- 1Department of Civil Engineering, City College of New York, City University of New York, New York, NY, United States
- 2Emeritus, Hungarian Power Company, Budapest, Hungary
- 3Center for Analysis and Prediction of Storms, Hydrometeorology and Remote Sensing Laboratory, University of Oklahoma, Norman, OK, United States
Moving away from fossil fuels is essential for a sustainable future. Carrying out this transition without reversing the improvements in the quality of life is the ultimate challenge. While minimizing the anticipated impacts of climate change is the primary driver of decarbonization, the inevitable exhaustion of fossil energy sources should provide just as strong or perhaps even stronger incentives. The vast majority of publications outlining the pathways to “net-zero carbon emission” fall short from leading to a truly “fossil fuel-free” future without falling back to some level of dependence on fossil fuels with carbon capture and sequestration. While carbon capture and sequestration might be a necessary step toward decarbonization, such intermediate goals might turn into a dead end without defining the end point. The main obstacle to wider adoption of renewable energy resources is their inherent intermittency. Solar and wind are, by far, the most abundant renewable energy sources that are expected to take the lion share in transitioning to a sustainable future. Intermittency arises at multiple levels. The most recognized are the short-term (minute-by-minute, hourly, or diurnal) variations that should be the easiest to address. Less frequently realized are the seasonal and inter-annual variabilities. Seasonality poses far greater challenges than minute-by-minute or hourly variations because they lead to the absence of energy resources for prolonged periods of time. Our interest is the feasibility of a future where all energy (100%) comes from renewable sources leaving no room for fossil fuels. We carry out rudimentary statistical analyses of solar radiation and wind speed time series records to quantify the degree of their intermittencies seasonally and inter-annually. We employ a simple but robust accounting of the shortfalls when the supplies do not meet demand via a modified cumulative supply/deficit analysis that incorporates energy losses arising from transporting excess energy to storage and retrieving it as needed. The presented analysis provides guidance for choosing between the installation of excess capacity or the deployment of energy storage to guarantee reliable energy services under the assumption that the energy system is powered exclusively by renewable energy sources. This paper examines the seasonal and inter-annual variability of hydropower and biofuel resources to estimate their potential to mitigate the intermittencies of solar and wind resources. The presented analyses are meant to provide crude, bulk part estimates and are not intended for planning or operational purposes of the actual energy infrastructures. The primary focus of this paper is the Northeast region of the United States using the conterminous United States as a reference to assess the viability of reducing the energy storage need in the study region via improved connectivity to the national grid. This paper builds on the modeling exercises carried out as part of the climate-induced extremes on food, energy, water systems studies.
1 Introduction
Our industrial economy, built dominantly on fossil fuels accumulated over hundreds of millions of years and likely exhausted in a few centuries, is indisputably unsustainable. The reduction and, ultimately, elimination of carbon-dioxide emissions has been the primary motivation for the decarbonization of the economy, but reducing the needs for increasingly dwindling fossil fuels should provide just as strong incentive.
In our quest to find alternatives to fossil fuels, the immense amount of energy emanating from the Sun and reaching Earth has been seen by many as the most viable means to satisfy the world’s energy needs. Alternative non-carbon energy sources (nuclear fission or geothermal resources) might be more abundant than fossil fuels and may last for millennia or more, but they are still inherently finite, “stock-limited” resources (Gleick and Palaniappan, 2010). Renewables (solar, wind, or hydropower, etc.) driven by the energy coming from the Sun are the only energy sources that are “flux limited” within the expected life of our Sun. If these forms of energy are consumed within their respective “flux limits,” they are steadily “renewed” and, therefore, sustainable.
In recent years, substantial drop in the cost of deploying renewable energy has led to the anticipation that they will soon be price competitive to other forms of energy sources and will become dominant in the energy sector in the not too far distant future (Obama, 2017). While the share of renewable energy sources is undoubtedly increasing rapidly, it is still to be seen if a truly “fossil fuel-free” future will be able to satisfy the energy needs of modern industrial societies. Our paper addresses this research question by investigating the challenges of dealing with the inherent intermittencies of renewable energy source.
The presented work was carried out as part of the studies on climate-induced extremes on food, energy, water systems (C-FEWS) (Vörösmarty et al., 2023a; Vörösmarty et al., 2023b) and intended for a special issue of Frontiers in Environmental Sciences series documenting the C-FEWS framework and its application.
1.1 Problem statement
The major challenge in relying exclusively on “flux-limited” energy sources is to ensure that the varying energy supply can be aligned with the energy demand all the time. “Stock-limited” resources provide both storage and supply by nature and make the alignment of the supply with the demand significantly easier. Although energy flows steadily from the Sun, solar or solar-derived renewable energy sources (e.g., wind) are highly intermittent. The intermittency is due to Earth’s rotation (diurnal) and its tilted axis relative to the orbital plane around the Sun (seasonal) that is exacerbated by the chaotic behaviors of Earth’s atmosphere leading to additional stochastic variabilities. As a result, the energy supplies provided by solar and wind energy sources are highly variable and rarely align with energy demands.
To address the disconnection between energy demand and variable renewable resources, three solutions have been proposed: 1) curtailing loads (that is, modify or fail to satisfy demands), 2) providing supplemental energy sources, or 3) deploying energy storage (Clack et al., 2017). An energy system that fails to satisfy demand, forcing users to accept blackouts or adjust their demand, hardly meets the expectation of reliable services, ruling out the first option as “solution.”
Providing supplemental energy from varying resources can be achieved by installing excess capacity to meet demand all time, but such overbuilding might remain infeasible. For example, at 45° latitude, the incoming solar radiation is three to four times higher in summer than that in winter (these differences are even greater at higher latitudes). Even if winter months were as cloud free as in the summer, solar installation to provide the same power output would require three to four times more solar panels and associated infrastructure to deliver the same energy in the winter.
When the generation of the renewable fails completely, no additional capacity will be able to step in. This situation actually happens in Germany to the extent that they gave a name “Dunkelflaute” (dark lull) referring to the situation that is quite common in October and November, when the sky is covered by thick gray clouds and the air does not move for weeks. In such situation, the only solution left is the deployment of energy storage.
Alternatively, long-distance grid connectivity might allow energy transmission between regions experiencing abundance and shortfalls of renewables at different times and cope with intermittency (Jacobson et al., 2015a; Jacobson et al., 2015b). Interconnected grids can redistribute excess power generated to areas in need. This requires the grid to connect regions experiencing significantly different climate regimes.
Hydropower, while limited in its contribution to satisfying energy demands globally (Fekete et al., 2010), is less affected by intermittency, particularly when substantial water storage behind dams comprises sufficient potential energy to decouple the variation in riverine water fluxes from power generation. Hydropower is expected to partially provide energy at times when solar and wind fall short to meet the demand. Hydropower is much more flexible than solar or wind to the point that hydropower is often operated to assist power generation during peak demands. However, the magnitude that hydropower could be scaled up is poorly understood.
The “capacity factor”—which is the ratio of the power generated at any given time or averaged over a longer time period with respect to the “nameplate capacity” of the installed infrastructure—has a very different meaning for hydropower than for solar or wind. Hydropower plants are not necessarily expected to operate 24/7. They are often built with “nameplate capacity” beyond the energy available if the plant is operated 24/7. Instead, the turbines are purposely left idle and are only turned on when additional power is needed to meet peak demand. This intentionally intermittent operation leads to low “capacity factors.” In contrast, the low-load “capacity factors” of solar or wind generations are unintentional. The low-load “capacity factors” of solar and wind are representing the degree to which they failed to deliver power.
From the perspectives of the grid operator, renewables represent risk that destabilizes power delivery. Although weather forecasts are steadily improving and provide more leeway to prepare for sudden changes in the power supplies, the degree to which grid operators can turn on alternative power sources or alert customers to adjust their power demand is limited. In a truly “fossil fuel-free” energy system that relies exclusively on various renewable energy sources, the only viable means of addressing intermittency is to deploy energy storage.
1.2 Literature review
In preparation for our paper, we compiled a database of publications containing 360+ references from authors dominantly associated with the work of the National Renewable Energy Laboratory (NREL). While our list of publications is unlikely to be fully representative of the entire community researching the transitioning to renewable energy sources, some striking patterns still emerged:
1) Out of the 360+ publications, only few (Doubleday et al., 2019; Kumler et al., 2019; Denholm et al., 2021; Keskar et al., 2023) addressed the seasonal and inter-annual variability of renewables.
2) Almost all publications regarding intermittency of renewables focused on short-term systematic (diurnal), stochastic minute-by-minute, or hourly ramping variabilities of the wind and solar resources.
3) The publications investigating the contribution of energy storage only consider few hours’ storage (typically far less than a day). Even when the need for seasonal storage is acknowledged, the suggested cost-competitive storage capacities are in the order of a couple days to a week at the most (Guerra et al., 2020).
4) Almost all of publications approached “sustainable” power generation from strictly carbon emission perspective and stop at “net-zero decarbonization” that permits fossil fuels in the form of “firm clean energy source” by including carbon capture and sequestration (Sepulveda et al., 2018).
5) Almost all publications were geared toward providing guidelines to integrate renewables into the existing energy system often using very complex and complicated algorithms, where the complexities primarily originated from attempting to optimize for multiple objectives (e.g., stable power generation, carbon emission reduction, and economic viability).
6) No publication attempted to address the entire energy sector, and all “high-decarbonization” and “net-zero” publications were limited to the electric generation sector, allowing some increase at best for partial electrification of other sectors. None of the publications explored a future energy system, where all the energy sectors (beyond the electricity generation) are powered entirely by renewables and, as a consequence, are fully electrified.
7) Even the most ambitious “deep decarbonization” or “high-penetration” scenarios envisioned phasing out somewhere between 25%–50% of the fossil fuels (Kroposki et al., 2017). The feasibility of 100% renewables in the electricity sector was only discussed for few small tropical islands (Holttinen et al., 2021).
Perhaps the most disturbing statement was “Many studies suggest that large (>50%) CO2 emission reductions will not be possible without carbon capture and sequestration (CCS)” (Loftus et al., 2015; Craig et al., 2017) citing the “Deep Decarbonization Project” (https://ddpinitiative.org). If this is a prevailing sentiment among researchers studying the viability of transitioning the energy sector to renewables, one would wish that they were louder and clearer several decades and trillions of dollar investments ago and informed the public that renewables are not sustainable since they will always require the assistance of fossil fuels.
Without dismissing the tremendous value of the scientific work represented in the 360+ publications, we can confidently state that none of them provided insight into a truly sustainable “fossil fuel-free” future. In these publications, most of the complexities arise from striking a balance between economics, carbon emission targets, and technical feasibility of integrating highly variable energy sources into firm power generation from fossil fuels. These studies are undoubtedly essential for a gradual transition where various renewable energy resources coexist with the current firm generation capabilities.
The prioritization of reducing carbon emission sometimes leads to peculiar outcomes when it comes to energy storage. Numerous publications—attempting to address the integration of various forms of storages into the energy mix—came to the conclusion that the added storage capacity has a) no, b) negligible, or c), sometimes, even negative effects (Huang et al., 2011; Arbabzadeh et al., 2015; de Sisternes et al., 2016; Lin et al., 2016). Given that all papers considered very little storage (hours up to a week at best), these peculiarities are not necessarily surprising. When batteries need to compete with “firm energy sources” (fossil fuels with carbon capture and storage), they are likely to come out as too expensive. One could probably arrive to the conclusion without any sophisticated modeling that 15 GW of added wind capacity even if it is idle most of the time will provide more power than 15 GWh (1 h at a rate of 15 GW power generation) energy storage (Huang et al., 2011).
Another surprising characteristic of the papers was that they expressed energy storage in watts (Johnson et al., 2014; Hodge et al., 2018), which we think is wrong. Some publications ultimately reveal what they mean (e.g., 289[MW] with 289[MWh] storage that could be simply referred to as 1[hr] storage) (Johnson et al., 2014). Some others express storage in complex metrics such as 250 MW/250 MWh for every 500[MW] capacity (Bromley et al., 1997), which means a half an hour storage at best that is stretched out for a full hour by delivering half of the power.
Additionally, it is customary to express energy use over time in some form of Wh (GWh, MWh). Since energy use over time (typically year) is a rate of energy use (or power for short), the reported quantities should be written as Wh/yr (kWh/yr, MWh/yr, GWh/yr, etc.), which could be simplified to W (kW, MW, or GW). We are not alone with this assertion, and the late Sir David J. C. MacKay (a physicist and former science advisor to the UK Department of Energy and Climate Change) also noted this in his book (MacKay, 2009). This might sound nitpicking for those who got used to working with these energy units, but we believe that neglecting to recognize that the annual energy use or supply is a rate of energy transfer leads to quite frequent confusion. Putting aside the fact that non-scientific publications often mix these units, but expressing quantities that technically have the same units, leads to obfuscation. For example, the Tinton Falls Solar Farm in New Jersey that we discuss later has a reported nameplate capacity of 19.88 [MW] and 26,652 [MWh] expected power generation in a year. If the annual energy production was expressed in power units (since it is the rate of energy produced over time), then 26,652 [MWh (yr−1)] = 3 [MW] would make it immediately clear that this solar facility has (3[MW/19.88[MW]) approximately 15% annual average “capacity factor.”
The consideration of only very limited energy storage capacities is probably driven by the absence of long duration storage technologies that could hold energy for months or years. This reality is clearly reflected in the distribution of the existing energy storage facilities around the world depicted by the Global Energy Storage Database (GESDB, https://sandia.gov/ess-ssl/gesdb/public, Figure 1) of the Department of Energy (DOE).
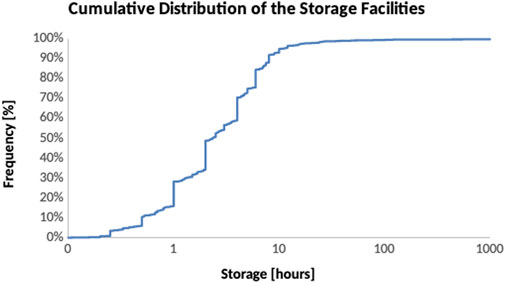
FIGURE 1. Storage distribution of the existing energy storage facilities from the DOE Global Energy Storage Database (GESDB).
In the DOE database, there are only two entries with over 250 h storage worldwide. The largest among them is the Alto Rabagão Hydro Power Plant in Portugal built in 1964. A large artificial rectangular lake (approximately 4 km wide and 20 km long, where the height of dam is 94 m and the water storage capacity is 1,117,000 m3) on the Rabagão River provides 596 days of storage. The second largest is Vilarinho Furnas Pumped Hydro Station also in Portugal with 46 days of storage. Given the big difference between the largest and the second largest storage facilities, one has to wonder if the data entries are correct. The rest of the storage facilities have less than 10 days of storage capacity. The majority of them appears to be pumped storage, although, this can be only inferred from the names of the facilities, because out of the mostly blank 119 attributes that the GESDB provides, none specifies explicitly the storage technology.
1.3 Energy accounting approach
In our view, an accounting of the supply and demand gives robust, first-order estimates about the feasibility of relying entirely on renewable energy. The approach we present is widely used in water resource management. Although, it appears to be absent in most of the energy studies we reviewed, except one (Ryu and Hodge, 2016), which incorporated a similar storage implementation into complex hourly simulation. Wikipedia (https://en.wikipedia.org/wiki/Grid_energy_storage, Figure 2) actually depicts our approach in its general description of energy storage in the grid so its absence in the relevant literature is puzzling.
The goal of this paper is to assess what combination possibilities of
a) building excess power generation capacity,
b) deploying energy storage, and
c) connecting distant regions
could lead to a reliable delivery of energy entirely from variable renewable energy sources. This paper carries out a set of computations offering first-order estimates of the problem. Only few studies attempted to address the feasibility of full decarbonization using renewable resources only (Delucchi and Jacobson, 2011; Jacobson and Delucchi, 2011). Notably, there are significant disagreements regarding the viability of these studies (Clack et al., 2017).
Our paper builds on the approach of recent papers exploring the intermittency of solar and wind resources (Tong et al., 2021; Wu et al., 2022). We analyze the spatially distributed solar and wind data for the conterminous United States (CONUS) and its relationship with energy demands. This paper applies normalized cumulative surplus/deficit analysis to estimate the storage needed to align energy demand with production. It also discusses the potential for alternative, renewable energy sources such as hydropower and biomass to alleviate storage needs.
2 Materials and methods
Our study applies a cumulative surplus/deficit analysis with respect to state-wide and national energy use data to estimate the energy storage needed to align the supply from solar and wind with the energy demand. We convert the spatially averaged daily solar radiation and wind speed time series computed over the study area into daily “capacity factor” (the ratio of the power delivered with respect to the “nameplate capacity”).
We approximate the power generation by a simplified power curve that specifies a minimum “starting threshold” for solar radiation and wind speed for the power generation to begin from where the power generation linearly increases to a “plateauing threshold” that is the “nameplate capacity” beyond which the power generation stops increasing irrespective of the solar or wind resources. Wind power generation has a third limit when the wind speed exceeds a critical value, and the wind turbines are stopped to avoid damages due to stormy weather conditions. It should be noted that our spatially averaged wind speed time series never reaches that limit. While the linear increase might appear to be inappropriate, particularly for wind since wind power is increasing by cubic exponent of the wind speed, wind power curves (Buatois et al., 2014; Maclaurin et al., 2019) are surprisingly close to linear between the “starting” and “plateauing thresholds” (Figure 3).
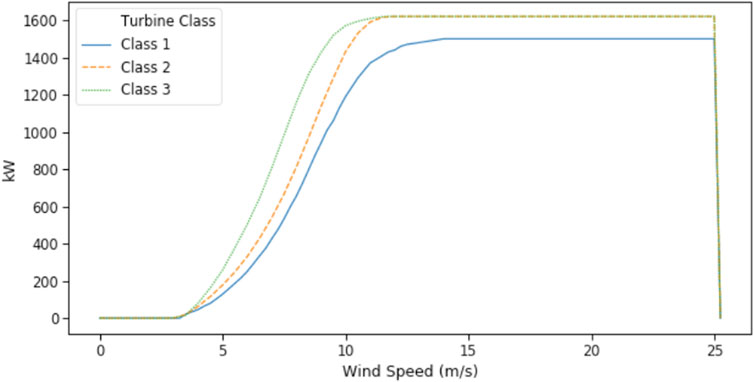
FIGURE 3. Land-based wind turbine power curves (Maclaurin et al., 2019).
We are aware of spatially and temporarily resolved datasets depicting solar (NREL Solar Integration National Dataset Toolkit, SIND) (GE Energy and National Renewable Energy Laboratory NREL, Golden, CO, 2010; Maclaurin et al., 2019) and wind (Draxl et al., 2015) power generation potential across the United States, but these datasets appear to be geared toward the high-frequency (hourly or by the minutes) variations of these renewable resources and depict short time spans (e.g., SIND is limited to 1-year data). Our primary interest is to better understand both the inter-annual and the seasonal variabilities. Furthermore, our team intends to expand the presented work to the entire North American continent and to the globe in future studies.
The plateau in the power generation curves allows the expression of the power generation as the “capacity factor” (
The cumulative surplus/deficit analyses were carried out using normalized power demand (power demand divided by its long-term average) that fluctuates around 1 and, therefore, averages at 1. The energy supply expressed as time-varying “capacity factors” (derived from the observed solar radiation and wind speed records) are also normalized by a constant “excess installation factor” that represents the additional power generation capacity needed to ensure that the energy demands are always met.
The “excess installation factor” can be tuned to eliminate the need for energy storage all time by finding the reciprocal of the lowest “capacity factor” ever arising from the solar radiation or the wind time series as long as the lowest “capacity factor” never reaches zero (0). When it does, then stable power supply cannot be provided without energy storage. Alternatively, the “excess installation factor” can be tuned to overcome longer periods of low-power generation. For example, computing the annual averages of the time-varying “capacity factors” year by year, one could express the “excess installation factor” as the reciprocal of the lowest annual average “capacity factor,” ensuring that the varying renewable resources meet the demand even at times when their annual average is at the lowest.
The normalization allows us to focus on the differences between supply and demand independent of their actual magnitudes without the need to consider where renewable installations are put in place or to align the elasticity of the energy consumptions to supply. We strictly focus on the “storage capacity” needed to balance out periods when there is a deficit between supply and demand.
A modified version of the cumulative surplus/deficit analysis is introduced to account for the round-trip energy losses associated with energy storage. The cumulative deficit calculations are carried out using a full annual cycle as the unit of time so the normalized computations conveniently can be interpreted as the fraction of the annual energy consumption that needs to be stored for reliable delivery of the energy. As a consequence, the “storage capacity” requirements reported here are expressed as a fraction of the annual energy consumption.
All three characteristics, “capacity factor,” “excess installation factor,” and “storage capacity,” are detached from the underlying physical energy quantities, and their dynamics are expressed in relative terms such that they are comparable across regions and scales.
2.1 Study area
The primary focus of this present paper is the Northeast region of the United States, which is part of the C-FEWS study area (Vörösmarty et al., 2023a; Vörösmarty et al., 2023b), comprising Connecticut, Delaware, Massachusetts, Maine, Maryland, New Hampshire, New Jersey, New York, Pennsylvania, Rhode Island, Vermont, and West Virginia. These 12 states span over considerably different climate regimes, while they are economically closely connected to each other.
This paper explores the differences between solar and wind energy generation potentials with respect to energy consumption. In addition to highlighting the differences between the selected 12 states, this paper contrasts those differences with the national averages of the 48 lower states of the CONUS territory. The CONUS serves both as a reference and as a guidance to assess the potential to offset the need for energy storage by interconnectivity to the rest of the nation.
The CONUS is used in this section to demonstrate the inner workings of the statistical analysis and the modified, cumulative surplus/deficit analysis. The methods section includes discussions of the interpretation of the statistical analysis and the results from the cumulative surplus/deficit analysis at considerable length to guide the design of the state level experiments in setting the stage for discussion of the results for the selected 12 states.
2.2 Energy consumption data
Energy consumption data for the United States as a whole and by individual states are available from the Energy Information Agency (EIA) of the Department of Energy (DOE) (EIA, 2022). EIA provides detailed annual and monthly time series of both energy production and consumption by energy sectors. The full time series of the monthly total energy consumption (Figure 4) have some characteristics that are important to highlight because they are relevant for the design of the presented experiments.
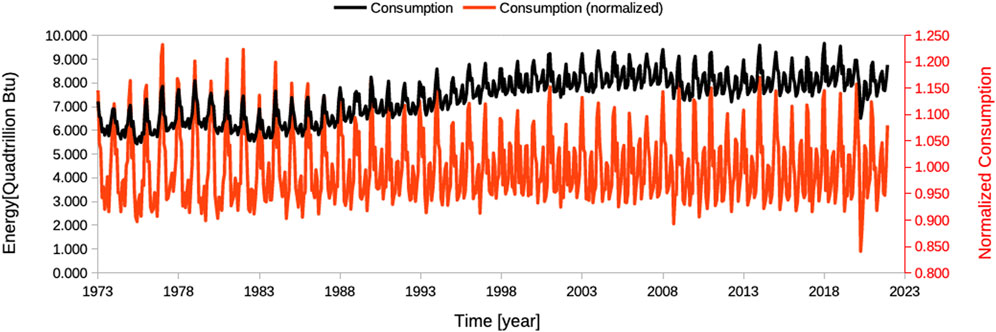
FIGURE 4. Energy consumption of the United States from the Energy Information Agency covering the period of 1973 to present. The black curve shows the EIA monthly energy consumption records, while the red line is the normalized energy consumption (dividing the monthly records by their respective annual means).
Figure 4 shows both the monthly time series of the energy consumption of the United States from 1973 to present and the normalized monthly energy consumption, where the monthly values are divided by their respective annual means. Without formal statistical analysis, one could see that the seasonal variability of the energy consumption is relatively modest and the deviation of normalized energy consumption from its annual average (that is 1 by definition) is only 15%–20%. The energy consumption apparently started to stabilize in the last 3 decades. The seasonal variability seems slightly narrowed in the last 2 decades.
The normalized monthly energy use has an apparent shift in the seasonality from pronounced winter peaks in the 70s to lower winter peaks in the more recent years along with increasing secondary peaks in the summer that were almost absent in the 70s (Figure 4). The quantification and attribution of these shifts would need more in-depth statistical analysis that is beyond the scope of our paper, but it is hard to not interpret the winter peak declines and the increasing summer peaks as a sign of climate change via lowering energy use for heating and increasing use for air conditioning.
Unfortunately, monthly total energy consumption data are only available for the entire United States, and at the state level, only electricity generation is available at monthly granularity. At first glance, our expectation was that the monthly electricity use would follow the seasonality of all the energy consumption. A closer look at the state-level data revealed that in most states, the electricity generation differs from the nationwide dynamics seen in Figure 4. The electricity demands peak in summer, while the total nationwide energy demands peak in winter.
In addition to the absence of energy consumption data depicting the seasonal variations state by state, the transitioning to a 100% renewable future will also require a fundamental shift in the energy consumption itself. Both solar and wind energy sources produce electricity; therefore, a 100% renewable future means that all sectors need to be electrified. It is customary to distinguish primary and secondary energy use, where the primary energy use reflects the energy content of the burnt fossil fuels, while the secondary energy use is the electricity produced after the heat to mechanical energy and to electricity conversions. Moving to renewables cuts off the heat to mechanical energy conversion, but considerable portion of our energy use is heat.
Since the seasonal variation in energy consumption is modest and the peaking in the future will likely change over time both as a consequence of climate change and changes in the power system, our team decided to assume seasonally and inter-annually uniform energy consumption in the present study. The proposed method works well with time-varying energy consumption data. We intend to explore the effects of time variations on energy consumption in future studies.
2.3 Solar radiation and wind speed data
Historical climate data from 1980 to 2019 are from the North American Land Data Assimilation Phase 2 (NLDAS-2 (Xia et al., 2012a; Xia et al., 2012b)), which was used in all studies in the C-FEWS framework (Vörösmarty et al., 2023a). NLDAS-2 data combine energy flux, water flux, and state variables for earth science studies, and the dataset contains 11 primary forcings including long/shortwave radiations and wind speed at 10 m above the surface, which were used in this study.
All 11 primary forcing data within the NLDAS-2 dataset were interpolated from the 3 hourly, 1/8 arc-degree NLDAS-1 dataset. As it concerns this study, the NLDAS solar radiation data were obtained through satellite observation from the Geostationary Operational Environmental Satellite (GOES), and the wind field was simulated from numerical weather prediction (NWP) models (Cosgrove et al., 2003). The dataset has been well studied and validated by the scientific community (Niu et al., 2011; Cai et al., 2014; Barlage et al., 2015; Zhang et al., 2020); thus, the additional validation of NLDAS-2 data was not conducted in this study. The NLDAS-2 data were obtained from the NASA Goddard Earth Sciences Data and Information Services Center (DES DISC, https://disc.gsfc.nasa.gov/, accessed on 05 October 2021), which covers the CONUS from 1980 to 2019.
The gridded, daily solar radiation and wind speed record values from the NLDAS-2 were zonally averaged over the selected states (Connecticut, Delaware, Massachusetts, Maine, Maryland, New Hampshire, New Jersey, New York, Pennsylvania, Rhode Island, Vermont, and West Virginia), comprising the Northeast study region and over the conterminous 48 states of the United States.
The core statistics of the seasonal cycles, such as the daily minimum, maximum, and 25 and 75 percentiles along median values, were computed for each state and the entire CONUS domain (Figure 5). These statistics show marked differences between solar and wind.
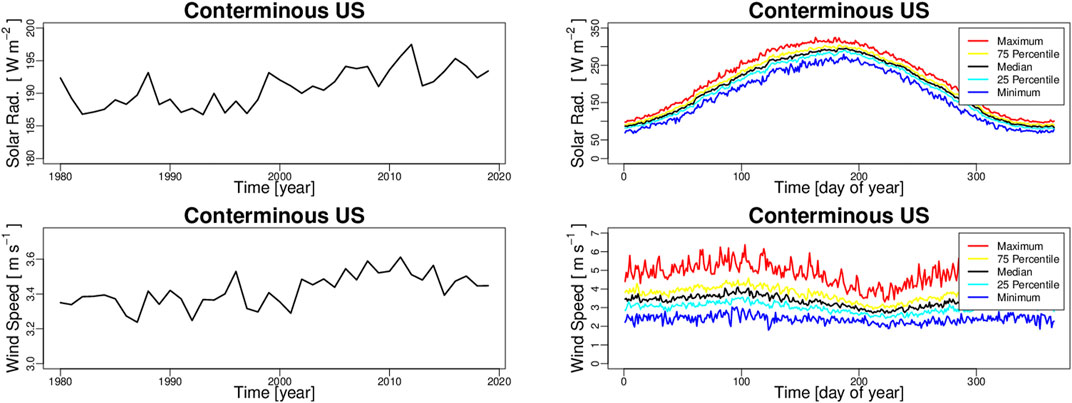
FIGURE 5. Left column: annual average solar radiation and wind speed. Right column: seasonal variability of solar radiation and wind speed over the conterminous United States derived from NLDAS forcing data.
Solar radiation has far greater seasonal variations than wind (considering its median value). Solar radiation goes through a 3.6 times increase between the winter (82.9 [W m-2]) and the summer seasons (295.5 [W m-2]). Wind is seasonally much more “steady” peaking at (4.1 [m s-1]) in April and bottoming out at (2.7 [m s-1]) in August (Figure 5, right column). While the median values of the daily wind speed appear to be less variable than the solar radiation, the range of wind speed on the same day in different years varies more wildly than solar radiations.
On a daily basis, the range of wind speeds averaged over the CONUS could vary as much as solar radiation and has a similar (2.8 times compared to the 3.6 times of solar) ratio between the highest and the lowest spatially averaged wind speed values. Based on seasonal variability, the only viable means of addressing seasonal variabilities is to install some form of energy storage since otherwise, the “excess installation factor” would be at least three to four times the energy demand.
Annually, the average solar and wind energy potential across the CONUS year by year only deviates by a few percentage points (2% for solar and 6% of wind); therefore, a power system relying solely on wind or solar will need only a minor excess capacity to accommodate inter-annual variability. Modest “excess installation factor” would ensure that the system can meet the annual demands even in years with the least amount of solar radiation or wind on an annual basis. Such an excess capacity is probably easier to install than deploying multi-year energy storage solutions. This finding is fundamental in the design of the cumulative surplus/deficit analysis described in the next section.
It was stated earlier that the NLDAS data represent wind speeds (
expressing the wind profile of the boundary layer (Dingman, 2015), where
In the present study, we assumed that the vegetation height was
2.4 Cumulative surplus/deficit (S-curve) calculations
Cumulative deficit curves were taught to hydrologists for decades to determine the storage capacity requirement of water supply reservoirs (Palotas, 1985). The original graphical method relied on establishing the cumulative S-curve of the incoming flow throughout the year and finding the largest difference between the S-curve and a line with slope representing the integral of steady water demands.
This method is easily extendable to varying demand, by numerically integrating the difference between supply and demand, but carrying out the accumulation only when the supply is less than the demand or the accumulated deficit (the already accumulated difference between demand and supply) is positive. The supply (
which is the ratio of the long-term annual average (
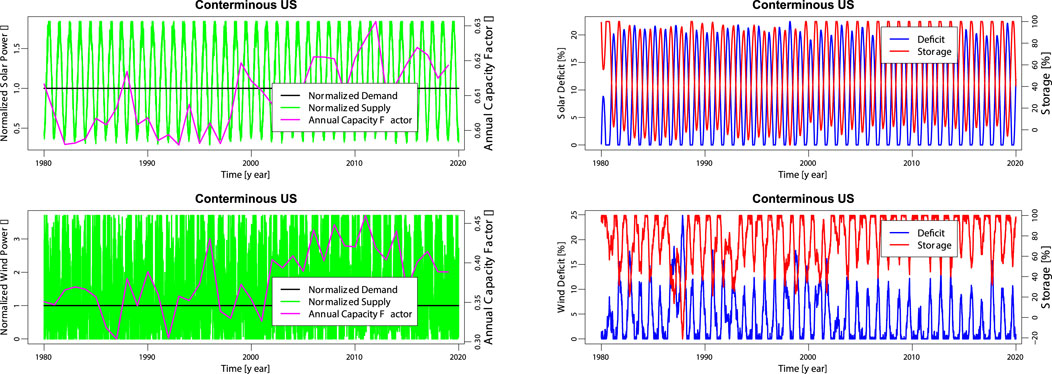
FIGURE 6. Left column: “normalized daily capacity factor” of solar and wind energy contrasted with the normalized energy demand (horizontal line) and “annual capacity factor” (right axis). Right column: modified cumulative deficit computation (for solar and wind) including the energy losses during transporting to and from energy storage and the energy losses due to storage decay.
The cumulative deficit computation can be formalized as follows:
where
This cumulative surplus/deficit analysis is regularly used for water reservoirs, where the water losses during recharging, discharging, and holding the reservoir storage are normally negligible but that is rarely the case for energy storage. Considering efficiency coefficients for recharge (
where
Along with the changes in cumulative deficit, the time series of energy losses (
The “total storage capacity” needed to accommodate the storage losses can be computed from the adjusted power deficit
Since the energy losses are initially not known, the modified cumulative deficit calculations need to be solved iteratively, where an estimate of the “adjusted excess installation factor” (
can serve as an initial value for the adjusted “adjusted excess installation factor” (
As a test of the modified cumulative deficit computation, a complementing storage operation algorithm was implemented that practically mirrors the cumulative deficits and starts from a full energy storage system and tracks the state of the energy storage over time. Figure 6, right column, shows the time series of the modified cumulative deficit along with the energy storage variations over time. The cumulative deficits are computed as a fraction of the annual consumption; therefore, “storage capacity” is also represented as a percentage of the computed annual energy consumption that needs to be stored at most to meet the energy demand all the time.
3 Results
The methods section demonstrated the use of a series of simple statistics along with a cumulative deficit calculation over the CONUS to address a series of important questions regarding the viable operation of energy systems solely relying on solar and wind energy. Table 1 provides a summary of the analysis results for the 12 selected states and the CONUS.
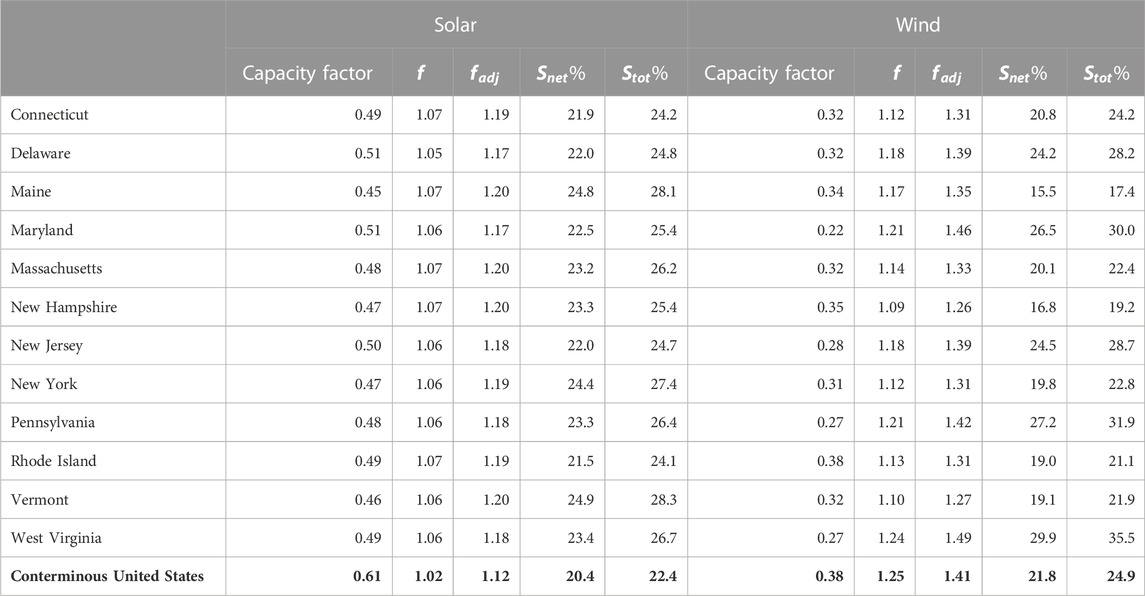
TABLE 1. Summary table of solar and wind resources by the selected states in the Northeast region and the Conterminous United States which serves as a baseline references highlighted in bold characters. The “capacity factor” represents the long-term average of the daily “capacity factors.” The “excess installation factor” (
Over the CONUS, solar radiation appears to have less inter-annual variability compared to wind based on their respective “adjusted excess installation factors” (
The seasonal variability is captured by the storage requirement. Solar power and wind energy require similar nationwide “storage capacities” (
The long-term mean “capacity factor” of the solar energy (61% over the CONUS, Table 1) is higher than that for wind (hovering at approximately 38%, Table 1). The 61% “capacity factor” is misleading here because it was computed on a daily average solar insolation that neglects the diurnal variations. The “nameplate capacity” of solar installation is determined by peak solar radiation around noon in summer; then, the “actual capacity factor” is further reduced. For example, the Tinton Falls Solar Farm in New Jersey discussed in Introduction has 15% “actual capacity factor.” Wind resources have much larger inter-annual variabilities (Figure 6) and require more stand-by (year around) storage that is only exhausted in years with the lowest power generations. The simulated storage (Figure 6) rarely tips down to 0 and remains more than half full most of the time.
The high seasonal variability of solar energy explains its smaller role (24.6 [TWh (yr-1)] = 2.8 [GW]) in the national energy mix than wind (74 [TWh (yr-1)] = 9.5 [GW]) (EIA, 2022) despite being more abundant (Jacobson and Delucchi, 2011). Wind offers steadier power supply seasonally but could have serious power shortfalls in some years. This might be acceptable when the missing power is supplemented by fossil fuels, but in a 100% renewable future when all energy is expected to come from renewables, the need for multi-year “stand-by” storage is likely to become less tolerable.
The exact “storage capacity” is a function of the difference between the seasonal variation of the supply and demand. In our experiments, the energy demand was kept constant seasonally and inter-annually. The result from considering the summer peaking electricity demand would be very different and in favor of the solar energy that also peaks in the summer. In contrast, winter peaking energy demand could increase the needed energy “storage capacity” when the energy system relies entirely on solar energy, while the seasonally steadier wind could serve both summer and winter peaking energy demands with similar “storage capacity.”
The most frequently expressed justification of renewable energy is its perceived abundance. Earth receives enough solar energy in a few hours to satisfy the world’s energy needs for a whole year (MacKay, 2009; Murphy, 2021). The abundance of the solar energy is not reflected in the actual deployment of solar or wind energy where the latter is much less abundant and still delivers more power nationwide as we showed earlier. Energy density clearly matters, and other forms of renewables such as wind, hydro, or biomass go through concentrations provided by Earth’s atmosphere, hydrosphere, or ecosystem.
Modern photovoltaic solar panels that are cheap enough for a large-scale deployment have an efficiency of 15–20% which is around their practical limits (Murphy, 2021). These solar panels cannot be placed wall to wall on the ground, so the overall efficiencies of solar farms are much less. For instance, the Tinton Falls Solar Farm (New Jersey) occupying 40 [ha], receiving 190 [W m-2] solar radiation (76 [MW] over its entire area) with a nameplate capacity of 19.88 [MW], has a power output of 26,652 [MWh (yr-1)] = 3 [MW] that is equal to (3 [MW]/76[MW]) 3.94% solar utilization efficiency (7.5 [W m-2]). In contrast, the Ivanpah Solar Power Facility (California, using concentrating mirrors) is not any better, occupying 1,420 [ha] with 310 [W m-2] site resources and producing 940 [GWh (yr-1)] = 107 [MW]. The resulting solar utilization efficiency is 2.4% (7.44 [W m-2]) so the energy produced over a unit land area is remarkably similar to that of Tinton Falls with much less (190 [W m-2] vs. 310 [W m-2]) solar resources.
In contrast, modern wind turbines can reach slightly over 50% efficiency (that is very close to their theoretical limit of 59%) although 40% is more typical (Murphy, 2021). The energy density of wind turbines can be derived from “the rule of thumb” of the turbine placement dictating that the distance between wind turbines side by side should be five to eight times their blade diameter, while their distance along the prevailing wind direction should be 7–15 times their blade diameter (Murphy, 2021). Based on these rules, the wind power produced over a unit area is in the range of 0.2–1.4 W m-2.
Since wind turbines can be co-located with other land uses, unlike solar farms, their overall footprint is much smaller. Combined with their less-severe seasonal variability and their significantly higher efficiency, it is not surprising that wind power has the larger share in renewable energy production in contrast to the abundance of solar energy argument. Studies outlining pathways to 100% renewables tend to propose the installation of more wind than solar (Jacobson and Delucchi, 2011; Jacobson et al., 2015a; Jacobson et al., 2015b; Denholm et al., 2022).
Comparing the nationwide and regional solar and wind energy resources helps to address the need for large, interconnected power transmission lines. The long-term average daily “capacity factor” of solar power across the CONUS (61%, Table 1) is significantly higher than that in any of the 12 states in the Northeast (hovering at approximately 50%, Table 1). This difference indicates that there are states with well above 61% long-term average daily “capacity factor.” Furthermore, the nationwide 1.12 (Table 1) “adjusted excess installation factor” indicates that there are inter-annually much more stable solar resources than those on the East Coast (between 1.18 and 1.20, Table 1). This means that solar deployment in this region would need to add 6%–8% more installed capacity to prepare for less sunny years.
Although the need for slightly more solar power generation alone would not justify the installation of inter-state power transmission lines, the higher long-term average daily “capacity factors” certainly do. Furthermore, the CONUS spans across four time zones. The diurnal variability shifts from the East Coast to the West; therefore, solar farms on the West Coast can provide power when the Sun goes down on the East Coast while the energy consumption peaks. On the other hand, the need for 22.4% (Table 1) of the annual consumption as energy storage compared to the 24.2%–28.3% (Table 1) in the Northeast region demonstrates that long distance interconnectivity only partially can reduce the need for energy storage.
The wind resources over the CONUS also have higher long-term average “capacity factor” nationwide (38%, Table 1) compared to the 12 states on the Northeast coast (ranging between 27%–38%, Table 1), so there are places around the nation with more steady wind resources than in the Northeast. On the other hand, the “adjusted excess installation factor” (between 1.21 and 1.49, Table 1) is a little bit better in some of the states in the Northeast than over the CONUS (1.41, Table 1). The 17.4%–35.5% (Table 1) energy “storage capacity” needs for wind energy in the Northeast region encapsulates the 24.9% nationwide average (Table 1). Some states (e.g., Maine or New Hampshire) have more steady wind resources on their own than if they were connected to a nationwide grid.
3.1 Regional inter-annual variabilities
The “(adjusted) excess installation factor” for solar installation ranging between 1.17 and 1.20 (Table 1) in the Northeast region is likely to be a robust metric of the inter-annual variability and the excess power generation capacities needed to weather out years, when solar insolation falls below the long-term average. The Northeast states from West Virginia to Maine have slightly higher “adjusted excess installation factor” than the nationwide average. Therefore, these states would need to deploy marginally more excess solar generation capacity to ensure that the energy demands are always met.
The “adjusted excess installation factors” for wind are slightly lower (in the 1.10–1.24 range, Table 1) in the Northeast states than the national average (1.25, Table 1), indicating that inter-annually, the wind resources are more stable than elsewhere on average in the nation. The proximity of these states to the Atlantic Ocean undermines the feasibility of the deployment of wind turbines since this region is prone to hurricanes and the wind turbines near to the coast or offshore are almost guaranteed to be hit by hurricanes during their 25+ years’ life span (Rose et al., 2012).
The annual averages of both the solar and the wind “capacity factors” appear to follow upward trends (Figures 9, 10) deserving more in-depth analyses in future studies.
3.2 Seasonal variability
The seasonality of solar radiation (Figure 7) is very similar to the nationwide conditions (Figure 5). The summer peak of solar radiation does not vary much between states despite the considerable latitudinal differences. Apparently, the lower Sun angles at higher latitudes are compensated by the increased length of the daylight periods during summer. Summer peaks appear to be quite uniform over the region although less sunny than over the CONUS. The differences are greater during winter, when the states further north have a larger drop than the southern states. These differences are quantified in Table 1, showing the “storage capacity” needed (in the range of 24.1%–28.3%, Table 1) to align power generation with consumption (as a measure of the seasonal variability) is clearly higher in these Northeast states than the national average (22.4%, Table 1).
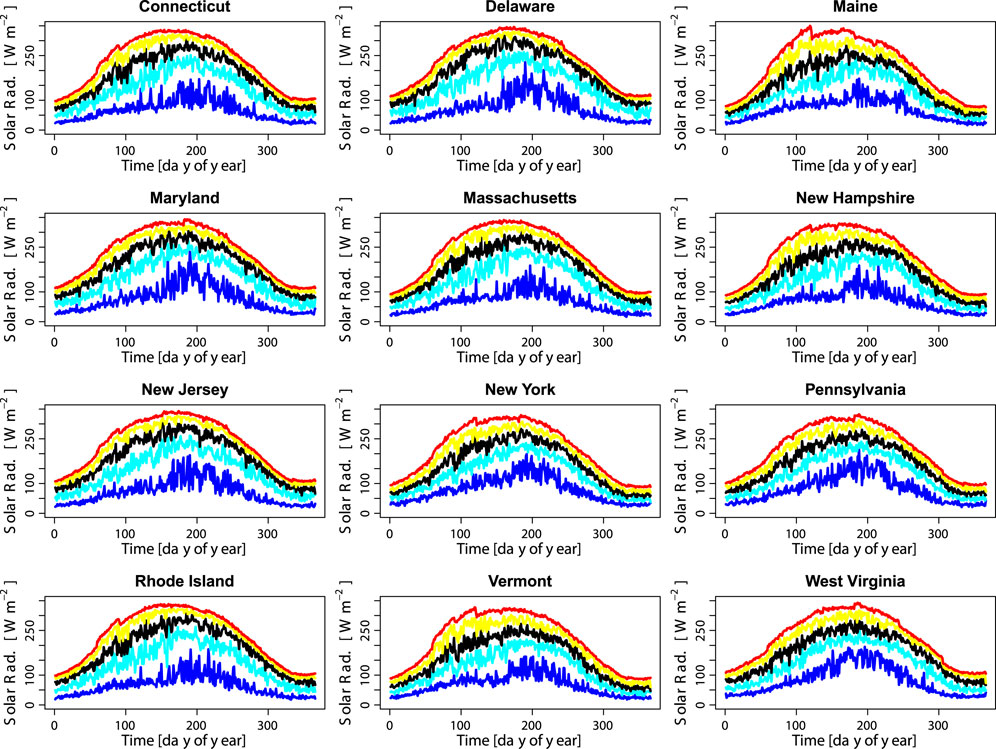
FIGURE 7. Seasonal variability of solar radiation for 12 selected states in the Northeast region of the United States. The presented statistics (minimum, 25 percentile, median, 75 percentile, and maximum) follow the legend in Figure 5.
The seasonal variability of wind is similarly uniform among the 12 states (Figure 8). The summer low and early spring high is more aligned with the consumption regime, so it is clearly better suited for power generation. The median value of the daily wind speeds appears to be closer to the 25 percentile than the 75 percentile (Figure 8), suggesting an asymmetric distribution that is skewed toward the lower values. The median daily values of solar radiation (Figure 7) are closer to the 75 percentile, which is a sign of an asymmetric distribution skewed toward higher values.
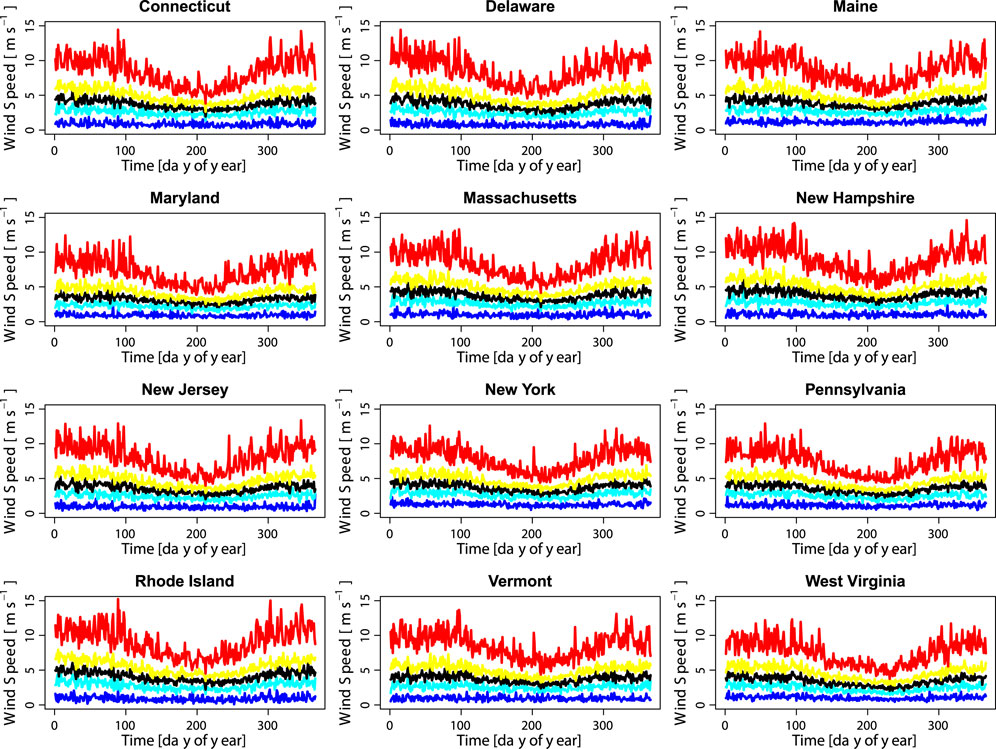
FIGURE 8. Seasonal variability of wind speed for 12 selected states in the Northeast region of the United States. The presented statistics (minimum, 25 percentile, median, 75 percentile, and maximum) follow the legend in Figure 5.
4 Discussion
Our accounting approach allows us to assess the feasibility of transitioning the energy sector to rely entirely on varying renewable energy sources in our study region. We can quantify the a) “excess, installation factor” and b) “storage capacity” needed, excluding the third option of coping with varying energy sources by curtailing energy demand.
Figures 9, 10 show normalized energy consumption along with normalized daily solar and wind energy resources (scaled to meet consumption by the “adjusted excess installation factor”). The daily variability of wind energy is clearly more hectic than solar.
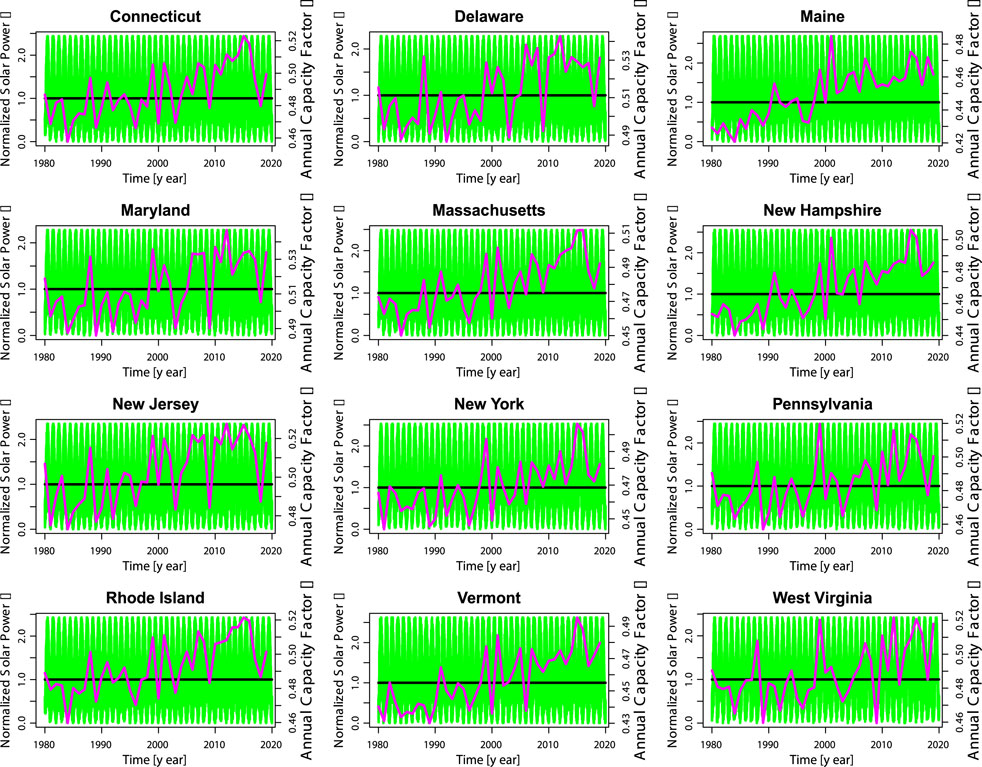
FIGURE 9. Normalized energy consumption (horizontal line at 1) along with daily normalized solar “capacity factor” and the mean annual average solar “capacity factor” (left axis).
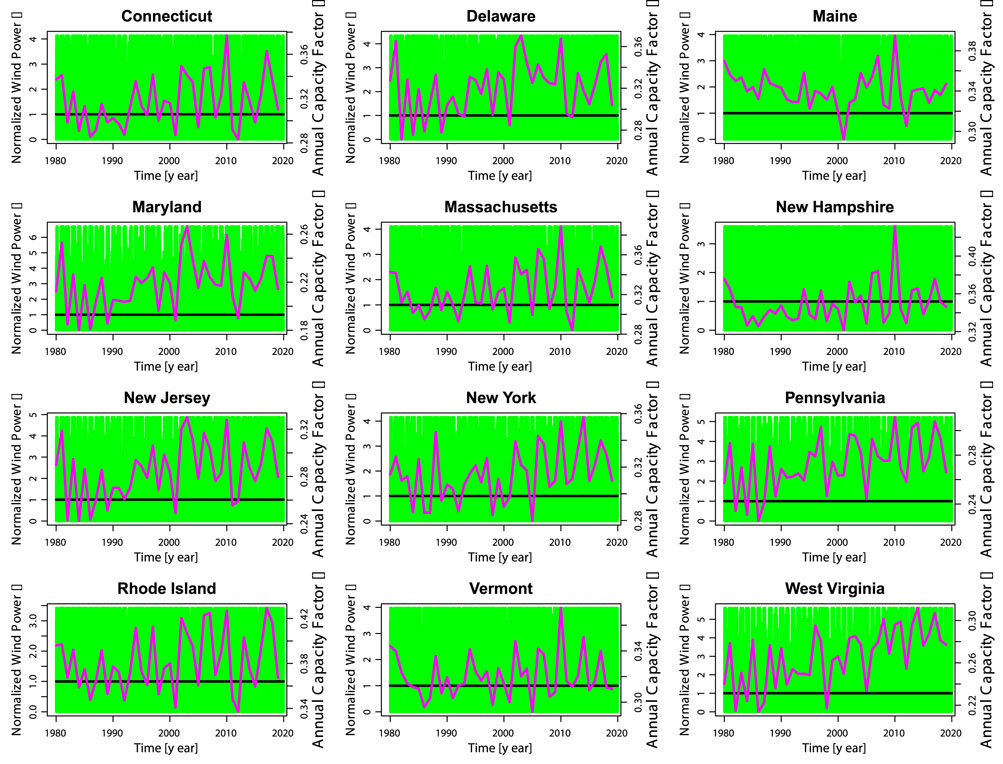
FIGURE 10. Normalized energy consumption (horizontal line at 1) along with daily normalized wind “capacity factor” and the mean annual average wind “capacity factor” (left axis).
The modified cumulative surplus/deficit analysis refined our estimates of the excess installation and storage requirements to factor in the round-trip energy losses due to storage. The three coefficients (
Pumped storage could be represented via a recharge coefficient (
These coefficients could be tuned to various forms of energy storage. Hydrogen economy could be represented as energy losses during hydrolysis (to separate hydrogen and oxygen from water) and transportation as the recharge coefficient (
In the present paper, the recharge coefficient was set to
Changes to these parameters impact both the “total storage capacity” needs (
Table 1 summarizes the energy “storage capacity” needed (
Since the bulk of the “total storage capacity” arises primarily from the cumulative deficit and the energy losses contribute less, our results are informative about the energy storage needs for a storage solution with modest energy losses. Future analyses might test the impacts of storage solutions such that a “hydrogen” economy where both the conversion of electricity to hydrogen and from hydrogen to electricity is highly inefficient, and therefore, the storage requirement leads to substantial excess power generation needs.
Figures 11, 12 show the application of the adjusted cumulative supply/deficit analysis for the 12 states. The deficit time series mirrors the simulated storage time series. The solar deficit and storage variation is more regular for each state, and most of the storage is utilized in each year. Wind resources are clearly more hectic. In some years, they only deplete the storage partially but in other years empty out the storage entirely.
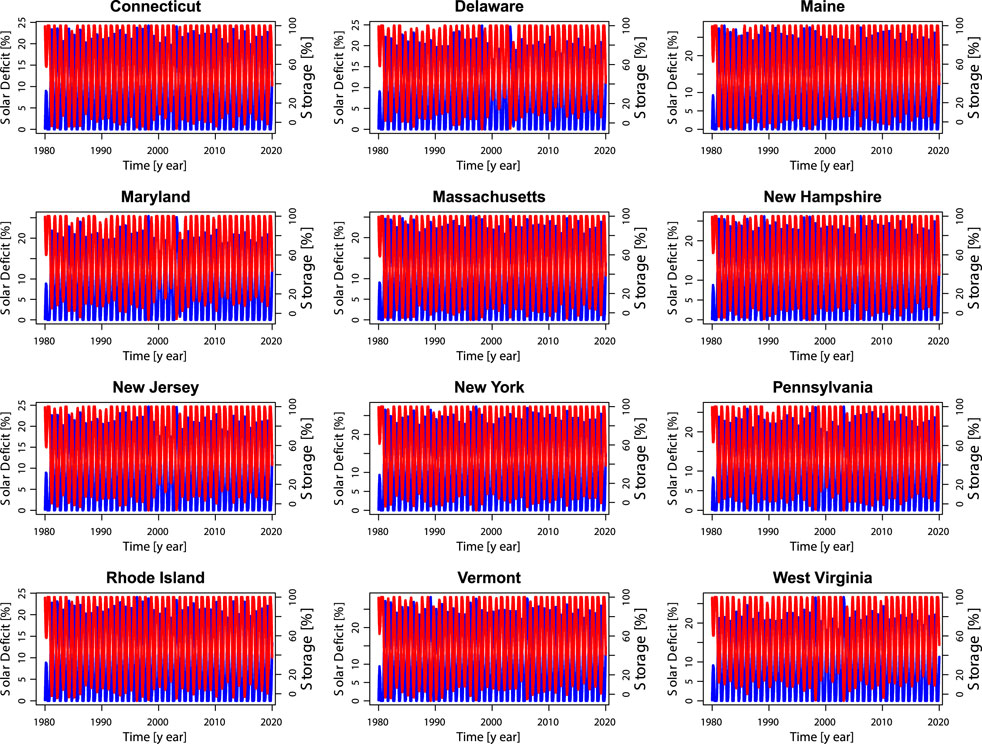
FIGURE 11. Modified cumulative solar deficit computation for the 12 states including the energy losses during transferring to and from energy storage and the energy losses due to storage decay. The figure follows the legend on the left column from Figure 6.
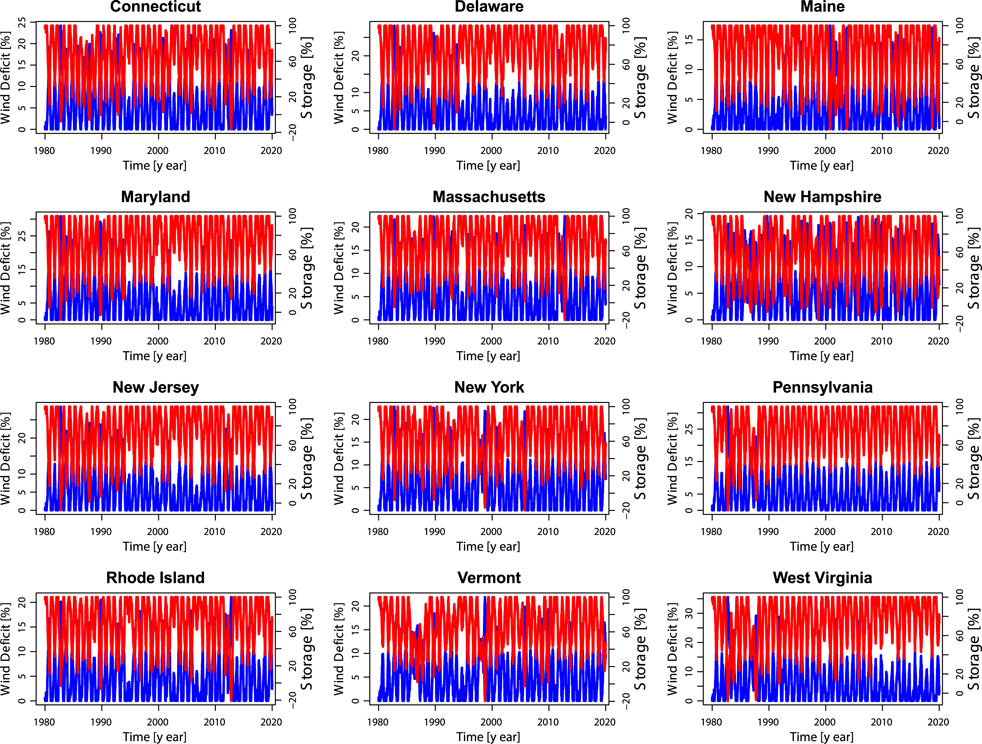
FIGURE 12. Modified cumulative wind deficit computation for the 12 states including the energy losses during transporting to and from energy storage and the energy losses due to storage decay. The figure follows the legend on the left column from Figure 6.
Nationwide, the “total storage capacity” needed for building out a renewable energy future solely using solar power is 22.4% vs. wind 24.9%. These ratios for all Northeast states are ranging between 24.2%–28.3% and 17.4%–35.5% for solar and wind, respectively (Table 1). The wind resources have more inter-annual variability just like nationwide. Just like in the nationwide application of our method, the more modest inter-annual variability of solar resources leads to a storage requirement that is exhausted more completely each year (the storage drops down to near 0 in almost every year (Figure 11), while the more hectic inter-annual variation offsets the more reliable seasonal variability of the wind power availability leading to “total storage capacity” requirement that have a large “stand-by” (year-to-year) part since the “storage capacity” is rarely exhausted entirely (Figure 12).
The difference between the regional (state-wide) needs and the national average appears to be a good indicator of the potential contribution of a nationwide electric transmission connectivity to reduce the need for energy storage. Long-distance energy transport (that has its own potentially substantial energy losses) can lower the need for energy storage, but the CONUS is on the same hemisphere experiencing the same climate regimes; therefore, the availabilities of solar power are closely correlated.
Based on the presented analyses, the 100% reliance on solar or wind energy in the Northeast region is not feasible without massive energy storage which is between the 24.1%–28.3% and 17.4%–35.5% of the annual energy consumption for solar and wind respectively. The nationwide connectivity can reduce these storage needs to the national averages (22.4% for solar and 24.9% for wind) or perhaps further if all the power generation moves to the more favorable places; however, even in Texas (one of the most Southern state with the best solar and wind resources), there exists substantial spatial variability in renewable energy resources (Kumler et al., 2019).
The “total storage capacity” far exceeds the few hours of energy storage that is typically factored in (if at all) levelized cost analyses comparing different energy sources (Branker, Pathak, and Pearce, 2011; Lai et al., 2017). The lack of accounting for the severe power shortages associated with varied renewable resources is leading to renewables regularly beating nuclear energy in operational costs despite the much more reliable and steady power.
Recent analysis outlining the path to a 100% renewable future (Jacobson et al., 2015a; Jacobson et al., 2015b; Denholm et al., 2022) envisioned the use of geothermal and hydrogen storage. It is necessary to note that neither of these storage solutions were ever deployed at regional scales, and the ability of these solutions to scale up is unproven, leaving both studies in the realm of fiction rather than engineering.
The geothermal storage (Jacobson et al., 2015b) was based on a single experiment (Sibbitt et al., 2012) carried out in Alberta, Canada, that utilized a thermal solar system for heating and cooling where the excess heat from air conditioning in the summer was stored in boreholes and retrieved for heating in the winter. The expectation that a single experiment can be scaled to nationwide application is undoubtedly brave.
The anticipation of building out hydrogen storage facilities in scale by 2035 is similarly ambitious, but at least, the authors (Denholm et al., 2022) admit that such storage system does not exist in industrial scales.
4.1 Alternative renewable energy sources
Alternative energy sources such as hydropower or biofuels are far behind solar and wind power in abundance. Globally, the total potential energy of runoff landing on the continental surfaces is only 3.5 [TW] (based on the product of the annual discharge to oceans
Another way to understand the role that hydropower can play is to consider the aforementioned continental runoff expressed as
One also must realize the poor energy density of hydropower. The energy content of lifting 1 [l] that is 1 [kg] mass by 1 m has a potential energy content of 9.81 [J]. In contrast, warming up the same amount of water by one degree Celsius takes 4,184 [J]. It is worth noting that the heat capacity of liquid water varies more—as a function of its temperature—than its potential energy, but it is normally assumed to be constant. Warming up 1 [l] of water from room temperature (20 [°C]) to boiling (100 [°C]) to make a pot of coffee takes up as much energy as lifting up the same amount of water to
In order to estimate national and state-by-state hydropower potentials, water balance/transport models (WBMs) (Vörösmarty et al., 1989; Fekete et al., 2010; Wisser et al., 2010) were carried out for the CONUS domain using the NLDAS forcing data (Xia et al., 2012a; Cai et al., 2014; Zhang et al., 2020) using a gridded network derived from HydroSHEDS (Lehner et al., 2006) 1’ resolution grid on geographic coordinates.
The hydropower potential was computed from monthly mean discharge estimates for each grid cell of the simulated gridded network assuming steady-state flow conditions when the kinetic energy of the flow is constant and the energy loss due to friction is compensated by the loss of potential energy. Under such conditions, the energy that can be extracted is a portion of the lost potential energy by creating impoundments that reduce the flow velocity and in return reducing the frictional energy losses. As an upper estimate of the hydropower of all the rivers, the potential energy loss
Hydropower potential appears to have far more inter-annual variability than solar. The ratio of the median and the minimum annual average hydropower potential (that was termed as excess factor) is
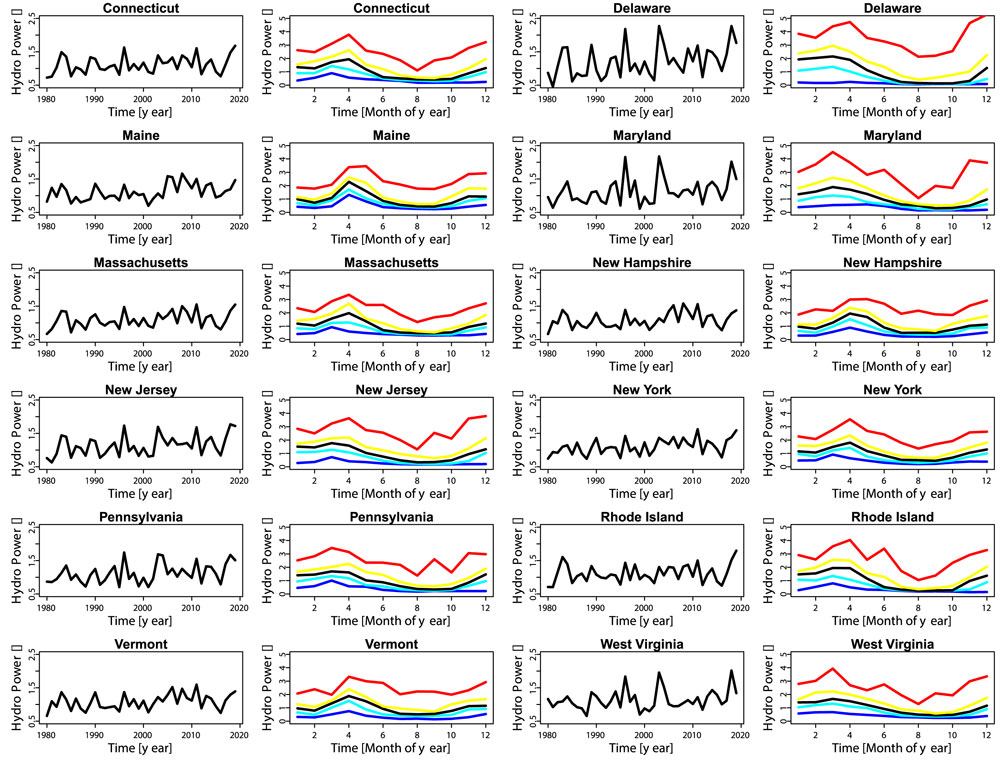
FIGURE 13. Inter-annual and seasonal variability of the normalized hydropower potential in the 12 Northeast states.
Table 2 shows the percentage of the annual consumption that hydropower can provide in the 12 Northeast states and over the CONUS. Maine, New Hampshire, and Vermont stand out with high potential hydropower which is misleading. Although these states are mountainous and in wet regions of the nation, they are also sparsely populated.
In addition to exploring the viability to complement solar and wind power generation with hydropower, plant growth modeling was performed by estimating the biofuel expressed as total energy that could be grown if all suitable croplands were converted to energy crops. This study utilized the crop modeling experiment carried out in this special issue (Lin et al., 2023). Since harvested crops are a form of energy storage, their energy content can be contrasted directly with the annual energy consumption to evaluate how that relates to the energy storage needed according to the cumulative surplus/deficit analysis.
The energy consumption of the CONUS and the 12 states was compared to the amount of biofuel that could be possibly grown in each state along with the hydropower potential (Table 2). It is important to note that the potential energy from biofuel and hydropower represents unrealistic extremes. Converting all croplands to energy crops or impounding all rivers to the point that they have no more potential energy to reach oceans is clearly impossible. It is safe to state that biofuel and hydropower cannot provide the missing energy to eliminate the significant energy storage for 100% reliance on solar or wind power generation. The CONUS value turns out far below the 12 states, which are clearly the wetter part of the country with more hydropower potential and crop production.
4.2 International outlook
In recent decades, Europe advanced aggressively in transitioning to renewable energy sources and reached high renewable penetration. Their experiences could serve as validation of the analyses presented in this paper. Europe in general resides higher north than most of the US territories. Benefiting from the Gulf current, Denmark, Finland, Norway, Scotland, and Sweden are significantly milder than comparable areas in Canada at the same latitude. Consequently, most European countries have less solar resources, particularly in the winter, than the United States.
The impact of the higher latitude is exacerbated further by more clouds in the winter period. Most of the aforementioned countries have 80% or more cloud cover in the winter, while few places reach 70% cloud cover in the United States according to the long-term mean monthly cloud cover from the Climate Research Unit of East Anglia (Mitchell et al., 2004).
Europe also invested heavily in the interconnectivity of the electric grid. European countries form the Continental Synchronous Area, which is the largest electrical grid in the world. This phase-locked electric grid maintains the same 50 Hz frequency across all participating nations and connects 24 countries, serving over 400 million people. The grid is steadily expanding and already has synchronized connections to Algeria, Morocco, Tunisia, and Turkey (Wikipedia, 2022). The expansion to the first three of these countries was motivated by plans to install large solar farms in deserts of Northern Africa where solar resources are far more plentiful than anywhere else in Europe.
The level of connectivity that the Continental Synchronous Area provides is valuable to shift excess power generation to places with power shortages. However, the degree to which it is capable in lowering the need for storage is limited. While the interconnectivity makes the grid more reliable most of the time, a sudden drop in power generation could trigger a snowball rolling event that potentially risks the operation of the entire grid. Europe already experienced such events (most recently on 8 January 2021) (Starn et al., 2021) resulting from a sudden increase in energy demand in Croatia, and similar collapses also happened in the past when renewables failed to deliver power.
The higher level of interconnectivity does not lead to higher energy security when the variabilities of power generation potentials of the connected regions are similar. This appears to be the case for wind resources around the North Sea (Buatois et al., 2014). When wind power generation is low in Scotland, it is often low everywhere else. The same is true for stormy weather such that when wind turbines are stopped around Denmark due to series of storms. They are likely experiencing high winds elsewhere around the North Sea.
The high penetration of renewables in electric power generation in Europe is enabled by fossil fuel (most notable natural gas) backup that is essentially serving as “battery.” This is evidenced by a recent vote in the European Union accepting natural gas (Cliford, 2022) in its “taxonomy of sustainable activities” (European Commission, 2020) in the middle of an energy crisis that is clearly emerging from Europe’s heavy reliance on natural gas imported from Russia.
Accepting natural gas as part of sustainable activities, the Council of the European Union has mandated its member states to maintain natural gas storage capacities that meet 35% of their annual gas consumption and recommended in 2022 to fill up these storages to 80% at a minimum as countries were heading into winter (European Council, 2022). Although this regulation is driven by the current usage of natural gas, the 35% appears to point to the similar magnitude for storage requirements that we found with cumulative deficit computation.
Hungary is among the countries with excess natural gas storage capacity where exhausted natural gas fields are converted into natural storage. While these storage facilities might serve as a sustainable green energy infrastructure in the future in storing hydrogen or some form of synthetic gas produced in the summer from excess power as an NREL study (Denholm et al., 2022) envisioned. Such energy storage likely would endure substantial energy losses, both during the production of the hydrogen or synthetic fuel and during their conversion back to mechanical power as electricity. When hydrogen or synthetic fuel is burned, they are also subject to the Carnot efficiency of thermal power generation; therefore, a hydrogen economy would need to add substantial excess power generation capacities to compensate for these energy losses.
4.3 Future directions
The presented work in our study is more of a proof of concept than a definite accounting that could be used for planning out the exact energy storage infrastructures. We are convinced that our approach is fundamentally solid, and it should be an integral part in future energy studies for outlining our truly “fossil fuel-free” future. Our team envisions three directions to refine the presented work.
First, utilizing the parameterization of the energy storage technologies—via recharge, discharge, and storage decay energy losses—can be applied to different energy storage pathways. For example, lithium batteries have modest recharge, discharge, or decay losses, but they are severely limited by the material requirements. Sodium have similar chemical properties to lithium but more abundant. While the energy density of sodium batteries is lower than lithium batteries, it is acceptable for grid-scale storage. Alternatively, aluminum batteries might also work well at grid scales. Aluminum batteries are not rechargeable, but recycling aluminum batteries might well serve seasonal power storage. In addition to batteries, synthetic fuels or hydrogen might also satisfy the energy storage needs.
The second direction is to study the seasonal variations in energy consumption and explore anticipated changes resulting from the shifting away from using fossil fuels to a full electrification of all the energy sectors. Combined with changing climate and consumer adoption of various technologies such as heat pumps and/or increasing demand for air conditioning, the seasonality of energy demands is likely to change considerably in future.
Third, the variability of renewable energy sources differs significantly spatially. Identifying places where the energy production is more in line with consumption could contribute to a significant reduction of the energy storage capacity needs. While the complete elimination is highly unlikely, any reduction in the energy storage needed by better sitting of the renewable deployment would be a significant step toward sustainable energy production.
In addition to the three directions to refine the present study, our team is also working on a thorough assessment of the roles that hydropower can play in providing energy storage. While the energy density of hydropower pales compared to solar or wind resources, there are a few places around the world where they can play significant roles. The unique geography of Norway allowed the installation of power generation turbines to existing lakes without much if any expansion of the inundated areas. Coastal areas around Scandinavia or Chile appear to be ideal locations for pumped storage possibly relying on sea water if the freshwater resources are limited.
Our plan is to revisit the Global Atlas of Closed-Loop Pumped Hydro Energy Storage (Stocks et al., 2021). This atlas identified 616,000 potential storage sites (with minimum
Assuming 18 h of operations producing
Another team attempting to assess the potential in pumped hydropower arrived to
5 Conclusion
Transitioning to sustainable green energy systems relying on renewable power sources is primarily driven by the anticipated catastrophes arising from climate change. The rapid decline of the cost of renewable energy sources—most notably solar and wind—has led to increasing optimism about the viability of a future relying 100% on renewables. In cost comparison to other forms of energy, it is customary to either neglect the intermittencies of renewable energy sources or assume that a few hours of energy storage will be sufficient to balance out periods when renewables fall short delivering power.
In the present study, modified cumulative surplus/deficit analysis (borrowed from water resource management practices to find the necessary storage capacity of future reservoirs) was adopted that included the energy losses occurring during recharging and discharging energy storage along with energy storage decay in the storage medium. This modified surplus/deficit analysis was applied to normalize the time series of solar radiation and wind speed records as “supply” along with state and nationwide energy consumption data in demand.
The key findings of our study are as follows:
1. Inter-annual variability is probably manageable via building modest excess capacity to ensure that the energy demands are met, even in those years when the availability of solar and wind resources were the lowest.
2. Seasonal variability can be handled only by sufficient “storage capacity” in the order of several months’ worth of energy use that far exceeds the customary few hours in levelized cost comparisons. The state-level “storage capacity” in the Northeast region is similar to the nationwide average.
3. A national grid will not be able to eliminate the need for energy storage but could enable the nation to strategically place renewable energy generation where the power generation is more favorable. In the case of the Northeast region, this would likely lead to moving much of the power generation to other parts of the nation.
4. Hydropower and/or biofuels will not have significant contribution to mitigate the intermittency of solar and wind.
The analyses presented in this study were complemented with an international outlook to countries with a high penetration of renewable power generation in Europe and confirmed our assertion that renewables can be deployed in the energy system only if sufficient backup energy sources are available that are currently provided by fossil fuels.
Natural gas is often viewed as a “bridge fuel” in our transition to a sustainable green economy, where natural gas with carbon capture and sequestration as a “firm clean energy” is playing the role of energy storage. Renewable energy sources today alone cannot meet all energy demands. Unless viable grid-scale energy storage solutions—which can store a quarter or more of the annual energy consumption—emerge, renewables plus fossil fuels such as natural gas (with or without carbon capture and sequestration) are bridges to nowhere.
Perhaps, it is time to revise our current focus on “decarbonization” and move toward a true “fossil fuel-free” future. “Fossil fuel-free” future would solve climate change as a by-product while taking the right step toward sustainability, unlike the clearly unsustainable “net-zero decarbonization” that still relies on fossil fuels via carbon capture and sequestration as “firm clean energy source.”
Data availability statement
Publicly available datasets were analyzed in this study. These data can be found at: https://ldas.gsfc.nasa.gov/nldas.
Author contributions
BF designed and implemented the cumulative deficit/surplus computations. MB provided the European outlook and the engineering oversight of the experiment designs. JZ carried out the water balance modeling and consecutive data processing to extract simulated hydropower generation and processed the energy consumption data. MC assembled and processed the climate data forcings for the solar radiation and wind speed analysis and for water balance modeling. All authors contributed to the article and approved the submitted version.
Funding
Financial support for this work was provided by the U.S. National Science Foundation’s Innovations at the Nexus of Food, Energy and Water Systems Program (INFEWS/T1 Grant #1856012).
Conflict of interest
Author MB was employed by the Hungarian Power Company.
The remaining authors declare that the research was conducted in the absence of any commercial or financial relationships that could be construed as a potential conflict of interest.
Publisher’s note
All claims expressed in this article are solely those of the authors and do not necessarily represent those of their affiliated organizations, or those of the publisher, the editors, and the reviewers. Any product that may be evaluated in this article, or claim that may be made by its manufacturer, is not guaranteed or endorsed by the publisher.
References
Arbabzadeh, M., Johnson, J. X., De Kleine, R., and Keoleian, G. A. (2015). Vanadium redox flow batteries to reach greenhouse gas emissions targets in an off-grid configuration. Appl. Energy 146, 397–408. doi:10.1016/j.apenergy.2015.02.005
Barlage, M., Mukul, T., Chen, F., Miguez-Macho, G., Yang, Z-L., and Niu, G-Y. (2015). The effect of groundwater interaction in north American regional climate simulations with WRF/Noah-MP. Clim. Change 129 (3–4), 485–498. doi:10.1007/s10584-014-1308-8
Branker, K., Pathak, M. J. M., and Pearce, J. M. (2011). A review of solar photovoltaic levelized cost of electricity. Renew. Sustain. Energy Rev. 15 (9), 4470–4482. doi:10.1016/j.rser.2011.07.104
Bromley, J., Brouwer, J., Barker, A. P., Gaze, S. R., and Valentin, C. (1997). The role of surface water redistribution in an area of patterned vegetation in a semi-arid environment, south-west Niger. J. Hydrology 198, 1–29. doi:10.1016/s0022-1694(96)03322-7
Buatois, A., Gibescu, M., Rawn, B., and van der Meijden, M. (2014). Analysis of North Sea offshore wind power variability. Resources 3 (2), 454–470. doi:10.3390/resources3020454
Cai, X., Yang, Z-L., Xia, Y., Huang, M., Wei, H., Ruby Leung, L., et al. (2014). Assessment of simulated water balance from noah, noah-MP, CLM, and VIC over CONUS using the NLDAS test bed. J. Geophys. Res. Atmos. 119 (24), 13,751–13,770. doi:10.1002/2014JD022113
Clack, C. T. M., Qvist, S. A., Apt, J., Morgan, B., Brandt, A. R., Caldeira, Ken, et al. (2017). Evaluation of a proposal for reliable low-cost grid power with 100% wind, water, and solar. Proc. Natl. Acad. Sci., 201610381. doi:10.1073/pnas.1610381114
Cliford, C. (2022). Europe will count natural gas and nuclear as green energy in some circumstances. CNBC 7, 2022. Available at: https://www.cnbc.com/2022/07/06/europe-natural-gas-nuclear-are-green-energy-in-some-circumstances-.html.
Cosgrove, B. A., Dag Lohmann, K. E. M., Houser, P. R., Wood, E. F., Schaake, J. C., Robock, A., et al. (2003). Real-time and retrospective forcing in the North American land data assimilation system (NLDAS) Project. J. Geophys. Res. Atmos. 108 (D22), 2002JD003118. doi:10.1029/2002JD003118
Craig, M. T., Jaramillo, P., Zhai, H., and Kelly, K. (2017). The economic merits of flexible carbon capture and sequestration as a compliance strategy with the clean power plan. Environ. Sci. Technol. 51 (3), 1102–1109. doi:10.1021/acs.est.6b03652
de Sisternes, F. J., Jenkins, J. D., and Botterud, A. (2016). The value of energy storage in decarbonizing the electricity sector. Appl. Energy 175, 368–379. doi:10.1016/j.apenergy.2016.05.014
Delucchi, M. A., and Jacobson, M. Z. (2011). Providing all global energy with wind, water, and solar power, Part II: reliability, system and transmission costs, and policies. Energy Policy 39 (3), 1170–1190. doi:10.1016/j.enpol.2010.11.045
Denholm, P., Arent, D. J., Baldwin, S. F., Bilello, D. E., Brinkman, G. L., Cochran, J. M., et al. (2021). The challenges of achieving a 100% renewable electricity system in the United States. Joule 5 (6), 1331–1352. doi:10.1016/j.joule.2021.03.028
Denholm, P., Brown, P., Cole, W., Mai, T., Sergi, B., Brown, M., et al. 2022. “Examining supply-side options to achieve 100% clean electricity by 2035.” NREL/TP-6A40-81644, 1885591, MainId:82417. doi:10.2172/1885591
Doubleday, K., Parker, A., Hafiz, F., Irwin, B., Hancock, S., Pless, S., et al. (2019). Toward a subhourly net zero energy district design through integrated building and distribution system modeling. J. Renew. Sustain. Energy 11 (3), 036301. doi:10.1063/1.5093917
Draxl, C., Hodge, B. M., Clifton, A., and McCaa, J. (2015). Overview and meteorological validation of the wind integration national dataset Toolkit. NREL/TP--5000-61740. doi:10.2172/12149851214985
EIA (2022). Annual energy outlook 2022. U.S. Energy Information Agency. Available at: https://www.eia.gov/outlooks/aeo/.
European Commission (2020). EU taxonomy for sustainable activities. Available at: https://finance.ec.europa.eu/sustainable-finance/tools-and-standards/eu-taxonomy-sustainable-activities_en.
European Council (2022). Council adopts regulation on gas storage. European Council, Council of the European Union. Available at: https://www.consilium.europa.eu/en/press/press-releases/2022/06/27/council-adopts-regulation-gas-storage/.
Fekete, B. M., Vörösmarty, C. J., and Grabs, W. (2002). High-resolution fields of global runoff combining observed river discharge and simulated water balances. Glob. Biogeochem. Cycles 16 (3), 15-1–15-10. doi:10.1029/1999GB001254
Fekete, B. M., Wisser, D., Kroeze, C., Mayorga, E., Bouwman, L., Wollheim, W. M., et al. (2010). Millennium ecosystem assessment scenario drivers (1970-2050): climate and hydrological alterations. Glob. Biogeochem. Cycles 24 (GB0A12). doi:10.1029/2009gb003593
GE Energy and National Renewable Energy Laboratory (NREL), Golden, CO (2010). Western wind and solar integration study. NREL/SR-550-47434. 981991. doi:10.2172/981991
Gleick, P. H., and Palaniappan, M. (2010). Peak water limits to freshwater withdrawal and use. Proc. Natl. Acad. Sci. U. S. A. 107 (25), 11155–11162. doi:10.1073/pnas.1004812107
Guerra, O. J., Zhang, J., Eichman, J., Paul, D., Kurtz, J., and Hodge, B-M. (2020). The value of seasonal energy storage technologies for the integration of wind and solar power. Energy & Environ. Sci. 13 (7), 1909–1922. doi:10.1039/D0EE00771D
Hodge, B-M., Carlo, B. M-A., Wang, Q., Chartan, E., Anthony, F., and Kiviluoma, J. (2018). The combined value of wind and solar power forecasting improvements and electricity storage. Appl. Energy 214, 1–15. doi:10.1016/j.apenergy.2017.12.120
Holttinen, H., Kiviluoma, J., and Helistö, N. (2021). Design and operation of energy systems with large amounts of variable generation. VTT Technol. FI VTT Tech. Res. Centre Finl. doi:10.32040/2242-122X.2021.T396
Huang, S., Hodge, B-M. S., Xiao, J., Reklaitis, G. V., and Pekny, J. F. (2011). The effects of electricity storage on large scale wind integration. Comput. Aided Chem. Eng. 29, 1879–1883. Elsevier. doi:10.1016/B978-0-444-54298-4.50154-9
Hunt, J. D., Byers, E., Wada, Y., Parkinson, S., DavidGernaat, E. H. J., Langan, S., et al. (2020). Global resource potential of seasonal pumped hydropower storage for energy and water storage. Nat. Commun. 11 (1), 947. doi:10.1038/s41467-020-14555-y
Jacobson, M. Z., and Delucchi, M. A. (2011). Providing all global energy with wind, water, and solar power, Part I: technologies, energy resources, quantities and areas of infrastructure, and materials. Energy Policy 39 (3), 1154–1169. doi:10.1016/j.enpol.2010.11.040
Jacobson, M. Z., Delucchi, M. A., Bazouin, G., Bauer, Z. A. F., Heavey, C. C., Fisher, E., et al. (2015a). 100% clean and renewable wind, water, and sunlight (WWS) all-sector energy roadmaps for the 50 United States. Energy & Environ. Sci. 8 (7), 2093–2117. doi:10.1039/C5EE01283J
Jacobson, M. Z., Delucchi, M. A., Cameron, M. A., and Frew, B. A. (2015b). Low-cost solution to the grid reliability problem with 100% penetration of intermittent wind, water, and solar for all purposes. Proc. Natl. Acad. Sci. 112 (49), 15060–15065. doi:10.1073/pnas.1510028112
Johnson, J. X., De Kleine, R., and Keoleian, G. A. (2014). Assessment of energy storage for transmission-constrained wind. Appl. Energy 124, 377–388. doi:10.1016/j.apenergy.2014.03.006
Keskar, A., Galik, C., and Johnson, J. X. (2023). Planning for winter peaking power systems in the United States. Energy Policy 173, 113376. doi:10.1016/j.enpol.2022.113376
Kroposki, B., Johnson, B., Zhang, Y., Gevorgian, V., Paul, D., Bri-Mathias, H., et al. (2017). Achieving a 100% renewable grid: operating electric power systems with extremely high levels of variable renewable energy. IEEE Power Energy Mag. 15 (2), 61–73. doi:10.1109/MPE.2016.2637122
Kumler, A., Losada Carreño, I., Craig, M. T., Bri-Mathias, H., Cole, W., and Brancucci, C. (2019). Inter-annual variability of wind and solar electricity generation and capacity values in Texas. Environ. Res. Lett. 14 (4), 044032. doi:10.1088/1748-9326/aaf935
Lai, C. S., Jia, Y., Zhao, X., Lai, L. L., Li, X., Cao, J., et al. (2017). Levelized cost of electricity for photovoltaic/biogas power plant hybrid system with electrical energy storage degradation costs. Energy Convers. Manag. 153, 34–47. doi:10.1016/j.enconman.2017.09.076
Lehner, B., Verdin, K. L., and Jarvis, A. (2006). Hydrological data and maps based on shuttle elevation derivatives at multiple scales (HydroSHEDS). US: World Wildlife Fund.
Lin, T-S., Kheshgi, H. S., Song, Y., Vörösmarty, C. J., and Jain, A. K. (2023). Which crop has the highest bioethanol yield in the United States? Frontiers in Energy Research 11, 1070186. doi:10.3389/fenrg.2023.1070186
Lin, Y., Johnson, J. X., and Mathieu, J. L. (2016). Emissions impacts of using energy storage for power system reserves. Appl. Energy 168, 444–456. doi:10.1016/j.apenergy.2016.01.061
Loftus, P. J., Armond, M. C., Long, J. C. S., and Jenkins, J. D. (2015). A critical review of global decarbonization scenarios: what do they tell us about feasibility? WIREs Clim. Change 6 (1), 93–112. doi:10.1002/wcc.324
MacKay, D. J. C. (2009). Sustainable energy - without the hot air. Cambridge, United Kingdom: UIT Cambridge Ltd.
Maclaurin, G., Grue, N., Lopez, A., Heimiller, D., Rossol, M., Grant, B., et al. (2019). The renewable energy potential (ReV) model: A geospatial platform for technical potential and supply curve modeling. NREL/TP-6A20-73067, 1563140, MainId, 13369. doi:10.2172/1563140
Mitchell, T. D., Carter, T. R., Jones, P. D., Hulme, M., and New, M. (2004). A comprehensive set of high-resolution grids of monthly climate for Europe and the Globe: The observed record (1901-2000) and 16 scenarios (2001-2100). July. Tyndall Centre for Climate Change Research. Available at: http://www.ipcc-data.org/docs/tyndall_working_papers_wp55.pdf.
Murphy, T. W. (2021). Energy and human ambitions on a finite planet. doi:10.21221/S2978-0-578-86717-5
Niu, G-Y., Yang, Z-L., Mitchell, K. E., Chen, F., Michael, B. E. K., Barlage, M., et al. (2011). The community noah land surface model with multiparameterization options (Noah-MP): 1. Model description and evaluation with local-scale measurements. J. Geophys. Res. 116 (D12), D12109. doi:10.1029/2010JD015139
Obama, B. (2017). The irreversible momentum of clean energy. Science 355 (6321), 126–129. doi:10.1126/science.aam6284
Rose, S. K., Jaramillo, P., Mitchell, J. S., Grossmann, I., and Apt, J. (2012). Quantifying the hurricane risk to offshore wind turbines. Proc. Natl. Acad. Sci. U. S. A., 1–6. doi:10.1073/pnas.1111769109
Ryu, J-H., and Hodge, B-M. (2016). Mathematical modelling-based energy system operation strategy considering energy storage systems. Comput. Aided Chem. Eng. 38, 1455–1460. Elsevier. doi:10.1016/B978-0-444-63428-3.50247-2
Sepulveda, N. A., Jenkins, J. D., De Sisternes, F. J., and Lester, R. K. (2018). The role of firm low-carbon electricity resources in deep decarbonization of power generation. Joule 2 (11), 2403–2420. doi:10.1016/j.joule.2018.08.006
Sibbitt, B., McClenahan, D., Djebbar, R., Thornton, J., Wong, B., Carriere, J., et al. (2012). The performance of a high solar fraction seasonal storage district heating system – five years of operation. Energy Procedia 30, 856–865. doi:10.1016/j.egypro.2012.11.097
Starn, J., Perkin, B., and Vilcu, I. (2021). The day Europe’s power grid came close to a massive blackout. Bloom. January 27, 2021. Available at: https://www.bloomberg.com/news/articles/2021-01-27/green-shift-brings-blackout-risk-to-world-s-biggest-power-grid.
Stocks, M., Ryan, S., Lu, B., Cheng, C., and Blakers, A. (2021). Global atlas of closed-loop pumped hydro energy storage. Joule 5 (1), 270–284. doi:10.1016/j.joule.2020.11.015
Tong, D., Farnham, D. J., Duan, L., Zhang, Q., Lewis, N. S., Caldeira, K., et al. (2021). Geophysical constraints on the reliability of solar and wind power worldwide. Nat. Commun. 12 (1), 6146. doi:10.1038/s41467-021-26355-z
Vörösmarty, C. J., Melillo, J. M., Wuebbles, D. J., Jain, A. K., and Ando, A. W. (2023a). Applying the framework to study climate-induced extremes on food, energy, and water systems (C-FEWS): the role of engineered and natural infrastructures, technology, and environmental management in the United States Northeast and Midwest. Front. Environ. Sci. Rev 11, 186. doi:10.3389/fenvs.2023.1070144
Vörösmarty, C. J., Melillo, J. M., Wuebbles, D. J., Jain, A. K., and Ando, A. W. (2023b). The C-FEWS framework: supporting studies of climate-induced extremes on food, energy, and water systems at the regional scale. Front. Environ. Sci. Rev 11, 13. doi:10.3389/fenvs.2023.1069613
Vörösmarty, C. J., Moore, B., Grace, A. L., Gildea, M. P., Jerry, M. M., Steudler, P. A., et al. (1989). Continental scale models of water balance and fluvial transport: An application to south America. Glob. Biogeochem. Cycles 3 (9), 241–265. doi:10.1029/gb003i003p00241
Wikipedia (2022). Synchronous grid of continental Europe. Wikipedia. Available at: https://en.wikipedia.org/wiki/Synchronous_grid_of_Continental_Europe September 3, 2022).
Wisser, D., Balázs, M. F., Vörösmarty, C. J., and Andreas, H. S. (2010). Reconstructing 20th century global hydrography: A contribution to the global terrestrial network- hydrology (gtn-H). Hydrology Earth Syst. Sci. 14 (1), 1–24. doi:10.5194/hess-14-1-2010
Wu, C., Zhang, X-P., and Sterling, M. (2022). Solar power generation intermittency and aggregation. Sci. Rep. 12 (1), 1363. doi:10.1038/s41598-022-05247-2
Xia, Y., Mitchell, K., Michael, E. K., Cosgrove, B., Sheffield, J., Luo, L., et al. (2012a). Continental-scale water and energy flux analysis and validation for north American land data assimilation system Project phase 2 (NLDAS-2): 2. Validation of model-simulated streamflow: validation of model-simulated streamflow. J. Geophys. Res. Atmos. 117 (D3), n/a. doi:10.1029/2011JD016051
Xia, Y., Mitchell, K., Michael, E. K., Justin, S., Cosgrove, B., Wood, E., et al. (2012b). Continental-scale water and energy flux analysis and validation for the North American land data assimilation system Project phase 2 (NLDAS-2): 1. Intercomparison and application of model products: water and energy flux analysis. J. Geophys. Res. Atmos. 117 (D3), n/a. doi:10.1029/2011JD016048
Zhang, B., Xia, Y., Long, B., Hobbins, M., Zhao, X., Hain, C., et al. (2020). Evaluation and comparison of multiple evapotranspiration data models over the contiguous United States: implications for the next phase of NLDAS (NLDAS-Testbed) development. Agric. For. Meteorology 280, 107810. doi:10.1016/j.agrformet.2019.107810
Keywords: renewables, intermittency, energy storage, energy generation, energy demand
Citation: Fekete BM, Bacskó M, Zhang J and Chen M (2023) Storage requirements to mitigate intermittent renewable energy sources: analysis for the US Northeast. Front. Environ. Sci. 11:1076830. doi: 10.3389/fenvs.2023.1076830
Received: 22 October 2022; Accepted: 29 August 2023;
Published: 18 September 2023.
Edited by:
Pietro Elia Campana, Mälardalen University, SwedenReviewed by:
Surendra Poonia, Central Arid Zone Research Institute (ICAR), IndiaDiyi Chen, Northwest A&F University, China
Nate Blair, US National Renewable Energy Laboratory, United States
Copyright © 2023 Fekete, Bacskó, Zhang and Chen. This is an open-access article distributed under the terms of the Creative Commons Attribution License (CC BY). The use, distribution or reproduction in other forums is permitted, provided the original author(s) and the copyright owner(s) are credited and that the original publication in this journal is cited, in accordance with accepted academic practice. No use, distribution or reproduction is permitted which does not comply with these terms.
*Correspondence: Balázs M. Fekete, bfekete@ccny.cuny.edu