- 1School of Statistics, Institute of Quantitative Economics, Huaqiao University, Xiamen, China
- 2School of Business, Minnan Normal University, Zhangzhou, China
Objective: To measure the low-carbon development level and digital transformation degree of China’s manufacturing industry, and to examine the impact of digital transformation on low-carbon development.
Methods: This paper uses Super Slack Based Measure (SBM) model and multi-regional input-output model to measure the low-carbon development level and digital transformation degree of 17 manufacturing industries in 30 provinces of China from 2012 to 2018, and uses high-dimensional fixed effect model and mediation model to study the impact of digital transformation on low-carbon development.
Results: 1) During the study period, China’s manufacturing industry showed an upward trend in terms of low-carbon development level and digital transformation, but there were significant regional and industrial disparities. 2) Digital transformation can significantly promote the low-carbon development of manufacturing industry, which is still valid in the robustness test. 3) For sub-indicators, digital industrialization has the most obvious effect on the low-carbon development of manufacturing industry, and the improvement of digital development environment also has a positive impact on low-carbon development. 4) The heterogeneity analysis indicate that digital transformation has a greater impact on promoting low-carbon development of manufacturing in underdeveloped regions, and the positive effect is obvious in medium-low-energy-consuming industries, but not in high-energy-consuming industries. 5) The mechanism test shows that technological innovation is a channel for digital transformation to promote low-carbon development.
Value: This paper provides empirical evidence for the environmental impact of digital transformation, and offers a scientific basis for relevant departments to formulate low-carbon development policies from the perspective of digital transformation.
1 Introduction
After more than 40 years of reform and opening up, China’s manufacturing industry has achieved leapfrog development. However, due to the long-term extensive production mode oriented by high energy consumption and high pollution in China’s traditional manufacturing industry, environmental problems have also expanded rapidly with the development of traditional manufacturing industry (Peng et al., 2022). According to data from CEADs, the energy consumption of 30 manufacturing industries in China increased from 0.61 billion tons of standard coal in 2000 to 2.3 billion tons of standard coal in 2019, an increase of nearly 2.8 times in 20 years; at the same time, carbon emissions increased from 1.12 billion tons to 3.51 billion tons, an increase of 2.1 times in 20 years. The continuous increase in energy consumption and carbon emissions not only has a negative impact on the sustainable development of China’s economy, but also seriously hinders China’s progress towards its peak carbon and carbon neutrality goals (Ge et al., 2022). Therefore, it is an urgent and practical issue to be studied how to break the crude development mode of some industries and realize the low-carbon development of China’s manufacturing industry.
At present, digital technologies represented by the Internet, artificial intelligence and big data are deeply integrated with various fields of economic and social development, gradually becoming a strong engine for the transformation of new and old kinetic energy in China (Zhang C et al., 2022). According to the “China Digital Economy Development Report (2022)” released by the China Academy of Information and Communications Technology, the scale of China’s digital economy reached 7.1 trillion US dollars in 2021, accounting for 39.8% of Gross Domestic Product (GDP), which shows that the digital economy has changed from an important component of China’s economy to a key leading force for economic development. In the critical period of China’s economic transformation, the application of digital technology will undoubtedly affect the mode of industrial production, and will also have a profound impact on the industry’s energy demand and carbon emissions (Ren et al., 2021; Wang J et al., 2022). From existing literature, most scholars focus on the economic effects of digital transformation, both exploring its important impact on the economic development of countries or regions (Mićić, 2017; Pan et al., 2022; Wu and Yang, 2022) and its key role in corporate development (Bhimani, 2015; Ballestar et al., 2021; Gaglio et al., 2022; Zhang J et al., 2022). With the development of digital economy and the tightening of resource and environmental constraints, the environmental effects of digital transformation have attracted the attention of scholars. Relevant studies show that the development of regional digital economy has a positive effect on reducing energy consumption (Ren et al., 2021), improving green total factor productivity (Li and Liao, 2022; Lyu et al., 2023), promoting clean energy development (Chen, 2022), and promoting green development efficiency (Luo et al., 2022). Some scholars have studied the impact of digital transformation on energy efficiency (Zhang L et al., 2022), green technology innovation (El-Kassar and Singh, 2019; Ning et al., 2022) and environmental management (Xia et al., 2022) from the enterprise level. Their research also confirms that digital transformation can promote green development. However, the relationship between digital transformation and carbon emissions remains controversial in academia. Most scholars (Ge et al., 2022; Yu et al., 2022; Zha et al., 2022) believe that digital transformation can help reduce carbon emissions. Zhang W et al. (2022) found that the development of digital industries has squeezed out carbon-intensive industries, optimized the industrial structure, and reduced carbon emissions. Yu et al. (2022) believes that the application of digital technology has greatly improved production conditions, optimized factors other than energy input, and helped to reduce carbon emissions. However, some scholars (Salahuddin and Alam, 2015; Avom et al., 2020) believe that digital transformation will increase the demand for energy sources such as electricity, which will lead to an increase in carbon emissions.
Although scholars have conducted extensive research on the economic and environmental effects of digital transformation from a regional or corporate perspective, few studies have explored the relationship between digital transformation and environmental performance from a combined regional and industry perspective, which hinders a comprehensive understanding of the impacts of digital transformation. Therefore, this paper extends the existing research as follows: 1) This paper explores the impact of digital transformation on low-carbon development of manufacturing industry from the perspective of sub-region and sub-industry. 2) Using matching data to measure the low-carbon development level of manufacturing industry in China’s provincial-level. This avoids measurement errors caused by ignoring the heterogeneity of regions or industries. 3) By combining the multi-regional input-output model with the evaluation system of digital economy development level, the measurement framework of the digital transformation of manufacturing industry in various provinces of China is constructed, which enriches the measurement research of digital transformation.
2 Theoretical analysis
Digital transformation refers to the process by which enterprises apply digital technologies such as networks, communications, and computing to transform organizational structures and business models to achieve workflow optimization, organizational efficiency improvement, and value creation (Vial, 2019). As a revolution, digital transformation may fundamentally change the structure and trading mode of production factors, which will have an important impact on production efficiency and ecological environment (Goldfarb et al., 2015; Verhoef et al., 2019).
2.1 Direct mechanism
The deep integration of digital technologies such as big data and traditional manufacturing industry can promote the low-carbon development of manufacturing industry by eliminating the information gap, achieving accurate matching of supply and demand, and adapting to the market environment (Wu et al., 2022). The acceleration of the digitization process has spawned a variety of information service platforms, which have profoundly changed the information search mode and resource allocation mode of market participants. The digital platform gradually reduces the information asymmetry in the field of resource allocation by aggregating massive resource demand information, which is conducive to the supply and demand sides to grasp each other‘s real needs in an instant and efficient manner, thereby improving resource utilization efficiency (Kaija et al., 2022). Producers use data mining technology to analyze consumer demand preferences, carry out targeted production activities, and form a dynamic and accurate matching mechanism between supply and demand, thereby reducing unnecessary waste in production.
Although the scale effect of digital transformation will lead to an increase in energy demand (Moyer and Hughes, 2012; Lange et al., 2020), the rapid penetration of digital technology profoundly affects the supply-demand structure and utilization efficiency of energy (Goldbach et al., 2018). The carbon reduction caused by the adjustment of energy consumption structure, the matching of energy supply-demand, and the improvement of energy efficiency is greater than the carbon increase caused by the expansion of production scale, which makes the “net” impact of digital transformation on carbon emissions show an inhibitory effect (Zhang Z et al., 2022). From the perspective of structural adjustment, the application of digital technology can strengthen the substitution role of clean energy for fossil energy, reduce the dependence of the industry on fossil energy. In addition, the application of digital technology has laid a technical foundation for the research and development and promotion of clean energy, which is conducive to changing the production mode of the industry based on fossil energy consumption. From the perspective of supply-demand matching, digital transformation is conducive to improving the coordinated and matching of energy supply side and demand side (Kaija et al., 2022). The application of digital technology makes it easy to collect and process information. Producers can use the information they have to judge the supply and demand of energy to match supply and demand (Goldbach et al., 2018). Improving energy efficiency is another effective way to achieve carbon emission reduction (Yi et al., 2022). On the one hand, the application of digital technology and data resources has spawned new technologies and formats related to energy production, helping to improve industrial energy efficiency. On the other hand, digital transformation can promote the penetration of digital technology into the enterprise’s energy scheduling system, which will help realize the efficient operation of procurement, storage and management of energy, and then promote the low-carbon development of industry (Zhang et al., 2023). Based on the above analysis, this paper proposes the research hypothesis.
H1:. The digital transformation has a positive effect on low-carbon development in manufacturing.
2.2 Indirect mechanism
Technological innovation is an effective way to achieve economic growth and protect the environment (Daron et al., 2012). The improvement of technology is conducive to cleaner production for enterprises, which has a positive effect on achieving carbon emission reduction (Leung et al., 2014; Xu et al., 2021). In theory, using digital technologies to improve production and management processes can have a positive impact on innovation (Nambisan et al., 2019; Ning et al., 2022). From Schumpeter’s explanation of innovation (Schumpeter, 1934), the essence of innovation lies in the recombination of elements. Digitization accelerates the construction of modern information communication networks. Data, knowledge and information, as the key innovation elements, are rapidly spread and applied through communication network technology. It is more convenient for enterprises to obtain heterogeneous innovation elements and realize knowledge linkage than before. In addition, the widespread application of digital technology facilitates the flow of knowledge and information between internal and external enterprises, which is conducive to breaking down invisible barriers to innovation (Niu et al., 2023). Digital transformation not only promotes the diffusion of innovation elements, but also gives birth to more innovation elements. The application of digital technologies such as big data and cloud computing enables the storage and analysis of data, knowledge and information. The accumulation of innovative resources provides favorable conditions for low-carbon technology innovation. Based on the above analysis, this paper proposes the research hypothesis.
H2:. Digital transformation improves the low-carbon development level of manufacturing industry by promoting the mechanism of technological innovation.
3 Measurement of core variable
3.1 Low-carbon development level
The existing literature points out that the low-carbon production efficiency calculated by taking carbon emissions as undesired output, regional GDP as expected output, labor, capital and energy as production factors can not only reflect the efficiency of economic output, but also take into account the problem of carbon emissions, which can better measure the extent to which the development model meets the dual goals of economic growth and energy conservation and emission reduction (Chen and Golley, 2014).
3.1.1 Method
The traditional Data Envelopment Analysis (DEA) calculation method does not consider the slack variables, and most of them are angle and radial models. There are problems such as the incomparability of decision making units on the efficiency Frontier (Andersen and Petersen, 1993) and the same proportion of input or output changes (Tone, 2001). Therefore, this paper selects the Super-SBM model, which is improved by Tone on the basis of its non-radial and non-angle SBM model (Tone, 2002), and fully takes into account the scale reward problem, selecting the more realistic variable returns to scale (VRS). In addition, the carbon emission constraint is treated as undesirable output, and the Super-SBM model considering undesirable output is constructed. The model relaxes the constraints of the same proportion change of each factor and the effective decision-making unit efficiency value
Specifically, assuming that there are
In the formula,
3.1.2 Indicators and data
This paper uses gross industrial output as the expected output index, carbon emissions as the unexpected output index; input indicators are general, including capital, labor and energy consumption. To estimate the total industrial output value data from 2012 to 2018, the industrial sales output value, the current year inventory and the previous year inventory are taken into account. Then, according to the producer price index of industrial products divided by provinces and industries in each year, the data was deflated to the comparable industrial output value based on 2012 as the base year. Capital investment is measured by the capital stock of the manufacturing industry in each province. This year’s capital stock is calculated according to the perpetual inventory method. The composition of energy consumption includes 20 energy types such as coal, oil, natural gas and electricity. Because the average low calorific value of each type of energy is not the same, it can not be directly added. Therefore, the reference coefficients of various types of energy converted into standard coal provided by the “China Energy Consumption Statistical Yearbook” are used to convert units of different energy types into 10,000 tons of standard coal and add them.
The original data of expected output, capital input and labor input required for the measurement of low-carbon development level of manufacturing industry are from the “China Industrial Statistical Yearbook”. The original data of unexpected output and energy consumption are from the CEADs database. The price deflator data is from the “China Price Statistical Yearbook”.
3.1.3 Results and analysis
This paper uses Matlab 2020b software to calculate the low-carbon development level of 17 manufacturing industries in 30 provinces of China from 2012 to 2018, and analyzes its evolution characteristics from the national level, regional level and industry level. As shown in Figure 1, from 2012 to 2018, the low-carbon development level of manufacturing industry in China and its regions showed an upward trend. According to the changing characteristics of low-carbon development level of manufacturing industry, the research interval can be divided into two stages. During the first stage from 2012 to 2015, the low-carbon development level of the manufacturing industry increased at a relatively low rate, and the growth trend was not obvious. The second stage is from 2016 to 2018. During this period, the low-carbon development level of China’s manufacturing industry showed a rapid upward trend, and the increase was obvious. The reason for this change trend may be that 2012–2015 is the early stage of China’s low-carbon transformation and development. Because the economic development model has a certain path dependence effect, the effect of low-carbon development in the short term is not significant. In addition, China’s digital transformation during this period is still in its infancy, and the digital economy and the real economy have not achieved deep integration, which makes the low-carbon development level of manufacturing industry grow more slowly. With the deepening of the concept of low-carbon development and the deep integration of digital economy and real economy, the low-carbon transformation kinetic energy accumulated in the early stage has been released, and the low-carbon development level of manufacturing industry has been rapidly improved.
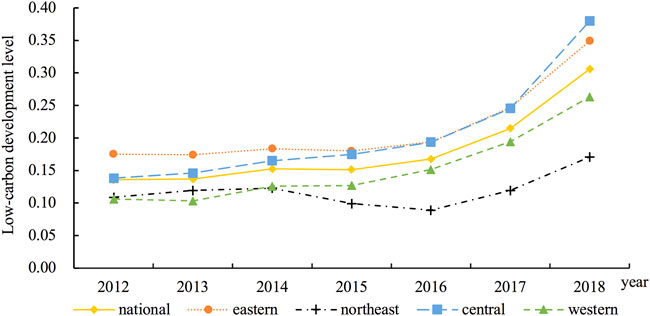
FIGURE 1. The evolution trend of the average value of low-carbon development of manufacturing industry in china and its four regions.
From the perspective of regional differences, the low-carbon development level of manufacturing industry in the eastern and central regions is relatively close, which is higher than the national average; the level of low-carbon development in the western region has greatly improved, but there is still a big gap with the eastern and central regions. The low-carbon development level of manufacturing in northeast is not only lower than the eastern and central regions, but also gradually lags behind the western region, and the gap with other regions gradually widened. This regional difference is highly correlated with China’s economic development, industrial layout and spatial distribution of resources.
Further, analyze the changes in the level of low-carbon development of China’s manufacturing industry from an industry perspective. Table 1 lists the calculation results for 2012, 2015, and 2018. On the whole, the low-carbon development level of each manufacturing industry is on the rise. From the perspective of industry differences, food and tobacco, communications electronic equipment and electrical machinery and equipment in the three industries of low-carbon development level in each year ranked high, and Paper printing cultural education sports, metal smelting and non-metallic products low-carbon development level ranked low. It can be found that industries with high levels of low-carbon development are mostly low-energy-consuming industries. These industries for energy dependence is not strong, low-carbon development action less resistance. Most of the industries with low low-carbon development levels belong to traditional manufacturing industries with high energy consumption and high pollution. Such industries have high demand for energy and many have overcapacity problems, so the level of low-carbon development is low. This industry difference shows that the traditional high-pollution and high-energy-consuming manufacturing industry is still the key industry of China’s low-carbon reform, and improving the low-carbon development level of such industries plays a key role in achieving the “dual-carbon goals” and promoting the high-quality development of the manufacturing industry.
3.2 Digital transformation
From the literature on digital economy measurement, most studies measure the level of digital economy development at the national and regional levels or the degree of digital transformation at the enterprise level. Scholars usually use input-output tables or macroeconomic indicators to measure the level of digital economic development including national, provincial and urban dimensions (Balcerzak and Pietrzak, 2017; Liu et al., 2022; Zhang C et al., 2022). Or use text analysis to measure the degree of digital transformation at the enterprise level (Feng et al., 2022), and a small number of studies have measured the degree of digital transformation at the industry level. These studies can reflect the development of digital economy or digital transformation in China to some extent. However, measuring the degree of digital transformation from the regional level or the industry level alone will lead to deviations in the measurement of digital transformation. Out of self-interest motivation, enterprises exaggerate the disclosure of digital related words, which will lead to distortion of digital measurement at the enterprise level. In view of this, this paper constructs a new measurement model of digital transformation degree.
3.2.1 Measurement model and data
The degree of digital transformation of manufacturing in different regions depends not only on the intensity of industry digital input, but also on the development of regional digital economy. Therefore, drawing on the research ideas of Arnold et al. (Arnold et al., 2016), using China’s multi-regional input-output model, combined with the measurement system of digital economy development level of each province, this paper constructs a framework for measuring the digital transformation degree of manufacturing industry in different provinces in China. The benchmark calculation formula is:
In the formula,
This paper uses input-output method to measure the digital input intensity of manufacturing industry. Industry digital input intensity is the proportion of industry digital intermediate input in total input. Among them, the digital intermediate input part includes direct digital intermediate input and complete digital intermediate input. In the case of only considering direct digital intermediate input, the calculation expression of digital input intensity is:
where
Where
The data of digital input intensity are derived from China’s multi-regional input-output table in 2012, 2015, and 2017 released by CEADs database. China’s multi-regional input-output table contains 31 provinces and 42 economic sectors. Some sectors related to the digital economy only have some digital content, so this paper constructs the digital industry stripping coefficient to separate the digital content part. The digital industry stripping coefficient is the proportion of digital output in the total output of the industry containing digital content. The formula is expressed as:
Among them,
According to the definition of the core industries of the digital economy in the “Statistical Classification of Digital Economy and Its Core Industries (2021)”, this paper constructs a measurement system for the development level of digital economy from three dimensions: digital industrialization, industrial digitization and digital economic development environment (Zhang J et al., 2022; Lyu et al., 2023), as shown in Table 2. The marketization index data in the sample are derived from the “China Provincial Marketization Index Report (2021)”, the Digital Inclusive Finance Index is derived from the “Peking University Digital Inclusive Finance Index (2011–2020)”, and other data are derived from the “China Statistical Yearbook”, “China Information Yearbook” and the CSMAR digital economy database. In order to avoid the subjectivity of the evaluation results and fully reflect the original information of the data, the entropy method is used to measure the level of digital economy development at the provincial level.
3.2.2 Results and analysis
According to the measurement model constructed above, the degree of digital transformation of manufacturing industry in China’s provinces from 2012 to 2018 is measured. Figure 2 shows the changes in the degree of digital transformation of manufacturing industry in the whole country and its four major regions. Overall, from 2012 to 2018, the degree of digital transformation of manufacturing in various regions of the country is on the rise. In terms of time nodes, 2012–2015 is a period of rapid growth of digital transformation of manufacturing industry in various regions. This period is a period of rapid integration of digital technology and real economy. The demand for digital input in manufacturing industry is strong, and the degree of digital transformation shows rapid growth. From 2016 to 2018, it was a stage of fluctuating growth. During this period, the growth rate of digital transformation of manufacturing industry slowed down, and it decreased slightly in 2017. The reason for this trend may be that the scale dividend in the early stage of digital transformation of manufacturing industry gradually disappeared, the transformation entered a mature stage of development, and the demand for digital input was relatively stable.
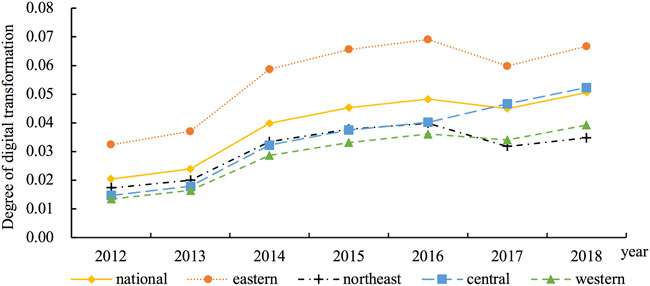
FIGURE 2. Evolution trend of average degree of digital transformation of manufacturing industry in china and four regions.
From the perspective of regional differences, the eastern region has the highest degree of digital transformation of manufacturing industry, which has remained above the national average. The degree of digital transformation of manufacturing industry in the central, western and northeastern regions is similar and lower than the national average. It is worth noting that the digital transformation gap between the eastern and central regions and the western and northeastern regions has been expanding year by year, which to some extent reflects the “digital divide” phenomenon caused by unbalanced regional development in the digital era.
Table 3 shows the average degree of digital transformation at the two-digit industry level in 2012, 2015, and 2018. On the whole, from 2012 to 2018, the degree of digital transformation in China’s manufacturing industries is on the rise. From the perspective of industry differences, communication electronic equipment, instrumentation and electrical machinery and equipment are the industries with the highest degree of digital transformation, which are mostly high-end manufacturing industries with low energy consumption and obvious technical characteristics. Petroleum processing, metal smelting, food and tobacco are industries with low degree of digital transformation. Most of these industries are traditional manufacturing industries. The traditional production mode is relatively solid, the pace of digital transformation is relatively slow, and the degree of transformation is low. This industry difference shows that it is urgent to promote the digital transformation of traditional manufacturing industry.
4 Empirical design
4.1 Empirical model
This paper uses high-dimensional panel data at the provincial and industry levels in China from 2012 to 2018 to empirically study the impact of digital transformation on low-carbon development of manufacturing. The high-dimensional fixed effect model is constructed as follows:
Among them,
4.2 Variables
The explanatory variable is the level of low-carbon development in manufacturing (
4.3 Data sources
The sample period of this paper is 2012–2018, and 17 two-digit manufacturing industries in 30 provinces in China are selected for the study. The control variable data comes from “China Statistical Yearbook”, “China Industrial Statistical Yearbook”, “China Economic Census Yearbook”, “China Science and Technology Statistical Yearbook”, provincial statistical yearbooks and CEADs database. Variable data are provided in the Supplementary Table S1. The descriptive statistics of the main variables are shown in Table 4.
5 Empirical results and analysis
5.1 Benchmark regression results
Considering that industry differences, regional differences and time factors may have an impact on the estimation results, this paper uses high-dimensional fixed effects model for parameter estimation. The benchmark regression results are shown in Table 5. Among them, column 1) is the estimation result without control variables, and the estimation coefficient of the core explanatory variable is significantly positive at the 5% level. Column 2) is the estimated result of adding control variables. The estimated coefficient of digital transformation is still significantly positive and can reject the null hypothesis at the 1% level. The above results show that digital transformation can significantly promote the low-carbon development level of manufacturing industry. H1 of this paper is verified. Digital transformation can strengthen the synergy between the upstream and downstream of the industrial chain and reduce unnecessary losses in the production process, which has a positive impact on promoting low-carbon development.
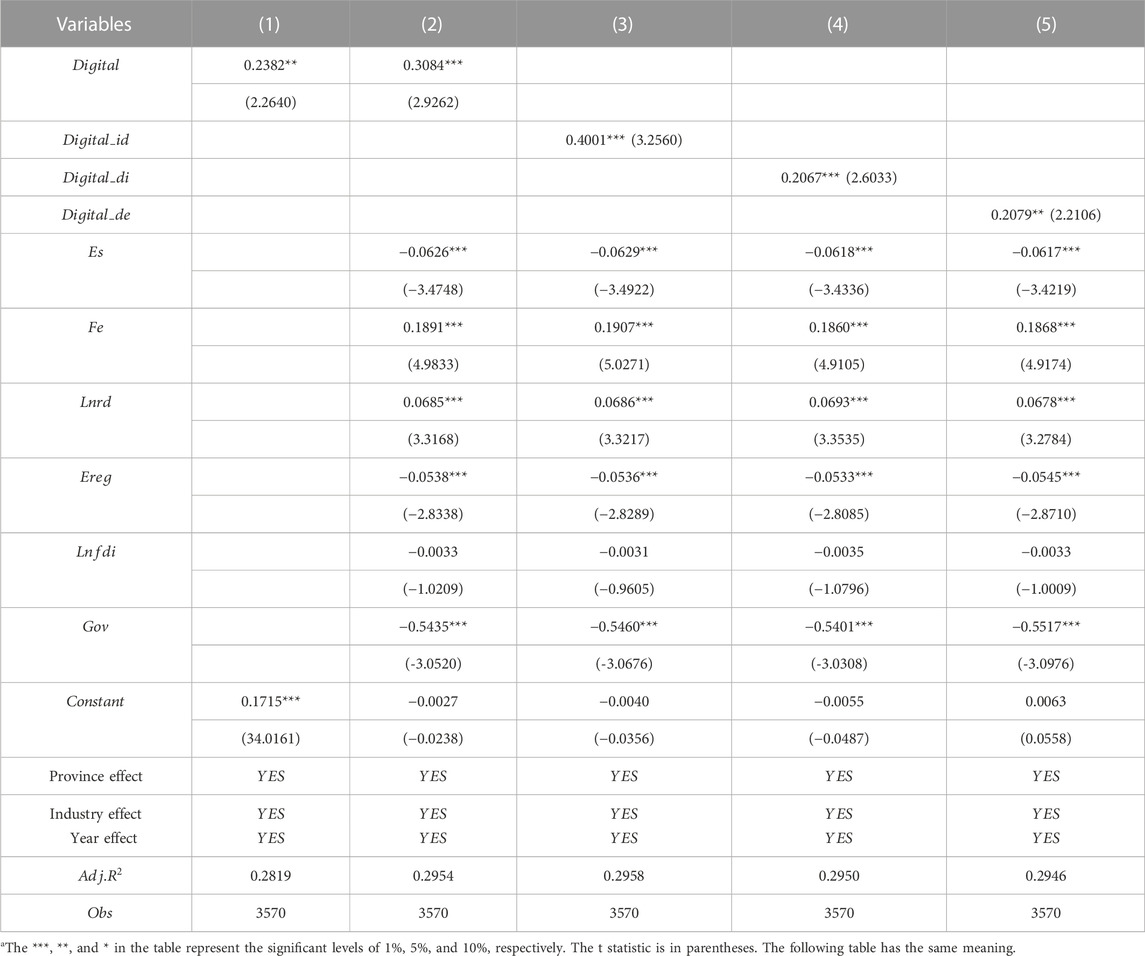
TABLE 5. Results of benchmark regression and further analysisa.
The control variable symbol is consistent with expectations. The impact coefficient of energy consumption structure on low-carbon development was significantly negative at the level of 1%, indicating that the excessive proportion of coal energy consumption was not conducive to the improvement of low-carbon development level of the manufacturing industry. The structure of production factors has a significant role in promoting the low-carbon development of manufacturing industry. The regression coefficient of R&D investment is positive, indicating that increasing R&D investment in manufacturing can help its low-carbon development. The impact of government intervention on low-carbon development is negative, which may be because excessive government intervention in the market harms the level playing field. The regression coefficient of environmental regulation is positive, indicating that environmental regulation can promote the low-carbon development of manufacturing industry. The regression coefficient of openness is negative, but the result is not significant, indicating that foreign investment has not effectively promoted the low-carbon development level of manufacturing industry.
5.2 Further analysis
Exploring the impact of sub-indicators of digital transformation on the low-carbon development of manufacturing industry is of great significance for optimizing the digital transformation strategy to give full play to its low-carbon effect. This paper divides the development level of regional digital economy into three dimensions: digital industrialization (
5.3 Robustness test
5.3.1 Replace the key variable measurement method
First, the SBM model is used to recalculate the low-carbon development level of manufacturing industry. Second, the measurement method of replacing the core explanatory variables. The degree of digital transformation (
5.3.2 Replace the measurement indicators of low-carbon development level
The connotation of low-carbon development of manufacturing industry is rich, and excessive reliance on single indicators will inevitably make the research conclusion one-sided. Therefore, drawing on the measurement of low-carbon development level in existing literature, single-factor low-carbon production efficiency (i.e., output per unit of carbon emissions) and carbon emission intensity are used as indicators of low-carbon development level of manufacturing industry to re-estimate the benchmark model (Kaya and Yokobori, 1997). The results are shown in columns (3)–(4) of Table 6.
5.3.3 Processing extreme values
OLS estimation method is susceptible to extreme values. If there are extreme values in the data set, it will cause the regression curve to shift in the direction of extreme values, making the estimated results deviate from the real situation. Therefore, the bilateral extreme values of all variables are indented according to the 5% and 95% quantiles, respectively, and the parameters are re-estimated. The estimation results are shown in column (5) of Table 6.
The above robustness test results show that the positive impact of digital transformation on low-carbon development is still significant, indicating that the core conclusions of this paper are robust.
5.4 Endogenous treatment
Effectively controlling endogeneity is key to accurately identifying the causal relationship between digital transformation and low-carbon development. First of all, this paper attempts to use digital transformation lag phase I, lag phase II and lag phase III as the instrumental variables of the current digital transformation. China’s industrial digital transformation often has the characteristics of top-down and step-by-step, so the current digital process is rooted in the previous accumulation. At the same time, the current low-carbon development level will not interfere with the previous digital process. This satisfies the exogeneity and relevance criteria for instrumental variable selection. Columns (1)–(3) in Table 7 show the estimation results of two-stage least squares (2SLS). Anderson test and Cragg-Donald test show that the model does not have the problem of unidentifiable and weak instrumental variables, indicating that the instrumental variables are effective. The regression coefficients of digital transformation are significantly positive, indicating that digital transformation can still effectively promote the low-carbon development of manufacturing industry after dealing with potential endogenous problems.
In addition, the instrumental variable construction method proposed by Lewbel has been widely used in existing research (Lewbel, 1997). Miruna (2022) used this idea to construct the instrumental variables of Industry 4.0. Wang Q et al. (2022) used this method to construct instrumental variables of the degree of digitization at the provincial level in China. The above research verifies the effectiveness of this instrumental variable construction method. Therefore, this paper refers to Lewbel’s idea, using the digital transformation variable and the corresponding industry digital transformation mean difference of three power as the instrumental variable of digital transformation (
Further, this paper uses the generalized moment estimation method (GMM) to alleviate the endogenous bias caused by problems such as two-way causality and missing variables. Considering that the difference generalized moment estimation method (DIF-GMM) still has the problem of weak instrumental variables, and the two-step estimation is more effective than the one-step estimation, this paper uses the two-step system generalized moment estimation method (SYS-GMM) to deal with endogeneity. The estimation results of the two-step SYS-GMM are shown in column (5) of Table 7. Among them, the Arellano-Bond test and the Hansen test show that the instrumental variables are valid and that the model does not have over-identification problems and satisfies the two-step SYS-GMM usage conditions (Arellano and Bover, 1995; Blundell and Bond, 1998). From the estimation results, the coefficient of the core explanatory variable is significantly positive at the 5% level. The two-step SYS-GMM regression results are basically consistent with the benchmark regression results, which verifies the robustness of the basic conclusions of this paper.
5.5 Heterogeneity analysis
5.5.1 Heterogeneity of regional economic development level
Heterogeneity analysis based on regional economic development level. In order to explore the impact of digital transformation on the low-carbon development of manufacturing industry in different economic development levels. In this paper, the average per capita GDP of each region is used as the standard, and the whole sample is divided into developed regions and underdeveloped regions and makes regression respectively. From the regression results in Table 8, digital transformation has a significant positive impact on the low-carbon development of the manufacturing industry, whether in developed regions or underdeveloped regions, but the magnitude of the impact is different. The impact of digital transformation in underdeveloped areas on the low-carbon development of manufacturing industry is higher than that in developed areas. This may be due to the fact that the manufacturing industry in developed regions is in a higher stage of development, the low-carbon production level itself is high, and the role of digital empowerment in its low-carbon development is relatively limited, while the low-carbon production capacity in underdeveloped regions is relatively weak, with greater room for improvement, and the effect of digital empowerment is higher than that in developed regions.
5.5.2 Heterogeneity of industrial energy consumption level
Heterogeneity analysis based on industry energy consumption types. There are great differences in energy consumption among different manufacturing industries. Therefore, according to the “2010 National Economic and Social Development Statistical Report”, this paper divides 17 manufacturing industries into high-energy-consuming industry groups and medium-low-energy-consuming industries groups for heterogeneity analysis. From the results of Table 8, it can be found that digital transformation has a positive impact on low-carbon development in both high-energy-consuming industries and medium-low-energy-consuming industries, but the regression results of high-energy-consuming industries are not significant. There may be two reasons: First, the degree of digital transformation of high-energy-consuming industries is relatively low, and the energy-saving effect, technological innovation effect and resource allocation optimization effect of digital transformation have not yet been formed. Second, high-energy-consuming industries are often traditional manufacturing sectors, many factors restricting green development, may lead to the promotion of digital transformation is offset.
5.6 Mechanism test
According to theoretical analysis, digital transformation promotes low-carbon development of manufacturing industry through technological innovation effect. This paper constructs a mediation model to test this mechanism. The model is constructed as follows:
Among them,
The mechanism test results are shown in Table 9, where column 1 shows that digital transformation has a significant role in promoting technological innovation and passes the 1% significance test. Column 2 shows that both digital transformation and technological innovation have significantly promoted low-carbon development and passed the 10% significance test. The above results show that digital transformation promotes low-carbon development of manufacturing industry through technological innovation effect. H2 of this paper is verified. Low-carbon development is closely related to technological innovation. Digital transformation can promote low-carbon technology innovation by diffusing innovation elements, which in turn can promote low-carbon development.
6 Conclusion
Using the matching data of China Industrial Economy Database, CEADs Database and China‘s multi-regional input-output table, this paper constructs a measurement framework for the low-carbon development level and digital transformation degree of 17 manufacturing industries in 30 provinces in China, and conducts an empirical study on the relationship between digital transformation and low-carbon development from the perspective of sub-regions and sub-industries. The results show that:
(1) The low-carbon development level of China‘s manufacturing industry is increasing year by year, but the development gap between regions and industries is large. At the regional level, the development level of the eastern and central regions is higher, and the development level of the western and northeastern regions is lower. At the industry level, the development level of medium-low-energy-consuming industries is higher, while that of high-energy-consuming industries is lower.
(2) The degree of digital transformation of China‘s manufacturing industry is on the rise, but there is an imbalance between regions and industries. At the regional level, the eastern region has a higher degree of transformation, while the central, western and northeastern regions have lagged behind. At the industry level, the high-end manufacturing industry has a higher degree of transformation, while the traditional manufacturing industry has a lower degree of transformation.
(3) The digital transformation of the manufacturing industry has a significant role in promoting its low-carbon development, and this conclusion still holds after the robustness tests such as changing the measurement method of variables, replacing core variables, dealing with extreme values and considering endogeneity.
(4) For sub-indicators, digital industrialization, industrial digitization and digital development environment can significantly promote the low-carbon development of manufacturing industry. Among them, the impact of digital industrialization is the most obvious.
(5) The impact of digital transformation of manufacturing on its low-carbon development is heterogeneous across regions and industries. The low-carbon effect of digital transformation in underdeveloped areas is higher than that in developed areas. The low-carbon effect of digital transformation in medium-low-energy-consuming industries is obvious, but the low-carbon effect of digital transformation in high-energy-consuming industries has not appeared.
(6) The mechanism test results show that technological innovation is an important channel for digital transformation to promote the low-carbon development of the manufacturing industry.
The policy orientation of the research conclusion is clear. The government should actively affirm the environmental performance of digital transformation and create a good external environment for the digital transformation of manufacturing industry. On the one hand, the government should continue to increase investment in digital infrastructure to provide support for the digital transformation of the manufacturing industry. On the other hand, the government should actively promote cooperation between enterprises and universities, scientific research institutions and other institutions, establish a technology exchange platform, open up digital technology application channels, and accelerate the application of digital technology. In addition, the differences in digital transformation in different regions and industries should be taken seriously to avoid further widening the gap. By formulating differentiated support policies, providing policy support for backward areas can narrow the development gap. At the same time, the construction of big data platform can provide more adequate data resource services for traditional manufacturing enterprises, which is conducive to the digital transformation of enterprises.
Data availability statement
The original contributions presented in the study are included in the article/Supplementary Material, further inquiries can be directed to the corresponding authors.
Author contributions
YL: Conceptualization, methodology, resources, validation. LZ: Data curation, Software, writing-original draft preparation, visualization. DW: Writing-reviewing and editing, supervision.
Funding
This work was supported by the National Social Science Foundation of China (Grant No. 18BJY006) and Fujian Social Science Foundation Project (Grant No. FJ2022B081).
Conflict of interest
The authors declare that the research was conducted in the absence of any commercial or financial relationships that could be construed as a potential conflict of interest.
Publisher’s note
All claims expressed in this article are solely those of the authors and do not necessarily represent those of their affiliated organizations, or those of the publisher, the editors and the reviewers. Any product that may be evaluated in this article, or claim that may be made by its manufacturer, is not guaranteed or endorsed by the publisher.
Supplementary material
The Supplementary Material for this article can be found online at: https://www.frontiersin.org/articles/10.3389/fenvs.2023.1134882/full#supplementary-material
References
Andersen, P., and Petersen, N. C. (1993). A procedure for ranking efficient units in data envelopment analysis. Manage. Sci. 39, 1261–1264. doi:10.1287/mnsc.39.10.1261
Arellano, M., and Bover, O. (1995). Another look at the instrumental variable estimation of error-components models. J. Econom. 68, 29–51. doi:10.1016/0304-4076(94)01642-D
Arnold, J. M., Javorcik, B., Lipscomb, M., and Mattoo, A. (2016). Services reform and manufacturing performance: Evidence from India. Econ. J. 126, 1–39. doi:10.1111/ecoj.12206
Avom, D., Nkengfack, H., Fotio, H. K., and Totouom, A. (2020). ICT and environmental quality in Sub-Saharan Africa: Effects and transmission channels. Technol. Forecast. Soc. 155, 120028. doi:10.1016/j.techfore.2020.120028
Balcerzak, A. P., and Pietrzak, M. B. (2017). Digital economy in Polish regions. Proposal of measurement via TOPSIS with generalized distance measure GDM. Working Papers.
Ballestar, M. T., Camiña, E., Díaz-Chao, Á., and Torrent-Sellens, J. (2021). Productivity and employment effects of digital complementarities. J. Innov. Knowl. 6, 177–190. doi:10.1016/j.jik.2020.10.006
Bhimani, A. (2015). Exploring big data's strategic consequences. J. Inf. Technol. 30, 66–69. doi:10.1057/jit.2014.29
Blundell, R., and Bond, S. (1998). Initial conditions and moment restrictions in dynamic panel data models. J. Econom. 87, 115–143. doi:10.1016/S0304-4076(98)00009-8
Chen, P. (2022). Is the digital economy driving clean energy development? New evidence from 276 cities in China. J. Clean. Prod. 372, 133783. doi:10.1016/j.jclepro.2022.133783
Chen, S., and Golley, J. (2014). Green' productivity growth in China's industrial economy. Energy Econ. 44, 89–98. doi:10.1016/j.eneco.2014.04.002
Daron, A., Philippe, A., Leonardo, B., and David, H. (2012). The environment and directed technical change. Am. Econ. Rev. 102, 131–166. doi:10.1257/aer.102.1.131
El-Kassar, A., and Singh, S. K. (2019). Green innovation and organizational performance: The influence of big data and the moderating role of management commitment and HR practices. Technol. Forecast. Soc. 144, 483–498. doi:10.1016/j.techfore.2017.12.016
Feng, H., Wang, F., Song, G., and Liu, L. (2022). Digital transformation on enterprise green innovation: Effect and transmission mechanism. Int. J. Environ. Res. Public Health 19, 10614. doi:10.3390/IJERPH191710614
Gaglio, C., Kraemer-Mbula, E., and Lorenz, E. (2022). The effects of digital transformation on innovation and productivity: Firm-level evidence of South African manufacturing micro and small enterprises. Technol. Forecast. Soc. 182, 121785. doi:10.1016/j.techfore.2022.121785
Ge, W., Xu, Y., Liu, G., Shen, B., Su, X., Liu, L., et al. (2022). Exploring the impact of the digital economy on carbon emission efficiency under factor misallocation constraints: New insights from China. Front. Environ. Sci. 10, 953070. doi:10.3389/FENVS.2022.953070
Goldbach, K., Rotaru, A. M., Reichert, S., Stiff, G., and Gölz, S. (2018). Which digital energy services improve energy efficiency? A multi-criteria investigation with European experts. Energy Policy 115, 239–248. doi:10.1016/j.enpol.2017.12.036
Goldfarb, A., Greenstein, S. M., and Tucker, C. E. (2015). Economic analysis of the digital economy. Chicago, America: University of Chicago Press.
Kaija, V., Ralf-Martin, S., and Einari, K. (2022). Implications of digitalization in facilitating socio-technical energy transitions in Europe. Energy Res. Soc. Sci. 91, 102720. doi:10.1016/J.ERSS.2022.102720
Kaya, Y., and Yokobori, K. (1997). Environment, energy and economy: Strategies for sustainability. Tokyo, Japan: United Nations University Press.
Lange, S., Pohl, J., and Santarius, T. (2020). Digitalization and energy consumption. Does ICT reduce energy demand? Ecol. Econ. 176, 106760. doi:10.1016/j.ecolecon.2020.106760
Leung, D. Y. C., Caramanna, G., and Maroto-Valer, M. M. (2014). An overview of current status of carbon dioxide capture and storage technologies. Renew. Sustain. Energy. Rev. 39, 426–443. doi:10.1016/j.rser.2014.07.093
Lewbel, A. (1997). Constructing instruments for regressions with measurement error when no additional data are available, with an application to patents and R&D. Econometrica 65, 1201. doi:10.2307/2171884
Li, G., and Liao, F. (2022). Input digitalization and green total factor productivity under the constraint of carbon emissions. J. Clean. Prod. 377, 134403. doi:10.1016/j.jclepro.2022.134403
Li, J., and Lin, B. (2017). Does energy and CO2 emissions performance of China benefit from regional integration? Energy Policy 101, 366–378. doi:10.1016/j.enpol.2016.10.036
Liu, Y., Yang, Y., Li, H., and Zhong, K. (2022). Digital economy development, industrial structure upgrading and green total factor productivity: Empirical evidence from China's cities. Int. J. Environ. Res. Public Health 19, 2414. doi:10.3390/IJERPH19042414
Luo, K., Liu, Y., Chen, P., and Zeng, M. (2022). Assessing the impact of digital economy on green development efficiency in the Yangtze River Economic Belt. Energy Econ. 112, 106127. doi:10.1016/j.eneco.2022.106127
Lyu, Y., Wang, W., Wu, Y., and Zhang, J. (2023). How does digital economy affect green total factor productivity? Evidence from China. Sci. Total Environ. 857, 159428. doi:10.1016/j.scitotenv.2022.159428
Mićić, L. (2017). Digital transformation and its influence on GDP. Economics 5, 135–147. doi:10.1515/eoik-2017-0028
Miruna, S. (2022). The impact of industry 4.0 on innovation performance: Insights from German manufacturing and service firms. Technovation 113, 102415. doi:10.1016/j.technovation.2021.102415
Moyer, J. D., and Hughes, B. B. (2012). ICTs: Do they contribute to increased carbon emissions? Technol. Forecast. Soc. 79, 919–931. doi:10.1016/j.techfore.2011.12.005
Nambisan, S., Wright, M., and Feldman, M. (2019). The digital transformation of innovation and entrepreneurship: Progress, challenges and key themes. Res. Policy 48, 103773. doi:10.1016/j.respol.2019.03.018
Ning, J., Yin, Q., and Yan, A. (2022). How does the digital economy promote green technology innovation by manufacturing enterprises? Evidence from China. Front. Environ. Sci. 10, 967588. doi:10.3389/FENVS.2022.967588
Niu, Y., Wen, W., Wang, S., and Li, S. (2023). Breaking barriers to innovation: The power of digital transformation. Financ. Res. Lett. 51, 103457. doi:10.1016/J.FRL.2022.103457
Pan, W., Xie, T., Wang, Z., and Ma, L. (2022). Digital economy: An innovation driver for total factor productivity. J. Bus. Res. 139, 303–311. doi:10.1016/j.jbusres.2021.09.061
Peng, C., Jia, X., and Zou, Y. (2022). Does digitalization drive corporate green transformation? based on evidence from Chinese listed companies. Front. Environ. Sci. 10, 963878. doi:10.3389/FENVS.2022.963878
Ren, S., Hao, Y., Xu, L., Wu, H., and Ba, N. (2021). Digitalization and energy: How does internet development affect China's energy consumption? Energy Econ. 98, 105220. doi:10.1016/j.eneco.2021.105220
Salahuddin, M., and Alam, K. (2015). Internet usage, electricity consumption and economic growth in Australia: A time series evidence. Telemat. Inf. 32, 862–878. doi:10.1016/j.tele.2015.04.011
Schumpeter, J. A. (1934). The theory of economic development: An inquiry into profits, capital, credit, interest, and the business cycle cambridge, britain. Cambridge, Mass: Harvard University Press.
Tone, K. (2001). A slacks-based measure of efficiency in data envelopment analysis. Eur. J. Oper. Res. 130, 498–509. doi:10.1016/S0377-2217(99)00407-5
Tone, K. (2002). A slacks-based measure of super-efficiency in data envelopment analysis. Eur. J. Oper. Res. 143, 32–41. doi:10.1016/S0377-2217(01)00324-1
Verhoef, P. C., Broekhuizen, T., Bart, Y., Bhattacharya, A., Dong, J. Q., Fabian, N., et al. (2019). Digital transformation: A multidisciplinary reflection and research agenda. J. Bus. Res. 122, 889–901. doi:10.1016/j.jbusres.2019.09.022
Vial, G. (2019). Understanding digital transformation: A review and a research agenda. J. Strateg. Inf. Syst. 28, 118–144. doi:10.1016/j.jsis.2019.01.003
Wang, J., Dong, K., Sha, Y., and Yan, C. (2022). Envisaging the carbon emissions efficiency of digitalization: The case of the internet economy for China. Technol. Forecast. Soc. 184, 121965. doi:10.1016/j.techfore.2022.121965
Wang, Q., Hu, A., and Tian, Z. (2022). Digital transformation and electricity consumption: Evidence from the Broadband China pilot policy. Energy Econ. 115, 106346. doi:10.1016/j.eneco.2022.106346
Wu, B., and Yang, W. (2022). Empirical test of the impact of the digital economy on China's employment structure. Financ. Res. Lett. 49, 103047. doi:10.1016/j.frl.2022.103047
Wu, L., Sun, L., Chang, Q., Zhang, D., and Qi, P. (2022). How do digitalization capabilities enable open innovation in manufacturing enterprises? A multiple case study based on resource integration perspective. Technol. Forecast. Soc. 184, 122019. doi:10.1016/j.techfore.2022.122019
Xia, J., Wu, Z., and Chen, B. (2022). How digital transformation improves corporate environmental management: A review and research agenda. Front. Environ. Sci. 10. doi:10.3389/FENVS.2022.943843
Xu, L., Fan, M., Yang, L., and Shao, S. (2021). Heterogeneous green innovations and carbon emission performance: Evidence at China's city level. Energy Econ. 99, 105269. doi:10.1016/J.ENECO.2021.105269
Yi, M., Liu, Y., Sheng, M. S., and Wen, L. (2022). Effects of digital economy on carbon emission reduction: New evidence from China. Energy Policy 171, 113271. doi:10.1016/j.enpol.2022.113271
Yu, Z., Liu, S., and Zhu, Z. (2022). Has the digital economy reduced carbon emissions? Analysis based on panel data of 278 cities in China. Int. J. Environ. Res. Public Health 19, 11814. doi:10.3390/IJERPH191811814
Zha, Q., Huang, C., and Kumari, S. (2022). The impact of digital economy development on carbon emissions - based on the Yangtze River Delta urban agglomeration. Front. Environ. Sci. 10, 750. doi:10.3389/FENVS.2022.1028750
Zhang, C., Chen, P., and Hao, Y. (2022). The impact of digital transformation on corporate sustainability-new evidence from Chinese listed companies. Front. Environ. Sci. 10, 418. doi:10.3389/FENVS.2022.1047418
Zhang, J., Lyu, Y., Li, Y., and Geng, Y. (2022). Digital economy: An innovation driving factor for low-carbon development. Environ. Impact Assess. Rev. 96, 106821. doi:10.1016/j.eiar.2022.106821
Zhang, L., Mu, R., Zhan, Y., Yu, J., Liu, L., Yu, Y., et al. (2022). Digital economy, energy efficiency, and carbon emissions: Evidence from provincial panel data in China. Sci. Total Environ. 852, 158403. doi:10.1016/j.scitotenv.2022.158403
Zhang, W., Liu, X., Wang, D., and Zhou, J. (2022). Digital economy and carbon emission performance: Evidence at China's city level. Energy Policy 165, 112927. doi:10.1016/J.ENPOL.2022.112927
Zhang, Z., Fu, W. K., and Ma, L. (2022). The impact of digital economy on green development in China. Front. Environ. Sci. 10. doi:10.3389/fenvs.2022.991278
Keywords: digital transformation, manufacturing, low-carbon development, multi-regional input-output model, high-dimensional fixed effects model
Citation: Lyu Y, Zhang L and Wang D (2023) The impact of digital transformation on low-carbon development of manufacturing. Front. Environ. Sci. 11:1134882. doi: 10.3389/fenvs.2023.1134882
Received: 31 December 2022; Accepted: 16 January 2023;
Published: 24 January 2023.
Edited by:
Grigorios L. Kyriakopoulos, National Technical University of Athens, GreeceReviewed by:
Guangqin Li, Anhui University of Finance and Economics, ChinaXinghua Cui, Jiangxi University of Finance and Economics, China
Ruonan Fang, Jimei University, China
Copyright © 2023 Lyu, Zhang and Wang. This is an open-access article distributed under the terms of the Creative Commons Attribution License (CC BY). The use, distribution or reproduction in other forums is permitted, provided the original author(s) and the copyright owner(s) are credited and that the original publication in this journal is cited, in accordance with accepted academic practice. No use, distribution or reproduction is permitted which does not comply with these terms.
*Correspondence: Yanfang Lyu, yanfang902@sina.com; Dong Wang, wangrichard72@sina.com