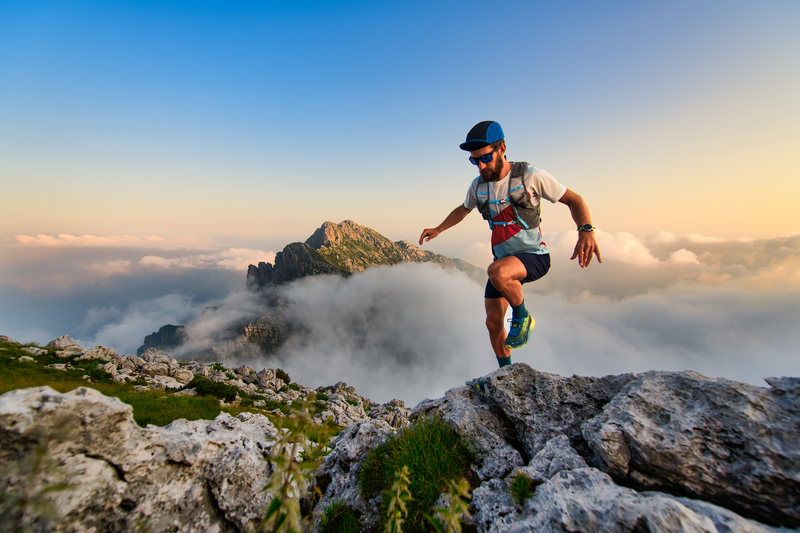
94% of researchers rate our articles as excellent or good
Learn more about the work of our research integrity team to safeguard the quality of each article we publish.
Find out more
ORIGINAL RESEARCH article
Front. Environ. Sci. , 16 February 2023
Sec. Environmental Economics and Management
Volume 11 - 2023 | https://doi.org/10.3389/fenvs.2023.1086403
Improving county economy is the key to realize high-quality economy and “dual carbon” target for developing countries like China where county economy has been weak. The aim of this study is to investigate influencing factors on high-quality economy from a novel perspective of opening high-speed railway (HSR). We apply panel data with time span from 2006 to 2018 and sample area in 396 counties in the upstream Yangtze River of China. As for methodology, we perform entropy weight method (EWM) to estimate the high-quality economy and multi-period Propensity Score Matching (PSM)-difference in difference (DID) methods for empirical analysis. Our results demonstrate that 1). Implementing HSR remarkably promotes high-quality economy at county level, which is stable after a series of verification and confirms our hypothesis 1. We also solve endogeneity by combining PSM-DID with instrumental variable method IV) and Generalized Method of Moment (GMM). 2). Further heterogeneity analysis reveals that opening HSR positively accelerates high-quality economy of counties above average, which proves our hypothesis 2. 3) Our impact mechanism analysis reveals that adjusting industrial structure is main channel, which also confirms our hypothesis 3. Accordingly, the findings provide policy implications: Firstly, a mechanism should be developed to facilitate implementing HSR by strengthening fiscal support from central government, attracting financial institutions with market mechanisms and optimizing spatial layout. Secondly, it is crucial to facilitate horizontal cooperation by hearings or joint meetings and facilitating horizontal financial transfer payments. Lastly, adjusting industrial structure needs to accelerate green industries, while transforming heavily polluted industries.
Improving county-level economies is a challenge facing developing countries, particularly in China. In 1987, Mrs. Brundtland first proposed the concept of “sustainable development”. In 1992, United Nations Conference on Environment and Development took the sustainable development initiative as the common development strategy for all countries in the world. In 2015, 193 member states of the United Nations adopted 17 sustainable development goals (SDGs) including 169 sub goals at the summit. There are lots of studies applying indicators to measure SDGs (e.g., D'Adamo et al., 2022). However, Wilbanks (1994) points out that the basic concept of sustainable development is relatively abstract. Partly because of difficulty to operate SDGs, partly because of China’s localization unlike developed countries, in 2017 China launched national strategy of high-quality economy, which covers high efficient, green, sustainable, and harmonious growth. Actually, high-quality economy inherits some idea and indicators from sustainable development and adapts to China’s localization.
How to promote high-quality economy at county level of China is crucial issue. Some scholars suggest to take advantage of technological progress, financial development, environmental regulation, and industrial structure. Recently, China’s high-speed railway (HSR) has achieved remarkable achievements. By 2021, the operating mileage exceeded 40,000 km, ranking number one in the world.
This motivates us to integrate HSR and high-quality economy at county level. In economic growth theory, constructing transportation infrastructure is considered as one key factor to promote economic growth and development. The simple logic of the endogenous growth mechanism is that in the short term, low-quality or unsustainable technology is allocated to ordinary skilled workers to promote economic growth through consumption channels. With the marginal cost of low-quality or dirty technology increases through environmental channels, which also leads to the loss of consumer welfare and consumption reduction, making the marginal return of low-quality technology decline, while HSR represents high-quality technology and its return enhances. On a dynamic equilibrium: marginal return of low-quality technology equals that of high-quality technology, implying that economic growth quality and improving sustainability tend to be realized at same time. Empirically, Kong et al. (2022) suggest that the introduction of HSR is the most critical driver and an essential part of economic development, and improving the quality of urban economic growth cannot be achieved without urban HSR development at city-level. The fact of urban high-quality economy matching HSR development has confirms the statement in China recently.
The aim of this study is to investigate influencing factors on high-quality economy from a novel perspective of opening high-speed railway (HSR) at county level in China. As for methodology, this paper combines multi-period Propensity Score Matching (PSM)-difference in difference (DID) method with instrumental variable method IV) and Generalized Method of Moment (GMM) to solve endogeneity problem generated by mutual causality or omitted variables or sample selection bias. On one side, the three methods have been widely performed in economics. On the other side, the three models are appropriate tools to answer three research questions posed in this study. As for data use, unlike previous studies focusing on provincial or city data, this study use 396 counties data with time span from 2006 to 2018 because county economy has been weak and is a key for China to achieve high-quality economy and carbon neutrality. Unlike previous studies concentrate whole country, this study chooses the upstream of Yangtze River as a sample area with a length of more than 4,500 km in order to match national strategy to develop rivers and harmonize ecological protection with economic growth.
Our results demonstrate that 1). Implementing HSR remarkably promotes high-quality economy at county level, which is stable after a series of verification and confirms our hypothesis 1. We also solve endogeneity by combining PSM-DID with IV and GMM. 2). Further heterogeneity analysis reveals that opening HSR positively accelerates economy of counties above average, which proves our hypothesis 2. 3) Our mediating effect analysis reveals adjusting industrial structure is main channel, which verifies our hypothesis 3.
Accordingly, the findings provide important policy implications: Firstly, a mechanism should be developed to facilitate implementing HSR by strengthening fiscal support from central government, attracting financial institutions with market mechanisms and optimizing spatial layout. Secondly, it is crucial to facilitate horizontal cooperation by hearings or joint meetings and facilitating horizontal financial transfer payments. Lastly, adjusting industrial structure needs to accelerate green industries, while transforming heavily polluted industries.
The contributions of this study to the literature are: First, this study adopts the entropy value method (EVM) to construct a comprehensive index of high-quality economy and investigates its promoting influence from a novel perspective of HSR, which enriches the theoretical analysis and has academic value. Second, this study use data in counties of China to test HSR influencing high-quality economy where county economy has been weak and is largely ignored in literature. More importantly, this study applies three methods (PSM-DID, IV, GMM) to solve endogeneity problem, which may answer why HSR influences high-quality economy and provides scientific evidence for governments in China to formulate national strategy and for firms to actively engage in HSR and has innovative significance. Further, this paper performs heterogeneity analysis and explores the mediating effects played by improving industrial structure, which explains how HSR influences high-quality economy at county level in China.
This study has some limitations. Due to data lack at county level in China, we cannot evaluate high-quality economy by choosing indicators according to literature on sustainable development or human development index. Another limitation is that we cannot investigate dynamic influence of opening HSR on high-quality development because it is not long before some high-speed railway lines are put into operation.
It is widely believed that opening HSR generates economic influence: 1) Regional accessibility. Hall (2009) believes that HSR causes spatial influences; Jiao and Fang (2018); Wang C. Y et al. (2020) consider different lines and demonstrate that opening HSR significantly improves accessibility. 2) Transfer of firm-level production factors. Li et al. (2020) indicate that HSR markedly accelerated regional labor circulation and capital accumulation. Zhao et al. (2021) suggest that constructing transportation infrastructure for HSR facilitates high-quality firms. Cai et al. (2021) show that high-speed rail affects polluting firms’ locations through effects such as environmental cleaning. 3) Industrial structure at city level. Wang Z. H et al. (2020) reveal that HSR facilitates industrial structure over time; Wang and Lu (2021) reveal that opening HSR tends to reduce emissions and increase economic efficiency at city level; Chen (2021) demonstrate that HSR reduces energy consumption by facilitating industrial upgrading at prefecture level; Wong et al. (2022) believe that HSR facilitates upgrading urban industrial structure and curbing pollutant emissions; Lin and Jia (2022) suggest that HSR tends to significantly enhance a city’s total-factor carbon productivity. 4). Economic growth. Magazzino and Mele (2021) use a time series approach and panel data for 28 provinces over the time 1990–2017 with a Machine Learning technique and an econometric approach (time lag model) and reveal that investment in transport is positively and significantly correlated with economic growth for the short-term test. Li and Jian (2022) construct a Spatial Lag Model (SLM) with panel data of 283 cities in China from 2007 to 2019 and indicate that carbon emissions are spatially correlated, and high-speed railways negatively impact carbon emissions. Sahu and Verma (2022) take advantage of the upcoming Science City at Challakere in the state of Karnataka, India and optimize infrastructure investment from an organizational perspective and demonstrate that maximum achievable productivity gets affected with transportation connectivity level. Sun et al. (2023) apply data on 285 prefecture-level cities of China from 2003 to 2018 and staggered DID and demonstrate that HSR has a positive effect on urban economy. Wang and Dong (2022) use data of more than 2000 counties with time period from 2005 to 2016 and DID model and reveal that HSR connection promotes economic growth measured by GDP per capita or nighttime light data.
Technological progress, financial development, environmental regulation, and industrial structure are cited as influencing factors on high-quality economy. 1) Technological progress. Zhao et al. (2020) demonstrate that the digital economy can promote high-quality development by increasing entrepreneurial activity. 2) Financial development or green finance. Sun and Tang (2022) demonstrate that digital finance tends to contribute to sustainable economy at provincial level. Yang et al. (2021) reveal that green finance comprehensively facilitates a high-quality economy at the provincial level. 3) Environmental regulation. Wang and Lu (2018) reveal that it significantly promotes China’s high-quality economy. Similarly; Song et al. (2022) utilize 28 prefecture-level cities from 2005 to 2018 with spatial Durbin model and suggest that the impact of environmental regulation on economic high quality is an inverted “U" shape. 4) Industrial structure. Sun (2021) suggest that advanced and rationalized industrial structures tend to positively influence high-quality economies at urban level. Zheng and He (2022) confirm that industrial co-agglomeration positively affects the high-quality economy of the Chengdu-Chongqing Economic Circle. Lin and Zhou (2022) apply 30 provincial data during 2000–2017 in China with entropy weight method and bidirectional fixed effects method and find a U-shaped relationship between energy efficiency and economic growth quality and the upgrading of industrial structure plays a mediating role. Zhou et al. (2022) use provincial data with fixed effect model and find that infrastructure construction promotes high-quality economic development.
On one side, at city level, Li and Wang (2021) use the DID model to reveal that HSR promotes a high-quality economy across 108 cities along the Yangtze River Economic Belt. Sun and Zhang (2021) demonstrate that implementing HSR facilitates urban economy and improves the urban environment. Kong et al. (2022) use 284 Chinese cities over 2005–2018 with DID and indicate that the introduction of HSR can promote urban economic growth at city level, but they do not conduct impact mechanism analysis. More microcosmically, at county level, Zhang (2017) reveals that operating HSR facilitates economic growth measured by GDP per capita or nighttime light data. On the other side, existing literature focuses on influencing factors on high-quality economy (e.g., Li and Jian, 2022). Very few studies have investigated HSR’s influence on high-quality economy at county level.
In summary, some scholars probe factors influencing a high-quality economy, such as technological progress, human capital, environmental regulation, financial development level, institutional factors, and the degree of openness, but few studies have investigated the opening of HSR’s influence on high-quality economy. While these studies focus on macro provincial or city levels, no research exists on its influence at county level, where HSR is the primary vehicle for achieving China’s high quality economy and “dual carbon” target. No research has explored its mediating effect at the county level, although data availability is limited. In China’s case, probing the HSR’s influence at the county level illuminates the Chinese high-quality economy and also provides evidence for other developing countries regarding HSR’s facilitation of high-quality economic development.
The growth pole theory points out that after a growth pole is formed, factors always flow to regions with convenient transportation and a developed economy, that is, the growth pole region, which produces a greater diffusion effect and stimulates surrounding areas. HSR implementation is an important manifestation of a potential growth pole. The point axis theory also suggests that HSR belongs to the development axis, which is conducive to extending the geographical scope and development pattern of “point” cities, attracting industries and population, promoting a county’s industrial development and the formation of a wider regional economic belt. Furthermore, new economic geography believes that HSR tends to speed up factor movement. Transportation cost reduction and the emergence of economies of scale will also continuously promote regional industrial agglomeration and economic development, form a virtuous cycle, and continuously promote the formation of a “core-periphery” economic system and regional economic growth. In China, HSR adopts electrification technology, which coincides with sustainable development of energy savings and environmental protection; it has obvious environmental protection advantages, helping China reach the “double carbon” goals. Lu and Wang (2020) and Sheng and Shi (2021) reveal that HSR markedly improved overall economic quality across 270 prefecture-level cities, and this positive promotion is particularly significant in central-western regions. Considering that most counties upstream of the Yangtze River are located in central-western China, HSR’s implementation greatly shortens the distance between counties, brings about rapid factors and resources flows, upgrades the industrial structure, and accelerates county level high-quality economy. Accordingly, this study posits research hypothesis 1: Implementing HSR positively influences a high-quality economy at the county level in the upstream Yangtze River area.
At present, HSR’s advantages include facilitating accessibility between counties and the circulation of factor resources, for which consensus exists in the literature. However, whether HSR influences high-quality economic growth at the county level across different regions requires further consideration and discussion. According to Zhang (2012), He and Liu, 2020, Wang et al. (2014), and Zhang (2017), transportation facilities’ influence on economic development depends on the “diffusion effect” and “agglomeration effect,” and HSR tends to cause different economic impacts in different regions along the line, forming a differentiated regional economic structure. Most studies conclude that opening HSR has differential impacts on different geographical locations or regions with differing economic scales depending on whether the “diffusion effect” and “agglomeration effect” are large or small. There are many involved counties in the upstream Yangtze River area; natural and social conditions between them differ, and HSR policy affects high-quality economy at the county level in these regions differently. Accordingly, we present research hypothesis 2: HSR has a heterogeneous influence on a high-quality economy at the county level in the upstream Yangtze River area.
Many scholars have concluded that operating HSR influences the industrial structure, and its improvement and optimization drive economic development. Chen and Hall (2011) demonstrate that Eurostar train in the United Kingdom accelerates factor gathering in tertiary industries and promotes industrial upgrading. From the perspective of division of labor, convergence, and learning effects, Sun et al. (2022) show that HSR raises population mobility and capital inflow, significantly improves urban industrial structure index, and positively influences economic growth.
Therefore, The above-mentioned literature reveals that opening a high-speed railway influences high-quality economy mainly through the intermediary effect of industrial structure, which means that the influence of HRS on high-quality economy is realized by M. That is, M is function of HRS and high-quality economy is function of M. Mathematically, this can be expressed as follows.
In formulae 1,2 and 3, Where Y indicates high-quality economy, X represents operating high-speed railway, M stands for industrial structure.
In the process of proposed hypotheses, this study poses relevant research questions as follows: Does HSR facilitate high-quality economy in county of China? A candidate method is to use PSM-DID model to identify if there is a causal relationship between HSR and high-quality economy. Previous studies with either FE or OLS model cannot answer the causal relationship because of endogeneity. Further, why would HSR influence high-quality economy? This causal relationship could be explored steadily after solving endogeneity problem brought by the presence of mutual causality or omitted variables or sample selection bias with PSM-DID, GMM and IV models, which have been largely ignored in the literature. The third question is: how could HSR influence high-quality economy? A proposed method is to perform mechanism analysis of improving industrial structure derived in this paper. Unfortunately, previous studies largely ignore the mechanism analysis.
Theoretically, while much attention has been paid to the economic effects of operating HSR, less attention has been paid to HSR’s influencing on high-quality economy. We attempt to investigate influencing factors on high-quality economy from novel perspective of HRS. As the core of modern economy, infrastructure and new economic geographic theory is quite rich. With developing HSR, it comes into being, but theoretical research is still very limited. Following the framework of Acemoglu et al. (2012), this study describes a simple theoretical framework for HSR to facilitate high-quality economy. Basic logic states that technological progress relies on relatively expected values of low-quality and high-quality capitals. HSR represents high-quality technology. Combining the market clearing conditions, we reveal that on endogenous growth path, there exists a dynamic equilibrium: marginal return of low-quality or unsustainable technology equals marginal return of high-quality or sustainable technology. This implies that economic growth quality and improving sustainability seem to be realized simultaneously, which enriches relevant literature.
Following literature (e.g., Zhang et al., 2016), a multiperiod DID model was built. A basic regression model was established as follows:
In this model,
(1) A high-quality economy. At county level of China, this study draws on previous research (Gong et al., 2021; Lin and Zhou, 2022) by combining with county data availability, and constructs an index for estimation by including GDP, number of students in ordinary middle schools, number of medical beds, total power of agricultural machinery, CO2 emissions, and PM2.5 concentration. In order to avoid the influence of subjectivity on the indicators’ weights, we used the entropy weighting method (EWM) to estimate the high-quality economy with 396 counties in upstream Yangtze River area from 2006 to 2018 (
First, standardization of indicators. Since the indicators within the evaluation index system for high-quality economic development in counties are different in nature, the units and directions are not exactly the same. Thus, it is necessary to standardize (also called dimensionless) the raw data of all indicators.
The two Eq. 5 represent the normalization method for positive and negative indicators, respectively. Where
Next, indicator normalization process. Normalization of the index
Next, calculate the information entropy of each index. Using
Next, calculate the redundancy of each index. In Eq. 8, subtract
Furthermore, calculate the weights of each indicator. Eq. 9 represents the calculation of the weights of each indicator based on the redundancy of the indicators
Lastly, calculate the comprehensive evaluation index. After calculating the weights accounted for by each index, multiply Eq. 9 with Eq. 6, and sum up all indicators for the ith county to obtain the economic quality development index
The constructed evaluation indicator system is demonstrated in Table 1.
(2) Opening high-speed railway (
(3) Control variables. Referring to the literature, this study employs the following five control variables: 1. The extent of government intervention, measured by the share of general public budget outlays over GDP (
In 2021, there were 512 county administrative units in the Yangtze River upstream area, including 183 in Sichuan, 38 in Chongqing, 44 in Qinghai, 30 in Hubei, 129 in Yunnan, and 88 in Guizhou provinces. Some counties in Qinghai and Sichuan provinces close to Tibet do not have two consecutive year data for fixed effect use. Because of low population density by 2.2 persons/km2 and location on frigid “Roof of the World” with some no man’s land unsuitable for human habitation, we cannot obtain economic data or values that are small and can be neglected. These missing counties are completely at random and independent of the value of any other variables or counties. On the other side, no HSR operates in these counties with missing whole county data in Figure 2. The limited of the information would not impact on our research theme. Thus, there are a total of 396 counties adopted for our empirical analysis where 65 counties operate HSR. We do summarize a timeline of HSR operation in the study area as Table 2.
With counties, for example, data on indicators or control variables are sometimes missing for certain years. The key issue with the unbalanced panel is determining why the panel is unbalanced. Provided the reason we have missing data for some i is not correlated with the idiosyncratic errors
Due to strict policy on geographic and social data disclosure in China, we explain the subdivision units are county bounaries in the map of the investigated area in data sources section. In addition, we added HSR operation county as a shaded area in red color. A map of the investigated area in shallow green color is provided in Figure 2.
The evaluation index system for high-quality economic development consists of five indicators including GDP per capita, number of general secondary school students, number of beds, and agricultural machinery from the 2019 China County Statistical Yearbook (CCSY, 2019), Data on CO2 sourced from the National Genome Data Center (NGDC, 2020), and PM2.5 from the Atmospheric Composition Analysis Group (ACAG, 2022) at Dalhousie University. The data on the opening of high-speed rail were obtained from the China Railway Yearbook (CRY, 2019), and the website of China National Railway Group Corporation (2020) (CENSD, 2020), and collected and compiled using Python crawler technology.
The control variables (government intervention, living standard of residents, construction of infrastructure, density of social activities, and industrial development) were obtained from the China Economic network statistical database (CENSD, 2020) and the mediating variables (industrial structure) were obtained from the 2019 China County Statistical Yearbook (CCSY, 2019). Data for all indicators were calculated per capita, except for PM2.5 indicator data, which were annual average concentrations. All monetary indicators were deflated. All indicators were log-transformed except for the evaluation indicator of high-quality economic development and the opening of high-speed rail.
Table 3 demonstrates that the average value of the high-quality economy of counties in the Yangtze River upstream area is 0.09, implying that there are distinctions in high-quality economy across counties; the average value of opening HSR for China between 2006 and 2018 was about 0.06 with a standard deviation of 0.05; the mean value of government intervention was 0.34 with a standard deviation of 0.35; the mean value of living standard of residents was 11,000 with a standard deviation of 9,701; the mean value of telephone number was 48100 with a standard deviation of 47,900; the mean value of intensity of economic and social activities was 0.03 with a standard deviation of 0.02; the mean value of development of industrial enterprises was 55.16 with a standard deviation of 60.95; the mean value of topographic relief was 1.84 with a standard deviation of 1.16; the mean value of Industrial structure was 0.36 with a standard deviation of 0.09.
In Table 3, we also list Skewness, kurtosis, variance inflation factor (VIF), which represent a measure of the direction and degree of skewness of statistical data distribution and a statistic compared with the normal distribution, avoiding multicollinearity, respectively. In order to prevent the estimation error caused by multicollinearity, we calculate the variance inflation factor (VIF) of each variable and reveal that the maximum VIF value of variables is 2.54, which is far less than 10, indicating strong independence between variables. Therefore, there is no serious multicollinearity problem between the variables.
Before the regression analysis, we conduct correlation analysis with Pearson test on each variable and demonstrate that all correlation coefficients are less than 0.7, indicating that there is a correlation between various variables without considering other factors (Table 4).
Stationary tests with Augmented Dickey-Fuller (ADF) and Philips Perron (PP) methods were performed for each time series of each variable, first on levels and then on the first differences. We can see that ADF and PP demonstrate that except for intensity of economic and social activities (Den) variable, we reject the null hypothesis of a unit root (0.05 significance level) for all other variables, while in the first difference we reject the null hypothesis for all variables (Table 5).
On one side, in the field of economics and statistics, scholars have widely used these three models: PSM-DID (Card and Krueger, 1994; Qin, 2014; Zhang, 2017), GMM (Hansen, 1982), and IV (Angrist and Krueger, 1991; Faber, 2014). On the other side, the three models are suitable to our questions. The key reason is that omitted variable bias, reverse causality, sample selection deviation would prompt endogeneity problems. No single method can overwhelm the problems. PSM can overcome problem of selection bias by calculating the propensity matching score for matching sample of treatment and control groups with similar values of measurable variables. Card won Nobel Economics Award in 2021 with application of DID that can obtain the net utility of policy implementation by two differences: comparing the differences between the control and treatment groups before and after the implementation of the policy or subtract the before-and-after change (difference) of the control group from the before-and-after change (difference) of the treatment group. It can effectively control the interaction effect between dependent and independent variables, thereby interpreting that these external events (operating HSR) cause the research object to be randomly divided into experimental and control groups. Combining PSM with DID makes model results more accurate. Angrist also won Nobel Economics Award in 2021 with application of IV that is suitable tool to solve autocorrelation in the disturbing terms. Hansen won Nobel Economics Award in 2013 with application of GMM that is applicable to the presence of reverse causality. Previous study uses advanced method like Machine Learning technique to solve endogeneity (Magazzino and Mele, 2021), but it is still rare to use the method in economics field. Thus, in this paper, we perform a combination of the three different methods.
1) Logit Model Construction and Propensity Score Estimation. Propensity Score Matching (PSM) is effective in addressing the problems of sample selection bias and heterogeneity bias. Thus, the treatment and control groups were identical in terms of their trend characteristics. Therefore, the relevant observable variables are matched to the counties of the treatment and control groups. For the sake of more accurately matching between the treatment and control groups, referring to Lin et al. (2020), the logit model for estimating the propensity score is established as follows:
2) Matching Balance and Cosupport Tests. Aiming at the differences before and after the matching of control variables, a balance test can be performed on the treatment and control groups to investigate whether the PSM-DID model is effective. After matching, the testing results showed that the differences between the treatment and control groups tended to be remarkably decreased, and the absolute value of the standard deviation of each pairing index was significantly less than 10%. Therefore, the use of PSM here was reasonable and met the requirements of randomized trials.
Another precondition for PSM is that the “common support hypothesis” must be satisfied. To satisfy this assumption, this study removes individuals with propensity scores close to the two tails in the counties of the treatment and control groups, and only uses these matched samples to assess HSR’s influence on high-quality economies. Figure 3 demonstrates that all sample-matching effects are good and the assumption is satisfied.
The plot about the common range of values for the propensity scores of the experimental and control groups, the vertical axis can be interpreted as the sample size. As can be seen in Figure 3, the majority of the propensity score values for the experimental and control groups lie within the common range of values. Only individual samples are outside the common range of values (green section). As a result, only a small number of samples are lost when propensity score matching is performed. Therefore, the common support hypothesis is satisfied.
Before using the above model for regression analysis, this study draws from Beck et al. (2010) to perform parallel trend tests to confirm that the change trend of the high-quality economy in the treatment and control groups before operating HSR is similar. Otherwise, the changes in the high-quality economy of counties in the upper Yangtze River area may not be caused by implementing HSR. Figure 4 shows that the parallel trend assumption holds in this case.
In the graph, the vertical axis is the policy effect, which indicates the level of high-quality economic development of the county in that year, and the horizontal axis is before and after the policy implementation. As can be seen from Figure 4, there is no significant difference in the level of high-quality economic development between the experimental and control groups before the operation of HSR, which is consistent with the parallel trend hypothesis. And after the implementation of the policy, especially after the third year of the policy implementation, the level of high-quality economic development in the county began to improve, so the model results estimated in this paper can be a more realistic response to the policy effect.
Referencing Shi et al. (2018), this study uses the PSM-DID method to perform stepwise regression to assess HSR’s effect on the high-quality economy of counties in the upstream Yangtze River area. Table 7 presents the benchmark findings with the PSM-DID models. Column 1) includes only core explanatory variables, and columns (2)–(6) are the regression outcomes obtained by gradually increasing the control variables one by one.
The regression results demonstrate that the coefficients are positive at the 1% level. These findings accord with the expectations of this study, suggesting that opening HSR tends to have a significantly positive influence on the high-quality economy of sample counties. Thus, Hypothesis one is supported. From the column-by-column comparison, it appears that, with the gradual addition of control variables, the coefficients of the core explanatory variable show an overall decreasing trend, from the initial 0.021 to 0.008, and the goodness of fit R2 continues to increase. This finding reveals that after controlling for the other factors, opening the HSR, which influences the high-quality economy revealed by the regression results of the PSM-DID model, is more accurate.
This study uses the variable substitution method and placebo test to check the robustness of the regression outcomes. Simultaneously, to resolve endogeneity problems that may arise from the feedback influence of a high-quality economy on opening a HSR, this study uses IV and systematic GMM estimation to further manage endogenous problems to guarantee robustness of regression outcomes and conclusion reliability.
Some scholars, such as Xu et al. (2015), Fan et al. (2016), Zhang (2017), and Niu and Xin, (2021), apply DMSP/OLS night satellite lighting data to measure regional economy as a proxy to avoid being affected by price factors in different regions at different times. This study selects each county’s average nighttime brightness data to replace the variable data of GDP, which measures economic growth, and uses the entropy weight method (EWM) to re-estimate the high-quality economy of counties. Table 8 presents our empirical findings.
TABLE 8. Robustness test results for calculating high-quality development by replacing GDP with nighttime light data.
As expected, core explanatory variables are markedly positive across different specifications, proving the above conclusion’s stability, namely, HSR significantly boosts the high-quality economy of counties. The signs of the control variables with regression models also coincide with expectations in the literature.
The placebo test in the DID model usually includes two methods: changing the time point of the policy and randomizing the treatment group. This study consults Fan and Tian, (2013), Zhang and Tao, (2016), and Ji and Yang, (2020) to conduct counterfactual tests and artificially change the HSR operating time. Specifically, taking the opening time by 2 years, 3 years, and 4 years in advance as a dummy year, we construct three virtual variables of “pseudo-high-speed rail opening” and artificially construct a new processing group to re-estimate. Table 9 presents the regression results.
The consequences of our dummy variables are not significant, indicating that if a counterfactual test is conducted to artificially advance the HSR opening time, a high-quality economy cannot be significantly improved. This further verifies that operating HSR substantially promotes a high-quality economy in the sample counties.
Referencing Lin and Tan (2019), terrain relief was chosen as the instrumental variable. Topographic relief satisfies the two properties of “correlation” and “exogenousness” as an instrumental variable, so it is effective and feasible to select it as an instrumental variable. Two-stage OLS and GMM methods were used for the evaluation. Tables 10 and 11 show the estimation results. With or without the control variables, the X coefficient of the core variable appears significantly positive. This implies that operating HSR enhances the high-quality economy of counties in the upstream Yangtze River area. Our research conclusion is robust and alleviates possible endogeneity problems.
Referring to Shen and Li, (2021), lagged variables of high-quality economic development are introduced into the regression model as explanatory variables, a dynamic panel data model is constructed, and the system GMM is used to perform regression analysis. Table 12 shows the GMM estimation outcomes of the dynamic panel one-stage system. The findings reveal that implementing HSR can remarkably promote the high-quality economy of sample counties. After the control variables were gradually added, the regression results remained stable. This method effectively alleviates the possible endogeneity problems.
Taking the average value of high-quality economy with level data acquired from the descriptive statistics of variables as the dividing point, we divide the county-level administrative regions into “regions with high-quality economy higher than average” and “regions with high-quality economy lower than average” according to their development level and conduct regression and heterogeneity test separately. The regression results are listed in Table 13. HSR tends to facilitate the high-quality economy of counties whose development level is higher than average, while it has a certain adverse influence on counties whose high-quality economies are lower than average.
A possible explanation is that when the level of a high-quality economy is lower than average, these counties often have a weak economic development foundation and insufficient support facilities, although HSR implementation seems to connect regions with different development levels and improve accessibility between regions. In addition, because of insufficient national support policies, lack of human resources, and relatively backward industrial infrastructure, implementing HSR tends to cause a large labor force to flow to developed counties, and the siphon effect is obvious. The radiation-driven effect of well-developed areas on these undeveloped areas cannot be quickly manifested in the short term, which will adversely affect areas with a lower-than-average levels of high-quality economy upon HSR implementation. Hypothesis two was confirmed in this case.
To further explore the heterogeneity of HSR’s influence on the high-quality economy of counties, this study regresses counties in different provinces in this area. Table 14 shows the empirical results. Our findings reveal that implementing HSR remarkably improves the high-quality economy of counties or districts in Qinghai and Hubei Provinces (up to Yichang). HSR has a positive but not significant effect on Sichuan, Yunnan, and Guizhou Provinces, and a relatively prominent negative suppressive influence on high-quality development in Chongqing counties.
A possible explanation is that owing to apparent regional differences in the efficiency of capital and labor redistribution, HSR’s influence on the high-quality economy of counties across different provinces is also heterogeneous. For example, because of the construction and development of the economic circle of twin cities in the Chengdu-Chongqing region, it has certain regional and economic advantages, and the transportation infrastructure has been relatively perfect. The promotion of high-quality economic advances by HSR partially is partially reduced to a certain extent. Therefore, we cannot conclude that HSR’s advent has certain positive or negative influences on the high-quality economy of counties in the Sichuan-Chongqing area. Hypothesis two was thus confirmed in this study.
According to hypothesis 3, Yan et al. (2020), and Gao et al. (2022), we choose the share of added value of tertiary industry over GDP to evaluate industrial structure and investigate the mechanism of HSR’s influence on a high-quality economy across sample counties. Referring to Wen et al. (2004), the mediation effect model was established as follows:
Where
Table 15 shows the stepwise regression results of the mediating influence. In column 1), the effect coefficient
In summary, we apply panel data with time span from 2006 to 2018 and sample area in 396 counties in the upstream Yangtze River of China. As for methodology, we perform entropy weight method (EWM) to estimate the high-quality economy and multi-period Propensity Score Matching (PSM)-difference in difference (DID) methods for empirical analysis. Our results demonstrate that (1). Implementing HSR remarkably promotes high-quality economy at county level, which is stable after a series of verification and confirms our hypothesis 1: Implementing HSR positively influences a high-quality economy at county level in China. We also solve endogeneity with instrumental variable method IV) and systematic Generalized Method of Moment (GMM). 2). Further heterogeneity analysis reveals that opening high-speed railway positively accelerates high-quality economy of counties above average, which proves our hypothesis 2: Operating HSR has a heterogeneous influence on a high-quality economy at county level in China. 3) Our mediating effect analysis reveals adjustment of industrial structure is main channel, which also verifies our hypothesis 3: opening HSR influencing high-quality economy is mainly through adjusting industrial structure at county level in China.
Opening high-speed railway (HSR) continues to advance rapidly, and high-quality development has become a national strategy for economic and social advancement in China. It is worth exploring whether implementing HSR promotes high-quality economy. However, county economy in China has been weak. The aim of this study is to investigate influencing factors on high-quality economy from a novel perspective of opening HSR. We apply panel data with time span from 2006 to 2018 and sample area in 396 counties in the upstream Yangtze River of China. As for methodology, we perform entropy weight method (EWM) to estimate the high-quality economy and multi-period Propensity Score Matching (PSM)-difference in difference (DID) methods for empirical analysis. Our results demonstrate that 1). Implementing HSR remarkably promotes high-quality economy at county level, which is stable after a series of verification and confirms our hypothesis 1. We also solve endogeneity by combining PSM-DID with instrumental variable method IV) and systematic Generalized Method of Moment (GMM). 2). Further heterogeneity analysis reveals that opening HSR positively accelerates high-quality economy of counties above average, which proves our hypothesis 2. 3) Our mediating effect analysis reveals adjustment of industrial structure is main channel, which also confirms our hypothesis 3.
Correspondingly, our research results have several policy implications. Firstly, a mechanism should be developed to facilitate implementing HSR because our results demonstrate that implementing HSR remarkably promotes high-quality economy at county level in China. Operating a HSR is a systematic project, and its complexity and importance determine the necessity of national macro-control. In the new era context, a mechanism should be developed to strengthen fiscal support from central government to provide multilevel financial subsidies for poor counties, while attracting financial institutions with market mechanisms. It is key to explore a new model of joint participation of social capital and win-win cooperation and development, and accelerate a new pattern of market-oriented and orderly competition among multiple parties under the leadership of the government in the construction of high-speed rail projects. More importantly, it is needed to coordinate the design according to landscape, orderly construct high-speed rail lines and optimize its spatial layout.
Secondly, a mechanism should be developed to facilitate horizontal cooperation and coordinated development because our heterogeneity analysis reveals that opening high-speed railway positively accelerates high-quality economy of counties above average. It is relatively easy for counties with high-quality economy, but they should exercise their potential and play radiation and driving roles to achieve win-win prosperity. It is indeed challenging for relatively poor counties to implementing HSR. The possible solution is that county governments should break administrative boundaries and strengthen horizontal cooperation among counties in HSR implementation according to objective and prudent research, judgment and inspection, and scientific and reasonable establishment of railway lines and stations. Hearings or joint meetings across different counties should be institutionalized and normalized. More importantly, a mechanism should be developed to facilitate financial transfer payments from relatively developed counties to developing counties.
Lastly, a mechanism should be developed to adjust industrial structure because our findings demonstrate that HSR facilitates the high-quality economy of counties through industrial structure. It is crucial to scientifically formulate industrial development policies according to actual conditions, match industrial layout with HSR lines, encourage developing green industries and accelerating fusion of cultural, tourism, and business industries in county with rich natural, ecological, and cultural resources, optimize industrial structure by green and low carbon transformation of heavily polluted industries, and establish industrial demonstration parks to improve the relatively backward industrial situation.
As for discussion on comparisons with previous studies, our findings on some degree coincide the literature stating that investment in transport is positively and significantly correlated with economic growth (Magazzino and Mele, 2021), which concentrates on general transport at provincial level of China. In contrast, this paper focuses on operating HSR at county level. Wang and Dong (2022) reveals that opening HSR contributes economic growth measured by GDP or satellite light data in county of China, while this study investigates HSR’s influencing on high-quality economy, which is different from traditional economic growth. More importantly, this paper applies PSM-DID, GMM and IV to solve endogeneity problem brought by the presence of mutual causality or omitted variables or sample selection bias, which steadily identify causal relationship and are largely ignored by existing literature. Lastly, this paper explores the mediating effects of HSR on high-quality economy through adjusting industrial structure at county level in China, which has been neglected by the literature.
This study does have some limitations. Due to data lack at county level in China, we cannot evaluate high-quality economy by choosing indicators according to literature on sustainable development or human development index. Another limitation is that we cannot investigate dynamic influence of opening HSR on high-quality development because it is not long before some high-speed railway lines are put into operation.
Further research should be focused on as follows: Because enterprise is main body of innovation and crucial to achieve high-quality development of economy, further research is need to explore the influence of HSR on firms’ high-quality development. In addition, it is urgent in future to investigate the dynamic characteristics of HSR’s influencing high-quality economy in the long run because the lag effect of opening HSR.
Nevertheless, our research provides similar references for China’s sustainable and high-quality economy and for other developing countries to facilitate a high-quality economy by building infrastructure, particularly HSR.
The original contributions presented in the study are included in the article/supplementary materials, further inquiries can be directed to the corresponding author.
Conceptualization: XL Data curation: XY Formal analysis and investigation: XL, XY Methodology: XY, XL Visualization: XY Writing-original draft preparation: XY, XL Manuscript Validation: XL, HJ Modification suggestions: XL, HJ Language polish: HJ, XL Writing-review and editing: HJ, XL.
This study is financially supported by National Social Science Fund of China (Nr. 22BJL037), Natural Science Foundation of Qinghai Province (2022-ZJ-954Q), Social Science Foundation of Qinghai Province (21050), and Academy of Plateau Science and Sustainability.
The authors thank referees for their helpful comments.
The authors declare that the research was conducted in the absence of any commercial or financial relationships that could be construed as a potential conflict of interest.
All claims expressed in this article are solely those of the authors and do not necessarily represent those of their affiliated organizations, or those of the publisher, the editors and the reviewers. Any product that may be evaluated in this article, or claim that may be made by its manufacturer, is not guaranteed or endorsed by the publisher.
Acemoglu, D., Aghion, P., Hemous, B. D., and Hemous, D. (2012). The environment and directed technical change. Am. Econ. Rev. 102 (1), 131–166. doi:10.1257/aer.102.1.131
Angrist, J. D., and Krueger, A. B. (1991). Does compulsory school attendance affect schooling and earnings? Q. J. Econ. 106, 979–1014. doi:10.2307/2937954
Beck, T., Levine, R., and Levkov, A. (2010). Big bad banks? The winners and losers from bank deregulation in the United States. J. Finance 65 (5), 1637–1667. doi:10.1111/j.1540-6261.2010.01589.x
Cai, H. B., Zhong, C., and Han, J. R. (2021). Upgrade of transportation infrastructure and location selection of polluting enterprises. China Ind. Econ. 10, 136–155. doi:10.19581/j.cnki.ciejournal.2021.10.007
Card, D., and Krueger, A. B. (1994). Minimum wages and employment: A case study of the fast-food industry in NewJersey and Pennsylvania. Am. Econ. Rev. 84 (4), 772–793.
CENSD (2020). China Economic network statistical database (CENSD). Available at: https://db.cei.cn/jsps/Home (Accessed January, 2020).
Chen, C. L., and Hall, P. (2011). The impacts of high-speed trains on British economic geography: A study of the UK's intercity 125/225 and its effects. J. Transp. Geogr. 19 (4), 689–704. doi:10.1016/j.jtrangeo.2010.08.010
Chen, J. (2021). High-speed rail and energy consumption in China: The intermediary roles of industry and technology. Energy 230, 120816. doi:10.1016/j.energy.2021.120816
China County Statistical Yearbook (CCSY) (2019). China county statistical Yearbook. Beijing: China Statistics Press.
D'Adamo, I., Gastaldi, M., and Morone, P. (2022). Economic sustainable development goals: Assessments and perspectives in Europe. J. Clean. Prod. 354, 131730. doi:10.1016/j.jclepro.2022.131730
Faber, B. (2014). Trade integration, market size and industrialization: Evidence from China’s national trunk highway system. Rev. Econ. Stud. 81 (3), 1046–1070. doi:10.1093/restud/rdu010
Fan, Z. Y., Peng, F., and Liu, C. (2016). Political connections and economic growth: Evidence from the DMSP/OLS satellite data. Econ. Res. J. 51 (01), 114–126.
Fan, Z. Y., and Tian, B. B. (2013). Tax competition, tax enforcement and tax avoidance. Econ. Res. J. 48 (09), 99–111.
Gao, P. Y., Yuan, F. H., Hu, H. G., and Liu, X. H. (2022). Motivation, mechanism and governance in high-quality development. Econ. Res. J. 55 (04), 4–19. doi:10.16110/j.cnki.issn2095-3151.2020.12.011
Gong, X., Wang, Y., and Lin, B. (2021). Assessing dynamic China’s energy security: Based on functional data analysis. Energy 217, 119324. doi:10.1016/j.energy.2020.119324
Hall, P. (2009). Magic carpets and seamless webs: Opportunities and constraints for high-speed trains in europe. Built Environ. 35, 59–69. doi:10.2148/benv.35.1.59
Hansen, L. P.10.2307/1912775 (1982). Large sample properties of generalized method of moments estimators. Econometrica 50, 1029–1054. doi:10.2307/1912775
He, D. M., and Liu, P. (2020). Population aging, manufacturing transformation and upgrade, and high-quality economic development—based on mediating effect model. Res. Econ. Manag. 41 (01), 3–20. doi:10.13502/j.cnki.issn1000-7636.2020.01.001
Ji, Y., and Yang, Q. (2020). Can the high-speed rail service promote enterprise innovation? A study based on quasi-natural experiments. J. World Econ. 43 (02), 147–166. doi:10.19985/j.cnki.cassjwe.2020.02.008
Jiao, H., and Fang, Y. C. (2018). A study on the influence of harbin-qiqihar high-speed railway on regional accessibility and economic linkage. Railw. Transp. Econ. 40 (11), 7–11. doi:10.16668/j.cnki.issn.1003-1421.2018.11.02
Kong, Q., Li, R., Jiang, X., Sun, P., and Peng, D. (2022). Has transportation infrastructure development improved the quality of economic growth in China’s cities? A quasi-natural experiment based on the introduction of high-speed rail. Res. Int. Bus. Finance 62, 101726. doi:10.1016/j.ribaf.2022.101726
Li, Q., and Wang, Y. C. (2021). Opening of high-speed rail and high-quality development of the Yangtze River Economic belt. J. Nanjing Univ. Finance Econ. 3, 25–35.
Li, S. K., Zhu, W. P., and Liu, D. (2020). Can high-speed railway promote lndustrial structure upgrading: Based on resource reconfiguration perspective. South China J. Econ. 2, 56–72. doi:10.19592/j.cnki.scje.370347
Li, W., and Jian, J. (2022). The spatial spillover effect of high-speed railway on CO2 emissions in China. Environ. Sustain. Indic. 16, 100204. doi:10.1016/j.indic.2022.100204
Li, Z. Y., and Zhu, Q. R. (2022). Spatial-temporal characteristics of China's high quality economic development level and its influencing factors. Statistics Decis. 6, 95–99. doi:10.13546/j.cnki.tjyjc.2022.06.019
Lin, B., and Jia, H. (2022). Does the development of China’s high-speed rail improve the total-factor carbon productivity of cities? Transp. Res. Part D 105, 103230. doi:10.1016/j.trd.2022.103230
Lin, B. Q., and Tan, R. P. (2019). Economic agglomeration and green economy efficiency in China. Econ. Res. J. 54 (02), 119–132.
Lin, B., and Zhou, Y. (2022). Does energy effificiency make sense in China? Based on the perspective of economic growth quality. Sci. Total Environ. 804, 149895. doi:10.1016/j.scitotenv.2021.149895
Lin, S. L., Meng, X. C., and Zhu, X. C. (2020). Research on the mechanism and heterogeneity effect of the high speed rail and their neighboring effect. Soft Sci. 34 (2), 66–71+91. doi:10.13956/j.ss.1001-8409.2020.02.11
Liu, Z. B. (2018). Understanding the high-quality development: Basic features, supporting elements and current key-issues. Acad. Mon. 50 (7), 39–45+59. doi:10.19862/j.cnki.xsyk.2018.07.004
Lu, F. Z., and Wang, Q. Y. (2020). Influence of the opening of high-speed railway on the quality of economic development and its mechanism. Urban Probl. 10, 56–67. doi:10.13239/j.bjsshkxy.cswt.201007
Ma, H. M., and Hao, M. Z. (2020). Research on the lmpact of high-speed railway construction on regional tourism and high-quality economic development: Taking the guangdong-guangxi-guizhou high-speed rail economic belt as an example. Chongqing Soc. Sci. 2, 79–90. doi:10.19631/j.cnki.css.2020.002.008
Magazzino, C., and Mele, M. (2021). On the relationship between transportation infrastructure and economic development in China. Res. Transp. Econ. 88, 100947. doi:10.1016/j.retrec.2020.100947
National Genomic Data Center (NGDC) (2020). National genomic data center. Available at: https://ngdc.cncb.ac.cn/(Accessed January, 2020).
Niu, F. Q., and Xin, Z. L. (2021). Spillover effect of China's high-speed railway stations and its spatial differentiation: An empirical study based on night-time light datasets. Geogr. Res. 40 (10), 2796–2807.
Qin, Y. (2014). “No county left behind?,” in The distributional impact of high-speed rail upgrade in China (United States: Cornell University Job Market paper).
Sahu, S., and Verma, A. (2022). Quantifying wider economic impacts of high-speed connectivity and accessibility: The case of the Karnataka high-speed rail. Transp. Res. Part A 158, 141–155. doi:10.1016/j.tra.2022.02.011
Shen, K., and Li, Y. N. (2021). How does population ageing impact technological innovation in China? Evidence based on system GMM method and dynamic threshold regression. Popul. Res. 45 (4), 100–113.
Sheng, X. F., and Shi, S. H. (2021). Transportation infrastructure, high quality economic development and haze pollution. Econ. Problems 1, 32–38. doi:10.16011/j.cnki.jjwt.2021.01.005
Shi, D. Q., Ding, H., Wei, P., and Liu, J. J. (2018). Can smart city construction reduce environmental pollution. China Ind. Econ. 6, 117–135. doi:10.19581/j.cnki.ciejournal.2018.06.008
Song, M., Tao, W., and Shen, Z. (2022). Improving high-quality development with environmental regulation and industrial structure in China. J. Clean. Prod. 366, 132997. doi:10.1016/j.jclepro.2022.132997
Sun, W. Z., Niu, D. X., and Wan, G. H. (2022). Transportation infrastructure and industrial structure upgrading: Evidence from China's high-speed railway. J. Manag. World 38 (3), 19–41. doi:10.19744/j.cnki.11-1235/f.2022.0045
Sun, X. T. (2021). Study on the influence of lndustrial structure change on the high-quality development of urban economy. Forum Sci. Technol. China 7, 86–96. doi:10.13580/j.cnki.fstc.2021.07.016
Sun, X. T., and Zhang, G. S. (2021). High-speed railway, environmental and urban economic development. Soft Sci. 35 (6), 103–108. doi:10.13956/j.ss.1001-8409.2021.06.16
Sun, X., Yan, S., Liu, T., and Wang, J. (2023). The impact of high-speed rail on urban economy: Synergy with urban agglomeration policy. Transp. Policy 130, 141–154. doi:10.1016/j.tranpol.2022.11.004
Sun, Y., and Tang, X. W. (2022). The impact of digital inclusive finance on sustainable economic growth in China. Finance Res. Lett. 50, 103234. doi:10.1016/j.frl.2022.103234
Wang, C. Y., Meng, W. D., and Hou, X. S. (2020). The impact of high-speed rails on urban economy: An investigation using night lighting data of Chinese cities. Res. Transp. Econ. 80, 100819. doi:10.1016/j.retrec.2020.100819
Wang, J. E., Jiao, J. J., and Jin, F. J. (2014). Spatial effects of high-speed rails on interurban economic linkages in China. Acta Geogr. Sin. 69 (12), 1833–1846.
Wang, Q. Y., and Lu, F. Z. (2018). Can environmental regulation boost the high-quality development of China's economy? An empirical test based on provincial panel data. J. Zhengzhou Univ. (Philosophy Soc. Sci. Ed. 51 (6), 64–70.
Wang, Q. Y., and Lu, F. Z. (2021). Economic effects of high-speed rail: Emission reduction and efficiency enhancement. Stat. Res. 38 (2), 29–44. doi:10.19343/j.cnki.11-1302/c.2021.02.003
Wang, Y., and Dong, W. (2022). How China’s high-speed rail promote local economy: New evidence from county-level panel data. Int. Rev. Econ. Finance 80, 67–81. doi:10.1016/j.iref.2022.02.040
Wang, Y. F., and Ni, P. F. (2016). Economic growth spillover and spatial optimization of high-speed railway. China Ind. Econ. 2, 21–36. doi:10.19581/j.cnki.ciejournal.2016.02.003
Wang, Z. H., Li, M. M., and Jiang, J. Q. (2020). Heterogeneous impact of traffic accessibility on high-quality development of urban economy. Res. Econ. Manag. 41 (2), 98–111.
Website of China National Railway Group Corporation (CNRGC) (2020). Website of China national railway group corporation. Available at: http://wap.china-railway.com.cn (Accessed January, 2020).
Wen, Z. L., Zhang, L., Hou, J. T., and Liu, H. Y. (2004). Testing and application of the mediating effects. Acta Psychol. Sin. 5, 614–620.
Wilbanks, T. J. (1994). Sustainable development in geographic perspective. Ann. Assoc. Am. Geogr. 84 (4), 541–556. doi:10.1111/j.1467-8306.1994.tb01876.x
Wong, Z., Chen, A. F., Shen, C. R., and Wu, D. L. (2022). Fiscal policy and the development of green transportation infrastructure: The case of China's high-speed railways. Econ. Change Restruct. 55, 2179–2213. doi:10.1007/s10644-021-09381-1
Wooldridge, J. (2001). Introductory econometrics: A modern approach. Massachusetts, United States: South-Western College Publishing, Thomson Learning.
Xu, K. N., Chen, F. L., and Liu, X. Y. (2015). The authenticity of China's economic growth: A test based on global night light data. Econ. Res. J. 50 (9), 17–29+57.
Yan, Y. G., Ni, P. F., and Liu, X. L. (2020). High-speed train, immobility factors and the development of periphery regions. China Ind. Econ. 8, 118–136. doi:10.19581/j.cnki.ciejournal.2020.08.007
Yang, Y. X., Su, X., and Yao, S. L. (2021). Nexus between green finance, fintech, and high-quality economic development: Empirical evidence from China. Resour. Policy 74, 102445. doi:10.1016/j.resourpol.2021.102445
Zhang, J. (2017). High-speed railway construction and county economic development—research based on satellite light data. China Econ. Q. 16 (4), 1533–1562. doi:10.13821/j.cnki.ceq.2017.03.12
Zhang, K. Z., and Tao, D. J. (2016). Economic distribution effects of transportation infrastructure: Evidence from the opening of high-speed rail. Econ. Perspect. 6, 62–73.
Zhang, X. L. (2012). Does China's transportation infrastructure promote regional economic growth—also on the spatial spillover effect of transportation infrastructure. Soc. Sci. China 3, 60–77.
Zhao, T. J., Xiao, X., and Dai, Q. H. (2021). Transportation infrastructure construction and high-quality development of enterprises: Evidence from the quasi-natural experiment of high-speed railway opening in China. Sustainability 13 (23), 13316. doi:10.3390/su132313316
Zhao, T., Zhang, Z., and Liang, S. K. (2020). Digital economy, entrepreneurship, and high-quality economic development: Empirical evidence from urban China. J. Manag. World 36 (10), 65–76. doi:10.19744/j.cnki.11-1235/f.2020.0154
Zheng, H., and He, Y. (2022). How does industrial co-agglomeration affect high-quality economic development? Evidence from chengdu-chongqing economic circle in China. J. Clean. Prod. 371, 133485. doi:10.1016/j.jclepro.2022.133485
Keywords: high-speed rail, high-quality economic development, PSM-DID, counties in the upper reaches of the Yangtze River, China
Citation: Liao X, Yan X and Ji H (2023) Opening high-speed railway’s influence on high-quality economy in China’s counties. Front. Environ. Sci. 11:1086403. doi: 10.3389/fenvs.2023.1086403
Received: 01 November 2022; Accepted: 06 February 2023;
Published: 16 February 2023.
Edited by:
Irem Batool, University of Management and Technology, PakistanReviewed by:
Adam Radzimski, Adam Mickiewicz University, PolandCopyright © 2023 Liao, Yan and Ji. This is an open-access article distributed under the terms of the Creative Commons Attribution License (CC BY). The use, distribution or reproduction in other forums is permitted, provided the original author(s) and the copyright owner(s) are credited and that the original publication in this journal is cited, in accordance with accepted academic practice. No use, distribution or reproduction is permitted which does not comply with these terms.
*Correspondence: Hairui Ji, amliaW5neWVAb3V0bG9vay5jb20=
Disclaimer: All claims expressed in this article are solely those of the authors and do not necessarily represent those of their affiliated organizations, or those of the publisher, the editors and the reviewers. Any product that may be evaluated in this article or claim that may be made by its manufacturer is not guaranteed or endorsed by the publisher.
Research integrity at Frontiers
Learn more about the work of our research integrity team to safeguard the quality of each article we publish.