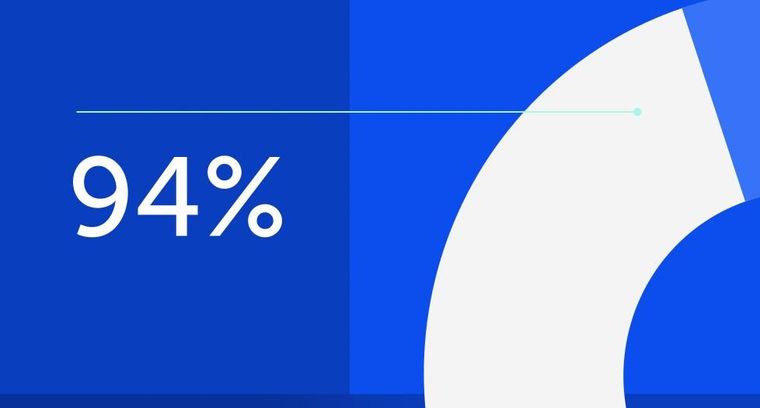
94% of researchers rate our articles as excellent or good
Learn more about the work of our research integrity team to safeguard the quality of each article we publish.
Find out more
ORIGINAL RESEARCH article
Front. Environ. Sci., 16 January 2023
Sec. Environmental Systems Engineering
Volume 11 - 2023 | https://doi.org/10.3389/fenvs.2023.1045464
Lake Ulansuhai, a typical shallow lake in an arid area that is economically and ecologically important along the Yellow River, is currently eutrophic. Long-term (2010–2020) data on chlorophyll-a, nutrient, and environmental factors were obtained from three Lake Ulansuhai monitoring stations. The temporal and spatial distribution characteristics of Chl-a were analyzed. Additionally, a hybrid evolutionary algorithm was established to simulate and predict Chl-a, and sensitivity analysis revealed the interaction between environmental factors and eutrophication. The results indicated that (1) the seasonal variation of eutrophication showed an obvious trend of spring > summer > autumn > winter, and the concentration of Chl-a in the inlet was significantly higher than that in the outlet; (2) The inlet, center, and outlet of Ulansuhai Lake are satisfactorily affected by HEA in the best suited method. The fitting coefficients (R2) of the optimal models were 0.58, 0.59, and 0.62 for the three monitoring stations, and the root mean square errors (RMSE) were 3.89, 3.21, and 3.56, respectively; (3) under certain range and threshold conditions, Chl-a increased with the increase of permanganate index, water temperature, dissolved oxygen concentration, and ammonia nitrogen concentration, but decreased with the increase of water depth, Secchi disk depth, pH, and fluoride concentration. The results indicate that the HEA can simulate and predict the dynamics of Chl-a, and identify and quantify the relationships between eutrophication and the threshold data. The research results provide theoretical basis and technical support for the prediction and have great significance for the improvement of water quality and environmental protection in arid and semi-arid inland lakes.
Eutrophication is one of the most challenging environmental problems in freshwater ecosystems around the world (Huisman et al., 2018; Li and Sang, 2021). In 2018, lakes and reservoirs in China were under severe threat from harmful algal blooms, which reached 775.4 km2 (Huang et al., 2020). Phytoplankton is an important primary producer in lake ecosystems, and changes in its composition and biomass will directly affect the structure, function, and stability of aquatic ecosystems. Chlorophyll-a (Chl-a) as an indicator of phytoplankton abundance and biomass amongst primary food producers in aquatic ecosystems can indicate the degree of lake eutrophication (Padisák et al., 2006). Therefore, measuring and predicting the levels of Chl-a and understanding the impact of environmental drivers on Chl-a are vital part of monitoring lake water quality and lake management programs. Modeling of Chl-a level in lakes is considered as one of the most important analytical tool for biological and ecological investigations (Di Toro et al., 1971; Tufffford and McKeller, 1999).
As the degree of lake eutrophication and the relationships between environmental factors are often complicated, researchers have used numerous non-linear methods (Recknagel et al., 1997). The complex non-linear dynamics of phytoplankton which is closely related to lake eutrophication and environmental factors can be accurately simulated using neural interpretation diagrams and the connection weight method in the artificial neural network model (Recknagel, 2001; Shi et al., 2020). The non-linear response relationship between Chl-a and environmental factors can be evaluated using generalized additive models (GAMs) (Quan et al., 2020). It is also of practical significance to establish a random forest model to evaluate the predictability of Chl-a and compare the relative importance of driving factors at different time scales (Shi et al., 2021). However, due to the long time series of water quality in Ulansuhai and the complex environmental changes in the watershed, we chose to use evolutionary simulation and genetic selection to determine the relationship between Chl-a concentration and other corresponding factors. Since pioneering work on evolutionary algorithms applications for ecological modelling by Whigham and Recknagel (2001), Cao et al. (2006) have developed the hybrid evolutionary algorithm (HEA) that is now applied for data-driven modelling of phytoplankton in lakes and rivers worldwide (Chan et al., 2007; Kim et al., 2007). The HEA model can use genetic evolutionary calculation methods to inductively reason and predict multivariate non-linear data. And the temporal changes of the data are used to reveal the ecological relationship, and the threshold of the data is calculated. Finally, both explanatory and predictive validity of the resulting are tested by comparisons between predicted and measured data as well as sensitivity analyses. The HEA model has a high level of fitting and prediction and has been successfully applied in the data-driven modeling of cyanobacteria blooms in lakes and rivers around the world (Recknagel et al., 2006b; Guallar et al., 2016; Kim. et al., 2019; Recknagel et al., 2017).
Lake Ulansuhai is the biggest lake along the Yellow River, which plays a unique ecological and environmental role in maintaining the ecosystem services of the whole watershed (Chan et al., 2007). However, this lake lies on the Hetao Irrigation District and is part of this largest irrigation district along the Yellow River. In recent years, the massive non-point agricultural waste water empties into the lake, resulting in increasingly serious eutrophication in Lake Ulansuhai (Koebbing et al., 2014), which then flows into the Yellow River and damages the aquatic ecosystem of the Yellow River.
To explore the driving effects of water quality physicochemical parameters on phytoplankton biomass in shallow lakes of arid area, water quality samples from Ulansuhai, a shallow lake in arid areas of Inner Mongolia, China, were collected and analyzed. We assumed that phytoplankton biomass was primarily affected by single or multiple water quality physicochemical parameters. Moreover, there is a complex non-linear relationship between them. This study aimed to: (1) study the temporal and spatial distribution characteristics of Chl-a concentration in Ulansuhai; (2) explore the ecological threshold and quantitative relationship model of water quality physicochemical parameters on Chl-a concentration in Ulansuhai; and (3) assess the promoting and inhibiting effects of water quality physicochemical parameters on Chl-a concentration through the sensitivity analysis of HEA. This study can predict the occurrence of algal blooms and provide data support for eutrophication management in Lake Ulansuhai. In the same time, it is of great significance to reveal the main controlling factors and seasonal variation of eutrophication in Lake Ulansuhai for water quality improvement, eutrophication management, and environmental protection in the Yellow River Basin.
Lake Ulansuhai (40°36′–41°03′N, 108°43′–108°57′E) is situated in the northwest part of Inner Mongolia (Figure 1), with a surface area of 293 km2. It is one of the eight largest freshwater lakes in China (Sun et al., 2006), as well as the largest freshwater lake region of its latitude. In recent years, with the development of industry and agriculture, the water quality of Lake Ulansuhai has deteriorated (Han et al., 2020). It has been in the eutrophication state, which has evolved into a shallow lake characterized by algal overgrowth. The watershed boundaries was extracted from ASTER GDEM elevation data from remote sensing image in the study area by ArcGIS10.2.
The data in this study were obtained from environmental monitoring stations, including the time series from 2010 to 2020 of three monitoring stations at the inlet, center and outlet of the lake. These data contained average monthly water quality monitoring data. The spatial distribution of each monitoring point had a good correspondence with the water flow pattern, water depth, water planting cover, and the spatial positions of the inlet and outlet points of the water flow in the lake. Table 1 summarises limnological variables of the 11-year period that has been utilized for modeling at the three monitoring sites. Because the interpolation method does not affect the model results (Cao et al., 2014), for the sake of simplicity, a linear interpolation method was used for the limnological properties data and the daily input data, and obtain the daily interpolation data. To allow for 7-day-ahead forecasting, the interpolated daily input data were shifted by 7 days against the daily output data of three monitoring sites.
HEA can discover rule sets from ecological time series data through genetic programming and optimize the parameters of a rule set by the genetic algorithm. The parameters of the model were optimized by the hill climbing (HC) method. HC originally is a local search technique which starts with a random solution, and iteratively makes small changes to the solution and each time improving it a little. When the algorithm cannot see any improvement anymore, it will terminates. Finally, the “IF-THEN-ELSE” rule sets were constructed according to the model with the highest fitting degree, where “IF” is the ecological threshold of the model. These rule sets provided high transparency in the ecological driving forces and relationships driving Chl-a concentration. In addition, HEA can automatically perform the sensitivity analysis for the input variables of each discovered model. It computes the output trajectories for each input range (mean ± SD) separately by keeping the average value of the input variables constant. Moreover, the resulting sensitivity curve outputs the visual trace as a percentage (0%–100%) within their range of each input.
Numerous studies have demonstrated that HEA can discover rule sets that provide good predictions of unseen data and represent causal relationships between physical and chemical variables and lake eutrophication (Cao et al., 2006; Cao et al., 2014; Kim. et al., 2019). Figure 2 shows a conceptual diagram of HEA based on water-quality data as an input and Chl-a concentration as an output.
FFIGURE 2. Conceptual diagram of the hybrid evolutionary algorithm (HEA) for evolving the IF-THEN-ELSE rule models.
We define the root mean square error (RMSE) as the fitness function:
where m is the number of data points, and
The whole data were divided into training data and test data. To improve the validity and stability of the model, the bootstrap method was used to select the training and testing data sets randomly based on a predefined division percentage (75% in this study). That is, during each run, the computer randomly selected 75% of the total data as training data and the remaining data as test data. Using this approach, a wider variety of models with greater robustness be obtained and the optimal model can be evaluated based on the validation results on the whole data set.
From 2010 to 2020, the daily interpolation values of water temperature (WT), water depth (WD), pH, Secchi disk depth, permanganate index (CODMn), fluoride concentration (F), ammonia nitrogen concentration (NH4_N), dissolved oxygen concentration (DO), and total phosphorus (TP) as the input variables as well as Chl-a concentration as the output variable, the optimal fitting prediction model of Chl-a in Lake Ulansuhai was established, and the content of Chl-a in Lake Ulansuhai was simulated and predicted. Sixty models were evolved by HEA to explore the Chl-a concentration at each monitoring point based on repeated bootstrap runs. Finally, the prediction model with the best fitting degree was determined by the “IF-THEN-ELSE” single rule set of HEA through multiple linear methods based on crossover and recombination. HC parameter settings of HEA for structure optimization were popsize = 100, maximum tree depth = 4, and maximum number of generations = 80 by using the programming language C++. The selection of these hyperparameters was based on repeated training and reference to previous studies (Cao et al., 2013; Cao et al., 2014).
From 2010 to 2020, according to the evaluation standard of lake eutrophication in China (Shu, 1990), the entire lake was in a moderate eutrophication state, and the concentration of Chl-a exceeded the standard. The spatiotemporal distribution and the significant differences in Chl-a concentration from 2010 to 2020 in Lake Ulansuhai were retrieved, as showed in Figure 3.
FIGURE 3. (A) Interannual variation of chlorophyll-a (Chl-a) concentration from 2010 to 2020. (B) Significant differences in the temporal and spatial distribution of Chl-a in Lake Ulansuhai. Values marked with different lowercase letters differ significantly at p < 0.05, after using Duncan’s tests for post hoc comparisons. The non-significant differences are not marked by any letters.
The Chl-a concentration in Lake Ulansuhai peaked in 2010 and 2017. During these 2 years, the water quality of the lake was in a state of eutrophication. The concentration of Chl-a in the other years was kept at about 10 mg/m3, which was considered a state of moderate eutrophication. From the perspective of the season, Chl-a concentration showed an obvious trend of spring > summer > autumn > winter, but there was no significant difference.
In terms of spatial distribution characteristics, the Chl-a of the lake inlet was higher than that in the center of the lake and that of the lake outlet, and the difference was significant, except for during winter (ANOVA, p < 0.05).
At the lake inlet, the input variables of the best fitting and prediction model included CODMn, TP, WD, F, and Secchi disk depth. The fitting degree of the lake inlet model was high, and its R2 = 0.58, RMSE = 3.89. The concentration of Chl-a fluctuated, mostly around 20 mg/m3. From 2010 to 2020, it showed a decreasing trend year by year, and the decreasing speed gradually accelerated. As can be seen from the residual plot, the model fluctuated greatly from 2016 to 2018 (Figure 4).
FIGURE 4. Diagram of chlorophyll-a (Chl-a) concentration prediction model and residual at the lake inlet.
According to fitting formulas below, the ecological threshold of Chl-a concentration change in the lake inlet area was CODMn<8.02 mg/L (formula (1)). When CODMn was less than 8.02 mg/L, the concentration of Chl-a was related to CODMn, TP, and WD. The concentration of Chl-a was directly proportional to WD and CODMn and inversely proportional to TP (formula (2)). When CODMn was greater than 8.02 mg/L, the concentration of Chl-a was related to WD, Secchi disk depth, F, and TP (formula (3)). Chl-a concentration was inversely proportional to the four variables:
Then
Else
In the lake center, the input variables for the fitting model of Chl-a concentration were Secchi disk depth, NH4_N, CODMn, F, and WT. The model fitting coefficient was R2 = 0.59, RMSE = 3.21. During 2010–2020, the concentration of Chl-a remained basically stable, and the overall concentration remained at about 7 mg/m3. The model residuals from 2010 to 2020 were small, indicating a high degree of fitting (Figure 5).
FIGURE 5. Diagram of chlorophyll-a (Chl-a) concentration prediction model and residual in the lake center.
According to fitting formulas below, the ecological threshold of Chl-a concentration change in the lake center was determined by the concentration of Secchi disk depth. When Secchi ≥64.71 cm (formula (4)), Chl-a concentration was calculated by the THEN branch (formula (5)), which was proportional to NH4_N and CODMn. Conversely, Chl-a concentration was calculated by the ELSE branch (formula (6)), which was proportional to WT and inversely proportional to F:
Then
Else
In the outlet of the lake, the input variables of the prediction model for Chl-a concentration were CODMn, pH, DO, Secchi, WT, and F, and the model fitting coefficient R2 = 0.62, RMSE = 3.56. From 2010 to 2020, the concentration of Chl-a in 2017 was significantly higher than that in other years, with the maximum value reaching 76 mg/m3. In other years, the concentration of Chl-a remained at about 7 mg/m3 and showed an increasing trend. It can be seen from the residual figure that there is a large residual between the individual fitted values and the measured values from 2016 to 2018 (Figure 6).
FIGURE 6. Diagram of chlorophyll-a (Chl-a) concentration prediction model and residual in the lake outlet.
According to fitting formulas below, the ecological threshold of Chl-a concentration in the outlet of Lake Ulansuhai was determined by F concentration. When F > 0.68 mg/L (formula (7)), Chl-a concentration was calculated by THEN branch (formula (8)), which was inversely proportional to F and directly proportional to CODMn. Instead (formula (9)), it was proportional to the DO and Secchi and inversely proportional to the pH and WT in the water:
Then
Else
Sensitivity analysis of the HEA model in Lake Ulansuhai inlet showed that CODMn, TP, WD, F, and Secchi disk depth all affected Chl-a concentration, while IF (CODMn<8.02 mg/L) was determined by the THEN branch ecological relationship. Within a certain range, with the increase of the input variable CODMn, the concentration of Chl-a also increased, and with the increase of input variable TP, the concentration of Chl-a gradually decreased, but the changes in Chl-a concentration and WD were not obvious. Conversely, determined by the ELSE branch, Chl-a concentration was inversely proportional to WD, Secchi, and F, and was not significantly correlated with TP (Figure 7).
FIGURE 7. Sensitivity analysis of the THEN branch and ELSE branch in the lake inlet. The THEN branch on the left, and the ELSE branch on the right.
Sensitivity analysis of the HEA model on the center of Lake Ulansuhai showed that CODMn, NH4_N, WT, and F all affected the concentration of Chl-a, while IF (Secchi ≥64.71 cm) was determined by the ecological relationship of the THEN branch. Within a certain range, with the increase of CODMn and NH4_N input, the concentration of Chl-a also increased. On the contrary, the concentration of Chl-a was proportional to WT as measured by the ELSE branch, and did not change significantly with the concentration of F (Figure 8).
FIGURE 8. Sensitivity analysis of THEN branch and ELSE branch in the lake center. The THEN branch on the left; the ELSE branch on the right.
The sensitivity analysis of the HEA model on the outlet of Lake Ulansuhai showed that CODMn, pH, WT, Secchi, and DO all affected the concentration of Chl-a, and when the ecological threshold F > 0.68 mg/L, the concentration of Chl-a was determined by the THEN branch ecological relationship. In a certain range, with the increase of CODMn input, the concentration of Chl-a also increased. But with the increase of pH and F, the Chl-a concentration gradually decreased. In contrast, determined by the ELSE branch, the Chl-a concentration was directly proportional to Secchi and DO and inversely proportional to the pH, but the change of WT had no significant effect on the Chl-a concentration (Figure 9).
FIGURE 9. Sensitivity analysis of the THEN branch and ELSE branch in the lake outlet. The THEN branch is on the left, and the ELSE branch is on the right.
The seasonal variation trend of Chl-a concentration in Lake Ulansuhai showed the highest in spring, followed by summer, and lower in autumn and winter. The causes of this trend were mainly related to the inflow load, precipitation, and the growth cycle of aquatic plants and algae. Water temperature rose in spring, and the decomposition of plant residues in the lake in addition to the frequent material exchange between the sediment and water column led to serious secondary pollution. Moreover, there were more sandstorms in spring, which accelerated water exchange (Guo et al., 2015). In summer, vegetation growth in the lake was stable and the biomass of phytoplankton increased rapidly, which maintained the concentration of Chl-a at a high level. In early autumn, many submerged plants grew in the surrounding shallow water and lakeshore buffer zones. These plants could purify the water quality and reduce the eutrophication degree gradually. This research result was consistent with the fitting result of the multiple linear regression model of the eutrophication state of Lake Ulansuhai established in previous research (Du et al., 2019), and was consistent with the kriging spatial interpolation of eutrophication index conducted in an earlier study (Li et al., 2011) on the spatiotemporal variation rule of the eutrophication of Lake Ulansuhai.
From the perspective of spatial distribution, the concentration of Chl-a in Lake Ulansuhai showed significant differences and decreased gradually from north to south. The concentration in the lake inlet was significantly higher than that in the lake outlet, reflecting the self-purification ability of the lake. This research result was consistent with the analysis conducted by Mao and Wei (2015) on the spatial variation law of 20 water quality monitoring points in Lake Ulansuhai. A great number of nutrients were absorbed, deposited, and degraded by aquatic plants during the flow from north to south, thus affecting the reproduction and growth of algae, which resulted in significant differences in the spatial distribution of Chl-a in Lake Ulansuhai (Luo and Li, 2011).
The seasonal variations of Chl-a were also potentially influenced by the physicochemical parameters of water, such as WT, DO and pH (Recknagel et al., 2006a; Jiang et al., 2018). Below certain nutrient levels, the increase in WT promotes the metabolic rate and cell division of phytoplankton, and increases productivity. Furthermore, the concentration of Chl-a was increased and the eutrophication of the lake was intensified (Kim et al., 2019). ELSE branch of sensitivity analysis of the HEA model in the center of Ulansuhai showed that Chl-a concentration increased continuously with the increase of WT. This result is consistent with Cha’s study on Chl-a and WT in four major rivers in South Korea (Cha et al., 2017). In addition, ELSE branch of HEA model in the outlet of Ulansuhai showed that DO is significantly positively correlated with Chl-a, which may have been due to phytoplankton absorbing CO2 and release O2, leading to an increase in DO (Cui et al., 2021). At the same time, high concentration of Chl-a will enhance the photosynthetic capacity of phytoplankton. Thus increasing the level of DO in the water. This result is consistent with the results of Jiang’s stepwise regression of Chl-a in Lake Ulansuhai (Jiang et al., 2019).
Chl-a showed an inhibitory relationships with pH. The pH value mainly affects the release of carbon, nitrogen, and phosphorus and the photosynthesis of aquatic plants by influencing the form of the carbon source and acid-base reactions (He et al., 2008), thus profoundly affecting the eutrophication process. Meanwhile, an increase in WT also leads to an increase in pH, which limits the growth of algae. The results of this study are the same as those of previous studies (Guo et al., 2015; Mao and Wei, 2015). The alkaline environment is conducive to algae reproduction and growth (Li et al., 2011). The HEA model in this study showed that Chl-a has a significant negative correlation with Secchi disk, because the low transparency of the water will reduce the photosynthesis of phytoplankton and slow down the growth rate. On the contrary, the transparency of the water will also be reduced due to the algal blooms (Deng, 2020). Many studies have shown that there was a strong correlation between Chl-a concentration and TP concentration in Lake Ulansuhai (Huang et al., 2020; Xu et al., 2020). According to the HEA at the entrance of Ulansuhai, TP showed an obvious decreasing trend with the increase of Chl-a in the two branches of THEN and ELSE, which was different from previous research results (Cao et al., 2013). This is due to the large-scale growth of reeds in Lake Ulansuhai inlet, shallow water depth and slow flow of lake water, which enable reeds to absorb nutrients. In addition, the absorption of submerged plants and the self purification of lake water lead to the gradual reduction of TP concentration. However, some research results show that TP has no significant effect on Chl-a (Peng et al., 2021). It can be seen that the relationship between TP and Chl-a concentration may be affected by lake morphology, land use types, climate and other factors. The fluorine element is closely related to human health. However, there have been few studies on fluorine in lakes in recent years. The relationship between variations in fluoride concentration and lake eutrophication needs to be further discussed while considering the influences of land use, landform, climate, and human activities.
HEA method was applied to establish the model of 7-day-ahead forecasting results of Chl-a and assess the relationship between the Chl-a and environmental factors of Lake Ulansuhai in this study. The results showed that the fitting coefficients (R2) of the optimal models are 0.56, 0.51 and 0.52 for the lake inlet, center and the lake outlet, respectively. Lake eutrophication is influenced by multiple environmental factors, and it is a long-term and complex change process. Under certain range and threshold conditions, Chl-a concentration increased with the increase of COD, CODMn, TP, and NH4_N, but decreased with the increase of water depth, Secchi disk, pH and F. Meanwhile, the concentration of Chl-a showed a strong spatial distribution difference, and it in the lake inlet it was significantly higher than that in the lake outlet, reflecting the self-purification ability of the lake, which indicated that Lake Ulansuhai was a grass and algae type lake with obvious spatial heterogeneity. The temporal distribution of lake eutrophication showed a trend of spring > summer > autumn > winter, which proved that climate factors also had a great influence on lake eutrophication. In the future, the model integration of single rule and multiple rule combinations can be considered, and the time series length of data and the number of monitoring points can be increased to further improve the prediction accuracy of rule sets. At the same time, the zooplankter data can be increased to reveal the internal mechanism of eutrophication in Lake Ulansuhai in a more systematic and comprehensive way.
The raw data supporting the conclusions of this article will be made available by the authors, without undue reservation.
LW, BP, XL and YL conceived the ideas and designed this study; XL and HL interpreted data and analysis, XL, HC and HL wrote the manuscript; ZX, XC, LM, LW reviewed the manuscript.
This work is funded by the National Natural Science Funds, P.R. China (No. 32161143025, 32160279, 31960249), Science and Technology Major Project of Inner Mongolia (No. 2022YFHH0017, 2021ZD0011), the Funds of Yinshanbeilu Grassland Eco-hydrology National Observation and Research Station (No. YSS2021007) and Ordos Science and Technology Plan (No. 2022EEDSKJZDZX010).
The authors declare that the research was conducted in the absence of any commercial or financial relationships that could be construed as a potential conflict of interest.
All claims expressed in this article are solely those of the authors and do not necessarily represent those of their affiliated organizations, or those of the publisher, the editors and the reviewers. Any product that may be evaluated in this article, or claim that may be made by its manufacturer, is not guaranteed or endorsed by the publisher.
Cao, H., Recknagel, F., Kim, B., and Takamura, N. (2006). “Hybrid evolutionary algorithm for rule set discovery in time-series data to forecast and explain algal population dynamics in two lakes different in morphometry and eutrophication,” in Ecological informatics. Editor F. Recknagel 2nd Edition (Verlag Berlin: Springer), 347–367. Chapter 17.
Cao, H., Recknagel, F., and Orr, P. T. (2013). Enhanced functionality of the redesigned hybrid evolutionary algorithm HEA demonstrated by predictive modelling of algal growth in the Wivenhoe Reservoir, Queensland (Australia). Ecol. Model. 252, 32–43. doi:10.1016/j.ecolmodel.2012.09.009
Cao, H., Recknagel, F., and Orr, P. T. (2014). Parameter optimization algorithms for evolving rule models applied to freshwater ecosystems. IEEE Trans. Evol. Comput. 18, 793–806. doi:10.1109/tevc.2013.2286404
Cha, Y. K., Cho, K. H., Lee, H., Kang, T., and Kim, J. H. (2017). The relative importance of water temperature and residence time in predicting cyanobacteria abundance in regulated rivers. Water Res. 124, 11–19. doi:10.1016/j.watres.2017.07.040
Chan, W. S., Recknagel, F., Cao, H., and Park, H. D. (2007). Elucidation and short-term forecasting of microcystin concentrations in Lake Suwa (Japan) by means of artificial neural networks and evolutionary algorithms. Water Res. 41, 2247–2255. doi:10.1016/j.watres.2007.02.001
Cui, G. Y., Wang, B. L., Xiao, J., Qiu, X. L., Liu, C. Q., and Li, X. D. (2021). Water column stability driving the succession of phytoplankton functional groups in karst hydroelectric reservoirs. J. Hydrol. (Amst) 592, 125607. doi:10.1016/j.jhydrol.2020.125607
Deng, X. J. (2020). Influence of water body area on water quality in the southern Jiangsu Plain, eastern China. J. Clean. Prod. 254, 120136. doi:10.1016/j.jclepro.2020.120136
Di Toro, D. M., O'Connor, D. J., and Thomann, R. V. (1971). “A dynamic model of the phytoplankton population in the Sacramento-San Joaquin Delta,” in Non equilibrium Systems in natural water chemistry. Advances in chemistry series (New York: American Chemical Society), 131–150.
Du, D., Li, C., Shi, X., Zhao, S., Quan, D., and Yang, Z. (2019). Seasonal changes of nutritional status of lake Wuliangsuhai. J. Arid Land Resour. Environ. 33, 186–192.
Guallar, C., Delgado, M., Diogène, J., and Fernández-Tejedor, M. (2016). Artificial neural network approach to population dynamics of harmful algal blooms in Alfacs Bay (NW Mediterranean): Case studies of Karlodinium and Pseudo-nitzschia. Ecol. Model. 338, 37–50. doi:10.1016/j.ecolmodel.2016.07.009
Guo, J., Wei, W., Yi-Lei, Y. U., Song, X. J., Zhang, M. Y., and Sheng-Nan, L. I. (2015). Research progress on the eutrophication of Wuliangsuhai wetland. Chin. J. Ecol. 34, 3244–3252.
Han, X., Yu, H., Song, Y., Chen, Y., and Qi, Q. (2020). Characterizing humic substances from a large-scale lake with irrigation return flows using 3DEEM-PARAFAC with CART and 2D-COS. J. Soils Sediments 20, 3514–3523. doi:10.1007/s11368-020-02688-y
He, J., Xiaohong, G. U., Liu, G., and Guo-feng, L. (2008). Aquatic macrophytes in East Lake Taihu and its interaction with water environment. J. Lake ences 20, 790–795. doi:10.18307/2008.0618
Huang, J., Zhang, Y., Arhonditsis, G. B., Gao, J., and Peng, J. (2020). The magnitude and drivers of harmful algal blooms in China's lakes and reservoirs: A national-scale characterization. Water Res. 181, 115902. doi:10.1016/j.watres.2020.115902
Huisman, J., Codd, G. A., Paerl, H. W., Ibelings, B. W., Verspagen, J. M. H., and Visser, P. M. (2018). Cyanobacterial blooms. Nat. Rev. Microbiol. 16, 471–483. doi:10.1038/s41579-018-0040-1
Jiang, L., Li, Y. P., Zhao, X., Tillotson, M. R., Wang, W. C., Zhang, S. S., et al. (2018). Parameter uncertainty and sensitivity analysis of water quality model in Lake Taihu, China. Ecol. Model. 375, 1–12. doi:10.1016/j.ecolmodel.2018.02.014
Jiang, X., Li, C., Shi, X., Sun, B., Zhao, S., Sun, C., et al. (2019). Melatonin enhances atherosclerotic plaque stability by inducing prolyl-4-hydroxylase α1 expression. Ecol. Environ. Sci. 028, 964–971. doi:10.1097/HJH.0000000000001979
Kim, D.-J., Cao, H., Jeong, K.-S., Recknagel, F., and Joo, G.-J. (2007). Predictive function and rules for population dynamics of Microcystis aeruginosa in the regulated Nakdong River (South Korea), discovered by evolutionary algorithms. Ecol. Model. 203, 147–156. doi:10.1016/j.ecolmodel.2006.03.040
Kim, J. H., Lee, D. H., and Kang, J. H. (2019). Associating the spatial properties of a watershed with downstream Chl-a concentration using spatial analysis and generalized additive models. Water Res. 154, 387–401. doi:10.1016/j.watres.2019.02.010
Koebbing, J. F., Patuzzi, F., Baratieri, M., Beckmann, V., Thevs, N., and Zerbe, S. (2014). Economic evaluation of common reed potential for energy production: A case study in wuliangsuhai lake (inner Mongolia, China). Biomass Bioenergy 70, 315–329. doi:10.1016/j.biombioe.2014.08.002
Li, Q., and Sang, G. (2021). Study on temporal and spatial distribution law of chlorophyll-a concentration in nansi lake. IOP Conf. Ser. Earth Environ. Sci. 676, 012030. doi:10.1088/1755-1315/676/1/012030
Li, X., Yang, Q. M., and Gou, M. M. (2011). Temporal and spatial distribution of water quality in Lake Wuliangsuhai, Inner Mongolia. Ecol. Environ. ences 20, 1301–1306.
Luo, Q., and Li, C. (2011). Seasonal variation and distribution of chlorophyll-a in WuliangsuHai lake. Water Sav. Irrig. 02, 36–39.
Mao, X., F., and Wei, X., Y. (2015). The spatial and temporal distribution of chlorophyll a and its influencing factors in an eutrophic lake. Environ. Monit. China 31, 65–70.
Padisák, J., Borics, G., Grigorszky, I., and Soróczki-Pintér, É. (2006). Use of phytoplankton assemblages for monitoring ecological status of lakes within the water framework directive: The assemblage index. Hydrobiologia 553, 1–14. doi:10.1007/s10750-005-1393-9
Peng, X., Zhang, L., Li, Y., Lin, Q. W., He, C., Huang, S. Z., et al. (2021). The changing characteristics of phytoplankton community and biomass in subtropical shallow lakes: Coupling effects of land use patterns and lake morphology. Water Res. 200, 117235. doi:10.1016/j.watres.2021.117235
Quan, D., Zhang, S., Shi, X., Sun, B., Guo, Z., Shuang, S., et al. (2020). Impact of water environment factors on eutrophication status of Lake Ulansuhai based on monitoring data in 2013-2018. J. Lake Sci. 32, 1610–1619. doi:10.18307/2020.0604
Recknagel, F. (2001). Applications of machine learning to ecological modelling. Ecol. Model. 146, 303–310. doi:10.1016/s0304-3800(01)00316-7
Recknagel, F., Cao, H., Kim, B., Takamura, N., and Welk, A. (2006a). Unravelling and forecasting algal population dynamics in two lakes different in morphometry and eutrophication by neural and evolutionary computation. Ecol. Inf. 1, 133–151. doi:10.1016/j.ecoinf.2006.02.004
Recknagel, F., French, M., Harkonen, P., and Yabunaka, K. I. (1997). Artificial neural network approach for modelling and prediction of algal blooms. Ecol. Model. 96, 11–28. doi:10.1016/s0304-3800(96)00049-x
Recknagel, F., Orr, P. T., Ba Rtkow, M., Swanepoel, A., and Cao, H. (2017). Early warning of limit-exceeding concentrations of cyanobacteria and cyanotoxins in drinking water reservoirs by inferential modelling. Harmful Algae 69, 18–27. doi:10.1016/j.hal.2017.09.003
Recknagel, F., Talib, A., and Molen, D. (2006b). Phytoplankton community dynamics of two adjacent Dutch lakes in response to seasons and eutrophication control unravelled by non-supervised artificial neural networks. Ecol. Inf. 1, 277–285. doi:10.1016/j.ecoinf.2006.01.001
Shi, R., Mao, R. Y., Zhang, M., Lü, Y., and Zhao, J. X. (2021). Distribution, sources, and ecological risks of polyfluoroalkyl substances in the surface water of the wuliangsuhai watershed. Environ. Sci. 42, 663–672. doi:10.13227/j.hjkx.202005216
Shi, R., Zhao, J., Shi, W., Song, S., and Wang, C. (2020). Comprehensive assessment of water quality and pollution source apportionment in wuliangsuhai lake, inner Mongolia, China. Int. J. Environ. Res. Public Health 17, 5054. doi:10.3390/ijerph17145054
Shu, J. (1990). The assessment methodology for eutrophication level of lakes in China. Environ. Pollut. Control 12, 2–7.
Sun, H. M., Jiang, H. E., Chang-Wei, L., Gao, X. D., Fan, Q. Y., and Xue, H. X. (2006). Nitrogen pollution and spatial distribution pattern of Wuliangsuhai Lake. Geogr. Res. 25, 1003–1012.
Tufffford, D. L., and McKeller, H. N. (1999). Spatial and temporal hydrodynamic and water quality modeling analysis of a large reservoir on the South Carolina (USA) coastal plain. Ecol. Model. 114, 137–173. doi:10.1016/s0304-3800(98)00122-7
Whigham, P., and Recknagel, F. (2001). Predicting chlorophyll-a in freshwater lakes by hybridising process-based models and genetic algorithms. Ecol. Model. 146, 243–251. doi:10.1016/s0304-3800(01)00310-6
Keywords: chlorophyll-a, hybrid evolutionary algorithm (HEA), ecological threshold, predictive modelling, differential evolution
Citation: Liu X, Liu H, Chen H, Liu Y, Xu Z, Cao X, Ma L, Pan B and Wang L (2023) Spatiotemporal distribution and prediction of chlorophyll-a in Ulansuhai lake from an arid area of China. Front. Environ. Sci. 11:1045464. doi: 10.3389/fenvs.2023.1045464
Received: 15 September 2022; Accepted: 06 January 2023;
Published: 16 January 2023.
Edited by:
Usman Atique, Chungnam National University, South KoreaReviewed by:
Salim Heddam, University of Skikda, AlgeriaCopyright © 2023 Liu, Liu, Chen, Liu, Xu, Cao, Ma, Pan and Wang. This is an open-access article distributed under the terms of the Creative Commons Attribution License (CC BY). The use, distribution or reproduction in other forums is permitted, provided the original author(s) and the copyright owner(s) are credited and that the original publication in this journal is cited, in accordance with accepted academic practice. No use, distribution or reproduction is permitted which does not comply with these terms.
*Correspondence: Lixin Wang, THh3YW5nQGltdS5lZHUuY24=
†These authors have contributed equally to this work
Disclaimer: All claims expressed in this article are solely those of the authors and do not necessarily represent those of their affiliated organizations, or those of the publisher, the editors and the reviewers. Any product that may be evaluated in this article or claim that may be made by its manufacturer is not guaranteed or endorsed by the publisher.
Research integrity at Frontiers
Learn more about the work of our research integrity team to safeguard the quality of each article we publish.