- 1Department of International Business and Economics, Bucharest Universityw of Economic Studies, Bucharest, Romania
- 2Department of International Business and Economics, Bucharest University of Economic Studies, Bucharest, Romania
- 3Department of Management, Marketing and Business Administration, “Lucian Blaga” University of Sibiu, Sibiu, Romania
In this study, we examine the contribution of nuclear, fossil (coal, oil, and gas), and renewable (hydro, solar, wind, biofuel) electricity sources to pollution in the globalization era, as measured by total greenhouse gases (GHG) produced by electricity per capita. We conduct an empirical investigation in a global panel of 163 countries which assesses both the concurrent and individual effects of alternative energy sources. Additionally, we implement a second model to assess the roles of various electricity sources on the carbon intensity of electricity generation. Robust GMM estimators show that fossil electricity is a major polluter and a driver of carbon intensity. Furthermore, nuclear and renewable energy reduce pollution on a global scale, with wind emerging as the most efficient energy source in the global fight against pollution and climate change. Moreover, globalization as measured by trade openness tends to reduce the carbon intensity of electricity production (CI), whereas biofuels have an increasing impact on CI. The findings have important policy implications, indicating that shifting to nuclear and renewable energy sources could help countries achieve their sustainable development goals more efficiently.
1 Introduction
The environmental degradation the world experiences for decades, with consequences in rising temperatures (Eluwole et al., 2020), changes in the climate of countries and regions (Whitmee, et al., 2015) and the increased frequencies of extreme natural events has severe repercussions for individuals’ health and life (Wei, et al., 2021), business’ plans and long-term strategies (Boiral, et al., 2012) and hinders countries’ further development (Zhao & Yuan, 2020). Carbon dioxide emissions have increased rapidly after WWII, fuelled by countries’ recovery after war and a fossil fuel driven economic growth, and have almost quadrupled in 1990 against 1950, reaching 22 billion tones, according to Our World in Data (OWID). Despite reduced activity in many sectors of the economy due to the coronavirus pandemic, the world emitted more than 34 billion tonnes in 2020, and emissions returned to near-pre-pandemic levels by the end of the year.
High polluting emissions are found in all countries, but the most densely populated and industrialised countries are at the top of global pollutants (Tudor & Sova, 2021). Even more concerning, very recent research shows that greenhouse gases emitted in a country may cause pollution and further warming in other countries, which significantly dampens economic growth (Callahan & Mankin, 2022). However, nuances enter the picture depending on the reference used to measure emissions, in terms of both total or per capita emissions, or indicator (carbon dioxide or greenhouse gas emissions). Thus, China was the biggest emitter of carbon dioxide from the burning of fossil fuels for energy and cement production in 2021 (10.67 billion tonnes), followed by the United States (4.71 billion tonnes) and India (2.44 billion tonnes), but in per capita terms the top is made of Mongolia (26.98 tonnes), Qatar (37.02 tonnes) and Kuwait (20.83 tonnes)—except for Mongolia, the other two countries are major oil producers. Nevertheless, CO2 is one of many greenhouse gases that are behind climate change, counting for 74.4% of all GHG emissions (OWID, data for 2016), so considering GHG emissions is equally important.
The global efforts towards fighting climate change were substantiated by the adoption in 2015 of the United Nations 2030 Sustainable Development Goals (SDG), under the assumed political goal of “leaving no one behind” (Weber, 2017), which aim at balancing social and economic well-being with environmental sustainability (UN, 2015; Fonseca, et al., 2020). The most prominent SDGs aiming at environmental protection are “Climate Change” (SDG 13) and “Affordable and clean energy” (SDG 7), both focusing on the integration of climate change measures into national policies and on increasing the ability of less developed countries to comply with environmental regulations (Sanchez Rodriguez, 2018). At the same time, the Paris Accords adopted at the COP21 Conference in 2015 began to be implemented, although the short period since the Agreement limits the ability to judge its efficacy, existing opinions have noted positive conclusions (Bulai, et al., 2021).
Fuel diversification for electricity production, accompanied by a larger share of renewable sources, is one of the most acclaimed measures that would lead to less carbon dioxide emissions. Low-carbon energy accounted for more than one-third (36.7%) of global electricity at the end of 2019, of which nuclear energy counted for 10.4% and renewable energy for 26.3% (with hydropower the most prominent renewable) but they represented only 15.7% in the global energy mix, due to the much higher reliance on fossil fuels by the other two components of the energy demand, i.e., transport and heating (OWID data based on BP Statistical Review of World Energy, 2020).
To quantify the relationship between the importance of energy sources in the energy mix and pollution, we use a two-pronged approach that distinguishes between “absolute pollution,” defined as GHG emissions from electricity per capita, and “relative pollution,” defined as carbon intensity from electricity. The model incorporates energy sources as the shares of various energy sources in total electricity output, allowing for an accurate estimation of the relationship between effect (electricity output) and potential causes (energy sources). This is a significant contribution to the literature, which is particularly scarce in addressing carbon intensity because it typically favours carbon dioxide or GHG emissions as variables depicting environmental quality. Furthermore, the econometric models we use allow for the estimation of energy mix impact on emissions generated by the electricity output in a combined manner - when all factors representing shares in electricity production of all energy sources, whether fossil or low-carbon—are used. In addition to assessing the cumulative impact of various energy sources on GHG emissions and carbon intensity, we investigate the individual or segregated effect of each energy source by incorporating their specific shares into the econometric estimation. Hence, we provide a global level ranking of the impact that each type of energy source, from fossil to nuclear and renewable, has on absolute and relative pollution, while assessing interactions between them when environmental quality is at stake. Thus, we contribute to the formulation of detailed approaches to tackle the relationship between energy sources and pollution to be included in environmental policies.
The rest of the paper is organized as follows. The following section provides a review of the related literature on the topic, followed by a description of the data and methodology. The sections Results and Discussion highlight the most important and relevant findings and compare them to the existing literature. The Conclusion section outlines the main implications of our study, as well as limitations and future research opportunities.
2 Literature review
2.1 Pollution and energy mix
The Environmental Kuznets Curve (EKC) represents the theoretical underpinning of the impact of economic growth and economic development on the environment (Grossman & Krueger, 1993). The EKC hypothesis, first discussed in the context of the NAFTA free-trade agreement’s impact on Mexico, posits a U-shaped non-linear relationship between environmental quality and economic growth/development; specifically, the EKC contends that environmental degradation is high in a country’s early development stages, but improves later, once economic development surges. From this, one can conclude that when an economy is in its early stages of development, environmental degradation rises faster than income, but it slows when income levels rise (Grossman & Krueger, 1993; Uchiyama, 2016).
The literature aimed at testing EKC has rapidly expanded, as concerns about climate change and the international effervescence surrounding environmental quality have increased significantly. However, there is no consensus yet on the validity of this hypothesis, as works have achieved mixed results. Dong, et al. (2018) confirmed the existence of EKC for China between 1993 and 2016, Sarkodie & Adams (2018) for South Africa between 1971 and 2017, Kim, et al. (2020) for the United States, Al-Mulali, et al. (2016) for Kenya, and the list may well continue. At the other end, Ng, et al. (2020) evidenced the validity of the EKC hypothesis for only 25 out of the 76 countries included in their global panel of countries, Miranda, et al. (2020) rejected the EKC for Canada, and Erdogan, et al. (2020) failed to confirm the hypothesis for 25 OECD countries between 1990 and 2014. Arnaut & Lindman (2021) suggested a simple U-shape of the EKC when investigated the impact of economic growth on carbon dioxide emissions in Greenland. In the same vein, Bandyopadhyay & Rej (2021) demonstrated the presence of an inverted N-shape for EKC in India, which shows that the initial stages of economic development were associated with higher environmental quality, but further development sparked environmental degradation; however, after the lowest level of environmental quality has been reached, it improves when fostered by more development. Similar findings have been provided by Koc & Bulus (2020) for Kenya over 1980–2012, and Balsalobre-Lorente, et al. (2018) for European countries.
Of the various factors or conditions that impact the EKC, the most interesting for our research are the energy mix and the use of low-carbon and renewable energy sources (including nuclear energy), and economic and financial globalization. For what concerns the mix of energy sources, Burke (2012) found that countries that are more endowed with fossil fuels are less likely to significantly alter their energy mix in favor of low-carbon sources compared to similar high-income countries. Zafar, et al. (2019) also stated that giving preference to low-carbon energy can successfully mitigate environmental damage without affecting economic growth. Nathaniel, et al. (2021) sustained that the more intensive use of nuclear and renewable energy can make an economy enter the descending part of the EKC sooner, thus allowing for a faster improvement of environmental conditions.
In a recent study, Murshed, et al. (2020) asserted that emissions depend on the type of energy resources consumed and concluded that renewable energy had a positive impact on emissions, while non-renewable energy led to increases in emissions. However, previous research on the impact of low-carbon energy on environmental quality has been mixed, but findings unequivocally show that the use of fossil fuels in the consumption and production of electricity has a negative impact on the environment (Davis, et al., 2010; Rashedi, et al., 2020; Bond, 2022) and even on the performance of economic sectors (Bulai, et al., 2021). To a large extent, renewables-based energy has a significant encouraging impact on pollution, as demonstrated by flourishing research on the topic–see, for example, Ahmed, et al. (2021), Dogan and Seker et al. (2016), Shahbaz, et al. (2019), Vo et al. (2019), and many more. Still, several studies disagreed with these findings and found that renewable energy was as responsible as non-renewable energy for environmental degradation–Adams & Nsiah (2019) for sub-Saharan African countries, Sinha, et al. (2019) for BRICS and Next11 countries. Others rejected the acclaimed positive influence of renewable energy on emissions–Hasnisah, et al. (2019) for developing economics from Asia, or Jebli & Youssef (2017) for North-African countries.
Among the low-carbon energy sources, the most controversial is nuclear energy, whose adoption and development stirred an effervescent debate in the literature. On the one hand, extant research has offered results that show the effectiveness of nuclear energy in bending emissions–see, for example (Apergis, et al., 2010), for 19 developing countries between 1984 and 2007, Baek & Pride (2014) for all top six nuclear energy producers (U.S., France, Japan, Korea, Canada, and Spain), in a multivariate cointegrated vector autoregression (CVAR) framework, or Nathaniel, et al. (2021) for G7 countries, except Canada and the US, using advanced panel models with cross-sectional dependence. Similar findings were reported by (Iwata, et al., 2012) for 11 OECD countries, in whose case a positive impact of nuclear power on carbon dioxide emissions was found only in Finland, Japan, Korea and Spain (Hassan, et al., 2020). studied BRICS countries between 1993 and 2017 and found that nuclear energy, although effective for emissions reduction, is suboptimal to renewable energy in fostering declines in pollution (Azam, et al., 2022). confirm the insignificant contribution that nuclear energy makes to mitigating environmental pollution for the top five polluting countries (China, United States, India, Japan, and South Korea), albeit accompanied by a strong positive impact of renewable energy consumption on emissions. On the other hand, many scholars, along with media non-governmental organizations and politicians, insisted on the disadvantages of using nuclear energy, which range from operational risks (such as explosion risk) with fatal consequences, the threat posed to the human health and environment by the disposal of nuclear waste, or the high investments and operational costs required to set up and operate nuclear plants (Schmidt, et al., 2019; Vossen, 2020; Yüksel and Çağlayan, 2020).
2.2 “Absolute” versus “relative” pollution
Despite this rich literature on the contribution of low-carbon energy sources to lowering environmental damage, which covered many countries and regions, periods, specific energy sources–from nuclear to various renewables–and used more or less advanced econometric methodologies, the debate over the specific impact of energy sources’ relative importance in the energy mix on environmental degradation, which would link the effect to its potential causes, has been launched only shyly in the literature. Moreover, the extant research has highly preferred carbon dioxide emissions as the proxy for environmental pollution, to a certain extent ignoring GHG emissions. Furthermore, researchers have focused to a higher extent their approaches on the “absolute pollution” (emissions quantity) instead of “relative pollution” (carbon intensity), which associates emissions to electricity produced. This is an important research direction that our paper addresses by providing insights into the specific energy sources’ contribution to both emissions and carbon intensity of generated electricity.
Burke (2013) is one of the few scholars that tackled environmental degradation through the lens of “relative pollution,” in the form of carbon intensity. He posited that countries climb the energy ladder differently, depending on their endowment in energy resources. Thus, countries with considerable energy resources are less likely to climb the energy ladder and fossil fuel-rich countries are less likely to adopt nuclear power and modern renewables as they get wealthier. However, a considerable portion of the world’s population resides in countries on the upward slope of the carbon intensity of energy curve, where energy systems are anticipated to become more dependent on carbon-intensive fossil fuels as per capita incomes rise. In his analysis of carbon intensity in renewable versus fossil fuel dominated electricity systems (Khan, 2019), argues that time-varying carbon intensity estimation can provide detailed insights into GHG emissions and help identify potential emission reduction opportunities from the electricity sector to combat pollution. The author contrasts Bangladesh, a developing country with very low carbon emissions that are expected to remain low despite industrialization and economic growth, with New Zealand, which generates more than 80 percent of its electricity from renewable sources and thus has few cost-effective options for further reducing emissions in the electricity sector. The author proposes an optimized use of fuels and seasonal emissions planning encapsulated in the development of energy policies for New Zealand, and a peak demand strategy for Bangladesh, combined with the integration of renewable sources in its electricity system, aimed at maintaining emissions low while allowing for the country’s industrialization.
The carbon intensity of electricity production depends on the electricity generation mix, hence both need to be considered when assessing carbon emissions and environmental degradation (Mattinen, et al., 2015; Tang, et al., 2022). In an analysis of the eco-efficiency of the electricity sector in EU-28 between 2010 and 2014, Tenente, et al. (2020) noted that a higher share of renewable sources in electricity production improves eco-efficiency of the production and consumption supply chain in the electricity sector (defined as the ratio between the value-added and the impacts produced, see Mahlberg & Luptacik (2014). The only exception within EU was Germany, where emissions increased in 2014 due to the discontinued operations of nuclear plants that were not replaced by renewable energy, the EU ETS crisis–where low prices for carbon emissions led to stagnant reductions in emissions, and the specific Staid aid fueled remuneration system for renewable energy in the country (Kirsten, 2014).
2.3 Environmental degradation and globalization
More recent empirical work in environmental degradation extends the models’ specifications by using globalization as a potential explanatory variable, building on the assumption that in a globalized world, the presence of foreign investors and the countries’ openness to trade are important channels to economic development; at the same time, foreign investments, either direct (FDI) and portfolio (FPI), as well as trade openness, may have effects on GHG emissions.
When the globalization–environmental degradation link was considered through the moderating impact of trade openness, which we also use in our investigation, researchers advanced the idea that carbon emissions may rise as a consequence of international trade, driven by three potential effects (Antweiler, et al., 2001): scale, which signifies heightened economic activity caused by trade, composition—which refers to the restructuring influence of trade on the economy (Rios & Gianmoena, 2018; Churchill, et al., 2020; Horobet, et al., 2021) -, and technology, supported by the multinational enterprises that introduce more advanced and environmentally friendlier technologies in host countries (Frankel & Rose, 2005; Radmehr, et al., 2021). Moreover, trading policies that encourage green activities can successfully propel a positive impact of trade on the environment, as suggested by Bandyopadhyay & Rej (2021) in the case of India. At the same time, there are scholars that validate a negative impact of trade and trade openness on emissions, usually in methodological settings that include other macroeconomic variables, such as GDP and energy consumption. For example, according to an early study by Chichilnisky (1994) developing nations were able to export low-priced environmental-intensive items because of an increase in trade openness, which had a negative impact on the quality of their own environment. More recently, Ertugrul, et al. (2016) investigated the top ten carbon dioxide emitters among developing nations between 1971 and 2011 and found that trade openness increased emissions, advising emerging countries to use environmentally-friendly technologies in the manufacture of trade goods. Also, Rafindadi, et al. (2018) concluded that trade implied carbon emissions were a key channel for pollution expansion in GCC countries. Wang & Zhang, (2021) concluded that a mitigating factor in the impact of trade openness on the environment is the country’s income level: for countries with lower level of income, the chance of a negative impact of trade on emissions is higher. This represents an indirect confirmation of the EKC.
3 Materials and methods
3.1 Data
This study uses annual data for 163 countries spanning the period 2000–2020. The sample period is chosen based on the fact that most of the renewable energy variables (for example, the share of solar and wind energy in electricity generation) were zero for most countries before 2000, which would have distorted results. Thus, to have more consistent findings, we chose the year 2000 as the starting point for the sample period. In turn, 2020 was the last year of available data at the moment of data collection.
Based on the data availability and aiming to keep the maximum number of available observations, we construct an unbalanced global panel that includes all countries with available data for the variables of interest for at least 3 years. This approach would also allow for the estimation of dynamic panel models capable to reveal any potential persistence of pollution in the sample panels.
Two pollution proxies were alternatively employed as dependent variables in empirical investigations: 1) greenhouse-gas emissions produced in the generation of electricity, measured in million tonnes of CO2 equivalent (GHG), and 2) carbon intensity of electricity production, measured in grams of carbon dioxide emitted per kilowatt-hour (CI). To obtain more consistent results, the GHG variable is further corrected by the total population, thus representing the total greenhouse-gas emissions produced in the generation of electricity per capita. The main regressors of interest are represented by the share of electricity generation that comes from alternative energy sources (i.e., fossil, nuclear, wind, solar, hydro, and biofuel). Economic development (GDP per capita) and globalization (trade openness) were also introduced in estimations as potential drivers for pollution and were retrieved from the Word Bank Indicators database. Table 1 summarizes the variables, including abbreviations, data sources, and description.
A visual inspection of the heterogeneity among countries (Figure 1) and the global trend Figure 2 of the two pollution indicators over the sample period reveals that both country and time effects are present and should be considered in reliable and consistent estimations. It is also worthwhile noting the declining trend of both mean GHG and mean CI, particularly after 2017 (for GHG) and 2015 (for CI), as well as the sharp drop in mean GHG in 2020, because of the Covid-19 pandemic. However, the wide variation across countries in GHG and CI is also observable, without a significant decline over years, which suggests that countries’ approaches to environmental degradation are quite diverse.
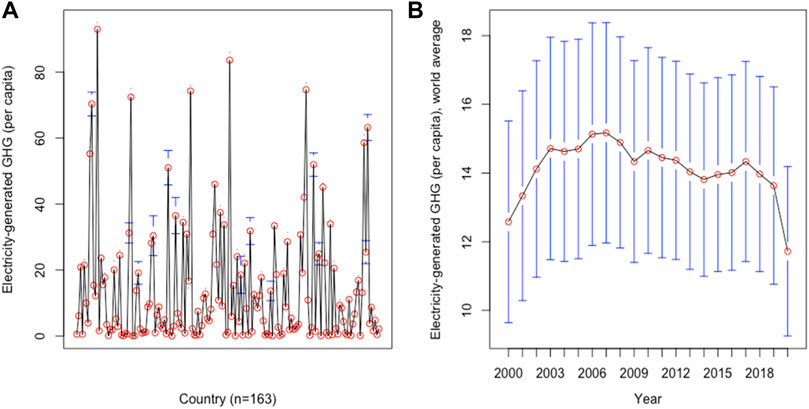
FIGURE 1. The mean level of greenhouse-gas emissions produced in the generation of electricity (GHG) by country over 2000–2020, including the confidence intervals Panel (A). The evolution of mean GHG worldwide over 2000–2020, with confidence intervals Panel (B). Source: Authors’ representation with data from Our World in Data, sourced from BP Statistical Review of World Energy and Ember.
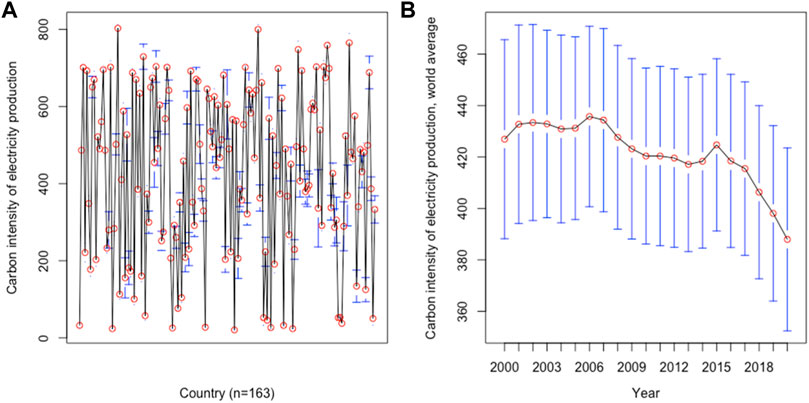
FIGURE 2. The mean level of the carbon intensity of electricity production (CI) by country over 2000–2020, including the confidence intervals Panel (A). The evolution of mean CI worldwide over 2000–2020, with confidence intervals Panel (B). Source: Authors’ representation with data from Our World in Data, sourced from BP Statistical Review of World Energy and Ember.
3.2 Method
The main relationships of interest that will be further assessed through a dynamic system-GMM panel model take the following form:
where, as per the previous discussion, the dependent variable X is alternatively the index representing the per capita greenhouse-gas emissions produced in the generation of electricity (GHG) and the carbon intensity of electricity production (CI). The other notations are explained in Table 1. The mix of regressors contains the economic development (the logarithm of the GDP per capita in constant 2015 US dollars), globalization (i.e., the trade openness index, defined as the sum of exports and imports of goods and services measured as a share of gross domestic product) and the factors representing the respective share of electricity generation that comes from renewable and unsustainable energy sources (fossil, nuclear, solar, wind, biofuel, and hydro). For more robust and consistent findings, we assess both the concurrent impact of the various energy sources for electricity generation (as per Eq. 1) and the individual or segregated effect of each source that is further introduced in estimations individually along with the factors representing globalization and economic output. Consequently, for each dependent variable, we estimate the extensive model depicted in Eq. 1 and six other models, allowing us to assess the segregated effect of each electricity factor.
In our models, GDP and GLOB may be considered control variables for the relationship between the shares of distinct energy sources in electricity generation and pollution. While the presence of GDP is highly motivated by the EKC hypothesis and the vast empirical literature testing it–see Section 2.1—globalization, included in our model as trade openness, is a variable driven by the assumption that international trade may have an impact on GHG and/or carbon dioxide emissions–see, in this regard, Section 2.3 on the extant literature that employs this variable in modelling pollution. We have decided to use only these two control variables in the model, as the issue of the accurate number of controls is still controversial. For example, Nielsen and Raswant (2018), citing Carlson and Wu (2012) and Spector and Brannick (2011), suggest that simply including more controls does not equate to rigor or even conservatism in terms of tests of hypotheses. Moreover, Becker et al. (2016) issued the famous recommendation: “when in doubt, leave them out!.” Also, due to the proliferation of instruments, it is problematic to conduct system GMM with many regressors, and in this case we already have six main variables of interest (the factors representing the share of distinct energy sources in electricity generation).
The generalized method of moments (GMM) that permits the exploration of the pollution variables persistence through the introduction of the lagged dependent variable is the estimation method of choice in this study. Of note, two types of GMM estimators are available, i.e., difference and system, and both can be used in a one-step or a two-step version (Tudor and Sova, 2022). We have chosen to employ the system-GMM (Sys-GMM) estimator of Arellano and Bover (1995) and Blundell and Bond (1998) which has been repeatedly acknowledged as a strong estimator (Canh et al., 2019). By including the lagged values of the dependent variable, the system-GMM is superior to the difference-GMM as it does not suffer from omitted dynamics in static panel data models (Omri & Nguyen, 2014). Furthermore, Sys-GMM is robust in the presence of potential endogeneity of regressors (Canh et al., 2019) and/or heteroscedasticity and autocorrelation within individuals (Roodman, 2009). Additionally, as the two-step GMM estimators can be biased downwards in finite samples (Blundell and Bond, 1998; Hoeffler, 2002), we choose to report one-step System GMM estimates (as per Biresselioglu et al., 2016; Berk et al., 2020; Tudor and Sova, 2022).
The final forms of the empirical models in their extensive form are depicted in Eqs 2, 3, respectively, where
To ensure the consistency of the Sys-GMM estimations, several model diagnostics were performed (Biresselioglu et al., 2016) and, consequently, the p-values for the J-test of over-identifying restrictions (Sargan, 1958; Hansen, 1982) for the null hypothesis of instrument validity and for the Arellano and Bond (1991) test for the second-order serial correlations in the residuals are reported in the results tables, along with Wald tests for coefficients and time dummies (Wooldridge, 2010). Of note, the time dummies would additionally capture any potential structural break in the time series (Corbacho et al., 2010; Rahman et al., 2019).
R software was employed to implement the method and carry out all estimations. In particular, the “pgmm” function within R’s “plm” (Croissant and Millo, 2008) was called to perform GMM estimations.
4 Results
Descriptive statistics for the global sample are reported in Table 2. The panel of sample countries reports a mean level of 60.88% for the share of electricity generation that comes from fossil fuels, with a maximum range of 100 percent. The same variation is encountered in the case of the share of electricity generation that comes from hydropower, although the mean value across the sample is lower (i.e., 29.09%). The share of electricity generation that comes from nuclear power registers a mean value of 4.76%, ranging from zero to almost 83 percent. Across renewable sources, solar energy is the least used in electricity generation at the world level, with an average value of 0.37% and a maximum of 15.12%. As expected for a global panel, the economic development as proxied by the GDP per capita also shows a high range, from a minimum of USD 281.97 to a maximum of USD 112417.9. Similarly, the carbon intensity pollution factor and the globalization factor show high variations.
4.1 The relationship between distinct electricity sources and electricity generated GHG emissions
Table 3 contains the estimation results of Eq. 2 with the Sys-GMM estimator over the period 2000–2020 for the unbalanced panel of 163 countries. As mentioned earlier, the factors representing economic income (GDP per capita) and globalization (trade openness) are introduced as control variables, and the GHG emissions from electricity represent the first explained variable.
We first notice that pollution is persistent and, thus, higher levels of GHG emissions in the previous period contribute to increased pollution in the current period. The autocorrelation parameter is statistically significant, such that a 1% increase in the lagged GHG advances the level of electricity generated GHG emissions in the current period by 0.44% at the world level.
The results further confirm prior findings that economic development is a major contributor to pollution, with a strong and significant effect (slope coefficient of 5.52, significant at 1%) on current GHG emissions generated from electricity when all electricity factors are concurrently introduced in the estimation.
Among the different electricity sources, wind and biofuel emerge as significant contributors to pollution mitigation in the global panel and the extensive model, with similar magnitudes (slope of −0,31 and −0.30, respectively).
Moreover, when individually introduced in estimations together with control variables (i.e., in M(2) to M(7)—see Table 4), all renewable sources decrease electricity-generated GHG emissions, with slope coefficients ranging from −0.05 for nuclear electricity to −0.36 for wind electricity. These findings thus indicate that wind emerges as the most efficient energy source in the global climate change combat. Solar and biofuel electricity are both pollution mitigators with similar slopes of −0.24 and −0.22, respectively, both significant at 10% level. It should be noted that wind and hydroelectricity sources have an indirect effect on pollution mitigation by eliminating the significant positive impact of the economic development variable on GHG emissions from electricity in the M(5) and M(7) model specifications.
Furthermore, results from the M(2) estimation confirm apriori expectations that fossil electricity spurs pollution, in line with previous studies. However, it should be noted that when all electricity factors are introduced in M(1), the slope coefficient of the fossil electricity factor decreases in magnitude (i.e., to −0.02) while losing statistical significance, whereas the economic income factor seems to take over much of its magnifying effect on pollution. In turn, we did not detect a significant link between globalization and pollution in any of the model specifications.
4.2 The relationship between distinct electricity sources and the carbon intensity of electricity production
The results for the CO2 intensity (CI) model estimation depicted in Eq. 3 also confirm apriori expectations by showing that an increased dependence on non-renewable energy leads to a rise in worldwide pollution as expressed by the carbon intensity of electricity production (see Table 5). Moreover, results of the extensive model that estimates the concurrent impact of the electricity factors reveal that biofuel is a promoter of increased CI for the global panel, indicating that increased carbon intensity can eliminate the emissions mitigation benefits of biofuels. In turn, trade openness seems to have a beneficial effect on CI, implying that green technology transfers via the trade route, contributing to increased efficiency in the production of electricity.
Subsequently, the impact of each electricity source on the carbon intensity index is also assessed individually. Table 6 reports the results for the global panel. Results indicate that hydroelectricity is again the most important contributor to pollution mitigation in EU countries, emerging as the strongest driver for energy efficiency (i.e., mitigator of carbon intensity). Wind electricity has the second strongest mitigating effect on carbon intensity worldwide, whereas nuclear and biofuel electricity were not found to contribute to an improved energy efficiency level when their segregated effects are assessed. Interestingly, economic development was not found to significantly alter CI, except for M(10) where nuclear electricity was used, which indicates that higher shares of nuclear sources in electricity production are, overall, specific to more developed countries. Of note, the economic development indicator changes its sign while gaining statistical significance when the hydroelectricity factor is introduced as the only independent variable among the distinct electricity factors in the model, suggesting that hydroelectricity can additionally foster a decreasing impact of economic development on carbon intensity.
5 Discussion
A difficult threat that the world faces today is generating sustainable development without any further environmental harm (Leal and Marques, 2021). In this context, the importance of alternative energy sources in reducing carbon emissions is becoming a major topic of discussion in the literature (Saidi and Omri, 2020). Thus, renewable energy and nuclear energy, defined as clean energy sources, have been shown to significantly reduce polluting emissions and thus promote worldwide decarbonization (Santoyo-Castelazo et al., 2014) without hampering economic growth (Azam et al., 2021). Consequently, one viable strategy for reducing global carbon footprints is to replace fossil fuels with low-carbon sources of energy for electricity generation (Ulucak and Erdogan, 2022).
The aforementioned causal relationships constituted a major motivator for this study. Therefore, for the first time, this paper assesses both the concurrent and individual impacts of multiple energy sources for electricity generation on greenhouse-gas emissions produced in the generation of electricity (“absolute pollution”) and on the carbon intensity of electricity production (“relative pollution”), while also considering the potential role of globalization at the world level. To achieve this goal, the study uses panel dynamic Generalised Method of Moments (GMM) models and the System GMM estimator, which produce reliable results by dealing with the usual issues that pertain to panel data. Moreover, all diagnostic tests attest that our models are properly specified, with the J test confirming the null hypothesis of instrument validity, the AR2 test confirming that there is no serious issue with the presence of second-order autocorrelation in the residuals, and the Wald test indicating that slope coefficients and time dummies are not simultaneously equal to zero.
First and most importantly, the results confirm the beneficial impact of renewable and nuclear energy on greenhouse-gas emissions produced in the generation of electricity, highlighting that wind and solar sources have the strongest mitigating effect on pollution at the world level, whereas, for EU countries, hydro and solar energy reduce electricity emissions the most. Therefore, in line with Lau et al. (2019), Saidi and Omri (2020), and Azam et al. (2021), we conclude that diversifying energy supplies to nuclear and renewable energy is paramount to mitigating global pollution. We also confirm the findings of (Simionescu, et al., 2022) that showed the substantial contribution of renewable energy consumption in reducing pollution in Romania, and of Horobet, et al. (2021) in the case of European Union.
However, in light of current findings (i.e., the magnitude of the coefficient of the nuclear factor is consistently smaller than the coefficient of “green” regressors), we fully agree with Hassan et al. (2020) that nuclear energy is less effective at reducing pollution than renewable sources, such as solar, wind, or hydro. Additionally, we confirm a positive effect of renewable (particularly hydro) and nuclear electricity on energy efficiency (as represented by a decreased carbon intensity of electricity generation). In this respect, current findings are in line with Cheng and Yao (2021) that confirm a negative and significant long-term effect of renewable energy technology innovation on carbon intensity in China. We also confirm the findings of Rahman et al. (2022) that an increase in renewable energy production and consumption can be an effective measure for carbon reduction. On the other hand, our findings also resonate with Budzianowski (2012) by showing that fossil-fuel electricity is inefficient in carbon conversion.
Moreover, current results also show that, while an increased dependence on renewable sources contributes to diminishing pollution, increased reliance on non-renewable (fossil) energy leads to a rise in environmental degradation. Thus, we agree with Destek and Sinha (2020) that countries should strive to reduce the share of fossil fuels in their energy mix and, in turn, increase the share of renewable energy sources. Moreover, we are in line with Rashid Khan et al. (2021) that studied the impact of two renewable sources, wind and solar electricity, on carbon intensity in a large panel of countries between 1990 and 2017 in an ARDL bounds testing framework and concluded that carbon intensity declines with increasing shares of wind and solar energy in electricity production, while the reverse is true in the case of coal and lignite. For Baltic states (Estonia, Latvia and Lithuania), Štreimikienė, et al. (2016) also argued that an increased share of renewables and improvements in energy efficiency have a direct impact on the economy’s energy carbon intensity, as well as on the reduction of energy import dependency, energy balance of trade, and energy mix diversification.
However, it should be noted that current results indicate that the increased carbon intensity of electricity production caused by using biofuels eliminates the GHG decline benefits, confirming previous findings (Njakou Djomo and Ceulemans, 2012). On the same vein, York (2016) warned that lowering carbon intensity of energy supply is generally coupled with rising energy demand, which means that the efforts to decarbonize the energy supply may be linked to excess energy consumption. This finding is worrying, as all types of energy contribute to environmental problems, although with different extent. Liu, et al. (2015) identified three types of factors that influence carbon intensity from electricity: energy intensity (i.e., ratio of energy consumption to the industrial added value), emission coefficient (i.e., ratio of carbon dioxide emissions to energy consumption) and energy structure (i.e., the ratio of added value between energy-intensive and non-energy-intensive industries). Of these, the energy intensity effect was the most important driver of carbon intensity decline between 1996 and 2012 in China. For US (Yi, 2015), learned that demand-side policies that favor clean energy had a positive impact on carbon emissions of the country’s electricity sector, while supply-side policies in the same direction propelled carbon intensity reduction.
Our models have not explicitly tested the EKC hypothesis, but we have included economic development among the independent variables in both GHG emissions and CI driving factors estimates. The results confirmed that economic development is a major contributor to pollution in our global panel of countries, in line with vast research on the topic, including works on the validity of the EKC–see, for example, the excellent review papers of Ali & de Oliveira (2018), He, et al. (2022), and Mardani, et al. (2019). Switching from fossil fuels to nuclear and renewable energy sources, on the other hand, increases the likelihood of mitigating this negative link between development and environmental degradation. Moving forward, an increased share of low-carbon and renewable energy in the total energy mix may have a positive impact on both economic growth and polluting emissions, resulting in a decoupling of economic growth and carbon emissions. Urban and Nordensvärd (2019) observed such a phenomenon in European Nordic countries, but these are higher-income countries that benefit from renewable energy as natural resources (particularly hydro and wind). Furthermore, they have made significant investments in fuel diversification and the promotion of renewable energy sources. For example, Denmark announced in 2020 plans to build two giant artificial “energy islands” that will cost up to 37 billion euro and are the core of a new climate package proposed by the Danish government that seeks to reduce by 70 per cent the country’s emissions by 2030 (Hook, 2020). Similarly, corporate investments in green transition are encouraged by the Swedish and Danish governments, as demonstrated by a new partnership established in 2021 with the aim of building solar and wind farms in both countries encompassing envisaged investments reach to EUR 200 million (European Energy, 2021).
A worrying finding is that pollution is a persistent phenomenon, as indicated by the statistical significance of the lagged values of GHG emissions, which is in line with the results of Liu, et al. (2020) and Solarin, et al. (2021) for various polluting substances Moreover, this implies that GHG emissions might continue to rise, as Tudor & Sova (2021) have predicted for a panel of twelve heaviest polluters globally, with a strong upward GHG trend in developing countries (Brazil, Indonesia and India) that seems to fully compensate the declines in emissions projected for the developed countries in the panel. This has dramatic consequences on population health, as well as on health systems and their costs (Hänninen, et al., 2011; Ragothaman & Anderson, 2017).
Finally, whereas previous results on the links between globalization and environmental pollution remain mixed, we did confirm that, for the global panel, globalization can decrease the carbon intensity of electricity production, and thus we agree with Leal and Marques (2021) that globalization can have a positive effect on environmental quality through green-technology spillovers, which in turn contribute to reducing the level of ecological footprint and enhancing the quality of the environment (Destek and Sinha, 2020). In fact, technological advancements brought through trade or foreign direct investments may have a positive impact on emissions (Smulders, et al., 2011; Pincheira & Zuniga, 2021; Su, et al., 2021) and even accelerate the meeting of the inflection point in the EKC, as Sun, et al. (2019) noted in their investigation of the direct and spillover effects of low-carbon innovations on carbon emissions. Moreover, we are in line with other results provided by extant literature that observe a fostering role for globalization on the connection between low-carbon and renewable energy and environmental quality (Dogan and Seker, 2016; Leitão & Balsalobre-Lorente, 2020; Horobet, et al., 2021).
6 Conclusion
Promoting economic development while preventing further environmental degradation is a global concern. The changing of the energy mix, for both consumption and production, is one of the most praised actions in the direction of sustainable development. In this context, our paper presents a thorough and comprehensive analysis of the contribution of conventional and low-carbon electricity sources to pollution in a diverse panel of 136 countries from all continents and all levels of development. The study makes several contributions to the field of research, the most important of which are 1) considering both GHG emissions and carbon intensity to measure pollution, thus distinguishing between “absolute” and “relative” pollution while linking the effect to the underlying causes; and 2) estimating the impact of the energy mix on the emissions generated by electricity output in a combined and segregated manner, thus providing a global level ranking of the effect of each energy source on pollution. Furthermore, we account for the impact of economic development and globalization on the relationship between energy mix and environmental degradation in the estimated models.
Our most important findings show that fossil-fuel-generated electricity is not only a major polluter but also a driver of carbon intensity. Unsurprisingly, nuclear and renewable energy have a global mitigating effect on environmental degradation, with wind emerging as the most efficient energy source in the global fight against climate change. Other significant findings show that, as measured by trade openness, globalization tends to reduce the carbon intensity of electricity production, whereas biofuels have an increasing impact on CI. These findings show that the energy mix matters when it comes to pollution prevention, and wind and biofuels are the most efficient energy sources for reducing GHG emissions and carbon intensity, respectively. Furthermore, we see globalization, specifically trade, as a driving force in pollution reduction.
Through these findings we further contribute to the formulation of detailed approaches to tackle the relationship between energy sources and pollution to be included in environmental policies. Thus, we consider that additional efforts to build an energy mix that is less intensive in conventional fuels and oriented towards renewable and nuclear energy will lead to reduced environmental degradation and foster economic development. However, changes in the energy mix need to be adapted to countries’ specific needs and resources endowments, optimizing the cost-effect component of the energy mix restructuring strategy.
Given the difficulty of decarbonizing transportation and heating, clean electricity will become increasingly important, and we are seeing initiatives around the world aimed at electrifying various components of the energy system, such as shifting to electric vehicles, increasing heating system efficiency, or creating sustainable energy. In this context, requiring countries to benefit from readily available energy sources such as wind or Sun should become a global priority, particularly for developing economies. Furthermore, the increased share of renewable sources in electricity output in the electricity mix of many low- and middle-income countries is a good starting point for transforming their economic development process into an environmentally friendly one.
Our study, like any other research, has limitations. They are caused by the data used and the availability of data, the time period under consideration, and the variables included in the models. All of these constraints can be addressed in future research, as well as the impact of specific policies on electricity-generated pollution. Furthermore, an avenue for future research endeavors that we believe is necessary to pursue is based on a difficult finding that indicates that the increased carbon intensity of electricity production caused by the use of biofuels negates the benefits of biofuels for GHG emissions. Also, globalization may be considered through foreign investment flows, both direct and portfolio, along with international trade and trade openness, to capture the potential interactions between globalization channels on environmental issues. Additionally, examining the effect of energy sources on pollution by focusing on different categories on countries, classified not only in terms of development level, but also depending on their existing energy mix, represents another interesting and potentially direction for future research.
Data availability statement
Publicly available datasets were analyzed in this study. This data can be found here: https://ourworldindata.org/ https://databank.worldbank.org/home.aspx.
Author contributions
AH and CT designed the research, CT collected the data and analyzed it in R software and wrote the Method and materials and Results sections. AH, LB, and DD wrote the Introduction and Literature review, AH and CT wrote Discussion and Conclusions. All authors revised the manuscript.
Funding
This work is supported by the Ministry of Research, Innovation and Digitization through Program 1—Development of the national research-development system, Subprogram 1.2—Institutional performance- Projects for financing excellence in RDI, Contract No. 28PFE/30.12.2021.
Conflict of interest
The authors declare that the research was conducted in the absence of any commercial or financial relationships that could be construed as a potential conflict of interest.
Publisher’s note
All claims expressed in this article are solely those of the authors and do not necessarily represent those of their affiliated organizations, or those of the publisher, the editors and the reviewers. Any product that may be evaluated in this article, or claim that may be made by its manufacturer, is not guaranteed or endorsed by the publisher.
References
Adams, S., and Nsiah, C. (2019). Reducing carbon dioxide emissions; Does renewable energy matter? Sci. Total Environ. 693, 133288–141393. doi:10.1016/j.scitotenv.2019.07.094
Ahmed, Z., Nathaniel, S. P., and Shahbaz, M. (2021). The criticality of information and communication technology and human capital in environmental sustainability: Evidence from Latin American and caribbean countries. J. Clean. Prod. 286, 125529. doi:10.1016/j.jclepro.2020.125529
Al-Mulali, U., Solarin, S. A., and Ozturk, I. (2016). Investigating the presence of the environmental kuznets curve (EKC) hypothesis in Kenya: An autoregressive distributed lag (ARDL) approach. Nat. Hazards (Dordr). 80 (3), 1729–1747. doi:10.1007/s11069-015-2050-x
Ali, S. H., and de Oliveira, J. A. P. (2018). Pollution and economic development: An empirical research review. Environ. Res. Lett. 13 (12), 123003. doi:10.1088/1748-9326/aaeea7
Antweiler, W., Brian, R. C. B., and Taylor, S. M. (2001). Is free trade good for the environment? Am. Econ. Rev. 91 (4), 877–908. doi:10.1257/aer.91.4.877
Apergis, N., Payne, J. E., Menyah, K., and Wolde-Rufael, Y. (2010). On the causal dynamics between emissions, nuclear energy, renewable energy, and economic growth. Ecol. Econ. 69 (11), 2255–2260. doi:10.1016/j.ecolecon.2010.06.014
Arellano, M., and Bond, S. (1991). Some tests of specification for panel data: Monte Carlo evidence and an application to em-ployment equations. Rev. Econ. Stud. 58, 277–297. doi:10.2307/2297968
Arellano, M., and Bover, O. (1995). Another look at the instrumental variable estimation of error-components models. J. Econ. 68 (1), 29–51. doi:10.1016/0304-4076(94)01642-d
Arnaut, J., and Lindman, J. (2021). Environmental sustainability and economic growth in Greenland: Testing the environmental kuznets curve. Sustainability 13 (3), 1228. doi:10.3390/su13031228
Azam, A., Rafiq, M., Shafique, M., and Yuan, J. (2022). Towards achieving environmental sustainability: The role of nuclear energy, renewable energy, and ICT in the top-five carbon emitting countries. Front. Energy Res. 9, 804706. doi:10.3389/fenrg.2021.804706
Azam, A., Rafiq, M., Shafique, M., Zhang, H., and Yuan, J. (2021). Analyzing the effect of natural gas, nuclear energy and renewable energy on GDP and carbon emissions: A multi-variate panel data analysis. Energy 219, 119592. doi:10.1016/j.energy.2020.119592
Baek, J., and Pride, D. (2014). On the income–nuclear energy–CO2 emissions nexus revisited. Energy Econ. 43, 6–10. doi:10.1016/j.eneco.2014.01.015
Balsalobre-Lorente, D., Shahbaz, M., Roubaud, D., and Farhani, S. (2018). How economic growth, renewable electricity and natural resources contribute to CO2 emissions? Energy Policy 113, 356–367. doi:10.1016/j.enpol.2017.10.050
Bandyopadhyay, A., and Rej, S. (2021). Can nuclear energy fuel an environmentally sustainable economic growth? Revisiting the EKC hypothesis for India. Environ. Sci. Pollut. Res. 28 (44), 63065–63086. doi:10.1007/s11356-021-15220-7
Becker, T. E., Atinc, G., Breaugh, J. A., Carlson, K. D., Edwards, J. R., and Spector, P. E. (2016). Statistical control in correlational studies: 10 essential recommendations for organizational researchers. J. Organ. Behav. 37 (2), 157–167. doi:10.1002/job.2053
Berk, I., Kasman, A., and Kılınç, D. (2020). Towards a common renewable future: The System-GMM approach to assess the convergence in renewable energy consumption of EU countries. Energy Econ. 87, 103922. doi:10.1016/j.eneco.2018.02.013
Biresselioglu, M. E., Kilinc, D., Onater-Isberk, E., and Yelkenci, T. (2016). Estimating the political, economic and environmental factors’ impact on the installed wind capacity development: A system GMM approach. Renew. Energy 96, 636–644. doi:10.1016/j.renene.2016.05.034
Blundell, R., and Bond, S. (1998). Initial conditions and moment restrictions in dynamic panel data models. J. Econ. 87 (1), 115–143. doi:10.1016/s0304-4076(98)00009-8
Boiral, O., Henri, J. F., and Talbot, D. (2012). Modeling the impacts of corporate commitment on climate change Business Strategy and the Environment 21 (8), 495–516.
Bond, D. (2022). Negative ecologies: Fossil fuels and the discovery of the environment. Oakland, California: University of California Press.
Budzianowski, W. M. (2012). Negative carbon intensity of renewable energy technologies involving biomass or carbon dioxide as inputs. Renew. Sustain. Energy Rev. 16 (9), 6507–6521. doi:10.1016/j.rser.2012.08.016
Bulai, V. C., Horobet, A., Popovici, O. C., Belascu, L., and Dumitrescu, S. A. (2021). A VaR-based methodology for assessing carbon price risk across European union economic sectors. Energies 14 (24), 8424. doi:10.3390/en14248424
Burke, P. (2012). Climbing the electricity ladder generates carbon Kuznets curve downturns. Aust. J. Agric. Resour. Econ. 56 (2), 260–279. doi:10.1111/j.1467-8489.2011.00572.x
Burke, P. (2013). The national-level energy ladder and its carbon implications. Environ. Dev. Econ. 18 (4), 484–503. doi:10.1017/s1355770x13000090
Callahan, C., and Mankin, J. (2022). National attribution of historical climate damages. Clim. Change 172, 40. doi:10.1007/s10584-022-03387-y
Canh, N. P., Schinckus, C., and Thanh, S. D. (2019). Do economic openness and institutional quality influence patents? Evidence from GMM systems estimates. Int. Econ. 157, 134–169. doi:10.1016/j.inteco.2018.10.002
Carlson, K. D., and Wu, J. (2012). The illusion of statistical control: Control variable practice in management research. Organ. Res. Methods 15 (3), 413–435. doi:10.1177/1094428111428817
Cheng, Y., and Yao, X. (2021). Carbon intensity reduction assessment of renewable energy technology innovation in China: A panel data model with cross-section dependence and slope heterogeneity. Renew. Sustain. Energy Rev. 135, 110157. doi:10.1016/j.rser.2020.110157
Churchill, S., Inekwe, J., and Ivanovski, K. (2020). Stochastic convergence in per capita CO2 emissions : Evidence from emerging economies. Energy Econ. 86, 104659. doi:10.1016/j.eneco.2019.104659
Corbacho, M. A., Medina, L., and Caceres, C. (2010). Structural breaks in fiscal performance: Did fiscal responsibility laws have anything to do with them? Washington D.C., US: International Monetary Fund.
Croissant, Y., and Millo, G. (2008). Panel data econometrics in R: The plm package. J. Stat. Softw. 27 (2), 1. doi:10.18637/jss.v027.i02
Davis, S. J., Caldeira, K., and Matthews, H. D. (2010). Future CO2 emissions and climate change from existing energy infrastructure. Science 329 (5997), 1330–1333. doi:10.1126/science.1188566
Destek, M. A., and Sinha, A. (2020). Renewable, non-renewable energy consumption, economic growth, trade openness and ecological footprint: Evidence from organisation for economic Co-operation and development countries. J. Clean. Prod. 242, 118537. doi:10.1016/j.jclepro.2019.118537
Dogan, E., and Seker, F. (2016). Determinants of CO2 emissions in the European Union: The role of renewable and non-renewable energy. Renew. Energy 94, 429–439. doi:10.1016/j.renene.2016.03.078
Dong, K., Sun, R., Jiang, H., and Zeng, X. (2018). CO2 emissions, economic growth, and the environmental kuznets curve in China: What roles can nuclear energy and renewable energy play? J. Clean. Prod. 196, 51–63. doi:10.1016/j.jclepro.2018.05.271
Eluwole, K. K., Saint Akadiri, S., Alola, A. A., Etokakpan, M. U., and Alola, A. A. (2020). Does the interaction between growth determinants a drive for global environmental sustainability? Evidence from world top 10 pollutant emissions countries. Sci. Total Environ. 705 (19), 135972
Erdogan, S., Okumus, I., and Okumus, A. E., G. (2020). Revisiting the environmental kuznets curve hypothesis in OECD countries: The role of renewable, non-renewable energy, and oil prices. Environ. Sci. Pollut. Res. 27 (19), 23655–23663. doi:10.1007/s11356-020-08520-x
Ertugrul, H. M., Cetin, M., Seker, F., and Dogan, E. (2016). The impact of trade openness on global carbon dioxide emissions: Evidence from the top ten emitters among developing countries. Ecol. Indic. 67, 543–555. doi:10.1016/j.ecolind.2016.03.027
European Energy (2021). New partnership to contribute to green energy in Denmark and Sweden. Soborg: European Energy.
Fonseca, L., Domingues, J., and Dima, A. (2020). Mapping the sustainable development goals relationships. Sustainability 12 (8), 3359. doi:10.3390/su12083359
Frankel, J., and Rose, A. (2005). Is trade good or bad for the environment? Sorting out the causality. Review of economics and statistics. Rev. Econ. Statistics 87 (1), 85–91. doi:10.1162/0034653053327577
Grossman, G., and Krueger, A. (1993). “Environmental impacts of the North American free trade agreement,” in The U.S. - Mexico free trade agreement. Editor P. Garber (Cambridge: MIT Press), 13–56.
Hänninen, O., Vardoulakis, S., Sarigiannis, D. A., Incecik, S., and Sokhi, R. S. (2011). Focus on exposure to air pollution and related health impacts. Air Qual. Atmos. Health 4 (3), 159–160. doi:10.1007/s11869-011-0137-4
Hansen, L. P. (1982). Large sample properties of generalized method of moments estimators. Econometrica 50, 1029–1054. doi:10.2307/1912775
Hasnisah, A., Azlina, A. A., and Che, C. M. I. (2019). The impact of renewable energy consumption on carbon dioxide emissions: Empirical evidence from developing countries in Asia. Int. J. Energy Econ. Policy 9 (3), 135–143. doi:10.32479/ijeep.7535
Hassan, S. T., Baloch, M. A., Tarar, Z. H., and Awais Baloch, M. (2020). Is nuclear energy a better alternative for mitigating CO2 emissions in BRICS countries? An empirical analysis. Nucl. Eng. Technol. 52 (12), 2969–2974. doi:10.1016/j.net.2020.05.016
He, Y., Liu, X., and Wang, X. (2022). How can environment get better? A research review of pollution governance. Manag. Environ. Qual. Int. J. 33 (2), 406–418. doi:10.1108/meq-08-2021-0187
Hoeffler, A. (2002). The augmented Solow model and the African growth debate. Available at: http://nrs.harvard.edu/urn-3:HUL.InstRepos:39469326.
Hook, L. (2020). Denmark reinforces green commitment with ‘energy islands’ plan. Lond. Financ. Times. MAY 20 2020.
Horobet, A., Popovici, O. C., Zlatea, E., Belascu, L., Dumitrescu, D. G., and Curea, S. C. (2021). Long-run dynamics of gas emissions, economic growth, and low-carbon energy in the European union: The fostering effect of FDI and trade. Energies 14 (10), 2858. doi:10.3390/en14102858
Iwata, H., Okada, K., and Samreth, S. (2012). Empirical study on the determinants of CO2 emissions: Evidence from OECD countries. Appl. Econ. 44 (27), 3513–3519. doi:10.1080/00036846.2011.577023
Jebli, B., and Youssef, B. (2017). The role of renewable energy and agriculture in reducing CO2 emissions: Evidence for North Africa countries. Ecol. Indic. 74, 295–301. doi:10.1016/j.ecolind.2016.11.032
Khan, I. (2019). Temporal carbon intensity analysis: Renewable versus fossil fuel dominated electricity systems. Energy Sources, Part A Recovery, Util. Environ. Eff. 41 (3), 309–323. doi:10.1080/15567036.2018.1516013
Kim, G., Choi, S. K., and Seok, J. H. (2020). Does biomass energy consumption reduce total energy CO2 emissions in the US? J. Policy Model. 42 (5), 953–967. doi:10.1016/j.jpolmod.2020.02.009
Kirsten, N. (2014). Renewable energy sources act and trading of emission certificates: A national and a supranational tool direct energy turnover to renewable electricity-supply in Germany. Energy Policy 64, 302–312. doi:10.1016/j.enpol.2013.08.030
Koc, S., and Bulus, G. (2020). Testing validity of the EKC hypothesis in South Korea: Role of renewable energy and trade openness. Environ. Sci. Pollut. Res. 27 (23), 29043–29054. doi:10.1007/s11356-020-09172-7
Lambropoulos, I., Alskaif, T., Schram, W., Bontekoe, E., Coccato, S., and van Sark, W. (2020). Review of energy in the built environment. Smart Cities 3 (2), 248–288. doi:10.3390/smartcities3020015
Lau, L. S., Choong, C. K., Ng, C. F., Liew, F. M., and Ching, S. L. (2019). Is nuclear energy clean? Revisit of environmental kuznets curve hypothesis in OECD countries. Econ. Model. 77, 12–20. doi:10.1016/j.econmod.2018.09.015
Leal, P. H., and Marques, A. C. (2021). The environmental impacts of globalisation and corruption: Evidence from a set of african countries. Environ. Sci. Policy 115, 116–124. doi:10.1016/j.envsci.2020.10.013
Leitão, N. C., and Balsalobre-Lorente, D. (2020). The linkage between economic growth, renewable energy, tourism, CO2 emissions, and international trade: The evidence for the European Union. Energies 13 (18), 4838. doi:10.3390/en13184838
Li, X., Chalvatzis, K. J., and Pappas, D. (2018). Life cycle greenhouse gas emissions from power generation in China’s provinces in 2020. Appl. Energy 223, 93–102. doi:10.1016/j.apenergy.2018.04.040
Liu, N., Ma, Z., and Kang, J. (2015). Changes in carbon intensity in China's industrial sector: Decomposition and attribution analysis. Energy Policy 87, 28–38. doi:10.1016/j.enpol.2015.08.035
Liu, S., Wang, L., and Guo, C. (2020). Heavy metal pollution and ecological risk assessment in brownfield soil from Xi’an, China: An integrated analysis of man-land interrelations. PLos One 15 (11), e0241398. doi:10.1371/journal.pone.0241398
Mahlberg, B., and Luptacik, M. (2014). Eco-efficiency and eco-productivity change over time in a multisectoral economic system. Eur. J. Operational Res. 234 (3), 885–897. doi:10.1016/j.ejor.2013.11.017
Mardani, A., Streimikiene, D., Cavallaro, F., Loganathan, N., and Khoshnoudi, M. (2019). Carbon dioxide (CO2) emissions and economic growth: A systematic review of two decades of research from 1995 to 2017. Sci. Total Environ. 649, 31–49. doi:10.1016/j.scitotenv.2018.08.229
Mattinen, M. K., Nissinen, A., Hyysalo, S., and Juntunen, J. K. (2015). Energy use and greenhouse gas emissions of air‐source heat pump and innovative ground source air heat pump in a cold climate. J. Industrial Ecol. 19 (1), 61–70. doi:10.1111/jiec.12166
Miranda, R., Hausler, R., Romero Lopez, R., Glaus, M., and Pasillas-Diaz, J. R. (2020). Testing the environmental kuznets curve hypothesis in North America’s free trade agreement (NAFTA) countries. Energies 13 (12), 3104–3113. doi:10.3390/en13123104
Moore, S. A., Rosenfeld, H., Nost, E., Vincent, K., and Roth, R. E. (2018). Undermining methodological nationalism: Cosmopolitan analysis and visualization of the North American hazardous waste trade. Environ. Plan. A 50 (8), 1558–1579. doi:10.1177/0308518x18784023
Murshed, M., Chadni, M. H., and Ferdaus, J. (2020). Does ICT trade facilitate renewable energy transition and environmental sustainability? Evidence from Bangladesh, India, Pakistan, Sri Lanka, Nepal and Maldives. Energy Ecol. Environ. 5 (6), 470–495. doi:10.1007/s40974-020-00190-2
Nathaniel, S., Alam, M. S., Murshed, M., Mahmood, H., and Ahmad, P. (2021). The roles of nuclear energy, renewable energy, and economic growth in the abatement of carbon dioxide emissions in the G7 countries. Environ. Sci. Pollut. Res. 28 (35), 47957–47972. doi:10.1007/s11356-021-13728-6
Ng, C. F., Choong, C. K., and Lau, L. S. (2020). Environmental Kuznets curve hypothesis: asymmetry analysis and robust estimation under cross-section dependence. Environmental Science and Pollution Research 27 (15), 18685–18698.
Nielsen, B. B., and Raswant, A. (2018). The selection, use, and reporting of control variables in international business research: A review and recommendations. J. World Bus. 53 (6), 958–968. doi:10.1016/j.jwb.2018.05.003
Njakou Djomo, S., and Ceulemans, R. (2012). A comparative analysis of the carbon intensity of biofuels caused by land use changes. Glob. Change. Biol. Bioenergy 4 (4), 392–407. doi:10.1111/j.1757-1707.2012.01176.x
Omri, A., and Nguyen, D. K. (2014). On the determinants of renewable energy consumption: International evidence. Energy 72, 554–560. doi:10.1016/j.energy.2014.05.081
Pincheira, R., and Zuniga, F. (2021). Environmental kuznets curve bibliographic map: A systematic literature review. Acc. Finance 61, 1931–1956. doi:10.1111/acfi.12648
Radmehr, R., Henneberry, S. R., and Shayanmehr, S. (2021). Renewable energy consumption, CO2 emissions, and economic growth nexus: A simultaneity spatial modeling analysis of EU countries. Struct. Chang. Econ. Dyn. 57, 13–27. doi:10.1016/j.strueco.2021.01.006
Rafindadi, A., Muhammad Muye, I., and Kaita, R. (2018). The effects of FDI and energy consumption on environmental pollution in predominantly resource-based economies of the GCC. Sustain. Energy Technol. Assessments 25, 126–137. doi:10.1016/j.seta.2017.12.008
Ragothaman, A., and Anderson, W. A. (2017). Air quality impacts of petroleum refining and petrochemical industries. Environments 4 (3), 66. doi:10.3390/environments4030066
Rahman, M. M., Rana, R. H., and Barua, S. (2019). The drivers of economic growth in south Asia: Evidence from a dynamic system GMM approach. J. Econ. Stud. 46 (3), 564–577. doi:10.1108/jes-01-2018-0013
Rahman, M. M., Sultana, N., and Velayutham, E. (2022). Renewable energy, energy intensity and carbon reduction: Experience of large emerging economies. Renew. Energy 184, 252–265. doi:10.1016/j.renene.2021.11.068
Rashedi, A., Khanam, T., and Jonkman, M. (2020). On reduced consumption of fossil fuels in 2020 and its consequences in global environment and exergy demand. Energies 13 (22), 6048. doi:10.3390/en13226048
Rashid Khan, H. U., Awan, U., Zaman, K., Nassani, A. A., Haffar, M., and Abro, M. M. Q. (2021). Assessing hybrid solar-wind potential for industrial decarbonization strategies: Global shift to green development. Energies 14 (22), 7620. doi:10.3390/en14227620
Rios, V., and Gianmoena, L. (2018). Convergence in CO2 emissions : A spatial economic analysis with cross-country interactions. Energy Econ. 75, 222–238. doi:10.1016/j.eneco.2018.08.009
Roodman, D. (2009). How to do xtabond2: An introduction to difference and system GMM in Stata. Stata J. 9 (1), 86–136. doi:10.1177/1536867x0900900106
Saidi, K., and Omri, A. (2020). Reducing CO2 emissions in OECD countries: Do renewable and nuclear energy matter? Prog. Nucl. Energy 126, 103425. doi:10.1016/j.pnucene.2020.103425
Sanchez Rodriguez, R., Urge-Vorsatz, D., and Barau, A. S. (2018). Sustainable Development Goals and climate change adaptation in cities. Nat. Clim. Chang. 8 (3), 181–183. doi:10.1038/s41558-018-0098-9
Santoyo-Castelazo, E., Stamford, L., and Azapagic, A. (2014). Environmental implications of decarbonising electricity supply in large economies: The case of Mexico. Energy Convers. Manag. 85, 272–291. doi:10.1016/j.enconman.2014.05.051
Sargan, J. D. (1958). The estimation of economic relationships using instrumental variables. Econometrica 26, 393–415. doi:10.2307/1907619
Sarkodie, S. A., and Adams, S. (2018). Renewable energy, nuclear energy, and environmental pollution: Accounting for political institutional quality in south Africa. Sci. Total Environ. 643, 1590–1601. doi:10.1016/j.scitotenv.2018.06.320
Schmidt, T. S., Schmid, N., and Sewerin, S. (2019). Policy goals, partisanship and paradigmatic change in energy policy–analyzing parliamentary discourse in Germany over 30 years. Clim. Policy 19 (6), 771–786. doi:10.1080/14693062.2019.1594667
Shahbaz, M., Balsalobre-Lorente, D., and Sinha, A. (2019). Foreign direct Investment–CO2 emissions nexus in Middle East and North African countries: Importance of biomass energy consumption. J. Clean. Prod. 217, 603–614. doi:10.1016/j.jclepro.2019.01.282
Simionescu, M., Neagu, O., and Gavurova, B. (2022). The role of quality of governance in reducing pollution in Romania: An ARDL and nonparametric bayesian approach. Front. Environ. Sci. 10, 358. doi:10.3389/fenvs.2022.892243
Sinha, A., Gupta, M., Shahbaz, M., and Sengupta, T. (2019). Impact of corruption in public sector on environmental quality: Implications for sustainability in BRICS and next 11 countries. J. Clean. Prod. 232, 1379–1393. doi:10.1016/j.jclepro.2019.06.066
Smulders, S., Bretschger, L., and Egli, H. (2011). Economic growth and the diffusion of clean technologies: Explaining environmental kuznets curves. Environ. Resour. Econ. (Dordr). 49 (1), 79–99. doi:10.1007/s10640-010-9425-y
Solarin, S. A., Gil-Alana, L. A., and Gonzalez-Blanch, M. J. (2021). Persistence of sulfur dioxide emissions in OECD countries between 1750–2014: A fractional integration approach. Int. J. Environ. Res. 15 (4), 701–708. doi:10.1007/s41742-021-00347-9
Spector, P. E., and Brannick, M. T. (2011). Methodological urban legends: The misuse of statistical control variables. Organ. Res. Methods 14 (2), 287–305. doi:10.1177/1094428110369842
Streck, C., Keenkyside, P., and Von Unger, M. (2016). The Paris agreement: A new beginning. J. Eur. Environ. Plan. Law 13 (1), 3–29. doi:10.1163/18760104-01301002
Štreimikienė, D., Strielkowski, W., Bilan, Y., and Mikalauskas, I. (2016). Energy dependency and sustainable regional development in the baltic states: A review. Geogr. Pannon. 20 (2), 79–87. doi:10.5937/geopan1602079s
Su, C. W., Xie, Y., Shahab, S., Faisal, C. M. N., Hafeez, M., and Qamri, G. M. (2021). Towards achieving sustainable development: Role of technology innovation, technology adoption and CO2 emission for BRICS. Int. J. Environ. Res. Public Health 18 (1), 277. doi:10.3390/ijerph18010277
Sun, H., Edziah, B. K., Sun, C. K. A. K., and Kporsu, A. K. (2019). Institutional quality, green innovation and energy efficiency. Energy Policy 135, 111002. doi:10.1016/j.enpol.2019.111002
Tang, B., Xu, Y., and Wang, M. (2022). Life cycle assessment of battery electric and internal combustion engine vehicles considering the impact of electricity generation mix: A case study in China. Atmosphere 13 (2), 252. doi:10.3390/atmos13020252
Tenente, M., Henriques, C., and da Silva, P. P. (2020). Eco-efficiency assessment of the electricity sector: Evidence from 28 European Union countries. Econ. Analysis Policy 66, 293–314. doi:10.1016/j.eap.2020.05.003
Tudor, C., and Sova, R. (2021). Benchmarking GHG emissions forecasting models for global climate policy. Electronics 10 (24), 3149. doi:10.3390/electronics10243149
Tudor, C., and Sova, R. (2022). Driving factors for R&D intensity: Evidence from global and income-level panels. Sustainability 14 (3), 1854. doi:10.3390/su14031854
Uchiyama, K. (2016). Environmental Kuznets curve hypothesis and carbon dioxide emissions. Tokyo: Springer Tokyo.
Ulucak, R., and Erdogan, S. (2022). The effect of nuclear energy on the environment in the context of globalization: Consumption vs production-based CO2 emissions. Nucl. Eng. Technol. 54 (4), 1312–1320. doi:10.1016/j.net.2021.10.030
UN (2015). Transforming our world: The 2030 agenda for sustainable development. New York, United States: United Nations, Division for Sustainable Development.
Urban, F., and Nordensvärd, J. (2019). Low carbon energy transitions in the Nordic countries: Evidence from the environmental Kuznets curve. Energies 11 (9), 2209. doi:10.3390/en11092209
Vo, A. T., Vo, D. H., and Le, Q. T. T. (2019). CO2 emissions, energy consumption, and economic growth: New evidence in the ASEAN countries. J. Risk Financ. Manag. 12 (3), 145. doi:10.3390/jrfm12030145
Vossen, M. (2020). Nuclear energy in the context of climate change: A frame analysis of the Dutch print media. Journal. Stud. 21 (10), 1439–1458. doi:10.1080/1461670x.2020.1760730
Wang, Q., and Zhang, F. (2021). The effects of trade openness on decoupling carbon emissions from economic growth – evidence from 182 countries. J. Clean. Prod. 279, 123838. doi:10.1016/j.jclepro.2020.123838
Weber, H. (2017). Politics of ‘leaving No one behind’: Contesting the 2030 sustainable development goals agenda. Globalizations 14 (3), 399–414. doi:10.1080/14747731.2016.1275404
Wei, P., Peng, Y., and Chen, W. (2021). Climate change vulnerability and key adaptation trajectory of the regional economic system. Discrete Dyn. Nat. Soc., 2021 1–14. doi:10.1155/2021/5540452
Whitmee, S., Haines, A., Beyrer, C., Boltz, F., Capon, A. G., de Souza Dias, B. F., et al. (2015). Safeguarding human health in the anthropocene epoch: Report of the rockefeller foundation–lancet commission on planetary health. Lancet 386 (10007), 1973–2028. doi:10.1016/s0140-6736(15)60901-1
Wooldridge, J. M. (2010). in Econometric analysis of cross–section and panel data. 2nd edition (Cambridge, Massachusetts, United States: MIT Press).
Yi, H. (2015). Clean-energy policies and electricity sector carbon emissions in the US states. Util. Policy 34, 19–29. doi:10.1016/j.jup.2015.04.001
York, R. (2016). Decarbonizing the energy supply may increase energy demand. Sociol. Dev. 2 (3), 265–272. doi:10.1525/sod.2016.2.3.265
Yüksel, S., and Çağlayan, Ç. (2020). The factors affecting the investors’ decisions: A study on nuclear energy investments. Elit. 2 (2), 177–185. doi:10.22440/elit.2.2.5
Zafar, M. W., Zaidi, S. A. H., Sinha, A., Gedikli, A., and Hou, F. (2019). The role of stock market and banking sector development, and renewable energy consumption in carbon emissions: Insights from G-7 and N-11 countries. Resour. Policy 62, 427–436. doi:10.1016/j.resourpol.2019.05.003
Keywords: pollution, GHG emissions, carbon intensity, globalization, system-GMM, nuclear energy, renewable energy
Citation: Horobet A, Tudor CD, Belascu L and Dumitrescu DG (2022) The role of distinct electricity sources on pollution abatement: Evidence from a wide global panel. Front. Environ. Sci. 10:996515. doi: 10.3389/fenvs.2022.996515
Received: 17 July 2022; Accepted: 07 September 2022;
Published: 19 September 2022.
Edited by:
Alex Oriel Godoy, Universidad del Desarrollo, ChileReviewed by:
Raufhon Salahodjaev, Tashkent State Economic University, UzbekistanAndrew Adewale Alola, Istanbul University, Turkey
Copyright © 2022 Horobet, Tudor, Belascu and Dumitrescu. This is an open-access article distributed under the terms of the Creative Commons Attribution License (CC BY). The use, distribution or reproduction in other forums is permitted, provided the original author(s) and the copyright owner(s) are credited and that the original publication in this journal is cited, in accordance with accepted academic practice. No use, distribution or reproduction is permitted which does not comply with these terms.
*Correspondence: Alexandra Horobet, YWxleGFuZHJhLmhvcm9iZXRAcmVpLmFzZS5ybw==