. (2010). Prodigal: Prokaryotic gene recognition and translation initiation site identification. BMC Bioinforma. 11 (1), 119–211. doi:10.1186/1471-2105-11-119
Kanehisa, M., Goto, S., Kawashima, S., Okuno, Y., and Hattori, M. (2004). The KEGG resource for deciphering the genome. Nucleic. Acids Res. 32 (1), D277–D280. doi:10.1093/nar/gkh063
Khan, A. K., Kausar, H., Jaferi, S. S., Drouet, S., Hano, C., Abbasi, B. H., et al. (2020). An insight into the algal evolution and genomics. Biomolecules 10 (11), 1524. doi:10.3390/biom10111524
Koonin, E. V., Fedorova, N. D., Jackson, J. D., Jacobs, A. R., Krylov, D. M., Makarova, K. S., et al. (2004). A comprehensive evolutionary classification of proteins encoded in complete eukaryotic genomes. Genome Biol. 5 (2), R7–R28. doi:10.1186/gb-2004-5-2-r7
Labarre, A., López-Escardó, D., Latorre, F., Leonard, G., Bucchini, F., Obiol, A., et al. (2021). Comparative genomics reveals new functional insights in uncultured MAST species. Isme. J. 15 (6), 1767–1781. doi:10.1038/s41396-020-00885-8
Lomsadze, A., Ter-Hovhannisyan, V., Chernoff, Y. O., and Borodovsky, M. (2005). Gene identification in novel eukaryotic genomes by self-training algorithm. Nucleic. Acids Res. 33 (20), 6494–6506. doi:10.1093/nar/gki937
Maeda, Y., Nojima, D., Sakurai, M., Nomaguchi, T., Ichikawa, M., Ishizuka, Y., et al. (2019). Genome analysis and genetic transformation of a water surface-floating microalga Chlorococcum sp. FFG039. Sci. Rep. 9 (1), 11200–11207. doi:10.1038/s41598-019-47612-8
Marella, T. K., Saxena, A., and Tiwari, A. (2020). Diatom mediated heavy metal remediation: A review. Bioresour. Technol. 305, 123068. doi:10.1016/j.biortech.2020.123068
Nelson, D. R., Hazzouri, K. M., Lauersen, K. J., Jaiswal, A., Chaiboonchoe, A., Mystikou, A., et al. (2021). Large-scale genome sequencing reveals the driving forces of viruses in microalgal evolution. Cell Host Microbe 29 (2), 250–266.e8. doi:10.1016/j.chom.2020.12.005
Pande, V., Pandey, S. C., Sati, D., Bhatt, P., and Samant, M. (2022). Microbial interventions in bioremediation of heavy metal contaminants in agroecosystem. Front. Microbiol. 13, 824084. doi:10.3389/fmicb.2022.824084
Sahoo, D., and Seckbach, J. (2015). Classification of algae" in The algae world. Dordrecht, Netherlands: Springer, 31–55.
Sarker, P. K., Kapuscinski, A. R., Bae, A. Y., Donaldson, E., Sitek, A. J., Fitzgerald, D. S., et al. (2018). Towards sustainable aquafeeds: Evaluating substitution of fishmeal with lipid-extracted microalgal co-product (Nannochloropsis oculata) in diets of juvenile Nile tilapia (Oreochromis niloticus). PLoS One 13 (7), e0201315. doi:10.1371/journal.pone.0201315
Sayers, E. W., Barrett, T., Benson, D. A., Bolton, E., Bryant, S. H., Canese, K., et al. (2010). Database resources of the national center for biotechnology information. Nucleic Acids Res. 39 (1), D38–D51. doi:10.1093/nar/gkq1172
Shamshad, I., Khan, S., Waqas, M., Asma, M., Nawab, J., Gul, N., et al. (2016). Heavy metal uptake capacity of fresh water algae (Oedogonium westti) from aqueous solution: A mesocosm research. Int. J. Phytoremediation 18 (4), 393–398. doi:10.1080/15226514.2015.1109594
Tatusov, R. L., Galperin, M. Y., Natale, D. A., and Koonin, E. V. (2000). The COG database: A tool for genome-scale analysis of protein functions and evolution. Nucleic Acids Res. 28 (1), 33–36. doi:10.1093/nar/28.1.33
Yip, Y. L., Famiglietti, M., Gos, A., Duek, P. D., David, F. P., Gateau, A., et al. (2008). Annotating single amino acid polymorphisms in the UniProt/Swiss-Prot knowledgebase. Hum. Mutat. 29 (3), 361–366. doi:10.1002/humu.20671
Keywords: freshwater algae, genomic data, functional annotation, BLAST, species identification
Citation: Lai J, Liang Q, Zhang X, Liu Y, Wang M, Yang W, Sun T, Li Y, Jin H, Liu Y, Li W, Wu S, Xie Z, Zhou L, Luo M, Zeng L, Yan Q, Feng J and Sun L (2023) FWAlgaeDB, an integrated genome database of freshwater algae. Front. Environ. Sci. 11:1178097. doi: 10.3389/fenvs.2023.1178097
Received: 02 March 2023; Accepted: 06 July 2023;
Published: 18 July 2023.
Edited by:
Herb E. Schellhorn, McMaster University, CanadaReviewed by:
Benwen Liu, Chinese Academy of Sciences (CAS), ChinaZhi-Kai Yang, Guangzhou Medical University, China
Copyright © 2023 Lai, Liang, Zhang, Liu, Wang, Yang, Sun, Li, Jin, Liu, Li, Wu, Xie, Zhou, Luo, Zeng, Yan, Feng and Sun. This is an open-access article distributed under the terms of the Creative Commons Attribution License (CC BY). The use, distribution or reproduction in other forums is permitted, provided the original author(s) and the copyright owner(s) are credited and that the original publication in this journal is cited, in accordance with accepted academic practice. No use, distribution or reproduction is permitted which does not comply with these terms.
*Correspondence: Jie Feng, amFuZXBob25lQDE2My5jb20=; Lei Sun, c3VubGVpQGdlbmVtaW5kLmNvbQ==
†These authors have contributed equally to this work and share first authorship