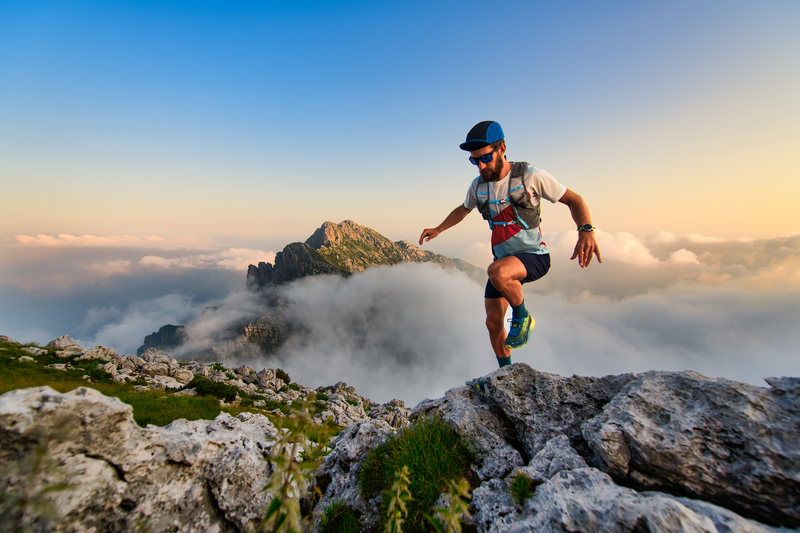
94% of researchers rate our articles as excellent or good
Learn more about the work of our research integrity team to safeguard the quality of each article we publish.
Find out more
ORIGINAL RESEARCH article
Front. Environ. Sci. , 16 January 2023
Sec. Environmental Economics and Management
Volume 11 - 2023 | https://doi.org/10.3389/fenvs.2023.1103625
This paper explores the impact of the Kyoto Protocol by investigating the correlation and risk spillover between the crude oil market and the stock markets of 28 countries during its two commitment periods. Besides time-varying Copula-CoVaR models, the Adaptive Lasso-VAR model with oracle properties is employed in generalized variance decomposition, and a risk connectedness network is constructed to explore risk spillovers between the stock markets of various countries when the crude oil market is at risk. The results reveal positive correlations between the crude oil market and stock markets, which become weaker in the second commitment period than in the first. The crude oil market has both upside and downside spillover effects to most stock markets during both commitment periods, and the upside risk spillover effect is stronger than the downside effect. Overall, most non-signatories of the Kyoto Protocol are net receivers of risk spillovers when the crude oil market is at risk, while most signatories are net exporters of risk spillovers.
On 13 November 2021, the 26th conference of the United Nations Framework Convention on Climate Change (UNFCCC) adopted the Glasgow Climate Agreement and formulated the implementation details of the Paris Agreement, including market mechanisms, transparency, and time frame. As an early exploration prior to the Paris Agreement, the Kyoto Protocol provided a valuable reference for it in terms of cooperation mechanisms and emission reduction methods, making a significant contribution to the reduction of greenhouse gas emissions (Ma, 2012). Analyzing the data of G7 countries from 2010 to 2019 with a GMM-PVAR model, Dogan et al. (2022) show that the Kyoto Protocol has a significant positive impact on energy transition. Maamoun (2019) uses the generalized synthetic control method (GSCM) to compare the emissions of industrialized countries participating in the Kyoto Protocol with their expected emissions had they not participated, and shows that the actual emissions are reduced by about 7% compared to the expected emissions under the “No-Kyoto” scenario. Specifically, the Kyoto Protocol encourages non-signatories to reduce carbon dioxide emissions while limiting carbon emissions of signatories by establishing cooperation mechanisms such as the joint implementation mechanism, the international emissions trading mechanism, and the clean development mechanism (Kuriyama and Abe, 2018; Tran, 2022).
The Kyoto Protocol includes two commitment periods, 2008–2012 and 2013–2020. Compared to the first commitment period, the legal effect and emission reduction efforts of the second commitment period are weakened, but the target adjustment mechanism is improved. The first commitment period has internationally legal binding force on all signatories, while the legal effect of the second commitment period has some uncertainty. To ensure the implementation of the second commitment period on track, the Doha World Climate Conference in 2012 proposed that the signatories should implement the emission reduction tasks as soon as possible in accordance with relevant laws that provisionally apply before completing the ratification. The emission reduction in the second commitment period is weaker, mainly because the signatories were struggling during the economic downturn post the global financial crisis so that greenhouse gas emissions slowed down accordingly. However, with the recovery of the economy, the emissions rebounded, which put pressure on signatories’ deep emission reduction. In terms of the target adjustment mechanism, the second commitment period differs in flexible mechanisms and applicable qualifications. The signatories had a large quantity of assigned amount unit (AAU), emission reduction unit (ERU), and certified emission reduction (CER) from the first commitment period that need to be carried forward to the second commitment period. Meanwhile, some signatories did not undertake quantitative emission reduction or set emission targets during the second commitment period.
It has been documented that financial development and carbon dioxide emissions in many countries are positively correlated (Jamil et al., 2022; Khan et al., 2022). As the pioneering legal instrument in human history to limit greenhouse gas emissions, the Kyoto Protocol attempts to explore a compromise path between economic growth and environmental protection on a global scale (Depledge, 2022). However, the Kyoto Protocol has also received criticism. In particular, the exclusion of developing countries from emissions targets has been portrayed as a fatal design flaw, and the countries’ legal obligations differ under the principle of “common but differentiated responsibilities” (Maamoun, 2019; Tran, 2022). Nevertheless, the Kyoto Protocol restricts the carbon dioxide emissions through its unique cooperation mechanisms and emission reduction methods (Madaleno and Moutinho, 2017; Mohammed, 2020). Did enterprises improve their green technologies and pursue technological transformation, thereby reducing dependence on crude oil? In the meantime, as the financial property of crude oil becomes increasingly apparent, many investors trade oil as a financial asset (Mensi et al., 2017). The price fluctuation of crude oil inevitably affects enterprises’ production costs as well as investing and financing decisions, which are reflected in the stock market. Does the implementation of the first and second commitment periods of the Kyoto Protocol reduce the risk spillover from the crude oil market to stock markets? Which countries’ stock markets are the main receivers of risk spillovers, and which are the exporters, when the oil market is at risk? This paper provides some answers to these questions.
There has been a large body of research regarding the correlation between crude oil market and stock market. On the one hand, some scholars argue for a negative correlation between the two markets, because higher crude oil prices would reduce current and expected profits, leading to a decline in stock prices. For example, Raza et al. (2016) find with a non-linear ARDL model that crude oil prices have a negative impact on the stock markets of emerging economies, which are vulnerable to extreme events. Maghyereh and Abdoh (2022) reveal that extreme oil price shocks have a negative impact on the stock markets of major oil exporters. On the other hand, many scholars believe that the crude oil market and the stock market should have a positive correlation. Kilian and Park (2009) argue that the rising oil prices in the context of global economic expansion will have a sustained positive impact on stock returns. This is because innovations in the global business cycle stimulate the economy, increasing business demand for industrial commodities, thereby driving up oil prices. Working with an ARJI-EGARCH model, Zhang and Chen (2011) find that the Chinese stock market is correlated with the expected volatility of international oil prices, and that international oil prices have a weak positive impact on the Chinese stock market. Alamgir and Amin (2021) investigate the relationship between the crude oil market and stock market indexes in four south Asian countries using a Non-linear Autoregressive Distributed Lag model and report positive correlations. Among the differentiating factors or angles provided in literature regarding the impact of crude oil market on stock markets are the type and timing of oil shocks, the credit status of a country’s economy, and the oil importer or exporter status of a country (Arampatzidis et al., 2021; Jiang et al., 2021; Ramos & Veiga, 2013).
As the dominant commodity with both consumptive and financial attributes, crude oil represents a risk contagion factor to the financial system (Liu et al., 2022). Therefore, the correlation between international crude oil market and stock markets is often accompanied by risk spillover effects. Studying the correlation and risk spillover effects between the two will not only help investors optimize investment portfolios, but also help financial regulators prevent risk contagion between crude oil and stock markets. Zhang and Ma (2019) study the risk spillover effect between the crude oil market and the stock markets of United States, United Kingdom, and Japan based on the EVaR method, and the results show significant two-way spillover effects. Xu et al. (2019) investigate volatility spillovers between crude oil and stock markets using spillover directional measures and asymmetric spillover measures. Using the VAR for VaR approach, Wen et al. (2019) conclude that risk spillovers are stronger after the 2008 financial crisis than before the crisis.
In order to measure the correlation between the crude oil and stock markets and the associated risk spillover effects, researchers often use the time-varying Copula-CoVaR model with non-linear and asymmetric characteristics in empirical analysis. Working on stock market data of U.S., United Kingdom, E.U. and the BRICS countries with the time-varying Copula model, Reboredo and Ugolini (2016) find that the extreme impact of the crude oil market on stock markets before the financial crisis is smaller than that after the crisis. Ji et al. (2020) use the VAR model and the time-varying Copula-GARCH model to measure the dynamic dependencies and risk spillovers between the BRICS stock markets and the crude oil market. Their results show that oil demand shocks pose significant spillover risks to stock returns.
In recent years, with the rapid development of complex network theory in energy economics research, network analysis has become an important tool for studying the correlation between the crude oil market and stock markets and financial risk contagion. Huang et al. (2018) employ co-movement matrixes to study the coherence of oil-stock nexuses in an integrated research framework composed by the wavelet coherence and the complex network. Liu et al. (2020) adopt a complex network approach to explore the characteristics and underlying mechanisms of self-similar behaviors in the ccrude oil market. Wang et al. (2022) combine
Despite all these studies on the relationship between crude oil and stock markets, none has explored the issue from the perspective of the impact of the Kyoto Protocol. In view of this, this paper examines the relationship between the crude oil market and 28 major stock markets in the world (including 17 signatories and 11 non-signatories). Their correlation and risk spillover effects when the crude oil market is at risk are investigated with a variety of models, including ARMA-TGARCH model, Markov regime switching model, time-varying Copula-CoVaR model, and generalized variance decomposition based on the Adaptive Lasso-VAR model. Our interest is to compare and evaluate the correlation and risk spillovers between the two commitment periods of the Kyoto Protocol. The empirical analyses yield several important findings. First, there are positive correlations between crude oil and stock markets. In comparison, the correlation and the risk spillover effects between the crude oil market and most stock markets in the second commitment period are weaker than in the first. Second, the crude oil market at risk has both upside and downside spillover effects to most stock markets, with the upside risk spillover effect being stronger than the downside effect. Third, non-signatories are generally the net receivers of risk spillovers, while signatories are mostly net exporters of risk spillovers conditional on crude oil market in extreme conditions.
The contribution of this paper is threefold. First, in terms of research methodology, the Adaptive Lasso-VAR model with Oracle properties, new to literature, is applied to generalized variance decomposition. It not only solves the estimation problem of high-dimensional VAR model when constructing the risk spillover network proposed by Diebold and Yilmaz (2012, 2014), but also reduces the estimation error of non-zero parameters. Moreover, Ren and Zhang (2010, 2013) do not integrate parameter estimation of the VAR model and the Adaptive Lasso model in a unified framework. This paper fills this void by describing parameter estimation of the Adaptive Lasso-VAR model in full detail.
Second, in terms of the research question, this paper systematically analyzes risk spillovers between the stock markets of 28 countries when the crude oil market is at risk during the first and second commitment periods of the Kyoto Protocol. This research question has not been fully explored in literature, which has focused mostly on stock markets in selected countries rather than providing a global perspective or comparing the effects in the two commitment periods (Reboredo and Ugolini, 2016; Ji et al., 2020). The Copula-CoVaR model employed in this study can effectively describe the dynamic risk spillover between the crude oil and global stock markets, and the risk spillover network can identify the exporters and importers of risk spillovers.
Third, in terms of the research perspective, both downside and upside risk spillover effects of the crude oil market on stock markets are investigated in this paper, while the literature usually examines downside spillover only (Liu et al., 2022). Investigations from the perspective of both long and short positions reveal evident asymmetry in risk spillovers, with upside spillover being more prominent. The findings provide valuable reference for formulating a financial risk firewall mechanism to prevent outbreak and spread of financial crises.
The rest of the paper proceeds as follows. Section 2 introduces the measures and methodology. Section 3 reports and analyzes the empirical results. Section 4 concludes and makes policy recommendations.
Popular measures of tail risk include VaR, ES, and CoVaR. VaR represents the maximum loss of an asset or portfolio in a given duration at the confidence level
Let
where
VaR only considers the loss of a single asset or portfolio in isolation, and does not assess risk transfer between markets. To address this issue, Adrian & Brunnermeier (2016) propose the concept of conditional VaR, or CoVaR. CoVaR represents the extreme risk value of an asset or portfolio when another related asset or portfolio is at extreme risk at the confidence level
As per the nature of the position—long or short—CoVaR can be specified as the downside conditional value at risk,
where
To describe the joint distribution of
where C is a copula function, and
The copula function
Introduced on the basis of static models, dynamic Copula models can characterize the ever-changing interdependence between two markets and predict the joint distribution of asset returns. It has wide applications in asset pricing and financial risk management. Popular dynamic Copula models include time-varying (TV) Normal Copula, TV Student t Copula, TV SJC Copula, TV Plackett Copula, TV Clayton Copula, and TV Gumbel Copula.
Next, upon converting the conditional distribution into the ratio of the joint distribution to the marginal distribution, we use a time-varying Copula function to transform Eqs 3, 4 into Eqs 7, 8, respectively.
Let
Following Adrian and Brunnermeier (2016), this paper uses
This paper adopts the risk spillover index proposed by Diebold and Yilmaz (2012, 2014) as the theoretical framework for risk contagion analysis. This method depicts the risk spillover between different variables through generalized variance decomposition based on the VAR model. The VAR model can be expressed as:
where
where the
where
When there are too many variables, the VAR model will face the curse of dimensionality. In order to solve the estimation problem of high-dimensional VAR, the Lasso method can be used for reduced-dimensional estimations. Nicholson et al. (2015) provide a Lasso-VAR model with penalty terms, for which the parameter estimation expression is
where T is the sample size. For the
In order to reduce the errors in non-zero parameter estimation, Zou (2006) proposes the Adaptive Lasso method, and proves that it enjoys oracle properties; namely, it performs as well as if the true underlying model were given in advance. The basic idea is to assign different penalty weights to parameters based on the Lasso method. The Adaptive Lasso method uses smaller weights to penalize variables with larger initial parameter estimates, and larger weights to penalize variables with smaller initial estimates. This strategy not only preserves the original strength of Lasso estimates, but also effectively reduces estimation errors. When Ren and Zhang (2010, 2013) discuss the Adaptive Lasso-VAR model, they only list the parameter estimation expressions separately for the VAR model and the Adaptive Lasso model, rather than placing them in a unified framework. Nor has other literature provided such a parameter estimation expression. New to literature, this paper provides the parameter estimation expression for the Adaptive Lasso-VAR model, as in Eq. 19:
where
Since the orthogonal assumption of the traditional Cholesky decomposition makes the prediction variance decomposition results very sensitive to the order of model variables, this paper applies the generalized variance decomposition method by following Diebold and Yilmaz (2012, 2014) as the framework for risk contagion analysis. The H-step-ahead forecast error variance decomposition is:
where
The sum of the elements in each row of the generalized forecast error variance matrix is not equal to 1, i.e.,
Now by construction
To present the information on connectedness more intuitively, based on the results of generalized variance decomposition, the risk spillover effect
Following Demirer et al. (2018) and Liu et al. (2022), the risk spillover (connectedness) matrix is constructed based on a network topology framework, as shown in Table 1.
The element
Similarly, the element
Then the net spillover effect
The total spillover effect
The international crude oil futures market has long been dominated by two influential benchmarks, West Texas Intermediate (WTI) on the New York Mercantile Exchange (NYMEX) and Brent on the Intercontinental Exchange (ICE). Compared to Brent crude oil, WTI crude has lower impurities, higher utilization rate, and can be refined into more types of fuel oil. WTI futures have stronger liquidity and relative insensitivity to speculative bubbles (Ajmi et al., 2021; Zhang & Zhang, 2015). This paper selects the daily data of WTI crude oil futures prices and the closing of 28 major global stock market indexes from 7 January 2008 to 30 December 2020 as the sample. All data are retrieved from the Wind financial database. Please see the data sheet in Supplementary material for the dataset. The 28 countries include 11 non-signatories—China, India, Brazil, Israel, South Korea, Mexico, Indonesia, South Africa, Thailand, Turkey, and Malaysia, and 17 signatories—Australia, Austria, Belgium, Denmark, Finland, France, Germany, Greece, Italy, Japan, Netherlands, New Zealand, Norway, Spain, Sweden, Switzerland, and United Kingdom.1 The first commitment period is from 7 January 2008 to 27 December 2012, and the second commitment period is from 7 January 2013 to 30 December 2020. In consideration of space, only the descriptive statistical results of the crude oil market and selected stock markets are reported in Table 2.
The standard deviations show that the volatility of most stock markets in the first commitment period is greater than that in the second commitment period, and the volatility of the crude oil market is much higher than that of the stock markets. The Jarque-Bera tests show that the return series do not follow the normal distribution during either commitment period. The LB and ARCH statistics indicate that most stock markets and the crude oil market exhibit autocorrelation and heteroscedasticity. The ADF statistics suggest that all return series are stationary.
According to the principle of maximum log-likelihood estimation, the ARMA (1,1)-TGARCH(1,1) model is selected for the crude oil market and all stock markets to characterize their marginal distributions. The estimation results and fitting effects of the crude oil market and selected stock markets are reported in Supplementary Tables A1, A2 in Supplementary Appendix.
The ARCH coefficient
To further analyze the return characteristics, this paper adopts the Markov regime switching model to examine the periodicity and asymmetry of the crude oil market and 28 stock markets. The estimation results are shown in Table 3. The transition probabilities indicate that both bear market and bull market have strong continuity, that is, the probabilities for a rising or falling state of the return to continue are almost all above .8. For example, the durations of bear and bull markets for the Chinese stock market are 7.519 and 17.857 days respectively. The bull market in China, India, United Kingdom, and Japan lasts longer than the bear market, while the bear market in the Brazilian and German stock markets as well as the crude oil market lasts longer than the bull market. The regime-switching behavior in stock and crude oil prices manifests financial market psychology and underlying capital movements. When institutional capital buys or sells stocks or crude oil futures according to a market’s expected prosperity, retail investors tend to follow due to the herding effect. Consequently, stock and crude oil prices often demonstrate a certain degree of trend within a short period of time.
Next, we use the TV Normal Copula function, the TV Student t Copula function, the TV SJC Copula function, the TV Plackett Copula function, the TV Clayton Copula function, and the TV Gumbel Copula function to connect the marginal distribution of the crude oil market and stock markets. The optimal Copula function is selected according to the AIC criterion. Table 4 shows the Copula parameter estimation results between the crude oil market and selected stock markets, where the TV Student t or SJC Copula function is the preferred model.
The last column in Table 4 shows the mean value of Spearman’s rank correlation coefficient
Time-varying Copula-CoVaR models can not only measure the correlation between the crude oil and stock markets, but also gauge the risk spillover effect between the two.
It is evident in Figure 2 that in both commitment periods, the upside tail risk of the crude oil market to the stock markets in various countries is significantly higher than the downside tail risk. The upside tail risk has obvious spillover effects (as indicated by the discrepancy between
Table 5 shows through
Based on the risk connectedness network proposed by Diebold and Yilmaz (2012, 2014), this section constructs a risk spillover index through the CoVaR of the crude oil market to the stock markets of various countries. An analysis of the topological characteristics of static and dynamic tail risk spillovers determines the risk exporters and risk receivers among signatories and non-signatories. A generalized variance decomposition based on the Adaptive Lasso-VAR model is established using CoVaR values, for which the optimal lag order is selected according to AIC. Following Diebold and Yilmaz (2012) and Liu et al. (2022), the forecast period H is set to 10 days (i.e., two trading weeks).
First, the static network analysis is conducted to examine the risk spillover characteristics among the stock markets conditional on the crude oil market at risk during the two commitment periods, with the results shown in Table 6. Due to space limitations, only the top five and bottom five countries in each category—TO, FROM, and NET—are listed. Take the risk spillover characteristics of
The static network analysis above explores risk spillover between the stock markets of various countries when the crude oil market is at risk by estimating the models’ fixed parameters over a sample period. Since risk spillovers between stock markets are time-varying, this section draws on Diebold and Yilmaz (2012) to conduct dynamic spillover analysis with a step of 1 and a rolling window of 200. That is, the first spillover is calculated with the data from the 1st to the 200th sample observations, and the second spillover is calculated with the data from the 2nd to the 201st sample observations, and so on.
Figure 3 depicts the total tail risk spillovers —
Three marked periods in Figure 3 are worth close examinations. The first is the 2008 global financial crisis and its aftermath. Due to deep debt crisis and high deficit levels, panic was widespread in the market and the economy in many countries went into recession. The total tail risk spillover,
Since the total spillovers do not reflect the directional information of risk spillover, “TO all others”
Figures 4, 5 characterize the net risk spillover (
FIGURE 4. Net spillovers (1st commitment period). Note: The unit of the vertical axis is percentage.
FIGURE 5. Net spillovers (2nd commitment period). Note: The unit of the vertical axis is percentage.
This paper examines and compares the correlation between the crude oil market and 28 major global stock markets and risk spillover effects during the first and second commitment periods of the Kyoto Protocol. Our major findings are as follows.
There are positive correlations between the crude oil market and stock markets in the world. In comparison, the correlations and risk spillovers between the crude oil and stock markets are weaker in the second commitment period. In addition, during both commitment periods, the crude oil market has risk spillover effects on most stock markets, and the upside risk spillover effect is stronger than the downside effect. This means that compared to long positions, short positions in stock markets are more susceptible to risk spillovers from the crude oil market. Last but not least, according to the total spillover, when the crude oil market is at risk, the tail risk of a stock market mainly comes from risk spillover of other countries rather than from within. The dynamic network analysis reveals that non-signatories are mostly net receivers of risk spillovers, while signatories are net exporters of risk spillovers when the crude oil market is at risk.
The findings in this study offer some advice to market participants and regulators. Stock market investors should be aware of the risk spillover effects from the crude oil market. When formulating and adjusting their portfolios, investors should assess the relationship between crude oil and stock markets to mitigate potential risk caused by extreme oil price fluctuations. As the upside spillover effect of the crude oil market on stock markets is much stronger than the downside effect, stock investors taking short positions should be particularly keen of risk spillover from extreme crude oil market. Overall, investors should maintain a prudent attitude and conduct rational analysis when making investment to avoid being overwhelmed by market sentiment or herding in financial markets. Financial regulators should be prepared for cross-market and cross-border risk contagion. Besides designing and implementing prudential policies to mitigate tail risks in individual markets, they should also pay attention to the risk spillover effects between different markets, and improve cross-market risk handling and regulatory coordination. In particular, since signatories usually export risk spillovers to non-signatories when the crude oil market is at risk, it would be worthwhile to discuss a financial firewall mechanism to prevent the outbreak and spread of financial crisis. Regular communications among regulators in different countries would facilitate international cooperation and coordination.
This study focuses on the relationship between global stock markets and the crude oil market. In reality, besides stock and crude oil markets, investors often also participate in foreign currencies, fixed income securities, and gold investment, thus benefiting from enhanced diversification or hedging. This paper uses the time-varying Copula-CoVaR model to study the correlation and risk spillover between stock and crude oil markets, but does not consider the impact of exchange rates, interest rates, gold, or other factors. The conventional multivariate Copula model requires that the correlation between each pair of variables is identical, which does not fit the reality of multi-market portfolios. As a future project, we plan to employ the vine Copula model to describe the complex interdependence among various financial markets. By calculating CoVaR, we can explore the risk spillover effects between the markets of crude oil, foreign currencies, fixed income securities, and gold, as well as stocks markets of various countries. Generalized variance decomposition based on the Adaptive Lasso-VAR model would enable the construction of a risk spillover network to identify risk exporters and receivers.
The original contributions presented in the study are included in the article/Supplementary Material, further inquiries can be directed to the corresponding author.
JS contributes mainly to the conceptualization and the research design; JL is mainly responsible for model construction and implementation using programming software; JY is dedicated to validation and editing the article; YW and JL are responsible for drafting the paper and data analysis.
This research is supported by the National Natural Science Foundation of China (grant numbers: 71973056 and 71561011) and F.C. Manning Chair in Business Administration endowment fund (Acadia University).
The authors declare that the research was conducted in the absence of any commercial or financial relationships that could be construed as a potential conflict of interest.
All claims expressed in this article are solely those of the authors and do not necessarily represent those of their affiliated organizations, or those of the publisher, the editors and the reviewers. Any product that may be evaluated in this article, or claim that may be made by its manufacturer, is not guaranteed or endorsed by the publisher.
The Supplementary Material for this article can be found online at: https://www.frontiersin.org/articles/10.3389/fenvs.2023.1103625/full#supplementary-material
Supplementary Figure A1 | Directional spillovers: TO all others (1st commitment period).
Supplementary Figure A2 | Directional spillovers: TO all others (2nd commitment period).
Supplementary Figure A3 | Directional spillovers: FROM all others (1st commitment period).
Supplementary Figure A4 | Directional spillovers: FROM all others (2nd commitment period).
Supplementary Table A1 | Estimates of marginal distribution models (1st commitment period).
Supplementary Table A2 | Estimates of marginal distribution models (2nd commitment period).
Supplementary Table A3 | Directional risk spillovers based on static network.
SUPPLEMENTARY Data Sheet 1 | Closing prices of oil and stock markets.
1Since the United States and Canada withdrew from the Kyoto Protocol, they are not included in the study.
2See the Supplementary Table A3 in Supplementary Appendix for the complete information of static risk spillovers for all sample countries.
Adrian, T., and Brunnermeier, M. K. (2016). CoVaR. Am. Econ. Rev. 106 (7), 1705–1741.doi:10.1257/aer.20120555
Ajmi, A. N., Hammoudeh, S., and Mokni, K. (2021). Detection of bubbles in WTI, brent, and dubai oil prices: A novel double recursive algorithm. Resour. Policy 70, 101956. doi:10.1016/j.resourpol.2020.101956
Alamgir, F., and Amin, S. B. (2021). The nexus between oil price and stock market: Evidence from South Asia. Energy Rep. 7, 693–703. doi:10.1016/j.egyr.2021.01.027
Arampatzidis, I., Dergiades, T., Kaufmann, R. K., and Panagiotidis, T. (2021). Oil and the U.S. stock market: Implications for low carbon policies. Energy Econ. 103, 105588. doi:10.1016/j.eneco.2021.105588
Balcilar, M., Ozdemir, H., and Agan, B. (2022). Effects of COVID-19 on cryptocurrency and emerging market connectedness: Empirical evidence from quantile, frequency, and lasso networks. Phys. A Stat. Mech. its Appl. 604, 127885. doi:10.1016/j.physa.2022.127885
Demirer, M., Diebold, F. X., Liu, L., and Yilmaz, K. (2018). Estimating global bank network connectedness. J. Appl. Econ. 33 (1), 1–15. doi:10.1002/jae.2585
Depledge, J. (2022). The “top-down” Kyoto Protocol? Exploring caricature and misrepresentation in literature on global climate change governance. Int. Environ. Agreements Polit. Law Econ. 22, 673–692. doi:10.1007/s10784-022-09580-9
Diebold, F. X., and Yilmaz, K. (2012). Better to give than to receive: Predictive directional measurement of volatility spillovers. Int. J. Forecast. 28 (1), 57–66. doi:10.1016/j.ijforecast.2011.02.006
Diebold, F. X., and Yilmaz, K. (2014). On the network topology of variance decompositions: Measuring the connectedness of financial firms. J. Econ. 182 (1), 119–134. doi:10.1016/j.jeconom.2014.04.012
Dogan, E., Chishti, M. Z., Alavijeh, N. K., and Tzeremes, P. (2022). The roles of technology and Kyoto Protocol in energy transition towards COP26 targets: Evidence from the novel GMM-PVAR approach for G-7 countries. Technol. Forecast. Soc. Change 181, 121756. doi:10.1016/j.techfore.2022.121756
Giot, P., and Laurent, S. (2003). Value-at-Risk for long and short trading positions. J. Appl. Econ. 18 (6), 641–663. doi:10.1002/jae.710
Hansen, B. E. (1994). Autoregressive conditional density estimation. Int. Econ. Rev. 35 (3), 705–730. doi:10.2307/2527081
Huang, S., An, H., Huang, X., and Jia, X. (2018). Co-movement of coherence between oil prices and the stock market from the joint time-frequency perspective. Appl. Energy 221, 122–130. doi:10.1016/j.apenergy.2018.03.172
Jamil, K., Liu, D., Gul, R. F., Hussain, Z., Mohsin, M., Qin, G., et al. (2022). Do remittance and renewable energy affect CO2 emissions? An empirical evidence from selected G-20 countries. Energy and Environ. 33 (5), 916–932. doi:10.1177/0958305X211029636
Ji, Q., Liu, B.-Y., Zhao, W.-L., and Fan, Y. (2020). Modelling dynamic dependence and risk spillover between all oil price shocks and stock market returns in the BRICS. Int. Rev. Financial Analysis 68, 101238. doi:10.1016/j.irfa.2018.08.002
Jiang, Y., Wang, G.-J., Ma, C., and Yang, X. (2021). Do credit conditions matter for the impact of oil price shocks on stock returns? Evidence from a structural threshold VAR model. Int. Rev. Econ. Finance 72, 1–15. doi:10.1016/j.iref.2020.10.019
Kang, S. H., McIver, R., and Yoon, S.-M. (2017). Dynamic spillover effects among crude oil, precious metal, and agricultural commodity futures markets. Energy Econ. 62, 19–32. doi:10.1016/j.eneco.2016.12.011
Khan, F. U., Rafique, A., Ullah, E., and Khan, F. (2022). Revisiting the relationship between remittances and CO2 emissions by applying a novel dynamic simulated ARDL: Empirical evidence from G-20 economies. Environ. Sci. Pollut. Res. 29 (47), 71190–71207. doi:10.1007/s11356-022-20768-z
Kilian, L., and Park, C. (2009). The impact of oil price shocks on the U.S. stock market. Int. Econ. Rev. 50 (4), 1267–1287. doi:10.1111/j.1468-2354.2009.00568.x
Kuriyama, A., and Abe, N. (2018). Ex-post assessment of the Kyoto Protocol-quantification of CO2 mitigation impact in both Annex B and non-Annex B countries. Appl. Energy 220, 286–295. doi:10.1016/j.apenergy.2018.03.025
Liu, B.-Y., Fan, Y., Ji, Q., and Hussain, N. (2022). High-dimensional CoVaR network connectedness for measuring conditional financial contagion and risk spillovers from oil markets to the G20 stock system. Energy Econ. 105, 105749. doi:10.1016/j.eneco.2021.105749
Liu, S. Y., Fang, W., Gao, X. Y., Wang, Z., An, F., and Wen, S. B. (2020). Self-similar behaviors in the crude oil market. Energy 211, 118682. doi:10.1016/j.energy.2020.118682
Ma, Z. F. (2012). The effectiveness of Kyoto Protocol and the legal institution for international technology transfer. J. Technol. Transf. 37 (1), 75–97. doi:10.1007/s10961-010-9190-7
Maamoun, N. (2019). The Kyoto protocol: Empirical evidence of a hidden success. J. Environ. Econ. Manag. 95, 227–256. doi:10.1016/j.jeem.2019.04.001
Madaleno, M., and Moutinho, V. (2017). A new LDMI decomposition approach to explain emission development in the EU: Individual and set contribution. Environ. Sci. Pollut. Res. 24 (11), 10234–10257. doi:10.1007/s11356-017-8547-y
Maghyereh, A., and Abdoh, H. (2022). Extreme dependence between structural oil shocks and stock markets in GCC countries. Resour. Policy 76, 102626. doi:10.1016/j.resourpol.2022.102626
Mensi, W., Hammoudeh, S., Shahzad, S. J. H., and Shahbaz, M. (2017). Modeling systemic risk and dependence structure between oil and stock markets using a variational mode decomposition-based copula method. J. Bank. Finance 75, 258–279. doi:10.1016/j.jbankfin.2016.11.017
Mohammed, S. D. (2020). Clean development mechanism and carbon emissions in Nigeria. Sustain. Account. Manag. Policy J. 11 (3), 523–551. doi:10.1108/Sampj-05-2017-0041
Nicholson, W. B., Matteson, D. S., and Bien, J. (2015). VARX-L: Structured regularization for large vector autoregressions with exogenous variables. Int. J. Forecast. 33 (3), 627–651. doi:10.1016/j.ijforecast.2017.01.003
Ramos, S. B., and Veiga, H. (2013). Oil price asymmetric effects: Answering the puzzle in international stock markets. Energy Econ. 38, 136–145. doi:10.1016/j.eneco.2013.03.011
Raza, N., Shahzad, S. J. H., Tiwari, A. K., and Shahbaz, M. (2016). Asymmetric impact of gold, oil prices and their volatilities on stock prices of emerging markets. Resour. Policy 49, 290–301. doi:10.1016/j.resourpol.2016.06.011
Reboredo, J. C., and Ugolini, A. (2016). Quantile dependence of oil price movements and stock returns. Energy Econ. 54, 33–49. doi:10.1016/j.eneco.2015.11.015
Ren, Y., and Zhang, X. (2013). Model selection for vector autoregressive processes via adaptive Lasso. Commun. Statistics - Theory Methods 42 (13), 2423–2436. doi:10.1080/03610926.2011.611317
Ren, Y., and Zhang, X. (2010). Subset selection for vector autoregressive processes via adaptive Lasso. Statistics Probab. Lett. 80 (23-24), 1705–1712. doi:10.1016/j.spl.2010.07.013
Tran, T. M. (2022). International environmental agreement and trade in environmental goods: The case of Kyoto Protocol. Environ. Resour. Econ. 83, 341–379. doi:10.1007/s10640-021-00625-2
Wang, G.-J., Xie, C., He, K., and Stanley, H. E. (2017). Extreme risk spillover network: Application to financial institutions. Quant. Finance 17 (9), 1417–1433. doi:10.1080/14697688.2016.1272762
Wang, Z., Gao, X., Huang, S., Sun, Q., Chen, Z., Tang, R., et al. (2022). Measuring systemic risk contribution of global stock markets: A dynamic tail risk network approach. Int. Rev. Financial Analysis 84, 102361. doi:10.1016/j.irfa.2022.102361
Wen, D., Wang, G.-J., Ma, C., and Wang, Y. (2019). Risk spillovers between oil and stock markets: A VAR for VaR analysis. Energy Econ. 80, 524–535. doi:10.1016/j.eneco.2019.02.005
Xu, W., Ma, F., Chen, W., and Zhang, B. (2019). Asymmetric volatility spillovers between oil and stock markets: Evidence from China and the United States. Energy Econ. 80, 310–320. doi:10.1016/j.eneco.2019.01.014
Zhang, C., and Chen, X. (2011). The impact of global oil price shocks on China's stock returns: Evidence from the ARJI(-ht)-EGARCH model. Energy 36 (11), 6627–6633. doi:10.1016/j.energy.2011.08.052
Zhang, Y.-J., and Ma, S.-J. (2019). How to effectively estimate the time-varying risk spillover between crude oil and stock markets? Evidence from the expectile perspective. Energy Econ. 84, 104562. doi:10.1016/j.eneco.2019.104562
Zhang, Y.-J., and Zhang, L. (2015). Interpreting the crude oil price movements: Evidence from the Markov regime switching model. Appl. Energy 143, 96–109. doi:10.1016/j.apenergy.2015.01.005
Keywords: Kyoto Protocol, risk spillover, crude oil market, time-varying copula-CoVaR model, generalized variance decomposition
Citation: Sheng J, Li J, Yang J, Wang Y and Li J (2023) High-dimensional CoVaR risk spillover network from oil market to global stock markets—Lessons from the Kyoto Protocol. Front. Environ. Sci. 11:1103625. doi: 10.3389/fenvs.2023.1103625
Received: 20 November 2022; Accepted: 02 January 2023;
Published: 16 January 2023.
Edited by:
Irfan Ullah, Nanjing University of Information Science and Technology, ChinaReviewed by:
Ze Wang, Beijing Normal University, ChinaCopyright © 2023 Sheng, Li, Yang, Wang and Li. This is an open-access article distributed under the terms of the Creative Commons Attribution License (CC BY). The use, distribution or reproduction in other forums is permitted, provided the original author(s) and the copyright owner(s) are credited and that the original publication in this journal is cited, in accordance with accepted academic practice. No use, distribution or reproduction is permitted which does not comply with these terms.
*Correspondence: Jun Yang, anVuLnlhbmdAYWNhZGlhdS5jYQ==
Disclaimer: All claims expressed in this article are solely those of the authors and do not necessarily represent those of their affiliated organizations, or those of the publisher, the editors and the reviewers. Any product that may be evaluated in this article or claim that may be made by its manufacturer is not guaranteed or endorsed by the publisher.
Research integrity at Frontiers
Learn more about the work of our research integrity team to safeguard the quality of each article we publish.