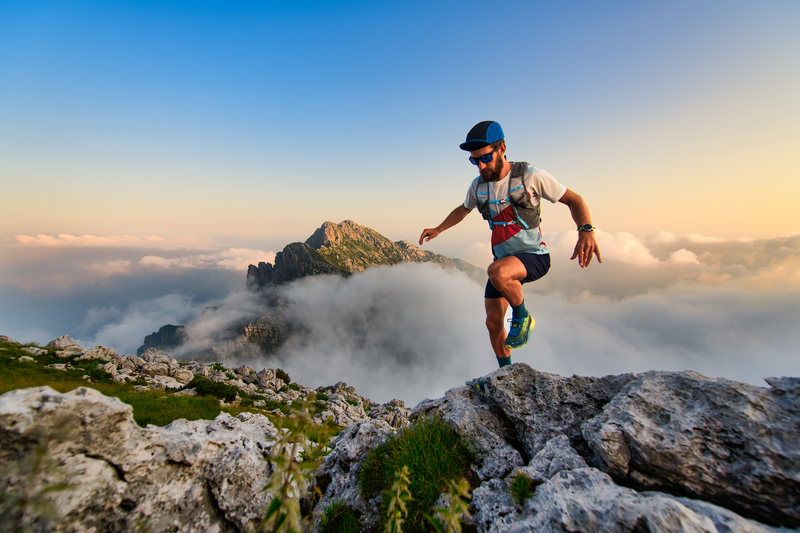
95% of researchers rate our articles as excellent or good
Learn more about the work of our research integrity team to safeguard the quality of each article we publish.
Find out more
ORIGINAL RESEARCH article
Front. Environ. Sci. , 30 May 2023
Sec. Freshwater Science
Volume 11 - 2023 | https://doi.org/10.3389/fenvs.2023.1051295
This article is part of the Research Topic Threatened Aquatic Gems: Freshwater Springs and Groundwater-Dependent Ecosystems View all 9 articles
Despite the close attention springs have received from a hydrologic perspective and as biodiversity hotspots, the multiple dimensions of spring meiofaunal assemblage diversity are still poorly investigated. Knowledge of beta diversity patterns and drivers can inform and improve management decisions on biodiversity conservation. Here, we analyzed beta diversity of copepod assemblages in karst springs in Central Italy by focusing on: 1) relative contributions of turnover and nestedness components to taxonomic and phylogenetic beta diversity; 2) temporal variation of species richness and beta diversity within and between the target springs in conjunction with models of the influence of physical-chemical parameters on within-spring diversity changes; 3) expected risk of habitat loss due to variation in groundwater recharge under climate change. To this end, we gathered data from 168 samples collected in four karst springs from 2004 to 2016. Overall, we found 48 copepod species, 22 of which are obligate groundwater dwellers while the remaining 26 usually occur in surface freshwaters. All springs showed significant changes in taxonomic and phylogenetic beta diversity over time. Total beta diversity was high for both the taxonomic and phylogenetic dimensions, and turnover was the main component. Inter-site variability in dissolved oxygen explained a noticeable part of temporal variation in beta diversity, likely reflecting the role of microhabitat heterogeneity in shaping site-specific assemblages. However, most of the temporal variation in species richness and beta diversity remained unexplained, suggesting a major role of other factors, such as seasonal discharge variations. Modelling of recharge rates for all the four springs over 2001–2020 suggested a potential >40% recharge deficit under dry conditions. Moreover, Cellular Automata-based modelling of rainfall over the Gran Sasso-Sirente hydrogeologic unit (feeding three of the four springs) predicted an overall precipitation decrease in the 2081–2095 period. Such changes could produce severe effects on springs’ microhabitats and related communities. Our results indicate that partitioning beta diversity, monitoring its temporal changes and assessing its environmental drivers are critical to evidence-based conservation of springs. Particularly, the high species turnover we have observed suggests that conservation strategies should seek to preserve as many microhabitats as possible within and among karst springs.
Groundwater ecosystems have been scarcely investigated compared to their surface counterparts (Alley et al., 2016; Boulton, 2020; Tickner et al., 2020; Iannella et al., 2021; Visser et al., 2022), although the two are closely interdependent and, according to Famiglietti (2014), they should be conjunctively managed as “one water”. This lack of attention translates into a noticeable knowledge gap about the ecological processes shaping biodiversity at the interfaces between groundwater and surface water environments. Springs are groundwater-dependent ecosystems, which provide relevant ecosystem services to human wellbeing (e.g., provisioning of drinking water), especially in arid and semiarid regions of the world (Khorrami and Malekmohammadi, 2021), and host a multifaceted biodiversity (Cantonati et al., 2012; Cantonati et al., 2020; Cantonati et al., 2022). Springs represent evolutionary refugia, hosting relics of ancient lineages, particularly concerning obligate groundwater-dwelling organisms (i.e., stygobites), whose existing representatives have persisted in these habitats across geologic timescales under generally stable conditions (Davis et al., 2013). On the other hand, springs also act as ecological refuges for epigean freshwater species, which may find shelter at the spring mouths in drying periods (Meyer and Meyer, 2000; Cartwright and Johnson, 2018; Cartwright et al., 2020). The hydrological regime of karst springs depends on aquifers whose discharge may vary seasonally, determining temporal variations in the arrangement of microhabitats and biological assemblages (Fiasca et al., 2014; Galassi et al., 2014; Stoch et al., 2015; Fattorini et al., 2017; 2018; Di Lorenzo et al., 2018). Springs are generally characterized by a constant thermal regime, although increases in spring water temperature due to global warming have already been reported (Jyväsjärvi et al., 2015). The ongoing modifications of precipitation regimes and topology due to anthropogenic climate change may directly influence the hydrological regime of springs, aquifers’ recharge (Eckhardt and Ulbrich, 2003), springs’ discharge (Sivelle et al., 2021) and water resource availability (Hsu et al., 2007; Owor et al., 2009; Taylor et al., 2013). The current climate-related pressures, along with the excess of water abstraction for human consumption, will likely lead not only to modifications of water quantity and quality in springs but also to a high risk of biodiversity loss. In this context, springs fed by small aquifers are more prone to dramatic ecological changes than those depending on large aquifers, characterized by a higher groundwater storage (Kløve et al., 2014; Fattorini et al., 2016).
Since the seminal work published by Odum (1957), springs have been considered ideal environments to analyze biodiversity patterns and the ecological processes at their base (Kreiling et al., 2022). The relative contributions of ecological processes and the assemblage composition of springs are likely to be diverse because geographic isolation is a common feature of spring environments (Davis et al., 2013). Accordingly, springs may function as “inland islands” (Fattorini et al., 2016) showing a high degree of endemism as they often host species with very narrow, or even spot-like, distributions. Moving the analysis to the intra-spring scale, the patchiness of microhabitats allows identifying “islands within the islands”, where each patch hosts a fauna noticeably different from the neighboring ones, even when closely adjacent (Fiasca et al., 2014; Stoch et al., 2015; Mori et al., 2018).
All these features make springs particularly suitable to investigate beta diversity patterns and the role of environmental and temporal factors in shaping them. Beta diversity, generally defined as the degree of inter-site change in assemblages’ composition (Whittaker, 1960; Baselga, 2012), represents a key conceptual tool in the assessment of ecosystems’ responses to natural (e.g., catastrophic events) and/or human-induced (e.g., overexploitation, pollution) stressors (Mori et al., 2018). Nonetheless, the application of beta diversity analyses to springs has been scant so far (e.g., Youssef et al., 2010; Schweiger and Beierkuhnlein, 2014; Cíbik et al., 2022).
In this contribution, we analyzed the beta diversity of meiofauna assemblages within four karst springs in the Central Apennines (Italy) and assessed the risk of losing part of this biodiversity due to climate change. We focused on copepods (Crustacea: Copepoda), which are among the major components of the freshwater meiofauna (Galassi et al., 2009a). First, we analyzed two components (turnover and nestedness-derived diversity; Baselga, 2010) of taxonomic and phylogenetic beta diversities. Second, we assessed the abiotic factors potentially driving the temporal variation of the observed beta diversity. Finally, we evaluated how the effects of climate change likely will impact the hydrogeologic setting defining springs’ recharge areas and the discharge regimes. The information presented in this study could help in optimizing the cost-effect ratio in the conservation management of karst springs.
We analyzed four perennial karst springs in Central Apennines (Abruzzo region, Italy) (Figure 1). The Capo Pescara-Santa Liberata spring at 250 m a.s.l. represents the main karst spring in Central Italy, with a mean annual discharge (hereafter mean discharge) of 7 m3 s-1. The spring is rheo-limnocrene (Di Sabatino et al., 2003; Cantonati et al., 2022) with high habitat heterogeneity represented by a limnocrenic sector (mainly defined by sand and gravel, and patches of hygrophilous vegetation), and rheocrenic areas, with patchy distribution of sediments, mosses and macrophytes (Stoch et al., 2015). The Presciano spring is located at 330 m a.s.l. at the contact between the karst bedrock of the aquifer and Quaternary alluvial deposits. It has a mean discharge of 2 m3 s-1 (Fiasca et al., 2014; Galassi et al., 2014) and is highly heterogeneous, with lentic sectors alternating with karst fractures with rheocrenic facies. Hygrophilous vegetation is abundant almost everywhere in the spring. The Cavuto spring is located at 550 m a.s.l. and represents the headwaters of the Sagittario River, with a mean discharge of 1.9 m3 s-1. Habitat heterogeneity is defined by rheocrenic sectors alternating with small groundwater-fed “ponds”. Mosses and macrophytes alternate with a sand-gravel substrate. Finally, the Chiarino spring, located in Monte Corvo, is a high-altitude spring (1250 m a.s.l.) with a mean discharge of 0.08 m3 s-1. Several karst fractures are present, and the groundwater flows downstream in the deeply incised Chiarino Valley, as a typical rheocrene spring. Habitat heterogeneity is mainly described by the bedrocks at the spring mouths, sand and gravel in the hypocrenon (the transitional zone between spring and spring-fed rivulet after Cantonati et al., 2022). The submerged vegetation is composed by mosses, which predominantly cover the bedrocks at the spring mouths. Additional details about the geographical setting of the analyzed springs are given in Table 1; pictures showing some of their main environmental features are reported in Figure 2.
FIGURE 2. The four springs investigated in this study. Top-left: Capo Pescara-Santa Liberata spring; top-right: Presciano spring; bottom-left: Chiarino spring; bottom-right: Cavuto spring.
Location of the studied springs in the Abruzzo region. Coordinates are reported using the WGS84/UTM zone 33 N projected coordinate system. CP_SL indicates the Capo Pescara-Santa Liberata spring.
The Capo Pescara-Santa Liberata, Presciano and Chiarino springs are fed by the Gran Sasso hydrogeologic unit, while Cavuto spring is fed by the Montagna Grande unit. The Gran Sasso hydrostructure is a calcareous-karst aquifer with a total extension of approximately 1000 km2, and its mean infiltration rate is approximately 600 mm yr-1 (Lorenzi et al., 2022). The regional groundwater table with a mean hydraulic gradient of 0.5%–2% (Amoruso et al., 2013) feeds a total discharge ranging from 18 m3 s-1–25 m3 s-1 from its springs, including a highway tunnel drainage tapped for drinking water on both sides.
The Gran Sasso aquifer has no clear groundwater divides, thus limiting the assessment of the recharge areas feeding each spring. On the northern slope of the Gran Sasso Massif, local sub-basins are supposed to recharge the Chiarino spring. On the southern slope of the massif, the recharge area of Campo Imperatore (about 75% of the recharge rate) is supposed to feed more than one spring. Indeed, the south-eastern spring groups of the Tirino River Valley (including the Presciano spring) and of Sulmona-Popoli Basin (which partly feeds the Capo Pescara-Santa Liberata spring) have a partial common recharge area, because of the diverging flow paths from the core of the massif (i.e., Campo Imperatore basin) to the south-eastern boundary of the aquifer. A peculiar hydrogeologic setting defines the Capo Pescara-Santa Liberata spring, which is fed both by the Gran Sasso aquifer and the Sirente Mountains aquifer (Stoch et al., 2015).
The Montagna Grande aquifer has a similar setting, feeding springs located at the boundary of the carbonate fractured system. The main hydrographic basin is the Tasso-Sagittario River, which is fed by streambed springs until the main basal spring of the Sagittario Gorges, namely, the Cavuto spring. The spring recharge area is clearly defined by the tectonic line which separates the northern hydrogeologic sub-basin that feeds the Cavuto spring from the remaining sector of the aquifer drained by other springs. The recharge area has an extension of about 60 km2 and the infiltration rate is about 1000 mm yr-1 (Boni and Rusi, 2005).
Sampling campaigns were carried out in different periods. The Presciano spring was sampled in January 2004 (5 sites), March 2004 (5 sites), July 2004 (5 sites), September 2004 (5 sites), January 2012 (5 sites), March 2012 (5 sites), July 2012 (7 sites), and October 2014 (7 sites). The Capo Pescara-Santa Liberata (hereafter CP_SL) spring was sampled in August 2010 (6 sites), September 2010 (3 sites), March 2011 (7 sites), July 2011 (3 sites), November 2011 (7 sites), May 2012 (6 sites), September 2012 (7 sites), January 2013 (11 sites), and March 2015 (11 sites). The Cavuto spring was sampled in October 2006 (6 sites), December 2006 (6 sites), February 2007 (4 sites), March 2007 (5 sites), October 2014 (8 sites), and March 2016 (8 sites). Finally, the Chiarino spring was sampled in July 2014 (6 sites), August 2014 (10 sites), May 2015 (10 sites).
All the microhabitats detectable were sampled (karst fractures, sediments devoid of vegetation, sediments with macrophytes, submerged mosses) and, where available, running waters from the rheocrenic sites and lentic waters from the limnocrenic sites. The surveys concerned both inbenthic (i.e., the topmost sediments at a maximum depth of 10 cm) and subsurface (at a depth of 40 cm) sites for each spring. Inbenthic samples were collected with a Hess sampler (mesh size: 60 μm; diameter: 40 cm) by washing the sediments contained in the cylinder and moving the sediment particles under the current generated. The finest grains and the fauna dislodged were thus collected into the downstream net (Dole-Olivier et al., 2014). Subsurface samples were collected by hammering mobile steel piezometers with a 5 mm-hole-screened tip within the sediment at a depth of about 40 cm; they were then connected to a membrane pump according to Malard et al. (2004). Ten liters of interstitial water were extracted, filtered with a 60-μm-mesh net, and fixed in a 70% alcohol solution. After sorting the invertebrates collected, the copepods were identified to the species level and classified as stygobites or non-stygobites according to their degree of adaption to the groundwater life (e.g., miniaturization, depigmentation, anophthalmy, mature females with larger but fewer eggs than their epigean counterparts, according to Galassi et al., 2009a).
We used a multiparametric probe (WTW Multi 3430 SET G) to measure, in the field, electrical conductivity at 25°C (µS cm-1), pH, dissolved oxygen (mg L-1), and temperature (°C) of water collected from each sampling site at each time and spring. To exclude the presence of a chemical contaminant affecting the patterns of species richness and beta diversity, we measured, for a subset of 48 sites encompassing all the four springs, the concentrations of 108 contaminants related to anthropogenic pressures (uncontrolled municipal waste landfills, intensive agriculture and farming, urban settlements, industries) occurring in the recharge areas of the springs. The targeted chemicals were sulphates, phosphates (method: APAT CNR IRSA 4020 Man 29 2003), nitrates, nitrites, ionized ammonium (method: 135 APAT CNR IRSA 4020 Man 29 2003), metals (method: EPA 3005A 1992+ EPA 6010C/APAT CNR IRSA 3150C Man 29), pesticides (method: EPA 3510C 1996+ EPA 8270D 2007), volatile organic compounds (method: EPA 5030C 2003+ EPA 8260C 2006; EPA 8260 C 2006), and hydrocarbons (method: EPA 3510C 1996+ EPA 8270D 2007). Their concentration was measured in the laboratory by retaining a 2-L sample for each sampling site.
All the analyses subsequently described were performed using the R software version 4.2.0 (R Core Team, 2022).
Total, stygobite, and non-stygobite copepod species richness were calculated for all the sampling sites and sampling dates. To assess the potential influence of in situ physical-chemical parameters (water temperature, electrical conductivity, pH and dissolved oxygen) on the temporal dynamics of species richness within the target springs, we used random intercept Generalized Linear Mixed Models (GLMMs), setting spring identity as a random effect, through the “lme4” package (Bates et al., 2015). In the mixed models, the fixed effects included the explanatory variables (and possibly their interactions) presumed to directly affect the response variable, while the random effects accounted for the portion of data variability deriving from inter-site relatedness along unmeasured dimensions (e.g., geographic proximity, Zuur et al., 2009). Different sites from a single spring were likely to be more similar in terms of biotic assemblages than sites from different springs; therefore, setting spring identity as random effect allowed us to model environment-richness and environment-beta diversity (see below) relationships taking this autocorrelation structure into account.
Before fitting the models, we applied the data exploration protocol according to Zuur et al. (2010). The distributions of the explanatory (i.e., physical-chemical parameters) and response (i.e., richness values) variables were inspected, and possible outliers in the dataset were detected and removed when they were likely to derive from data recording errors. Then, a stepwise Variance Inflation Factor (VIF) analysis was performed through the “usdm” package (Naimi et al., 2014) to lower multicollinearity among the explanatory variables, which could lead to incorrect estimation of the coefficients of the fixed effects and related p-values (Zuur et al., 2010; Cerasoli et al., 2021). Specifically, VIF = 3 was set as the threshold VIF score for variables’ retention (Zuur et al., 2010).
Once the set of non-collinear physical-chemical parameters was identified, an initial Poisson GLMM was fitted on these parameters, also including the corresponding interaction terms. To facilitate model convergence, the values of the physical-chemical parameters were scaled (i.e.,
Successively, we performed a simulation-based model validation on the refined GLMM (i.e., the model obtained at the end of the variables’ selection phase). Specifically, we used the “simulateResiduals” function from the “DHARMa” (Hartig, 2022) package to simulate new data from the fitted model and successively derive scaled residuals by comparing the simulated data with observed values. This process was repeated 500 times and the resulting Q-Q plot and scatterplot of scaled residuals versus model predictions were examined to confirm uniformity of residuals and the absence of overdispersion. In case of remaining uniformity or overdispersion issues, we fitted again the GLMM on the same set of predictors by using a negative binomial distribution instead of Poisson distribution. The simulation-based validation was then applied to the newly obtained model to verify the absence of any remaining issues.
After the model selection and validation steps, we used the “sjPlot” package (Lüdecke, 2022) to extract from the final model: (i) estimated value, standard error, 95th percentile confidence interval and associated p-value for each fixed effect term; (ii) variance attributed to the random effect; (iii) Nakagawa’s pseudo-R2 (Nakagawa et al., 2017); (iv) AICc and log-Likelihood scores; (v) marginal effect plots for the fixed effect terms resulting as statistically significant (i.e., p-value ≤ 0.05).
We used the “betapart” (Baselga et al., 2022) package to investigate within-spring temporal variation in taxonomic and phylogenetic beta diversity. Total beta diversity and its two components, turnover and nestedness-derived diversity (hereafter nestedness), were first measured using the corresponding multiple-site dissimilarity metrics as in Baselga (2012). Calculation of the beta diversity components was performed for each spring and sampling date through the “beta.multi” function and using the Sørensen family of dissimilarity indices (i.e., BetaSIM for turnover, BetaSNE for nestedness, and BetaSOR for total beta diversity).
To assess the statistical significance (considering α = 0.05 as threshold for Type I error) of the observed variation in beta diversity over time, we implemented a random resampling approach to derive a distribution of values for each of the above-mentioned diversity metrics, computed on random subsets of the available sites. This analysis was carried out, for each spring, using the “beta.sample” function. Specifically, 30 iterations of the resampling procedure were run, considering only the sampling dates for which at least 7 different sites were sampled: in this way, 35 unique combinations of four distinct sites were extracted for each of the considered sampling dates; 30 out of these 35 unique combinations of sites were then randomly chosen to compute multiple-site beta diversity metrics. Statistically significant differences among the distributions of dissimilarity values obtained for the different sampling dates were assessed, for each diversity metric, using non-parametric tests because preliminary linear models did not fulfill the normality, homoscedasticity, and independence assumptions (Zuur et al., 2009). For the Cavuto, Chiarino, and Presciano springs, only two sampling dates included enough sites to perform the resampling procedure. Therefore, for these springs we carried out two-sided Mann-Whitney U tests to compare the distributions of resampled beta diversity values from the two considered dates. For the CP_SL spring we had five sampling dates (March 2011, November 2011, September 2012, January 2013, and March 2015) with at least 7 sampled sites. Thus, we first performed a Kruskal-Wallis Rank Sum Test for each diversity metric, to check for statistically significant differences among the five sampling dates. Then, to compare each pair of sampling dates, we performed post hoc two-sided Mann-Whitney U tests with Benjamini and Hochberg (1995) p-value correction.
The phylogenetic counterparts of the above-mentioned beta diversity metrics were computed through the “phylo.beta.multi” function of the “betapart” package. As a proxy of phylogenetic information to be included in this calculation, we derived an ultrametric phylogram of the copepod species recorded in the studied springs, based on Linnean taxonomy (Supplementary Figure S1), through the “ape” (Paradis and Schliep, 2019) package. The tree was rooted in the Harpacticoida order, following previous studies indicating the Harpacticoida as more basal than the Cyclopoida (Eyun, 2017; Bernot et al., 2021).
To assess if, and to what extent, the physical-chemical conditions of the springs influenced variation in the components of taxonomic and phylogenetic beta diversity, we initially fitted GLMMs with beta distribution (as beta diversity metrics are bounded between 0 and 1) and logit link functions through the “glmmTMB” (Brooks et al., 2017) package. The candidate explanatory variables included were the maximum, minimum and standard deviation values of temperature, pH and dissolved oxygen computed, for each spring and sampling date, across the sampled sites. This choice permitted contrast of the beta diversity observed at each sampling date with the corresponding inter-site variability and extreme values of the physical-chemical parameters. Within the resulting set of “summary” physical-chemical parameters, the ones not affected by multicollinearity were selected through the same VIF-based approach used for the species richness modelling.
As for the GLMM built for species richness, explanatory variables were scaled before model fitting. A different model was fitted for each possible combination of two explanatory variables and corresponding two-way interaction term. The sample size was relatively low (n = 26, corresponding to the spring × sampling date combinations), so that including more than two explanatory variables would have likely hampered model convergence. Nonetheless, preliminary model fitting and validation runs still showed convergence issues and unreliable estimations of model parameters (e.g., negative residual and random effect variances). Thus, we moved from the initial GLMMs to Linear Mixed Effect (LME) models with normal distribution, fitted through the “lme4” package. Among the LMEs fitted on the distinct combinations of explanatory variables, we selected the ones leading to the lowest AICc score. We performed model refinement through sequential dropping of the retained explanatory variables. Then, the simulation-based model validation as well as the extraction of model statistics and related marginal effect plots were performed following the same steps described for the analysis of species richness.
To assess potential variations in groundwater recharge of the four selected springs under a climate change scenario, we: i) assessed the recharge areas of the springs and the corresponding distributed recharge; ii) graphically estimated the relationship between the distribution of precipitation and flow discharge, using the Presciano spring as a case study; (iii) modelled future variations in precipitation across the Gran Sasso-Sirente hydrogeologic basin (1408 km2) based on different Regional Climate Models (RCMs).
For the Cavuto spring, we used the recharge area identified in previous studies (Boni and Rusi, 2005). To define the recharge areas of the springs fed by the Gran Sasso aquifer (i.e., Capo Pescara-Santa Liberata, Chiarino and Presciano), we applied values of distributed recharge, as modelled in Lorenzi et al. (2022) for the average year (across the 2001–2020 period) using the Turc (1955) and Thornthwaite (Thornthwaite and Mather, 1957) methods, to the spring catchment defined by geo-structural setting (e.g., main fault role). The resulting distributed recharge estimates were then compared with the known mean discharge of the target springs. To validate this approach, we also compared the mean elevation of the derived recharge areas with the Computed Isotope Recharge Elevation (CIRE) calculated on the correlation line proposed by Barbieri et al. (2005) with the values of the δ18O measured at the corresponding springs during several surveys in the years 2020–2022. In fact, δ18O measured at spring outlets is a clear indicator of the mean recharge elevation of the recharge area (Tallini et al., 2014). As usual for basal regional springs (Barbieri et al., 2017), the recharge catchment of the Capo Pescara-Santa Liberata spring was more difficult to assess. Indeed, two different aquifers (Gran Sasso and Sirente) feed the spring. Moreover, its high discharge implies long and potentially deep flow paths, with longer renewable rate and limited fast contribution by infiltration to the discharge. In this case, we adopted a weight-based approach, separating the recharge catchment into five increasing elevation ranges.
Once we identified the recharge areas, we estimated the amount and distribution of precipitation across the Gran Sasso-Sirente hydrogeologic basin. The data of up to 122 rain gauges were used as input information (Nikolopoulos et al., 2010) to feed the Cellular Automata (CA) algorithm implemented in the CETEMPS Hydrological Model (CHyM) (Coppola et al., 2007; Verdecchia et al., 2009) software, which improves the assimilation and spatialization of data from sparse gauge stations. According to Packard and Wolfram (1985), CA is a simple mathematical idealization of natural systems which models the behavior that each single element of the target system may assume in subsequent spatial-temporal steps. Through the CHyM software, we modelled the rain field across the Gran Sasso-Sirente basin as an Areal Precipitation Estimate (APE) (270 m cell resolution, longitude gradient = 710 cells, latitude gradient = 470 cells, Supplementary Figure S2) with hourly temporal resolution. Then, we carried out a qualitative analysis by comparing the trend of maximum and minimum monthly flow discharge values extracted from the “Madonnina” hydrometric station of the Tirino River, located downstream of the Presciano spring (the sole spring for which such data were available from the Abruzzo Region Hydrological Annals over the 2005–2019 period), with the trend resulting from the CHyM-derived monthly cumulative rain, averaged across the considered area.
Finally, we investigated possible long-term variations in the precipitation trends over the Gran Sasso-Sirente hydrogeologic basin by simulating monthly cumulative rain over the 2081–2095 period through the CHyM software, considering three Regional Climate Models (i.e., CNRM, IPSL, and ICHEC) under the Representative Concentration Pathway (RCP) 8.5 (i.e., “business as usual” scenario, Rihai et al., 2017). We chose 2081 as the starting year of our future rainfall projections to match the starting year of long-term climate projections (i.e., 2081–2100) of the Coupled Model Intercomparison Project Phase 6 (CMIP6) featuring the IPCC Sixth Assessment Report (Lee et al., 2021). Moreover, the choice of the 2081–2095 period aimed at evaluating long-term precipitation trends over the Gran Sasso-Sirente basin within a timespan of comparable duration to that (i.e., 2005–2019) used for modelling assimilated rainfall, as proposed in Sangelantoni et al. (2019).
A total of 48 species, comprising 22 stygobites and 26 non-stygobites, were recorded within the 168 sites sampled across the four springs (Supplementary Table S1). Nine species were collected from at least one site in all springs, while twenty-five species were found in a single spring (Supplementary Table S2). Among these latter, it is worth mentioning some still undescribed species, such as a Nitocrella species sampled from a single pool in the Cavuto spring and two new species belonging to the canthocamptid genera Moraria and Paramorariopsis which were found in the Chiarino spring. Bryocamptus echinatus was the most widespread species in all the springs analyzed, with Bryocamptus zschokkei and Diacyclops paolae being among the top-five species in terms of occurrences in three out of the four springs (Supplementary Table S3).
Total species richness varied among the four springs, as well as within each spring over time (Figure 3). The Presciano spring showed the highest species richness (SR), with maximum values in July 2012 and October 2014 (22 and 24 copepod species, respectively). The CP_SL spring showed lower maximum SR (15 species) than the Cavuto (18 species) and Chiarino (20 species) springs. The Presciano spring harbored the highest number of stygobites (hereafter Sb) among the four springs, with 9 Sb species found in July 2012 and October 2014, followed by CP_SL (6 Sb species in March 2015), Cavuto (5 Sb species in October 2014) and Chiarino (6 Sb species in May 2015).
FIGURE 3. Changes in copepod species richness over time. Temporal variation of total species richness (SR) (upper panel), species richness of stygobites (middle panel) and non-stygobites (lower panel) for each analyzed spring.
Total beta diversity was higher than 0.6 for almost all the sampling dates, with species turnover generally higher than nestedness (Figure 4). An apparent increase in overall beta diversity over time, driven by rising turnover, emerged for the Chiarino spring and the Presciano spring (starting from March 2012 for the latter), while the values of the three diversity components more markedly fluctuated over time in the Cavuto and CP_SL springs. For Capo Pescara-Santa Liberata spring, statistically significant differences among the five compared sampling dates emerged for all the dissimilarity metrics (nestedness: χ2 = 99.44, p < 0.001; turnover: χ2 = 94.53, p < 0.001; total beta diversity: χ2 = 52.99, p < 0.001). Fluctuations in turnover, nestedness and total beta diversity values between the compared pairs of sampling dates were statistically significant for all the springs, with few exceptions (Supplementary Tables S4, S5).
FIGURE 4. Changes in taxonomic beta diversity over time. Temporal variation of turnover (orange), nestedness-derived diversity (green) and overall beta diversity (blue), computed through the Sörensen family of indices for each analyzed spring.
When accounting for among-sites phylogenetic differences, trends across springs and sampling dates were similar to those previously observed for taxonomic diversity (Figure 5). Indeed, phylogenetic turnover was higher than phylogenetic nestedness for most samples, thus suggesting that several sites host unique representatives of higher-order taxonomic groups, such as species belonging to subfamilies and/or genera not recorded in other sites from the same spring.
FIGURE 5. Changes in phylogenetic beta diversity over time. Temporal variation of phylogenetic turnover (orange), phylogenetic nestedness (green) and overall phylogenetic diversity (blue), computed through the Sörensen family of indices for each analyzed spring.
All the springs showed pristine conditions, as the average concentrations were below the LOD (Limit of Detection) for almost all the considered contaminants (Supplementary Table S6). Physical-chemical parameters varied considerably among the springs, with Chiarino and Cavuto showing lower temperature and electrical conductivity, and higher pH and dissolved oxygen concentration than CP_SL and Presciano (Supplementary Figure S3).
The electrical conductivity (VIF score = 5.49) determined most of the multicollinearity among the four physical-chemical variables. Once discarded, pairwise collinearity among the remaining variables was lower than 0.7 and VIF scores were lower than 3.
The Poisson GLMM relating total SR to the retained physical-chemical variables showed both overdispersion (DHARMa dispersion = 1.63, p-value = 0.046) and non-uniformity of residuals (one-sample two-sided Kolmogorov–Smirnov test: D = 0.15, p-value <0.001). The negative binomial GLMM fitted on the same fixed effect terms was not affected by these issues (DHARMa dispersion = 0.98208, p-value = 0.424; one-sample two-sided Kolmogorov–Smirnov test: D = 0.075, p-value = 0.328). The single physical-chemical parameters did not significantly contribute to the model, whereas the corresponding two-way interaction terms did (Table 2). Specifically, above average values for dissolved oxygen led to steeply increasing predicted SR when temperature (Figure 6A) and, more prominently, pH (Figure 6B) showed below average values (i.e., along negative semiaxis of the scaled gradient). Overall, the selected model did not explain much of the observed temporal variation in species richness; indeed, the marginal pseudo-R2 was as low as 0.06, although it noticeably increased when considering the spring-related random effect (i.e., conditional pseudo-R2 = 0.23).
TABLE 2. Results from the negative binomial Generalized Linear Mixed Model fitted to relate observed species richness to temperature, pH and dissolved oxygen, with spring set as random effect. Estimate = estimated coefficients; Std. err. = standard error of coefficients; C.I. 95th = 95th percentile confidence interval for the coefficient values; τSpring = spring-related variance (random effect); σ2 = residual variance; AICc = Akaike’s Information Criterion corrected for small samples; R2 = Nakagawa pseudo-R2.
FIGURE 6. Relationships between species richness and water physical-chemical parameters. (A) Observed species richness values (empty triangles) along the scaled temperature gradient (left plot), and marginal effect plot showing how predicted species richness resulting from the negative binomial Generalized Linear Mixed Model (fitted including spring as random effect) varied along the combined gradients of scaled temperature and scaled dissolved oxygen values (right plot). (B) Observed species richness values (empty triangles) along the scaled values of pH (left plot) and marginal effect plot showing how predicted species richness resulting from the same negative binomial GLMM varied along the combined gradients of scaled pH and scaled dissolved oxygen (right plot). In the marginal effect plots, solid lines represent estimated values and shaded colored areas the corresponding 95th percentile confidence intervals.
The Linear Mixed Effect (LME) model fitted with total beta diversity (BetaSOR) as response variable showed non-negligible explanatory power even without considering the spring-related random effect (marginal pseudo-R2 = 0.26). In particular, among-sites standard deviation in dissolved oxygen (hereafter O2 sd) was positively related to predicted beta diversity (Table 3; Supplementary Figure S4).
TABLE 3. Results from the Linear Mixed Effect model relating total beta diversity (BetaSOR) to maximum dissolved oxygen value (O2 max) across the sites sampled for each spring × sampling date combination, and to standard deviation in dissolved oxygen (O2 sd) among the same sites, with spring set as random effect. Estimate = estimated coefficients; Std. err. = standard error of coefficients; C.I. 95th = 95th percentile confidence interval for the coefficient values; τSpring = spring-related variance (random effect); σ2 = residual variance; AICc = Akaike’s Information Criterion corrected for small samples; R2 = Nakagawa pseudo- R2.
The interaction between O2 sd and maximum within-spring dissolved oxygen values (hereafter O2 max) emerged as a statistically significant term in the LMEs fitted with turnover (BetaSIM) and nestedness (BetaSNE) as response variables (Table 4). Predictions from these LMEs showed a good fit to observed values of nestedness and turnover, particularly at the extremes (either low or high) of the O2 max gradient, which is also confirmed by the relatively high marginal pseudo-R2 (Table 4). In particular, when maximum O2 values within the springs were below average (i.e., negative scaled O2 max), high inter-site O2 variability (i.e., positive scaled O2 sd) produced an increase in predicted turnover (Figure 7A) with a corresponding decrease in predicted nestedness (Figure 7B); the opposite occurred, with steeper variations, when O2 variability was low (i.e., when O2 sd was below average).
TABLE 4. Results from the Linear Mixed Effect models relating turnover (BetaSIM) and nestedness (BetaSNE) to maximum dissolved oxygen value (O2 max) across the sites sampled for each spring × sampling date combination, and to standard deviation in dissolved oxygen (O2 sd) among the same sites, with spring set as random effect. Estimate = estimated coefficients; Std. err. = standard error of coefficients; C.I. 95th = 95th percentile confidence interval for the coefficient values; τSpring = spring-related variance (random effect); σ2 = residual variance; AICc = Akaike’s Information Criterion corrected for small samples; R2 = Nakagawa pseudo- R2. The “−” symbol for conditional R2 of BetaSNE indicates that conditional R2 could not be computed for this model due to null variance associated to the spring-related random effect.
FIGURE 7. Relationships between taxonomic beta diversity components and dissolved oxygen. (A) Observed Sörensen-based turnover (BetaSIM) values (empty triangles) along the gradient of scaled maximum dissolved oxygen concentration (O2 max) among the sites sampled for each spring
None of the LMEs fitted on the taxonomic beta diversity components showed residual overdispersion or uniformity issues (p-value >0.05 in all the validation tests).
Outcomes of the LMEs fitted for the different components of phylogenetic beta diversity resembled those obtained for taxonomic diversity in terms of significant fixed effect terms and associated estimated coefficients (Supplementary Tables S7, S8). Particularly, the predicted positive effect of O2 sd on total phylogenetic diversity (Supplementary Figure S5) as well as the synergistic influence of O2 sd and O2 max on phylogenetic turnover and nestedness (Supplementary Figure S6) recalled the patterns emerging from the LMEs fitted on taxonomic beta diversity.
Capo Pescara-Santa Liberata was by far the spring with the widest estimated recharge area (Figure 8; Supplementary Table S9). For the Chiarino spring, the mean elevation of the estimated recharge area closely approached the CIRE (about 1700–1750 m a.s.l.). For the Presciano spring, the inclusion of Campo Imperatore as preferential recharge area resulted in relatively tight correspondence between mean recharge elevation and CIRE, respectively calculated in 1450 m a.s.l. and 1500 m a.s.l. As shown in Supplementary Table S10, the contribution of the areas located below 600 m a.s.l. to the recharge of the Gran Sasso aquifer can be considered negligible (about 1%–2% of the total), while areas between 600 m a.s.l. and 1000 m a.s.l. contributed about half of the higher areas (>1000 m a.s.l.). Thus, by applying a different infiltration rate with increasing elevation to model the recharge area of the CP_SL spring, we obtained a sufficient agreement among the infiltration in the assigned recharge area, spring discharge and CIRE (Supplementary Table S9).
FIGURE 8. Recharge areas of the studied springs. Recharge areas within the Gran Sasso aquifer (left map), feeding Chiarino, Capo Pescara-Santa Liberata, and Presciano springs, and within the Montagna Grande aquifer (right map) feeding the Cavuto spring. Coordinates are reported using the WGS84/UTM zone 33 N projected coordinate system.
The cumulative monthly precipitation over the Gran Sasso-Sirente hydrogeologic basin, modelled through the CHyM software, showed a slightly increasing trend over the 2005–2019 period, despite clear seasonal fluctuations (Figure 9). A similar overall positive trend emerged for maximum and minimum monthly discharge measured downstream of the Presciano spring (Figure 9).
FIGURE 9. Temporal trends in rainfall across the Gran Sasso-Sirente hydrogeologic basin and flow discharge downstream of the Presciano spring. Upper panel: cumulative monthly precipitation between January 2005 and December 2019, modelled through the CHyM software across the Gran Sasso-Sirente hydrogeologic basin. The trend summarizing the modelled values is represented through a linear model (regression line and related shaded area corresponding to 95th percentile C.I.) interpolating the timeseries. Lower panel: maximum (green) and minimum (orange) discharge downstream of the Tirino system of springs (including the Presciano spring), recorded between 2005 and 2019. The trend summarizing the recorded values is represented through a linear model (regression line and related shaded area corresponding to 95th percentile C.I.) interpolating the timeseries.
Two out of the three Regional Climate Models used to simulate precipitation over the Gran Sasso-Sirente basin in the 2081–2095 period predicted an overall reduction in rainfall (Supplementary material Fig. S7)
Additionally, by simulating extreme recharge rates from the Gran Sasso aquifer, considering the recharge of the driest year and that of the rainiest year in the 2001–2020 period (Supplementary Table S10), we found that the Chiarino spring could undergo a decrease in recharge equaling 11% during the driest year while an increase of 50% may occur during the rainiest period, compared to the average recharge value. Wider potential variations in recharge were estimated for the Presciano spring (reduction of up to 42% during the driest year, balanced by a possible increase of 46% during the rainiest period) and the CP_SL spring (40% recharge reduction during the driest year, up to 56% recharge increment during the rainiest year). However, such wide variations in the recharge rate of the Gran Sasso aquifer cannot directly translate into similar changes in discharge for the Presciano and CP_SL springs. This decoupling was related to the overlapping of their recharge areas (Figure 8), which also would involve other springs located in the upstream sectors of the Tirino and Pescara rivers, so that the surplus or the deficit in recharge could be differently distributed between the two target springs. Thus, negligible influence of seasonal and yearly oscillations could be expected for basal springs as CP_SL, while moderate decrease in discharge would be likely to affect the Presciano spring. Finally, the preliminary assessment performed for the Cavuto spring led to an estimated decrease in recharge of up to 55% for the driest year and an increase of up to 150% for the rainiest year, compared to the mean recharge rate.
Springs are known to be hotspots of biodiversity for plants and vertebrates, but little specific information is available about their unique aquatic invertebrate fauna (Cantonati et al., 2022). This trend could be further accentuated when focusing on a single meiofaunal target group, even when it is as widespread and greatly diversified as the Crustacea Copepoda. In this study, we found high diversity, both within and between springs, at the taxonomic and phylogenetic levels. Both species richness and beta diversity varied over time in the four target springs. Further, inter-site turnover exceeded the nestedness-derived diversity component for most of the springs and sampling dates.
The pristine water conditions of the studied springs likely positively contributed to the diversity of recorded copepod species. Indeed, Särkkä et al. (1997) showed that undisturbed Finnish springs host more copepod and cladoceran species than springs affected by direct anthropogenic pressures. The high inter-site beta diversity we found in these four karst springs extends to the within-spring scale previous evidence for high inter-spring taxonomic turnover in aquatic invertebrate metacommunities (e.g., Bottazzi et al., 2011; Cíbik et al., 2022). Our results also recall recent findings about high variability in invertebrate assemblages between sites located along a short (about 50 m) rheocrenic-hypocrenic gradient in a spring-springbrook system from Central Italy (Di Sabatino et al., 2021). Thus, the composition of invertebrate assemblages populating karst springs appears to widely vary along multiple spatial, temporal, and environmental axes.
From a conservation perspective, high within-spring taxonomic and phylogenetic turnover suggests the importance of preserving all the microhabitats of each spring because each of them harbors unique assemblages. Thus, the alteration of even one spring site may determine the local extinction of species or higher-order taxonomic groups. For instance, the genus Simplicaris, with the species Simplicaris lethaea, has been described from one site of the Presciano spring (Galassi and De Laurentiis, 2004). The species was found in a site with sediments represented by sand and gravel, and it has never been collected from other sites of the same spring with hygrophilous vegetation, or close to the main karst fractures. The genus is known with another species only, Simplicaris veneris (Cottarelli and Maiolini, 1980) from the interstitial habitat of Lake Vico (Central Italy) (Cottarelli and Maiolini, 1980). Based on present knowledge, the parastenocarid genus Simplicaris is at high risk of extinction. Further, the record of the genus Paramorariopsis from the Chiarino spring is the first one for the Apennine ridge in Central Italy, and the southernmost one: indeed, Paramorariopsis is known worldwide with three species from the karst groundwaters of Slovenia, and an undescribed species collected from the Lessinian unsaturated karst (Galassi et al., 2009b; Vaccarelli et al., 2023). This disjunct distribution also suggests an ancient origin of the genus. Another phylogenetic relic is Pseudectinosoma reductum, described from one site only of the Presciano spring (Galassi et al., 1999; Fiasca et al., 2014).
In this context, the identification of the environmental drivers behind variation in species richness and beta diversity in spring habitats is critical to monitoring and preserving their biodiversity. In the springs analyzed in the present study, the hydrochemistry did not drive a substantial portion of temporal variability in species richness, while within-spring between-sites variability in oxygen concentrations had a prominent effect on overall beta diversity and on the ratio between turnover and nestedness, as also observed in previous studies (Stevens et al., 2021). We hypothesize that the positive relationship between dissolved oxygen variability and copepod beta diversity comes from the fact that variation in O2 concentrations (Becher et al., 2022) is expression of diversified microhabitat conditions (e.g., macrophyte coverage, microbial activity on organic matter); diversified microhabitats, in turn, provide shelter to copepod species with different ecological requirements. Further studies investigating the variability in functional invertebrate diversity along physical-chemical and habitat gradients would help in clarifying this point.
Since springs’ hydrochemistry did not comprehensively explain temporal patterns of species richness and beta diversity, other factors may be involved. For instance, regional climate, topography, and land cover were shown to complement water hydrochemistry in shaping the composition of the aquatic vegetation communities in helocrene subarctic springs (Miller et al., 2021) and in Central Europe (Strohbach et al., 2009). As mentioned above, composite aquatic vegetation may sustain high microhabitat heterogeneity, in turn affecting invertebrate diversity. Other studies specifically targeting invertebrate communities of spring habitats suggested that substratum type (Dumnicka et al., 2007) and discharge timing of the feeding aquifers (Hoffsten and Malmqvist, 2000) have a strong influence on diversity patterns. We speculate that the marked difference in discharge during the hydrologic year characterizing the four karst springs of this study might have strong effects on the composition of their meiofauna assemblages, as also observed by Di Lorenzo et al. (2018) in the Mazzoccolo karst spring (Central Italy). Thus, aquifer discharge dynamics may be the primary factor behind the unexplained portion of the observed temporal variation in species richness and beta diversity. For instance, the higher number of stygobite species recorded in the most recent sampling campaigns in the Presciano spring may be related to the L'Aquila earthquake (Mw 6.3) which happened on 6 April 2009, and dramatically changed the groundwater flow of the Gran Sasso aquifer (Galassi et al., 2014). Indeed, the 2009 earthquake induced an aquifer strain, triggering a massive flushing of groundwater, containing the obligate groundwater dwellers living in the aquifer, towards the spring outflows. The obligate groundwater copepod species were flushed out and remained trapped in the interstices of the springbed sediments, which somewhat mirror the true groundwater habitats where they live and complete their whole life cycle. The long lifespan of several stygobite copepod species (Galassi et al., 2009a; Hose et al., 2022), and the ability of some of them to reproduce in “transitional habitats”, such as the hyporheic zone of streams and groundwater-fed springs, likely allowed the survival of their populations in the interstitial microhabitats of the Presciano spring and thus the retrieval of individuals in the subsequent sampling sessions. In contrast, the Capo Pescara-Santa Liberata spring, despite being fed at least in part by the same Gran Sasso aquifer, did not show a similar trend in the variation of obligate groundwater species richness after the L'Aquila earthquake, likely due to the large distance of this spring from the epicenter. The Cavuto spring is fed by an aquifer with fast infiltration flow, which does not favor the survival of the stygobite species in the less developed annex capacitive subsystems of the aquifer, where most of them live. Autumn corresponds to the high-discharge period for this spring, and the peak in the richness of stygobite species was observed in October 2014. Consequently, the stygobite species richness of the Cavuto spring, which was noticeably lower than that of Presciano, despite the two springs having comparable mean annual discharge, mirrored the hydrogeologic setting of the feeding aquifer. In the Chiarino spring, whose stygobite species richness is relatively low and comparable to that of the Cavuto spring, the peak of recorded stygobites observed in May 2015 may be due to a fast response in discharge related to snowmelt. In summary, we hypothesize that the “piston effect” (Aquilina et al., 2006), namely, a pressure transfer due to pushing freshly infiltrated waters and mobilizing resident groundwater together with its fauna, may be one of the main drivers of the observed ratio of stygobite versus non-stygobite species richness variation over time. Hence, potential discharge deficit in drying climate scenarios could profoundly affect springs’ assemblages (Cartwright et al., 2020). This effect may be particularly strong for sites close to the spring banks or distant from the main karst fractures, because these are the sites more vulnerable to a collapse in discharge and the first ones at risk of going dry.
The similarity of trends between precipitation over the Gran Sasso-Sirente hydrogeologic unit and flow discharge downstream of the Presciano spring would be particularly important in a climate change scenario. As future CHyM-based rainfall projections over this area depicted an overall decrease in precipitation by the end of the century, careful discharge monitoring at the spring outlets would be required to manage possible water deficits and related effects on the spring’s community. Moreover, modelling of recharge rates over the last 2 decades (under average and extremely dry or wet conditions) for the recharge areas feeding the four springs suggested possible decreases in the groundwater recharge of up to 40%–50%, depending on the considered springs. This trend further warns that severe changes in the timing and amount of groundwater discharge at the target springs may occur in the near future, which might produce more or less severe effects on the corresponding communities, depending on the aquifers’ resilience (Kløve et al., 2014).
Overall, the results of this study reinforce the significant role of springs as islands of biodiversity. Moreover, each spring may be thought as a sort of archipelago, every single site representing an island, where only the hydrological continuity ensured by the aquifer will guarantee that they can remain ecological, hydrologic and evolutionary refugia (Davis et al., 2013; Cartwright, 2019; Cartwright et al., 2020). Our work assumes that, from a conservation perspective, the four karst springs analyzed in this study, which showed high inter-site species turnover, should be targeted with management measures aiming at preserving most of their microhabitat diversity to avoid population declines, or even local extinctions (Tuomisto et al., 2003; Socolar et al., 2016). In springs where the inter-site nestedness is higher than turnover, or both have approximately the same weight, conservation efforts could be focused on sites hosting most of the springs’ alpha diversity. The paradigmatic role of springs as biodiversity hotspots calls for a huge effort to preserve them from human-induced environmental alterations.
The original contributions presented in the study are included in the article/Supplementary Material, further inquiries can be directed to the corresponding author.
FC and DG: Conceptualization, Methodology, Validation, Formal analysis, Writing—original draft; DG: Funding acquisition; BF: Data curation, Review, and editing, Investigation, Methodology; TD: Review and editing, Data curation; MP and VL: Hydrogeologic modelling, recharge rates assessment, hydrogeologic data validation, Investigation; AL and BT: Hydrogeologic modelling; ChyM software application; hydrometric data analysis, Validation. MD and IV: Writing—Review and editing, Validation. All authors contributed to the article and approved the submitted version.
This research was partially granted by the European Commission AQUALIFE LIFE12 BIO/IT/000231 “Development of an innovative and user-friendly indicator system for biodiversity in groundwater dependent ecosystems” and by the Biodiversa + project DarCo. FC is granted by the PNR 2021–2027 (Italian Ministry of University and Research, DM 737/2021). TD acknowledges the support of NBFC to CNR, funded by the Italian Ministry of University and Research, P.N.R.R., Missione 4 Componente 2, “Dalla ricerca all’impresa”, Investimento 1.4, Project CN00000033. MP acknowledges the support of the European Commission through the “Partnership for Research and Innovation in the Mediterranean Area (PRIMA)” programme under Horizon 2020 (KARMA project, grant agreement number 01DH19022A).
The Authors would like to thank Giancarlo Boscaino, Ing. Angelo Tarquini and Adelaide Memmo (Ufficio Idrografico e Mareografico, Pescara, Abruzzo, Italy) for having provided access to the long time series data on monthly flow discharge of the “Madonnina” hydrometric station, extracted from the Hydrological Annals of the Abruzzo Region (https://www.regione.abruzzo.it/content/annali-idrologici).
The authors declare that the research was conducted in the absence of any commercial or financial relationships that could be construed as a potential conflict of interest.
All claims expressed in this article are solely those of the authors and do not necessarily represent those of their affiliated organizations, or those of the publisher, the editors and the reviewers. Any product that may be evaluated in this article, or claim that may be made by its manufacturer, is not guaranteed or endorsed by the publisher.
The Supplementary Material for this article can be found online at: https://www.frontiersin.org/articles/10.3389/fenvs.2023.1051295/full#supplementary-material
Alley, W. M., Beutler, L., Campana, M. E., Megdal, S. B., and Tracy, J. C. (2016). Groundwater visibility: The missing link. Groundwater 54, 758–760. doi:10.1111/gwat.12466
Amoruso, A., Crescentini, L., Petitta, M., and Tallini, M. (2013). Parsimonious recharge/discharge modeling in carbonate fractured aquifers: The groundwater flow in the Gran Sasso aquifer (Central Italy). J. Hydrology 476, 136–146. doi:10.1016/j.jhydrol.2012.10.026
Aquilina, L., Ladouche, B., and Dörfliger, N. (2006). Water storage and transfer in the epikarst of karstic systems during high flow periods. J. Hydrology 327, 472–485. doi:10.1016/j.jhydrol.2005.11.054
Barbieri, M., Boschetti, T., Petitta, M., and Tallini, M. (2005). Stable isotope (2H, 18O and 87Sr/86Sr) and hydrochemistry monitoring for groundwater hydrodynamics analysis in a karst aquifer (Gran Sasso, Central Italy). Appl. Geochem. 20, 2063–2081. doi:10.1016/j.apgeochem.2005.07.008
Barbieri, M., Nigro, A., and Petitta, M. (2017). Groundwater mixing in the discharge area of san vittorino plain (central Italy): Geochemical characterization and implication for drinking uses. Environ. Earth Sci. 76, 1–14. doi:10.1007/s12665-017-6719-1
Baselga, A., Orme, D., Villeger, S., De Bortoli, J., Leprieur, F., and Logez, M. (2022). betapart: Partitioning beta diversity into turnover and nestedness components. R package version 1.5.6. Available at: https://CRAN.R-project.org/package=betapart (Accessed March 14th, 2023)
Baselga, A. (2010). Partitioning the turnover and nestedness components of beta diversity. Glob. Ecol. Biogeogr. 19, 134–143. doi:10.1111/j.1466-8238.2009.00490.x
Baselga, A. (2012). The relationship between species replacement, dissimilarity derived from nestedness, and nestedness. Glob. Ecol. Biogeogr. 21, 1223–1232. doi:10.1111/j.1466-8238.2011.00756.x
Bates, D., Maechler, M., Bolker, B., and Walker, S. (2015). Fitting linear mixed-effects models using lme4. J. Stat. Softw. 67, 1–48. doi:10.18637/jss.v067.i01
Becher, J., Englisch, C., Griebler, C., and Bayer, P. (2022). Groundwater fauna downtown – drivers, impacts and implications for subsurface ecosystems in urban areas. J. Contam. Hydrology 248, 104021. doi:10.1016/j.jconhyd.2022.104021
Benjamini, Y., and Hochberg, Y. (1995). Controlling the false discovery rate: A practical and powerful approach to multiple testing. J. R. Stat. Soc. Ser. B 57, 289–300. doi:10.1111/j.2517-6161.1995.tb02031.x
Bernot, J. P., Boxshall, G. A., and Crandall, K. A. (2021). A synthesis tree of the Copepoda: Integrating phylogenetic and taxonomic data reveals multiple origins of parasitism. PeerJ 9, e12034. doi:10.7717/peerj.12034
Boni, C., and Rusi, M. (2005). Carta idrogeologica della Marsica orientale (Monte marsicano-montagna Grande). Roma: CNR – Gruppo Nazionale Difesa Catastrofi Idrogeologiche (GNDCI-CNR.
Bottazzi, E., Bruno, M. C., Pieri, V., Di Sabatino, A., Silveri, L., Carolli, M., et al. (2011). Spatial and seasonal distribution of invertebrates in Northern Apennine rheocrene springs. J. Limnol. 70, 77–92. doi:10.3274/JL11-70-S1-06
Boulton, A. J. (2020). Conservation of groundwaters and their dependent ecosystems: Integrating molecular taxonomy, systematic reserve planning and cultural values. Aquatic Conservation Mar. Freshw. Ecosyst. 30, 1–7. doi:10.1002/aqc.3268
Brooks, M. E., Kristensen, K., van Benthem, K. J., Magnusson, A., Berg, C. W., Nielsen, A., et al. (2017). glmmTMB balances speed and flexibility among packages for zero-inflated generalized linear mixed modeling. R J., 9, 378–400. ISSN: 2073–4859.
Cantonati, M., Füreder, L., Gerecke, R., Jüttner, I., and Cox, E. J. (2012). Crenic habitats, hotspots for freshwater biodiversity conservation: Toward an understanding of their ecology. Freshw. Sci. 31, 463–480. doi:10.1899/11-111.1
Cantonati, M., Lichtenwöhrer, K., Leonhardt, G., Seifert, L., Mustoni, A., Hotzy, R., et al. (2022). Using springs as sentinels of climate change in nature parks north and south of the alps: A critical evaluation of methodological aspects and recommendations for long-term monitoring. Water 14, 2843. doi:10.3390/w14182843
Cantonati, M., Stevens, L. E., Segadelli, S., Springer, A. E., Goldscheider, N., Celico, F., et al. (2020). Ecohydrogeology: The interdisciplinary convergence needed to improve the study and stewardship of springs and other groundwater-dependent habitats, biota, and ecosystems. Ecol. Indic. 110, 105803. doi:10.1016/j.ecolind.2019.105803
Cartwright, J. (2019). Ecological islands: Conserving biodiversity hotspots in a changing climate. Front. Ecol. Environ. 17, 331–340. doi:10.1002/fee.2058
Cartwright, J., and Johnson, H. M. (2018). Springs as hydrologic refugia in a changing climate? A remote-sensing approach. Ecosphere 9, e02155. doi:10.1002/ecs2.2155
Cartwright, J. M., Dwire, K. A., Freed, Z., Hammer, S. J., McLaughlin, B., Misztal, L. W., et al. (2020). Oases of the future? Springs as potential hydrologic refugia in drying climates. Front. Ecol. Environ. 18, 245–253. doi:10.1002/fee.2191
Cerasoli, F., Besnard, A., Marchand, M.-A., D'Alessandro, P., Iannella, M., and Biondi, M. (2021). Determinants of habitat suitability models transferability across geographically disjunct populations: Insights from Vipera ursinii ursinii. Ecol. Evol. 11, 3991–4011. doi:10.1002/ece3.7294
Cíbik, J., Beracko, P., Bulánková, E., Čiamporová Zaťovičová, Z., Gregušová, K., Kodada, J., and Derka, T. (2022). Are springs hotspots of benthic invertebrate diversity? Biodiversity and conservation priority of rheocrene springs in the karst landscape. Aquatic Conservation Mar. Freshw. Ecosyst. 32, 843–858. doi:10.1002/aqc.3802
Coppola, E., Tomassetti, B., Mariotti, L., Verdecchia, M., and Visconti, G. (2007). Cellular automata algorithms for drainage network extraction and rainfall data assimilation. Hydrological Sci. J. 52, 579–592. doi:10.1623/hysj.52.3.579
Cottarelli, V., and Maiolini, B. (1980). Parastenocaris veneris n. sp., un nuovo arpacticoide del lago di Vico (Crustacea, Copepoda). Fragm. Entomol. 15, 243–252.
Davis, J., Pavlova, A., Thompson, R., and Sunnucks, P. (2013). Evolutionary refugia and ecological refuges: Key concepts for conserving Australian arid zone freshwater biodiversity under climate change. Glob. Change Biol. 19, 1970–1984. doi:10.1111/gcb.12203
Di Lorenzo, T., Cipriani, D., Fiasca, B., Rusi, S., and Galassi, D. M. P. (2018). Groundwater drift monitoring as a tool to assess the spatial distribution of groundwater species into karst aquifers. Hydrobiologia 813, 137–156. doi:10.1007/s10750-018-3515-1
Di Sabatino, A., Cicolani, B., and Gerecke, R. (2003). Biodiversity and distribution of water mites (Acari, Hydrachnidia) in spring habitats. Freshw. Biol. 48, 2163–2173. doi:10.1046/j.1365-2427.2003.01151.x
Di Sabatino, A., Coscieme, L., Miccoli, F. P., and Cristiano, G. (2021). Benthic invertebrate assemblages and leaf-litter breakdown along the eucrenal–hypocrenal ecotone of a rheocrene spring in Central Italy: Are there spatial and seasonal differences? Ecohydrology 14, e2289. doi:10.1002/eco.22
Dole-Olivier, M.-J., Maazouzi, C., Cellot, B., Fiers, F., Galassi, D. M. P., Claret, C., and Marmonier, P. (2014). Assessing invertebrate assemblages in the subsurface zone of stream sediments (0–15 cm deep) using a hyporheic sampler. Water Resour. Res. 50, 453–465. doi:10.1002/2012WR013207
Dumnicka, E., Galas, J., and Koperski, P. (2007). Benthic invertebrates in karst springs: Does substratum or location define communities? Int. Rev. Hydrobiology 92, 452–464. doi:10.1002/iroh.200610991
Eckhardt, K., and Ulbrich, U. (2003). Potential impacts of climate change on groundwater recharge and streamflow in a central European low mountain range. J. Hydrology 284, 244–252. doi:10.1016/j.jhydrol.2003.08.005
Eyun, S. I. (2017). Phylogenomic analysis of Copepoda (Arthropoda, Crustacea) reveals unexpected similarities with earlier proposed morphological phylogenies. BMC Evol. Biol. 17 (1), 1–12. doi:10.1186/s12862-017-0883-5
Famiglietti, J. (2014). The global groundwater crisis. Nat. Clim. Change 4, 945–948. doi:10.1038/nclimate2425
Fattorini, S., Borges, P. A. V., Fiasca, B., and Galassi, D. M. P. (2016). Trapped in the web of water: Groundwater-fed springs are island-like ecosystems for the meiofauna. Ecol. Evol. 6, 8389–8401. doi:10.1002/ece3.2535
Fattorini, S., Di Lorenzo, T., and Galassi, D. M. P. (2018). Earthquake impacts on microcrustacean communities inhabiting groundwater-fed springs alter species-abundance distribution patterns. Sci. Rep. 8, 1501. doi:10.1038/s41598-018-20011-1
Fattorini, S., Lombardo, P., Fiasca, B., Di Cioccio, A., Di Lorenzo, T., and Galassi, D. M. P. (2017). Earthquake-related changes in species spatial niche overlaps in spring communities. Sci. Rep. 7, 443. doi:10.1038/s41598-017-00592-z
Fiasca, B., Stoch, F., Olivier, M.-J., Maazouzi, C., Petitta, M., Di Cioccio, A., et al. (2014). The dark side of springs: What drives small-scale spatial patterns of subsurface meiofaunal assemblages? J. Limnol. 73, 55–64. doi:10.4081/jlimnol.2014.848
Galassi, D. M. P., and De Laurentiis, P. (2004). Towards a revision of the genus parastenocaris kessler, 1913: Establishment of Simplicaris gen. Nov. From groundwaters in central Italy and review of the P. brevipes-group (Copepoda, Harpacticoida, parastenocarididae). Zoological J. Linn. Soc. 140, 417–436. doi:10.1111/j.1096-3642.2003.00107.x
Galassi, D. M. P., De Laurentiis, P., and Dole-Olivier, M.-J. (1999). Phylogeny and biogeography of the genus Pseudectinosoma, and description of P. janineae sp. n. (Crustacea, Copepoda, Ectinosomatidae). Zool. Scr. 28, 289–303. doi:10.1046/j.1463-6409.1999.00018.x
Galassi, D. M. P., Huys, R., and Reid, J. W. (2009a). Diversity, ecology and evolution of groundwater copepods. Freshw. Biol. 54, 691–708. doi:10.1111/j.1365-2427.2009.02185.x
Galassi, D. M. P., Lombardo, P., Fiasca, B., Di Cioccio, A., Di Lorenzo, T., Petitta, M., et al. (2014). Earthquakes trigger the loss of groundwater biodiversity. Sci. Rep. 4, 6273. doi:10.1038/srep06273
Galassi, D. M. P., Stoch, F., Fiasca, B., Di Lorenzo, T., and Gattone, E. (2009b). Groundwater biodiversity patterns in the Lessinian Massif of northern Italy. Freshw. Biol. 54, 830–847. doi:10.1111/j.1365-2427.2009.02203.x
Hartig, F. (2022). DHARMa: Residual diagnostics for hierarchical (Multi-Level/mixed) regression models. R package version 0.4.5. Available at: https://CRAN.R-project.org/package=DHARMa (Accessed March 14th, 2023)
Hoffsten, P. O., and Malmqvist, B. (2000). The macroinvertebrate fauna and hydrogeology of springs in central Sweden. Hydrobiologia 436, 91–104. doi:10.1023/A:1026550207764
Hose, G. C., Chariton, A. A., Daam, M. A., Di Lorenzo, T., Galassi, D. M. P., Halse, S. A., and Korbel, K. L. (2022). Invertebrate traits, diversity and the vulnerability of groundwater ecosystems. Funct. Ecol. 36, 2200–2214. doi:10.1111/1365-2435.14125
Hsu, K.-C., Wang, C.-H., Chen, K.-C., Chen, C.-T., and Ma, K.-W. (2007). Climate-induced hydrological impacts on the groundwater system of the Pingtung Plain, Taiwan. Hydrogeology J. 15, 903–913. doi:10.1007/s10040-006-0137-x
Iannella, M., Fiasca, B., Di Lorenzo, T., Di CiccoBiondi, M. M., Mammola, S., and Galassi, D. M. P. (2021). Getting the ‘most out of the hotspot’ for practical conservation of groundwater biodiversity. Glob. Ecol. Conservation 31, e01844. doi:10.1016/j.gecco.2021.e01844
Jyväsjärvi, J., Marttila, H., Rossi, P. M., Ala-Aho, P., Olofsson, B. O., Nisell, J., et al. (2015). Climate-induced warming imposes a threat to north European spring ecosystems. Glob. Change Biol. 21 (12), 4561–4569. doi:10.1111/gcb.13067
Khorrami, M., and Malekmohammadi, B. (2021). Effects of excessive water extraction on groundwater ecosystem services: Vulnerability assessments using biophysical approaches. Sci. Total Environ. 799, 149304. doi:10.1016/j.scitotenv.2021.149304
Kløve, B., Ala-Aho, P., Bertrand, G., Gurdak, J. J., Kupfersberger, H., Kværner, J., and Rossi, P. (2014). Climate change impacts on groundwater and dependent ecosystems. J. Hydrology 518, 250–266. doi:10.1016/j.jhydrol.2013.06.037
Kreiling, A.-K., Govoni, D. P., Pálsson, S., Ólafsson, J. S., and Kristjánsson, B. K. (2022). Invertebrate communities in springs across a gradient in thermal regimes. PLoS ONE 17, e0264501. doi:10.1371/journal.pone.0264501
Lee, J.-Y., Marotzke, J., Bala, G., Cao, L., Corti, S., Dunne, J. P., et al. (2021). “Future global climate: Scenario-based projections and near-term information,” in Climate change 2021: The physical science basis. Contribution of working group I to the Sixth assessment Report of the intergovernmental panel on climate change V. Masson-Delmotte, P. Zhai, A. S. L. PiraniConnors, C. Péan, S. Berger, N. Caudet al. (Cambridge, United Kingdom and New York, NY, USA: Cambridge University Press). 553–672. doi:10.1017/9781009157896.006
Lorenzi, V., Sbarbati, C., Banzato, F., Lacchini, A., and Petitta, M. (2022). Recharge assessment of the Gran Sasso aquifer (Central Italy): Time-variable infiltration and influence of snow cover extension. J. Hydrology Regional Study 41, 101090.
Lüdecke, D. (2022). sjPlot: Data visualization for statistics in social science. R package version 2.8.11, Available at: https://CRAN.R-project.org/package=sjPlot (Accessed March 14th, 2023).
Malard, F., Dole-Olivier, M.-J., Mathieu, J., and Stoch, F. (2004). “Sampling manual for the assessment of regional groundwater biodiversity. PASCALIS project (V framework programme. Key action 2: Global change, climate, and biodiversity,” in 2.2. 3 assessing and conserving biodiversity) F. Malard Villeurbanne (France): Pascalis. Contract n°: N° EVK2-CT-2001-00121, 110. Available at: https://www.researchgate.net/publication/267567541_Sampling_Manual_for_the_Assessment_of_Regional_Groundwater_Biodiversity#fullTextFileContent (Accessed July 12th, 2022).
Meyer, A., and Meyer, E. I. (2000). Discharge regime and the effect of drying on macroinvertebrate communities in a temporary karst stream in East Westphalia (Germany). Aquat. Sci. 62, 216–231. doi:10.1007/PL00001333
Miller, T. K., Heegaard, E., Hassel, K., and Kapfer, J. (2021). Environmental variables driving species composition in subarctic springs in the face of climate change. J. Veg. Sci. 32, e12955. doi:10.1111/jvs.12955
Mori, A. S., Isbell, F., and Seidl, R. (2018). β-Diversity, community assembly, and ecosystem functioning. Trends Ecol. Evol. 33, 549–564. doi:10.1016/j.tree.2018.04.012
Mori, N., Kanduč, T., Opalički Slabe, M., and Brancelj, A. (2015). Groundwater drift as a tracer for identifying sources of spring discharge. Groundwater 53, 123–132. doi:10.1111/gwat.12314
Naimi, B., Hamm, N. A., Groen, T. A., Skidmore, A. K., and Toxopeus, A. G ( (2014). Where is positional uncertainty a problem for species distribution modelling? Ecography 37, 191–203. doi:10.1111/j.1600-0587.2013.00205.x
Nakagawa, S., Johnson, P. C. D., and Schielzeth, H. (2017). The coefficient of determination R2 and intra-class correlation coefficient from generalized linear mixed-effects models revisited and expanded. J. R. Soc. Interface 14, 20170213. doi:10.1098/rsif.2017.0213
Nikolopoulos, E. I., Anagnostou, E. N., Hossain, F., Gebremichael, M., and Borga, M. (2010). Understanding the scale relationships of uncertainty propagation of satellite rainfall through a distributed hydrologic model. J. Hydrometeorol. 11 (2), 520–532. doi:10.1175/2009JHM1169.1
Odum, H. T. (1957). Trophic structure and productivity of silver springs, Florida. Ecol. Monogr. 27, 55–112. doi:10.2307/1948571
Owor, M., Taylor, R. G., Tindimugaya, C., and Mwesigwa, D. (2009). Rainfall intensity and groundwater recharge: Empirical evidence from the upper nile basin. Environ. Res. Lett. 4, 035009. doi:10.1088/1748-9326/4/3/035009
Packard, N. H., and Wolfram, S. (1985). Two-dimensional cellular automata. J. Stat. Phys. 38, 901–946. doi:10.1007/BF01010423
Paradis, E., and Schliep, K. (2019). Ape 5.0: an environment for modern phylogenetics and evolutionary analyses in R. Bioinformatics 35, 526–528. doi:10.1093/bioinformatics/bty633
R Core Team (2022). R: A language and environment for statistical computing. Vienna, Austria: R Foundation for Statistical Computing. Available at: https://www.R-project.org/(Accessed March 14th, 2023).
Riahi, K., Van Vuuren, D. P., Kriegler, E., Edmonds, J., O’neill, B. C., Fujimori, S., et al. (2017). The shared socioeconomic pathways and their energy, land use, and greenhouse gas emissions implications: An overview. Glob. Environ. Change 42, 153–168. doi:10.1016/j.gloenvcha.2016.05.009
Sangelantoni, L., Tomassetti, B., Colaiuda, V., Lombardi, A., Verdecchia, M., Ferretti, R., et al. (2019). On the use of original and bias-corrected climate simulations in regional-scale hydrological scenarios in the mediterranean basin. Atmosphere 10, 1. doi:10.3390/atmos10120799
Särkkä, J., Levonen, L., and Mäkelä, J. (1997). Meiofauna of springs in Finland in relation to environmental factors. Hydrobiologia 347, 139–150. doi:10.1023/A:1003031621659
Schweiger, A., and Beierkuhnlein, C. (2014). Water temperature and acidity regime shape dominance and beta-diversity patterns in the plant communities of springs. Front. Biogeogr. 6, 132–143. doi:10.21425/F5FBG21845
Sivelle, V., Jourde, H., Bittner, D., Mazzilli, N., and Tramblay, Y. (2021). Assessment of the relative impacts of climate changes and anthropogenic forcing on spring discharge of a Mediterranean karst system. J. Hydrology 598, 126396. doi:10.1016/j.jhydrol.2021.126396
Socolar, J. B., Gilroy, J. J., Kunin, W. E., and Edwards, D. P. (2016). How should beta-diversity inform biodiversity conservation? Trends Ecol. Evol. 31, 67–80. doi:10.1016/j.tree.2015.11.005
Stevens, L. E., Schenk, E. R., and Springer, A. E. (2021). Springs ecosystem classification. Ecol. Appl. 31, e2218. doi:10.1002/eap.2218
Stoch, F., FiascaDi Lorenzo, B. T., Porfirio, S., Petitta, M., and Galassi, D. M. P. (2015). Exploring copepod distribution patterns at three nested spatial scales in a spring system: Habitat partitioning and potential for hydrological bioindication. J. Limnol. 75, 1–13. doi:10.4081/jlimnol.2015.1209
Strohbach, M., Audorff, V., and Beierkuhnlein, C. (2009). Drivers of plant species composition in siliceous spring ecosystems: Groundwater chemistry, catchment traits or spatial factors? J. Limnol. 68, 375–384. doi:10.4081/jlimnol.2009.375
Tallini, M., Adinolfi Falcone, R., Carucci, V., Falgiani, A., Parisse, B., and Petitta, M. (2014). Isotope hydrology and geochemical modeling: New insights into the recharge processes and water-rock interactions of a fissured carbonate aquifer (gran Sasso). Environ. Earth Sci. 72, 4957–4971. doi:10.1007/s12665-014-3364-9
Taylor, R., Todd, M. C., Kongola, L., Maurice, L., Nahozya, E., Sanga, H., et al. (2013). Evidence of the dependence of groundwater resources on extreme rainfall in East Africa. Nat. Clim. Change 3, 374–378. doi:10.1038/nclimate1731
Thornthwaite, C. W., and Mather, J. R. (1957). Instructions and tables for computing potential evapotranspiration and the water balance, 10. New Jersey (USA): Publications in climatology.
Tickner, D., Opperman, J. J., Abell, R., Acreman, M., Arthington, A. H., Bunn, S. E., and Young, L. (2020). Bending the curve of global freshwater biodiversity loss: An emergency recovery plan. BioScience 70, 330–342. doi:10.1093/biosci/biaa002
Tuomisto, H., Ruokolainen, K., and Yli-Halla, M. (2003). Dispersal, environment, and floristic variation of Western Amazonian forests. Science 299, 241–244. doi:10.1126/science.1078037
Turc, L. (1955). Le bilan d’eau des sols: Relations entre les precipitations, l’evaporation et l’ecoulement. Journées l'hydraulique 3, 36–44.
Vaccarelli, I., Cerasoli, F., Mammola, S., Fiasca, B., Di Cicco, M., Di Lorenzo, T., and Galassi, D. M. P. (2023). Environmental factors shaping copepod distributions in cave waters of the Lessinian unsaturated karst (NE-Italy). Front. Ecol. Evol. 11. doi:10.3389/fevo.2023.1143874
Verdecchia, M., Coppola, E., Tomassetti, B., and Visconti, G. (2009). “Cetemps Hydrological Model (CHyM), a distributed grid-based model assimilating different rainfall data sources,” in Hydrological modelling and the water cycle S. Sorooshian, K.-L. Kuo-Hsu, E. Coppola, B. Barbara Tomassetti, M. Verdecchia, and G. Visconti (Berlin, Heidelberg: Springer), 165–201.
Visser, H., Evers, N., Bontsema, A., Rost, J., de Niet, A., Vethman, P., et al. (2022). What drives the ecological quality of surface waters? A review of 11 predictive modeling tools. Water Res. 208, 117851. doi:10.1016/j.watres.2021.117851
Whittaker, R. H. (1960). Vegetation of the siskiyou Mountains, Oregon and California. Ecol. Monogr. 30, 280–338.
Youssef, N. H., Couger, M. B., and Elshahed, M. S. (2010). Fine-scale bacterial beta diversity within a complex ecosystem (zodletone spring, OK, USA): The role of the rare biosphere. PLoS ONE 5, e12414. doi:10.1371/journal.pone.0012414
Zuur, A. F., Ieno, E. N., and Elphick, C. S. (2010). A protocol for data exploration to avoid common statistical problems. Methods Ecol. Evol. 1, 3–14. doi:10.1111/j.2041-210X.2009.00001.x
Keywords: groundwater, karst springs, crustacea copepoda, climate change, conservation, beta diversity
Citation: Cerasoli F, Fiasca B, Di Lorenzo T, Lombardi A, Tomassetti B, Lorenzi V, Vaccarelli I, Di Cicco M, Petitta M and Galassi DMP (2023) Assessing spatial and temporal changes in diversity of copepod crustaceans: a key step for biodiversity conservation in groundwater-fed springs. Front. Environ. Sci. 11:1051295. doi: 10.3389/fenvs.2023.1051295
Received: 22 September 2022; Accepted: 19 May 2023;
Published: 30 May 2023.
Edited by:
Kirsten Work, Stetson University, United StatesReviewed by:
Raphael Ligeiro, Federal University of Pará, BrazilCopyright © 2023 Cerasoli, Fiasca, Di Lorenzo, Lombardi, Tomassetti, Lorenzi, Vaccarelli, Di Cicco, Petitta and Galassi. This is an open-access article distributed under the terms of the Creative Commons Attribution License (CC BY). The use, distribution or reproduction in other forums is permitted, provided the original author(s) and the copyright owner(s) are credited and that the original publication in this journal is cited, in accordance with accepted academic practice. No use, distribution or reproduction is permitted which does not comply with these terms.
*Correspondence: Diana M. P. Galassi, ZGlhbmFtYXJpYXBhb2xhLmdhbGFzc2lAdW5pdmFxLml0
Disclaimer: All claims expressed in this article are solely those of the authors and do not necessarily represent those of their affiliated organizations, or those of the publisher, the editors and the reviewers. Any product that may be evaluated in this article or claim that may be made by its manufacturer is not guaranteed or endorsed by the publisher.
Research integrity at Frontiers
Learn more about the work of our research integrity team to safeguard the quality of each article we publish.