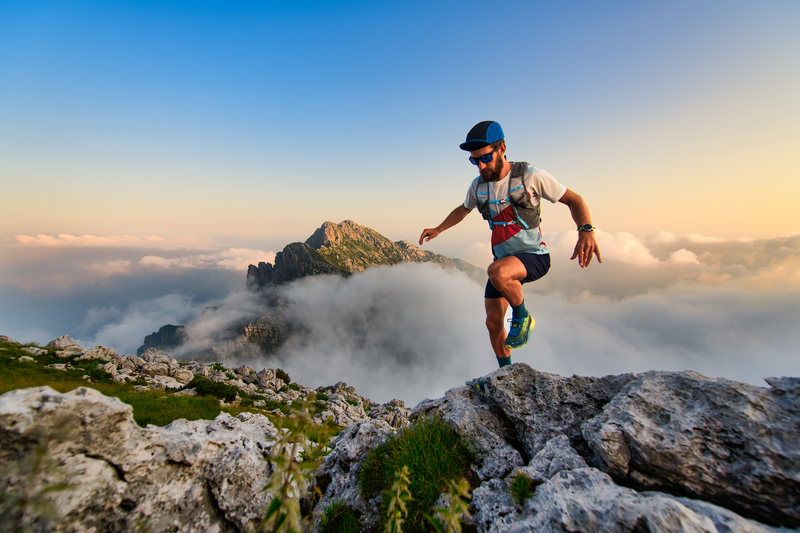
94% of researchers rate our articles as excellent or good
Learn more about the work of our research integrity team to safeguard the quality of each article we publish.
Find out more
ORIGINAL RESEARCH article
Front. Environ. Sci. , 24 March 2023
Sec. Environmental Economics and Management
Volume 11 - 2023 | https://doi.org/10.3389/fenvs.2023.1138876
This article is part of the Research Topic Nexus Between Innovations, Environmental Challenges and Labor Mobility View all 13 articles
Over the past few decades, the transportation sector has been the largest contributor to CO2 emissions in China. Research and Development spending leads to technological innovation in the country and could affect the CO2 emission in the country. Therefore, this study analyzes the nexus between CO2 emissions, transport infrastructure and R&D spending in China. A QARDL approach was used for the data analysis, which revealed Research and Development and Transport infrastructure has a positive impact on CO2 emissions. R&D was only significant in the first 25% quantile, while transportation was significant in almost all quantiles. These results suggest that R&D spending in China is mainly allocated to the sectors that emit the CO2 emission. It is recommended that government should allocate more R&D to carbon-reducing sectors. Furthermore, the government should consider green transportation investments and renewable energy projects in the transportation sector to reduce CO2 emissions in the country.
Research and development (R&D) spending has been attracting interest in research and academic contexts due to its impact on innovations in different sectors of the economy. In the transport sector, R&D spending has played a significant role in developing new tools, designs, and solutions that promote transport infrastructure efficiency and reduce CO2 emissions (Lin and Chen, 2020). R&D investments have been associated with improvement in different aspects of infrastructure. The government’s expenditure on generating innovations, knowledge and processes is essential in providing solutions to current challenges facing transport infrastructure (Saboori et al., 2014). Through R&D, new transport infrastructures can help provide cost-effective, disaster-resilient, long-lasting, environmentally friendly, and safe roads and railways. Consequently, a high level of service and efficiency can be achieved, contributing to developing a sustainable transport system (Frazier, 2010). Furthermore, R&D spending can be used to address the problems associated with the cost and durability of infrastructure. In order to realize self-healing and self-monitoring transport systems, new tools, methods, and test procedures can be developed through research. Long-life, high-performance, and advanced materials for infrastructure construction can reduce reconstruction and maintenance costs (Lounis and McAllister, 2016). Nevertheless, research indicates that R&D spending coast is high and maintaining such a budget may increase government expenditure (Rust and Sampson, 2020). High-speed rails and highways have been developed in China as a model for other countries. As of 2021, the country had highways exceeding 169,000 km (Liu and Wang, 2022). According to Mouhamed et al. (2017) Chinese transportation infrastructure has replaced the traditional transport structure; the port infrastructure has evolved into one of the most modern ports, the highway mileage used by traffic has increased to the second position, and the railway business has reached the third position, which includes the largest passenger and freight traffic as well as establishes the most important airports and aviation services in the world. Due to the rapid development and improvement of infrastructure in China during the last 4 decades, it is essential to examine how R&D has contributed to development and innovation in the transport sector. Although transport infrastructure has brought significant prosperity of economic growth and social development, it is the main factor responsible for environmental degradation (Ozcan et al., 2020).
There are significant externalities associated with the construction of transport infrastructures, as well as a long operational cycle and large-scale investment that have irreversible effects on the environment (Luo et al., 2018). For instance, constructing roads, rails, and ports requires using machines powered by fuel, which emit significant amounts of carbon dioxide into the environment (Huang et al., 2020). A recent study has revealed that China has the highest level of CO2 emissions from the transportation sector in the world (Xu et al., 2022). Since the Chinese transport sector is experiencing rapid growth, issues such as traffic flow, demand for transport, active transportation, and increased usage of diesel and gasoline will contribute to high levels of CO2 emissions (Khanali et al., 2021). The expansion of highway and rail networks contributes to CO2 emissions by promoting inter-regional activities, for example, the transportation of goods and industrial and manufacturing processes dependent on transportation. However, Kim and Lee, (2019) found that increased road and rail mileage results in increased transport mobility and reduced distance and travel time, which leads to decreased CO2 emissions. In contrast Tao and Chao, (2019) reported that transport infrastructure development does not have a direct impact on the amount of CO2 emissions generated by the users of roads, airlines, rails, and ports. There further argued that various factors, including the ability of people to purchase more vehicles, planes, water vessels, and trains, can affect the amount of CO2 emissions produced. Due to these conflicting viewpoints, there is a need for additional research to fill these gaps. In spite of the fact that green transport infrastructure is still at an early stage of development, the costs associated with implementation are high (Melo et al., 2020). Consequently, countries like China, which have heavily invested in non-green transport infrastructures, are increasingly using normal transport infrastructures despite the negative impact on the environment. To support green infrastructure, it is necessary to understand the extent to which infrastructure contributes to environmental pollution. There is a conflicting debate regarding whether R&D development and digitalization reduce or increase CO2 emissions, despite the positive impacts of R&D development, particularly in the business world (Umar et al., 2020; Ramos-Meza et al., 2021). The important aspect of R&D activities is that it makes recommendations regarding green transport infrastructures, such as electric rails and electric vehicles. In the transport sector, these green solutions contributed to significant reductions in CO2 emissions. (Sohail et al., 2021). However, the implementation of green transport infrastructure is limited due to its high cost. Research and development spending in the field of green transportation focuses on the design, production, and commercialization of new technologies that can reduce the environmental impact of transportation. This can include investments in the development of electric vehicles, hybrid powertrains, and sustainable transportation infrastructure, as well as efforts to improve the efficiency of existing technologies and systems. Governments, private companies, and research institutions can all contribute to research and development spending in the field of green transportation. The goals of these efforts typically include reducing greenhouse gas emissions, reducing dependence on fossil fuels, improving energy efficiency, and reducing the overall environmental impact of transportation. Petrović and Lobanov (2020) found that 1 percent of R&D investments reduces CO2 emissions by 0.09%–0.15% on average. In addition, studies have found that R&D activities in the transport sector can only have a significant impact on reducing CO2 emissions in the long run, while it may not affect CO2 emissions. Other studies have found that increased R&D expenditures increase CO2 emissions; For instance, Koçak and Ulucak (2019) Research, revealed no significant relationship between renewable energy, R&D expenditures and CO2 emissions. Research and development activities could increase emissions if the R&D activities involve the use of resources which contribute to the CO2 emissions. R&D activities at a higher level are expected to reduce pollution in the country; however, it may take a long time to achieve certain environmental objectives due to various social and economic constraints. Since literature has conflicting results on R&D spending, transport infrastructure and CO2 emissions. It is essential to re-examine this phenomenon with advanced statistical techniques. Co2 emissions and R&D spending both have an increasing trend in China economic history; therefore, it is essential to estimate the relationship between R&D spending, transport infrastructure development, and CO2 emissions in China, which may help to understand the impact of R&D spending and infrastructure development on the environment. As one of the largest economies and emitters of greenhouse gases, China’s actions in these areas could have global implications. The study could suggest some policy recommendations and contribute to efforts to reduce emissions and promote sustainable development. Therefore, the main objective of this paper is to investigate the relationship between R&D spending, transportation infrastructure and CO2 emission in China. There are several ways in which this research could provide new insights that can help to bridge the existing research; firstly, according to the best of our knowledge, there is no study that interlinks the R&D spending and transportation infrastructure in the context of China. Secondly, we use the case of China for our study; since infrastructure has significantly improved in China, the transport infrastructure has significantly increased in the last few decades in China; therefore, this study will provide implications of transport infrastructure for the CO2 emission in China. Thirdly, we applied a novel QARDL technique, which provides robust results compared to the past studies, and better policy recommendations can be established in the reliable results. The rest of the paper is organized as section 2 contains the review of the literature. The second three trend of transportation, CO2 emission and R&D in and four and four provides methodology, results and discussion; respectively, the section explains the conclusion of the study.
Marrero et al. (2021) investigated the relationship between road transport and CO2 emissions among 22 countries in Europe. Their finding reveals that road transport and related infrastructure released 27% of the total emissions in the countries. Furthermore, they concluded that most of the CO2 emissions associated with road transport are caused by the burning of fossil fuels, which implies that the amount of CO2 released into the atmosphere is primarily determined by the amount of energy consumed.
Pani et al. (2021) analyzed the ten largest countries of the world to determine how freight transport impacts carbon emissions. CO2 emissions from freight transport and infrastructure construction are the most significant contributors to CO2 emissions in these countries. Truck vehicles were mainly responsible for stimulating the demand for energy in transportation and shipments. Therefore, these trucks deteriorate the environment by burning fossil fuels. Cardenete and López-Cabaco (2021) investigated the case of Spain and reported that freight transport is one of the most cost-effective methods of transporting cargo, but it contributes 30% more CO2 emissions than other means of transportation. Arvin et al. (2021) also found similar results for Germany. The diesel and gasoline transport system released the most significant amount of CO2 into the atmosphere. According to these findings, highways and roads used by trucks and other types of vehicles indirectly impact transport infrastructure. Umar et al. (2020) Examined the impact of fossil and biomass energy consumption on CO2 emissions in the United States. transportation sector for the period 1981–2019 and used Spectral Breitung Candelon causality test, cointegration regression. The results of their study indicated that biomass energy is negative to carbon dioxide emissions. However, fossil energy consumption had a significant and positive impact on CO2 emissions. In addition, they found a U-shaped inverted curve relationship between total energy consumption and carbon dioxide emissions. The study conducted by Hussain et al. (2020) found contrasting results. In their study, different dimensions of infrastructure were considered, such as soft and hard infrastructure in Asian countries. Their findings suggest that transportation infrastructure and CO2 emissions were negatively correlated. Additionally, their results indicated that infrastructure development is a significant factor in CO2 emissions. Still, increased climate change in Asia was found to reduce the level of transport activities, reducing emissions through critical infrastructure. Despite these findings, it was found that level of transport activities reduces climate change in a given Asian region, thereby reducing the emission levels through critical infrastructure.
Churchill et al. (2019) investigated the relationship between R&D intensity and CO2 emissions in the transport sector in selected seven countries for the period 1870–2014, and results are extracted using non-parametric linear estimates. It was found that between 1955 and 1990, the intensity of R&D led to an increase in CO2 emissions, and between 1990 and 2014, CO2 emissions decreased. This suggests that other factors could have contributed to the variation in results. Koçak and Ulucak (2019) investigated how R&D expenditures affected energy consumption in transport infrastructures and transport in member countries of the OECD using the period 2003–2015. They applied regression analysis and suggested no relationship between R&D spending and CO2 emissions in the OECD countries. Nevertheless, they discovered that R&D expenditures on storage and power contributed to reducing CO2 emissions. Petrović and Lobanov (2020) examine the relationship between R&D spending and CO2 emissions for 16 OECD countries. The study used panel data from 1981 to 2014 and applied regression models for analysis. According to their findings, growth in R&D investments reduced the amount of CO2 emissions in the long run. Nevertheless, the results from individual countries indicated that the impact could either be negative or positive. Based on their study, “in most cases, higher R&D expenditures result in lower CO2 emissions, but this does not apply to 40% of countries. Sohail et al. (2021) studied the association between green transport and environmental pollution, suggested that green transport helped to reduce the amount of CO2 emissions. Specifically, their findings indicated that countries investing heavily in green energy, such as electricity, could have a minimal impact on CO2 emissions. Similarly, Oryani et al. (2021) explored the effectiveness of renewable energy in promoting a sustainable environment. They reported the adoption of green transport CO2 emissions per capita in the country. In the literature, it has been found that transport infrastructure is positively related to CO2 emissions. In addition, R&D and CO2 emission have mix findings.
Hidalgo Nuchera et al. (2009) examined the impact of adopting R&D on the transport and logistics industry. Their study adopted Structure-Conduct-Performance (SCP) model. In the transport and logistics sector, the level of IT knowledge and skills among employees was among the most important factors in facilitating R&D adoption. Additionally, their findings suggested new transportation models, new knowledge and supply chains emerging in the industry due to the adoption of R&D. Rust and Sampson (2020) used a system-based R&D management model to investigate the role of road and transport engineering in helping communities access roads and other transportation services. Their findings revealed that the systems-based approach had been demonstrated to improve the impact assessment indicators of R&D programmes for community access roads and transportation. In other words, the transport system is improved to provide solutions to the challenges facing road users. Parast, (2020) examine R&D mitigated challenges and disruptions experienced by United States firms. R&D was found to be a key factor in the development of new models that contributed to improving the transport sector’s resilience capabilities. Innovations improve resilience; it helps address challenges such as disruptions in demand, supply, and processes.
Kahouli, (2018) examine the impact of innovation on carbon emissions in the transportation industry, both in developed and developing Mediterranean countries. The results suggest that increases in innovation in developing nations lead to a reduction in carbon emissions from transportation. In developed countries, more innovation in the transportation sector is expected to result in a reduction in carbon. R&D innovation has long been recognized as an essential tool in reducing carbon emissions from transportation. The transportation sector can improve its energy consumption patterns through scientific research. J. Zhang et al. (2022) suggests that the growth of the digital economy lowers carbon emissions because it improves the energy structure by reducing the demand for fossil fuels. Arslan et al. (2022a) suggested that growing urban populations, merchandise trade, and financial development contribute to environmental degradation. Arslan et al. (2022b) argued that in modern research, the adverse impacts of climate change had increased the importance of sustainability disclosures. Furthermore, Bilal et al. (2022) suggests that companies with more carbon emissions have better financial reporting quality, indicating a negative relationship between carbon emission disclosures and discretionary accruals. Adebayo et al. (2022) among the other factors, the recent emergence of COVID-19 effect CO2 emissions, and they suggested that COVID-19 decreases the CO2 emissions in United Kingdom.
Figure 1 illustrates the trends in transportation and research and development (R&D) in China from 1990 to 2021. According to the data, R&D has increased significantly from 1990 to 2021, although this trend levelled between 1995 and 1996. China has significantly invested in R&D for the past 20 years, which explains this trend. China’s National Bureau of Statistics reports that investment in R&D accounted for 2.4% of the total gross domestic product. For instance, the country had 350 national engineering research centres and 522 national key laboratories in 2020 (Daily, 2022). According to a 10-year comparison with European countries, China’s R&D is currently higher than the Europeans’ average. According to Shead (2021), in 2020, China’s spending on research and development increased 10.3% to 2.44 trillion Chinese yuan ($378 billion). China invested $405 billion in research and development in 2020, which is 14.6% increase over the previous year. It should be noted, however, that despite this rising trend in research and development, the trend in transportation shows fluctuating in the given period. Based on the information provided in the data, it is difficult to conclude that R&D contributed to the improvement of the transport system in China. For instance, in the graph, China’s transport went to zero in the years 1990, 1992, 1995, 1999–2004, and 2008 despite rising investments in R&D. However, between 2004 and 2005 there was a rising trend in the improvement of transport. Also, there is an upward trend from 2013 to 2018, which then drops dramatically in the year 2020. The drop-down in public and private transport investment after 2018 mainly occurred because the Chinese government gave attention to the cross-border transportation infrastructure. Since China introduced its Belt Road initiative, which led to the development of tarns-continental passages connecting China with Asia and Europe by land and sea (Zhao, 2020), these initiatives created investment opportunities in aboard, and large investments have been diverted abroad. China reported that “in 1949, the total railway length of the country was only 21,800 km, of which half was barely functional. A total of 52,000 km of railway were operational by the end of 1978. Approximately 132,000 km of railways were in operation in China by 2018, five times longer than in 1949, with a 2.6% annual growth rate. (Special Report, 2019). Still, the introduction of high-speed trains in the country had reached 29,000 km, which was more than two-thirds of the total. In terms of roads, specifically expressways, China has the longest expressway in the world at 143,000 km (Ke and Yan, 2021). It is difficult for a country like China to achieve such innovative transport infrastructure without the help of R&D, despite the graphical data barely illustrating the impact of R&D and transport improvements. Thus, the statistical analysis is required to understand the linkages between R&D and transport upgradation.
Figure 2 shows trends in transport and CO2 emissions in China between 19,990 and 2021. In general, the graph shows that transport and CO2 emissions tend to follow similar trends. Between 1990 and 1992 there was a spike in transport development and the amount of CO2 emissions. There was a slight decline in both transport and CO2 emissions between 1994 and 1995, which was followed by an increase in both. There was a gradual decrease in CO2 emissions between 2000 and 2011, transport had a rising and dropping trend during this period, and between 2016 and 2020, the transportation sector is expected to experience a rapid increase. CO2 emissions, however, remain the same during this period. The trend of CO2 emissions has generally been rising from 1990 to 2021, while the trend for transport has been rising and dropping, and there have been several spikes or fluctuations during this period. In China, the transport sector accounted for 9% of all CO2 emissions, which makes it the third largest source after the building sector and the industrial sector. Considering that the construction of transportation infrastructures typically requires the use of fossil fuels and it is expected that it might have a higher percentage. Additionally, China’s vehicle ownership ratio is a major contributor to its current and future CO2 emissions. Based on the study, only 200 people out of a thousand own a vehicle, which is a bit lower than in other countries such as United States and the European Union.
According to Bank (2021), it is challenging to decarbonize the transportation sector, particularly as the number of people purchasing vehicles in middle income countries like China continues to increase rapidly. It is projected that China will consume 50% less energy and emit 80% less CO2 by 2050 in the transportation sector (K. Zhang et al., 2019). In response to the increased emission of CO2 in the transportation sector, China has adopted a series of mitigation measures, including the adoption of CO2 emission standards and the acceleration of the electrification of vehicles. Although China’s EV market share is set to exceed 80 percent by 2050, soaring motorization rates would still result in excessive carbon dioxide emissions from transportation by 2050. Consequently, China faces a challenging situation due to the increased number of vehicles on the roads.
Figure 3 above shows the trends in CO2 emissions and R&D spending in China between 1990 and 2021. According to the graph above, there is an overall upward trend in the amount of CO2 emissions and R&D spending in China. The country’s R&D expenditures increased continuously between 1990 and 1995. In 1996, this trend slightly decreased, after which a rising trend continued until 2021. In the period 1990–1993, CO2 emissions increased steadily. CO2 levels slightly declined between 1994 and 1995, followed by a spike; there was a gradual decrease in CO2 emissions between 2000 and 201, while CO2 emissions steadily increased after 2012. These trends go beyond the expectations that R&D would help reduce CO2 emissions to the environment. Research indicates that most environmental innovations in China were successful in reducing carbon dioxide (CO2) emissions. Li and Wang (2017) examined 95 countries between 1996 and 2007 for the impact of technology shifts on CO2 emissions.
The results indicate that technological progress has a negative effect on CO2 emissions when only magnitude and intensity are considered. Lee and Min (2015) studied the influence of green research and development investment on CO2 emissions and the financial performance of Japanese manufacturing enterprises. Their findings indicate that funding green research and development negatively impacts carbon dioxide emissions. Garrone and Grilli, (2010) analysed the public spending in energy R&D on carbon emissions for 13 industrialized countries between 1980 and 2004. This study indicates that government investments in energy R &D do not affect CO2 emissions.
Climate change, environmental dextrorotation, and temperature are continuously increasing, and it is recognized that carbon emission is a crucial factor in determining environmental pollution. Therefore, to enlist the influence of fossil fuel utilization, transportation, research and development are arranged to capture the impact on carbon emissions. Several researchers employed various methods to investigate the implication of R&D, and transportation on CO2 emission. The following model is used for the data analysis
The above equation presents a linear form of the baseline model; the CO2 presents the CO2 emissions in the country, which is the dependent variable. FS is the fossil fuel consumption; T is the transport development and R is the research and development spending China, FS, T and R are the explanatory variables. According to theory, FS and T has a positive effect the CO2 emissions. While is assumed either positive or negative association with CO2 emissions.
Cho et al. (2015) suggested the quantile form QARDL (p,q,r,s,u) for the model presented in Equation 1, which is an extension of the original model.
The QARDL model depicted by Equation 2 is generalized due to the possibility of serial correlation.
The following is a generalized restatement of Equation 3 demonstrating the QARDL-ECM model:
Whereas short-term cumulative effects of historical and present FS, T, R, and CO2 levels are calculated by:
To determine the long-term parameter for FS, T, R, and CO2, we use the formula:
The ECM parameter (
Moreover, to determine the stationary in the data, Augmented Dicky Fuller (ADF) is utilized, mathematically expressed in Equations (7), (8). In order to start the empirical analysis, the unit root test will be estimated at the beginning. Theoretically, it is assumed that a series should have no unit root. To start, the unit root test reveals if certain data are integrated. To test the random shocks in the data, the unit root test combines them at many scales. Such consistent results may be more useful for long-term forecasting. However, the proposed strategies may be unsuitable if the series is not stable, and the data contains random shocks. The unit root test estimates the unit root properties of the variable.
Equations (7) and (8) examine the series on the intercept, trend, and intercept to capture the unit root in all series. The Zivot-Adrews test is applied in this scenario to determine if the data has a structural break. The data should be transformed by differencing the variables before being included in the regression model if the unit root tests determine that a series has a single unit root. One of the limitations of ADF unit root tests is that structural discontinuities may be incorrectly interpreted as indicators of non-stationarity. It is possible that they will not be able to reject the unit root hypothesis in the case of a structural break that exists in the data. If the structure break on a given variable is related to a specific event, such as a change in policy, a currency crisis, or a war, the process may identify the exact moment when the break occurred. The Zivot-Andrews test permits only a single crack in the structure.
The Wald test is used to determine if the explanatory variables in a model are statistically significant; the significance of variables indicates that they contribute to the model in some way. Multiple models, including ones with binary variables, are suitable for the test. Mathematically expressed as.
Autocorrelation refers to the correlation between two-time series over a range of time. An autocorrelation analysis determines how closely a variable’s present value relates to its historical values.
In particular, it determines if the response variable can be explained by combining non-linear combinations of the fitted values. The idea behind the test is that the response variable can be described using only non-linear combinations of the explanatory variables. Then the model is incorrectly specified, and a polynomial or other non-linear functional form would better approximate the process by which the data were generated.
The current study investigates the influence of transportation infrastructure and research and development expenditures on carbon emissions in consideration of the Chines economy. In order to achieve this objective, a time series dataset (1990–2021) has been compiled from the World Development Indicator (WDI). To summarize the variables statistics, the study employed descriptive analysis. A descriptive statistic is composed of three segments; the first segment defines the tendency of the data, which includes on mean, median, maximum, and minimum statistics. Secondly, descriptive elaborates the standard deviation, which measures the deviation of factors values from the mean. Thirdly, this analysis captures the symmetry of data (skewness) and high or low-tailed (kurtosis) data spread. Lastly, Jarque-Bera (JB) measures the model’s goodness of fit. The outcomes are articulated in Table 1.
Table 1 shows the tendency of data ranges between the maximum and minimum values since the average values of each factor are within the defined ranges. While the deviation from means also lies in the thumb rule statistics value such as 2. Moreover, the symmetry of the data lies in thumb rule values such as 3. Whereas the high or low tailed of the dataset also lies in the accepted statistics range such as 10. Additionally, JB discloses the model’s goodness of fit, which is one more positive aspect of the study. Therefore, it can be concluded that the overall spread of the dataset, deviation from the mean, and central tendency, along with the model’s fitness, all support the validity of the data results and estimations. The pre-requisite condition of time series is that data should be stationary before performing baseline estimation. For this purpose, the study employed the unit root test. The outcome is reported in Table 2.
The result in Table 2 elaborates that only R has a unit root at a 1% level of significance. At the same time, all the factors have zero mean and constant variance at first difference. Moreover, FS is stationary at 5%, and CO2, T, and R have a unit root at a 1% significant level. Next, the study uses the Zivot Andrew test to determine the discontinuity in the dataset. The results are mentioned in Table 3.
The information in Table 3 suggests that all the variables have a break in different years at the intercept and the on-trend break in the dataset. Consequently, it concludes that all the factors have unit roots at first differences and a break in the dataset. Recently, the QARDL model has gained considerable traction in the field of time series analysis. It is a flexible model for autocorrelation, separating long- and short-term connections and handling asymmetry relationships. The result of Q-ARDL is reported in Table 4.
We calculate the long-term and short-term effects of FS, T, and R&D on carbon emission in quantiles by using quantile ARDL. The results are presented in table 4, which suggests that in the long run, first quantile (Q = 0.25), only research and development spending influences carbon emission, and it has no effect second, third and fourth quantiles. In addition, in the second quantile (q = 5), all the factors like FS and T influence carbon emission. More importantly, the coefficient of FS, T, and R&D is significant at 1, five and10 percent, showing the long-term impact on carbon emission, respectively. Additionally, FS has a 1% significant impact on carbon emission in the third quantile (q = 75). While FS has a 5% significant impact on carbon emission in the fourth quantile (q = 85). However, in the short run, FS with one lag significantly affected the carbon emanation at 5%. In contrast, transportation infrastructure at a 5% significant level affects carbon emission in the first quantile of the short run. In the second quantile, T (−1) is associated with carbon emission, with a 5% significance level. Spending on research and development also interlinks with the carbon emission at a 1% significant level. Moreover, the association of FS and R has a connection with carbon emission in the third quantile at 10% and 1% significant levels. However, in the fourth quantile (q = 85), all the factors FS, T, and R are affected by carbon emission. The outcomes indicate that fossil fuel consumption, transportation system, and research and development expenditures are affecting the CO2 emission by 5% and 10% level of significance. Besides, the study performs numerous tests to determine the reliability of results and stability of the model. For this purpose, the study used Wald, serial correlation, and the Ramsey test. The outcomes are reported in Table 5.
The Wald test in Table 5 reported that all the datasets of factors significantly influencing the carbon emission, rejecting H0, i.e., this implies that all variables jointly affect the CO2 emission. Whereas the outcomes of Ramsey and serial correlation also show no autocorrelation problem in the data. Furthermore, diagnostic tests (residual, stability, and coefficient) are all positive for the research. There is a continuous increase in fossil fuel consumption in China. Moreover, the transportation infrastructure, which assists in transporting and stimulating the business, also consumes a considerable quantity of fossil fuel (Rahman, 2019). By contrast, R&D expenditures are increasing to locate modern methods of production, distribution and to introduce the latest technologies to affect CO2 emissions (Anser et al., 2020). Climate change, environmental degradation, and increased temperatures are the major threats that the world faces today; tremendous carbon emissions represent the primary threat to the environment (Hwei et al., 2021). On the contrary, carbon emission is increasing significantly in developing economies due to the expansion of economic activities and industrialization. In general, all nations are experiencing high levels of carbon emissions.
The results reported that fossil fuel consumption, R&D, and transportation play a positive role in emitting carbon in China. Undoubtedly, each factor is responsible for emitting carbon in the economy, but fossil fuel consumption is at the top because of its high utilization. Every economic sector utilizes fossil fuels, especially industry and transportation. The energy shortage in developing economies is one of the reasons they heavily rely on fossil fuels. The outcomes elaborate that with a 1% growth in fossil fuel utilization, carbon emission in the economy increased by 0.19%. A rise in fossil fuel consumption will lead to an increase in carbon emissions, which pollute the environment and threaten the environment, causing environmental degradation. These findings are in support by Qiu et al. (2020); Martins et al. (2021), Koondhar et al. (2021); Atsu et al. (2021); Rehman et al. (2019), and RAHMAN, (2019).
A total of 37% of CO2 emissions are attributed to terminal sectors in 2021, and transportation is the industry that is most dependent on fossil fuels (Zhao et al., 2022). Following a historic decline in 2020, transportation-related CO2 emissions increased by approximately eight percent in 2021 as pandemic restrictions were relaxed and passenger and freight operations resumed (Huang et al., 2021). While the transportation sector is forecast to grow by nearly 20% by 2030, to meet the Net Zero Scenario, the sector’s emissions will need to decline by almost 20% to less than 6 metric tons (Zhao et al., 2022). The findings are in line with Li et al. (2021); Jiang et al. (2019); Huang et al. (2021); Wang et al. (2021); and Zhang et al. (2021).
Based on the projections, it appears that R&D spending has a negative average influence on CO2 emissions. The average CO2 emissions are reduced by 0.09%–0.15% for every 1 percent increase in R&D spending (Petrović and Lobanov, 2020). Estimated regressions for individual countries show that R&D spending can positively or negatively impact CO2 emissions, ranging from 0.79% in Denmark to 0.52% in Belgium (Petrović and Lobanov, 2020). In other words, increased spending on R&D often results in lower CO2 emissions in the long run, albeit this is not the case for around 40% of nations (Petrović and Lobanov, 2020). The estimate of the short-run time-varying coefficient panel data models also showed that the influence of R&D might be positive, negative, or neutral (insignificant) over a long period. The analysis finds that R&D positively affects carbon emission, indicating that as a 1% upsurge in R&D, carbon increased by 0.02% in the economy. However, the findings of the study are supported by Artha et al. (2021); Shahbaz et al. (2020); Qin et al. (2021); Gan and Smith (2011); and Aldakhil et al. (2019). Table 6 presents the Granger Causality Tests results, which is used for the robustness of baseline results. The Granger Causality test suggests a unidirectional causality from CO2 to FS. There is unidirectional causality from R to CO2 emissions, which indicates that R&D spending causes the CO2 emissions. In addition, there is causality running from T to CO2 emissions which indicates that transport development causes the CO2 emissions in China. Furthermore, R is causing T, which implies that research and development cases transportation development. These findings reported the validity of the bassline results.
Literature suggests that carbon plays an important role in environmental degradation in developed and developing countries. Among the number of factors, transportation and R&D are the main factors that determine the CO2 emission. Therefore, the study mainly focuses on the nexus between transport infrastructure, CO2 emissions and R&D spending in China. Modern econometric approaches, such as the ZA test and Q-ARDL, are used for the data analysis. The finding suggests that transportation infrastructure and R&D both have positive implications for CO2 emissions. The spending on research and development also influences carbon emissions. Because of more expenditure in the research and development sector, countries are producing modern machinery for production purposes, which has an inverted U-shape affiliation with the environment. The condition of the transportation network affects the emission of carbon dioxide, and transportation infrastructure upgradation increases the number of vehicles, stimulating business activities and heavy fissile fuel consumption that leads to higher CO2 emissions in the country.
These findings suggest a few policy recommendations; firstly, the positive association of R&D implies that most of the R&D is allocated to projects that contributed to the CO2 emission in the country. Besides, the R&D spending ignores the environmental factor engaged in the innovation’s activities. Therefore, it is necessary that R&D spending should allocate to green energy projects such as green technologies, green energy, and green vehicles to improve environmental quality, which would help the economy to mitigate CO2 emissions. Secondly, the transport infrastructure is positively related to CO2 emission, which indicates with the transport infrastructure upgradation; the CO2 emission in the country increases. Therefore, the government should focus on green transportation and other modes such as “electrification of vehicles, etc. Thirdly R&D government should allocate R&D activities to the electrification of vehicles and green transportation that could help to achieve the transportation and environmental goals. In this study, there are some limitations since the focus is only on one country; in the future, the study may be expanded to include multiple countries as well. It is also possible that future studies may adopt this new technique in order to reexamine this relationship by applying the advanced statistical technique. This study has some limitations; firstly, this study focuses on one country, and the results of the study may not be generalizable to other countries and regions with different cultural, economic, and political conditions. Future studies may test this hypothesis by including multiple countries that allow the comparison of results across different countries. This can help to identify any country-specific effects and to better understand the underlying factors that influence the relationship between variables. Secondly, this study uses QARLD method future studies may advance techniques such as Quantile on Quantile approach, which provide more robust results and policy recommendations. Thirdly, future studies can also consider incorporating additional variables and control variables to better account for relationship between R&D spending, Transport development and CO2 emissions to increase the robustness of the analysis.
The original contributions presented in the study are included in the article/supplementary material, further inquiries can be directed to the corresponding author.
JY, JM, YF, and SW conceptualized the contribution. LH wrote and edit the manuscript. All authors listed have made a substantial, direct and intellectual contribution to the work, and approved the submission of the manuscript.
The authors declare that the research was conducted in the absence of any commercial or financial relationships that could be construed as a potential conflict of interest.
All claims expressed in this article are solely those of the authors and do not necessarily represent those of their affiliated organizations, or those of the publisher, the editors and the reviewers. Any product that may be evaluated in this article, or claim that may be made by its manufacturer, is not guaranteed or endorsed by the publisher.
CO2—, Carbon Dioxide; FS—, Fossil Fuel; R—, Research and Development Spending; T—, transport development; QARDL—, Quantile ARDL; SCP—, Structure-Conduct-Performance; ECM—, Error Correction Model; WDI—, World Development Indicator; JB—, Jarque-Bera.
Adebayo, T. S., AbdulKareem, H. K., Kirikkaleli, D., Shah, M. I., and Abbas, S. (2022). CO2 behavior amidst the COVID-19 pandemic in the United Kingdom: The role of renewable and non-renewable energy development. Renew. Energy 189, 492–501. doi:10.1016/j.renene.2022.02.111
Aldakhil, A. M., Zaheer, A., Younas, S., Nassani, A. A., Abro, M. M. Q., and Zaman, K. (2019). Efficiently managing green information and communication technologies, high-technology exports, and research and development expenditures: A case study. J. Clean. Prod. 240, 118164. doi:10.1016/j.jclepro.2019.118164
Anser, M. K., Hanif, I., Alharthi, M., and Chaudhry, I. S. (2020). Impact of fossil fuels, renewable energy consumption and industrial growth on carbon emissions in Latin American and Caribbean economies. Atmosfera. doi:10.20937/ATM.52732
Arslan, H. M., Khan, I., Latif, M. I., Komal, B., and Chen, S. (2022a). Understanding the dynamics of natural resources rents, environmental sustainability, and sustainable economic growth: New insights from China. Environ. Sci. Pollut. Res. 29 (39), 58746–58761. doi:10.1007/s11356-022-19952-y
Arslan, H. M., Chengang, Y., Komal, B., and Chen, S. (2022b). Nexus between environmental disclosures and top management team characteristics: A systematic review. Environ. Sci. Pollut. Res. 30, 9763–9781. doi:10.1007/s11356-022-22615-7
Artha, B., Bahri, B., Khairi, A., and Fernando, F. (2021). The effect of R&D expenditure on CO2 emissions in Austria. J. Bus. Manag. Rev. 2, 394–400. doi:10.47153/jbmr26.1482021
Arvin, M. B., Pradhan, R. P., and Nair, M. S. (2021). Are there links between institutional quality, government expenditure, tax revenue and economic growth? Evidence from low-income and lower middle-income countries. Econ. Anal. Policy 70, 468–489. doi:10.1016/j.eap.2021.03.011
Atsu, F., Adams, S., and Adjei, J. (2021). ICT, energy consumption, financial development, and environmental degradation in South Africa. Heliyon 7, e07328. doi:10.1016/j.heliyon.2021.e07328
Bank, W. (2021). The 500-million-vehicle question: What will it take for China to decarbonize transport?
Cardenete, M. A., and López-Cabaco, R. J. T. P. (2021). Economic and environmental impact of the new mediterranean rail corridor in andalusia: A dynamic cge approach. Transp. Policy (Oxf). 102, 25–34. doi:10.1016/j.tranpol.2020.12.007
Churchill, S. A., Inekwe, J., Smyth, R., and Zhang, X. (2019). R&D intensity and carbon emissions in the G7: 1870–2014. Energy Econ. 80, 30–37. doi:10.1016/j.eneco.2018.12.020
Frazier, K. A. (2010). “The role of research in improving infrastructure: An analysis of US transportation research and development,” in Massachusetts institute of technology.
Gan, J., and Smith, C. T. (2011). Drivers for renewable energy: A comparison among OECD countries. Biomass Bioenergy 35, 4497–4503. doi:10.1016/j.biombioe.2011.03.022
Garrone, P., and Grilli, L. (2010). Is there a relationship between public expenditures in energy R&D and carbon emissions per GDP? An empirical investigation. Energy Policy 38 (10), 5600–5613. doi:10.1016/j.enpol.2010.04.057
Hashmi, S. M., Chang, B. H., Huang, L., and Uche, E. (2022). Revisiting the relationship between oil prices, exchange rate, and stock prices: An application of quantile ARDL model. Resour. Policy. 75, 102543. doi:10.1016/j.resourpol.2021.102543
Hidalgo Nuchera, A., López Rodríguez, V., and Albors Garrigós, J. (2009). Drivers and impacts of R&D adoption on transport and logistics services.
Huang, D., Han, M., and Jiang, Y. (2021). Research on freight transportation carbon emission reduction based on system dynamics. Appl. Sci. 11, 2041. doi:10.3390/app11052041
Huang, G., Zhang, J., Yu, J., and Shi, X. (2020). Impact of transportation infrastructure on industrial pollution in Chinese cities: A spatial econometric analysis. Energy Econ. 92, 104973. doi:10.1016/j.eneco.2020.104973
Hussain, Z., Shahenn, W. A., and Raza, S. H. (2020). Trade, infrastructure and geography: An application of gravity model on Asian economies. Int. J. Transp. Econ. 47, 145–169.
Hwei Khaw, K. L., and Jia Ni, T. (2021). Fossil fuel price, carbon dioxide emission, and renewable energy capacity: Evidence from asian developing countries. Int. J. Bank. Financ. 16, 79–96. doi:10.32890/ijbf2021.16.1.5
Jiang, Y., Zhou, Z., and Liu, C. (2019). The impact of public transportation on carbon emissions: A panel quantile analysis based on Chinese provincial data. Environ. Sci. Pollut. Res. 26, 4000–4012. doi:10.1007/s11356-018-3921-y
Kahouli, B. (2018). The causality link between energy electricity consumption, CO2 emissions, R&D stocks and economic growth in Mediterranean countries (MCs). Energy 145, 388–399. doi:10.1016/j.energy.2017.12.136
Ke, X., and Yan, Y. (2021). Can proactive fiscal policy achieve the goal of “beyond keynesianism”? Evidence from China’s national highway system. Rev. Dev. Econ. 25 (2), 1078–1103. doi:10.1111/rode.12752
Khanali, M., Akram, A., Behzadi, J., Mostashari-Rad, F., Saber, Z., Chau, K.-w., et al. (2021). Multi-objective optimization of energy use and environmental emissions for walnut production using imperialist competitive algorithm. Appl. Energy 284, 116342. doi:10.1016/j.apenergy.2020.116342
Kim, J., and Lee, B. (2019). More than travel time: New accessibility index capturing the connectivity of transit services. J. Transp. Geogr. 78, 8–18. doi:10.1016/j.jtrangeo.2019.05.008
Koçak, E., and Ulucak, Z. Ş. (2019). The effect of energy R&D expenditures on CO 2 emission reduction: Estimation of the STIRPAT model for OECD countries. Environ. Sci. Pollut. Res. 26, 14328–14338. doi:10.1007/s11356-019-04712-2
Koondhar, M. A., Tan, Z., Alam, G. M., Khan, Z. A., Wang, L., and Kong, R. (2021). Bioenergy consumption, carbon emissions, and agricultural bioeconomic growth: A systematic approach to carbon neutrality in China. J. Environ. Manage. 296, 113242. doi:10.1016/j.jenvman.2021.113242
Lee, K.-H., and Min, B. (2015). Green R&D for eco-innovation and its impact on carbon emissions and firm performance. J. Clean. Prod. 108, 534–542. doi:10.1016/j.jclepro.2015.05.114
Li, M., and Wang, Q. (2017). Will technology advances alleviate climate change? Dual effects of technology change on aggregate carbon dioxide emissions. Energy sustain. Dev. 41, 61–68. doi:10.1016/j.esd.2017.08.004
Li, Y., Dong, H. K., and Lu, S. (2021). Research on application of a hybrid heuristic algorithm in transportation carbon emission. Environ. Sci. Pollut. Res. 28, 48610–48627. doi:10.1007/s11356-021-14079-y
Lin, B., and Chen, Y. (2020). Will land transport infrastructure affect the energy and carbon dioxide emissions performance of China’s manufacturing industry? Appl. Energy 260, 114266. doi:10.1016/j.apenergy.2019.114266
Liu, S., and Wang, J. (2022). Coupling coordination between marine innovation and the high-quality development of the marine economy: A case study of China’s coastal provinces. Sustainability 14 (12), 7373. doi:10.3390/su14127373
Lounis, Z., and McAllister, T. P. (2016). Risk-based decision making for sustainable and resilient infrastructure systems. J. Struct. Eng. 142 (9), F4016005. doi:10.1061/(asce)st.1943-541x.0001545
Luo, Z., Wan, G., Wang, C., and Zhang, X. (2018). Urban pollution and road infrastructure: A case study of China. China Econ. Rev. 49, 171–183. doi:10.1016/j.chieco.2017.04.008
Marrero, Á. S., Marrero, G. A., González, R. M., and Rodríguez-López, J. (2021). Convergence in road transport CO2 emissions in Europe. Energy Econ. 99, 105322. doi:10.1016/j.eneco.2021.105322
Martins, T., Barreto, A. C., Souza, F. M., and Souza, A. M. (2021). Fossil fuels consumption and carbon dioxide emissions in G7 countries: Empirical evidence from ARDL bounds testing approach. Environ. Pollut. 291, 118093. doi:10.1016/j.envpol.2021.118093
Melo, C., Teotónio, I., Silva, C. M., and Cruz, C. O. (2020). What’s the economic value of greening transport infrastructures? The case of the underground passages in lisbon. Sustain. Cities Soc. 56, 102083. doi:10.1016/j.scs.2020.102083
Mouhamed, B. B., and Qiu, Y. (2017). Transport infrastructure development in China. J. Sustain. Dev. Transp. Logist. 2 (1), 29–39. doi:10.14254/jsdtl.2017.2-1.3
Oryani, B., Koo, Y., Rezania, S., Shafiee, A., Khan, M. K., and Mahdavian, S. M. (2021). The role of electricity mix and transportation sector in designing a green-growth strategy in Iran. Energy 233, 121178. doi:10.1016/j.energy.2021.121178
Ozcan, B., Tzeremes, P. G., and Tzeremes, N. G. (2020). Energy consumption, economic growth and environmental degradation in OECD countries. Econ. Model. 84, 203–213. doi:10.1016/j.econmod.2019.04.010
Pani, A., Sahu, P. K., and Holguín-Veras, J. (2021). Examining the determinants of freight transport emissions using a fleet segmentation approach. Transp. Res. Part D: Transp. Environ. 92, 102726. doi:10.1016/j.trd.2021.102726
Parast, M. M. (2020). The impact of investment on mitigating supply chain disruptions: Empirical evidence from U.S. firms. Empir. Evid. US firms 227, 107671. doi:10.1016/j.ijpe.2020.107671
Petrović, P., and Lobanov, M. M. (2020). The impact of R&D expenditures on CO2 emissions: Evidence from sixteen OECD countries. J. Clean. Prod. 248, 119187. doi:10.1016/j.jclepro.2019.119187
Qin, L., Kirikkaleli, D., Hou, Y., Miao, X., and Tufail, M. (2021). Carbon neutrality target for G7 economies: Examining the role of environmental policy, green innovation and composite risk index. J. Environ. Manage. 295, 113119. doi:10.1016/j.jenvman.2021.113119
Qiu, R., Xu, J., Xie, H., Zeng, Z., and Lv, C. (2020). Carbon tax incentive policy towards air passenger transport carbon emissions reduction. Transp. Res. Part D. Transp. Environ. 85, 102441. doi:10.1016/j.trd.2020.102441
Ramos-Meza, C. S., Zhanbayev, R., Bilal, H., Sultan, M., Pekergin, Z. B., and Arslan, H. M. (2021). Does digitalization matter in green preferences in nexus of output volatility and environmental quality? Environ. Sci. Pollut. Res. 28, 66957–66967. doi:10.1007/s11356-021-15095-8
Rehman, A., Rauf, A., Ahmad, M., Chandio, A. A., and Deyuan, Z. (2019). The effect of carbon dioxide emission and the consumption of electrical energy, fossil fuel energy, and renewable energy, on economic performance: Evidence from Pakistan. Environ. Sci. Pollut. Res. 26, 21760–21773. doi:10.1007/s11356-019-05550-y
Rust, F. C., and Sampson, L. R. (2020). A systems-based R&D management model for the road and transport engineering sector applied to a community access roads and transport research programme. Afr. J. Sci. Technol. Innovation Dev. 12 (5), 547–560. doi:10.1080/20421338.2019.1640344
Saboori, B., Sapri, M., and bin Baba, M. (2014). Economic growth, energy consumption and CO2 emissions in OECD (organization for economic Co-operation and Development)'s transport sector: A fully modified bi-directional relationship approach. Energy 66, 150–161. doi:10.1016/j.energy.2013.12.048
Shahbaz, M., Nasir, M. A., Hille, E., and Mahalik, M. K. (2020). UK's net-zero carbon emissions target: Investigating the potential role of economic growth, financial development, and R&D expenditures based on historical data (1870–2017). Technol. Forecast. Soc. Change 161, 120255. doi:10.1016/j.techfore.2020.120255
Sohail, M. T., Ullah, S., Majeed, M. T., and Usman, A. (2021). Pakistan management of green transportation and environmental pollution: A nonlinear ARDL analysis. Environ. Sci. Polluti 28 (23), 29046–29055. doi:10.1007/s11356-021-12654-x
Special Report (2019). 70 years of China’s transport development. Available at: china.org.cn.
Tao, K., and Chao, Y. (2019). Rethinking port role as transport corridor under symbiosis theory-case study of China-Europe trade transportation. Int. J. Sustain. Dev. World Policy 8 (1), 51–61. doi:10.18488/journal.26.2019.81.51.61
Umar, M., Ji, X., Kirikkaleli, D., and Xu, Q. (2020). COP21 Roadmap: Do innovation, financial development, and transportation infrastructure matter for environmental sustainability in China? J. Environ. Manage. 271, 111026. doi:10.1016/j.jenvman.2020.111026
Rahman, Z. U. (2019). Does CO2 and its possible determinants are playing their role in the environmental degradation in Turkey. Environment kuznets curve does exist in Turkey. J. Wellbeing Manag. Appl. Psychol. 2, 19–37. Preston University. doi:10.13106/jwmap.2019.Vol2.no2.19
Wang, W., Wang, S., and Su, J. (2021). Integrated production and transportation scheduling in e-commerce supply chain with carbon emission constraints. J. Theor. Appl. Electron. Commer. Res. 16, 2554–2570. doi:10.3390/jtaer16070140
Xu, H., Cao, S., and Xu, X. (2022). The development of highway infrastructure and CO2 emissions: The mediating role of agglomeration. J. Clean. Prod. 337, 130501. doi:10.1016/j.jclepro.2022.130501
Zhang, J., Jin, W., Philbin, S. P., Lu, Q. C., Ballesteros-Pérez, P., Skitmore, M., et al. (2021). Impact of environmental regulations on carbon emissions of transportation infrastructure: China's evidence. Clean. Responsible Consum. 2, 100010. doi:10.1016/j.clrc.2021.100010
Zhang, J., Lyu, Y., Li, Y., and Geng, Y. (2022). Digital economy: An innovation driving factor for low-carbon development. Environ. Impact Assess. Rev. 96, 106821.
Zhang, K., Liu, X., and Yao, J. (2019). Identifying the driving forces of CO2 emissions of China’s transport sector from temporal and spatial decomposition perspectives. Environ. Sci. Pollut. Res. 26 (17), 17383–17406. doi:10.1007/s11356-019-05076-3
Zhao, P., Zeng, L., Li, P., Lu, H., Hu, H., Li, C., et al. (2022). China's transportation sector carbon dioxide emissions efficiency and its influencing factors based on the EBM DEA model with undesirable outputs and spatial Durbin model. Energy 238, 121934. doi:10.1016/j.energy.2021.121934
Keywords: R&D spending, transport infrastructure development, CO2 emissions, QARDL, China
Citation: Han LM, You JQ, Meng JN, Fu YL and Wu SL (2023) Empirical analysis of R&D spending, transport infrastructure development and CO2 emissions in China. Front. Environ. Sci. 11:1138876. doi: 10.3389/fenvs.2023.1138876
Received: 06 January 2023; Accepted: 03 March 2023;
Published: 24 March 2023.
Edited by:
Bilal, Hubei University of Economics, ChinaReviewed by:
Sami Ullah Khan, Gomal University, PakistanCopyright © 2023 Han, You, Meng, Fu and Wu. This is an open-access article distributed under the terms of the Creative Commons Attribution License (CC BY). The use, distribution or reproduction in other forums is permitted, provided the original author(s) and the copyright owner(s) are credited and that the original publication in this journal is cited, in accordance with accepted academic practice. No use, distribution or reproduction is permitted which does not comply with these terms.
*Correspondence: Ling Mei Han, OTkwMTYxN0BoYXVzdC5lZHUuY24=
Disclaimer: All claims expressed in this article are solely those of the authors and do not necessarily represent those of their affiliated organizations, or those of the publisher, the editors and the reviewers. Any product that may be evaluated in this article or claim that may be made by its manufacturer is not guaranteed or endorsed by the publisher.
Research integrity at Frontiers
Learn more about the work of our research integrity team to safeguard the quality of each article we publish.