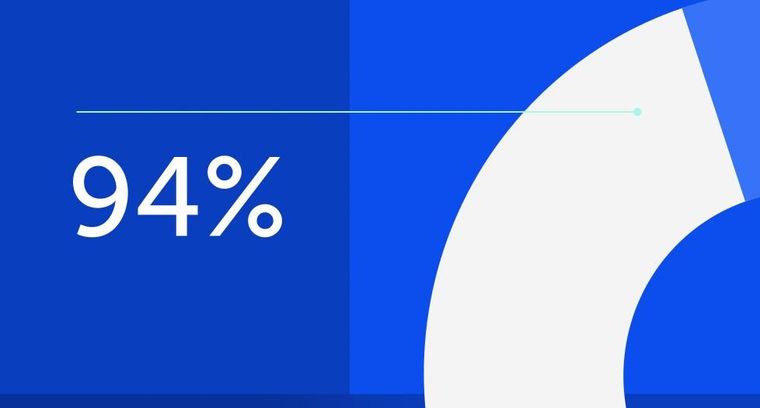
94% of researchers rate our articles as excellent or good
Learn more about the work of our research integrity team to safeguard the quality of each article we publish.
Find out more
ORIGINAL RESEARCH article
Front. Environ. Sci., 15 September 2022
Sec. Environmental Economics and Management
Volume 10 - 2022 | https://doi.org/10.3389/fenvs.2022.995990
This article is part of the Research TopicEconomic Development, Social Consequences, and Technological Innovation Under Climate Change COVID-19 Pandemic ConditionsView all 48 articles
China has been upgrading the industrial structure (ISU) at the regional level through innovation to achieve socioeconomic progress, but there is less known about the complex intermediary mechanisms regulating the government’s green innovation preference (GGIP)-ISU nexus from a regional perspective in China. Experts agree that varying environmental regulations (ER) intensity alters the relationship dynamics between innovation and ISU. Thus, using regional panel data (2005–2019), the paper develops an ER-based threshold regression model to assess the GGIP-ISU nexus under various ER levels in China. Following the statistically acceptable stationarity test outcomes, the regression corroborated the GGIP had disrupted ISU in China. Second, the ER-threshold model depicted that GGIP flexibility and ER rigidity were found interconnected, while the GGIP-ISU connection was characterized by a U-shaped relationship in which ER acted as a threshold variable. Third, the region-based heterogeneity test reflected that there was significant disparity in the inhibitory effect of GGIP on ISU between resource and non-resource-based regions, i.e., GGIP had a more significant inhibitory influence on the ISU in the non-resource-based regions than in the resource-based regions. The paper recommends critical policy implications for the enhancement of ISU in China.
Rapid economic progress in the emerging and developed economies has uplifted human living standards, but it has simultaneously produced ecological issues, e.g., desertification and pollution. As environmental governance issues and high economic quality have become important issues for sustainable development, many economies have optimized and upgraded their industrial structures (hereafter ISU) to accomplish sustainable development and growth. As an emerging global economy, China mainly relies on cheap labor, abundant resources, and large-scale production. China has been facing the problem of excess capacity in traditional industries and a low proportion of high-tech, low-carbon, and environmental protection industries (Ali et al., 2021; Alvarado et al., 2022; Baig et al., 2022; Hussain et al., 2022; Isik et al., 2022). Experts argue that an imbalanced industrial structure creates improper resource allocations and environmental pollution (cf. Hsieh & Klenow, 2009; Sarwar et al., 2019), posing a threat to sustainable development goals. In the 19th congress, many top Chinese Communist Party leaders emphasized the need to shift the Chinese economic growth model from high-speed to high-quality. The government has been trying to replace the traditional economic growth model of high energy consumption and pollution with an intensive economic growth model dominated by high-end manufacturing and the tertiary industry. Therefore, the central and provincial governments of China are promoting rationalization and ISU as the way forward for economic transformation. Chinese President Xi Jinping, in the Fourth Plenary Session of the 19th CPC Central Committee, stated that the innovation would promote the mechanism transformation of scientific and technological achievements by actively developing new driving forces, strengthening standardized guidance, and improving the essential capacity of the industry and the modernization of the industrial chain. Green technology innovation (GTI) is an effective way to promote the transformation of environmental governance from end-to-end governance to cleaner production and to achieve ISU (Zhong et al., 2022). Li and Zou (2018) noted that government preference for GTI could meet the practical needs of ISU and technology transformation in China. ISU is a vital way to achieve coordinated development, resource efficiency, and environmental up-gradation to address the current constraints of resources, energy, environment, and other factors. Beyond the above, ISU can enable China to manage the new domestic and international “dual-circulation” patterns while addressing the challenges of global climate change (Liao et al., 2020).
From a research viewpoint, growing global emphasis on achieving economic transformation using high-quality development has attracted significant academic focus where many studies have factors promoting ISU. Advocates of urban development recognize technological innovation (TI) and ISU as central to modern and sustainable urbanization while recognizing the role of government as critical to the whole process (e.g., Mieg, 2012; Wei et al., 2021). While Nilssen (2019) emphasized innovation typology as a critical matrix for defining smart urban cities and development, others (e.g., Pan et al., 2020) recommended inter-industry spillovers as a vital instrument for efficient urban land uses and economic prosperity. In a series of studies, Yao et al. highlighted the significance of social and innovation networks in shaping urban communities and cities (Yao et al., 2020; Yao et al., 2022). Academically, ISU is driven by different factors, including domestic enterprises (Brandt & Thun, 2010), regional innovation capability (Pickles, 2006), human capital level (Zhou, 2018), financial development (Jiang et al., 2020; Wang & Wang, 2021), economic growth (Dong et al., 2020), digital economy (Su et al., 2021), openness (He & Zeng, 2013), and environmental policies (Blair & Hite, 2005; Liu X. et al., 2021). In the development of cities, there is a coupling effect between ER, TI, and green development (Yin et al., 2022). Based on the causal inference framework of policy spillover effect, Gao and Yuan (2022) proved that the innovation intervention represented by the national innovative city pilot has a positive impact on the urban innovation performance and innovation convergence of the local and surrounding areas. Past academic view supports a close relationship between TI and green development (Zhao et al., 2019), which provides a series of theoretical bases for the relevant research in this paper. To summarize, there is a consensus among scholars that ISU and government support for innovation enable coupling, coordination, and innovative industrial development for socioeconomic development.
Therefore, many economists have mainly focused on the internal mechanisms governing the relationship between government innovation and industrial structure (Zhou & Li, 2012; Li & Lin, 2017; Zhu, 2022). Governments shape the market and drive the upgrading of enterprises through task-oriented innovation investment (TOII) and R&D subsidies (Mazzucato, 2016). The role of ISU can be traced back to Schumpeter’s “innovation theory.” Varum et al. (2009) used the same notion to defend that government innovation facilitates high productivity and growth in different industries to realize ISU, yet there are mixed views concerning this argument. For instance, Cai and Wang (2018) argued that government innovation stimulates industrial development by improving TI, production efficiency, and demand structure. Li and Yang (2015) contradict that inappropriate and unreasonable innovation investments hinder the ISU advancement to an advanced level, leading to ISU solidification. He and Zeng (2013) state that, under the existing capacity levels in China, only TI and improvement of production efficiency can break through the development of the low-end position of the industrial chain but marginally promote ISU as a whole. These contrasting views and the scarcity of empirical data make it difficult to claim whether [or not] GGIP’s influence on ISU is positive, negative, linear, or non-linear across regions in China. More so, there is little data on the relationship dynamics of GGIP, ISU, and ER in resource and non-resource-based regions.
In terms of the knowledge gap, there is an absence of a unified research framework combining GGIP, ER, and ISU, although many studies have documented various influencing factors of ISU, including environmental regulations (hereafter ER), technological innovation, financial policy, human capital, and other aspects (Martić and Savić, 2001; Ngai & Pissarides, 2007; Guo & Yuan, 2020; Han & Ma, 2020; Luo & Qi, 2021). Despite the plethora of previous academic research on ISU, the existing research has the following limitations: Firstly, the existing literature mainly focuses on the internal mechanism of government innovation and the influencing factors of the industrial structure or generally classifies the government’s influence on the industrial structure as an external driving factor. Only a few studies have explored the precise influence mechanism of government innovation preference on industrial structure. Moreover, scholars have studied the relationship between ER, GGIP, and industrial structure transformation. Yet, there is scarce information on the relationship between ER preference, GGIP, and ISU from the perspective of green motivation (Chen J. et al., 2021; Liu S. et al., 2022; Quan et al., 2022).
The main purpose of this paper is to explore the influence mechanism of GGIP and ISU in resource-based and non-resource-based areas of China. Taking ER as a threshold variable, this paper uses panel data from 30 provinces in China from 2005 to 2019 to verify the non-linear relationship using the double-threshold regression model. This paper uses green patents as a proxy for the GGIP. This factor has important implications for deeply understanding the importance of technological innovation and implementing appropriate ER. At the same time, ISU means that several high-polluting, high-energy-consumption, and low-value-added enterprises will be eliminated, thereby optimizing the industrial structure (Pan and Chen 2021; Deng and Zhao 2022; Miao et al., 2022). Therefore, this study is devoted to the influence of the GGIP on ISU and explores the threshold effect of ER and the linkage effect between the “flexible” GGIP and “rigid” ER, a novel aspect distinguishing this paper from existing literature.
The current research contributes to existing theory and research. First, the paper constructs a novel ER-based threshold model to explain the internal mechanism of ISU from an under researched context (China) while explaining how “flexible” GGIP and “rigid” ER are interconnected. Second, existing research generally regards GGIP as a general concept from the macro perspective (Tao et al., 2020). This paper discusses the influence of government innovation on ISU from the perspective of green motivation, providing a new perspective for studying ISU.
The rest of this paper can be divided into four parts: The second part is a literature review, which introduces the research status of government green innovation preference, environmental regulation, and industrial structure. The third part elaborates on the research design and empirical model analysis, variable selection, and data sources. The fourth section makes recommendations based on previous empirical evidence.
The topic of innovation has gained popularity in the urban development literature. Technological innovation (TI) and ISU are considered the main ways to promote new urbanization (Wei et al., 2021). Green TI and ISU can achieve the economy’s green transformation through environmental regulation (Du et al., 2021). Therefore, scholars have incorporated innovation into various studies of urban development. For instance, Mieg (2012) discussed commonalities between sustainable urban development and innovation, further explaining that identity seems as a particular governance-related resource in the relationships based on a resource-based view of urban project management. Nilssen (2019) comprised four dimensions of innovation (i.e., technological, organizational, collaborative, and experimental) to analyze empirical data from a Norwegian urban development project triggered by a critical juncture. The author supported the need for a typology of smart cities as multifaceted urban innovation. Furthermore, Pan et al. (2020) considered cross-industry spillovers as a missing link between land-use planning and urban economic futures and found that cross-industry spillovers positively impacted local employment on growth. Adopting a social network perspective, Yao et al. (2020) linked urban innovation with its location in the urban network. They confirmed that city innovation is not only determined by local innovation activities but also is enhanced when cities are deeply embedded in inter-city innovative networks. Yao et al. (2022) focused on the relationships between compact city indicators and comprehensive/technical efficiency, a multi-indicator system of compact cities, including population density, boundary limitation, and road density. The following part reviews the important studies on the relationships of ISU with ER and GGIP.
Economists agree that science and technology development is pivotal for industrial structure transformation (Ma & Yuan, 2004; Fu et al., 2013). Low-cost growth based on labor-intensive products is not sustainable without technological innovation (TI). Industrial upgradation and success often push wage rates and multiply costs in developing economies, yet the developing or under-developed competing countries benefit from low wage rates. Tech innovation can break through the original technical level, improve production efficiency, create new emerging industries and new markets (Zhou et al., 2016), adjust the structure of production and utilization demand, and promote the flow of factors (Ji, 2018), a few factors enabling ISU. Yan et al. (2020) noted that renewable energy-led TI significantly and positively uplifted green productivity in cases where a region’s relative income level exceeded a critical turning point. In another study, Jiang and Ji (2019) observed that, besides optimizing ISU at the local level, TI had contributed to the rational development of the adjacent regional economies. The authors stressed that the direction and intensity of the impact of innovation on the ISU vary among different regions, especially in China. Thus, there may be a non-linear relationship between innovation and ISU due to the synergistic and crowding effect of industrial agglomeration.
In the same vein, Endrikat et al. (2014) stated that achieving a win-win scenario appears impossible because green production and economic interests may be at odds with each other or sometimes even in a severe conflict. When the degree of outward diffusion of TI is relatively low, the demand for technological products will become the main driving force pushing the adjustment of the industrial structure. When the technological diffusion level is large, the allocation of technological elements and the demand for technological products are two factors (Chen Y. et al., 2021; Wu and Zhu 2021; Sheng et al., 2022). The combined effect of various aspects has reduced the industrial efficiency, thus making the industrial structure adjustment develop in the opposite direction. Therefore, the ISU adjustment and optimization (due to TI) create an inverted U-shaped relationship (Li & Dong, 2018). In a series of studies, Wang et al. (2020) found that green TI can promote total factor productivity, thereby driving the transformation of the economy to green and sustainable growth. In another study, Wang L. et al. (2021) asserted that implementing green innovation strategies can improve the resource utilization rate of enterprises, encourage the conversion of development models (from traditional labor-intensive to knowledge-based), enabling sustainable development of firms, a view consistent with many studies (e.g., Zhang et al., 2019; Zhang et al., 2020).
Previous works reflect an acute emphasis on the effects of the pollution-haven hypothesis on the adjustment of industrial structure. Most studies have concluded that variations in the standards of ER contribute to the production cost of carbon-intensive industries, affecting the industrial structure in a region (Kheder & Zugravu, 2012). Based on the relevant US data (1979–1990), Shadbegian and Gray, (2004) empirically analyzed the ER-production efficiency nexus for the steel, paper, and petroleum industries. The author observed a significant upsurge in the production costs and lower productivity due to ER in the selected industries. In another study, Gray and Shadbegian (1995) found that the high intensity of ER inhibits polluting enterprises from improving their production efficiency and offers a low financial incentive for adopting environmentally friendly production methods. Therefore, some experts assert the need for balanced and incremental implementation of ER policies, enabling enterprises to improve innovation capabilities and scientifically allocate resources, thereby enhancing their competitiveness (Michael et al., 1995). Another study by Cole et al. (2005) examined how formal and informal ER affect ISU. The author observed that ISU demonstrated a significant U-shaped threshold characteristic when the intensity of formal ER increased. Chen L. et al. (2022) found that informal ER exhibited a double-threshold effect in China. In another study, Wang M. et al. (2021) empirically tested the impact of heterogeneous ER on ISU, deducing that compared to command-controlled ER (CER) and the voluntary public participation ER (VER), market-incentive ER (MER) had a stronger effect on ISU.
Nonetheless, the majority of previous findings reflect two opposing views: 1) ER pushes ISU; 2) the relationship between ER and ISU is uncertain. In line with the former perspective, Lu (2007) found that ER pushes technological progress and promotes ISU. In another study, Gao et al. (2012) observed a positive correlation between the intensity of ER and the optimization of the industrial structure of the manufacturing industry. As per Qu and Wang (2002), the implementation of strict ER standards is conducive to ISU in the long run. Xue (2016) stated that ER affects the industrial structure of the regional equipment manufacturing industry by affecting economic growth. Alternatively, in support of the second perspective, Wu et al. (2019) drew from previous studies to conclude that the effect of ER on ISU in the manufacturing industry differs across different regions in China, i.e., the progress in East China is superior to the Central and West China. Some recent studies show that ER suppresses ISU if the industries exhibit a low level of TI. If the industry holds a medium and high level of scientific and TI, ER can promote the ISU (Sun et al., 2020). Another study explained that ER significantly inhibits ISU in industries with a low level of human capital, but it encourages ISU in industries with a medium or high level of human capital. Considering the industrial modernization of China, some economists argue that ER has played a substantial role in ISU of major cities, even though it is not easy to gauge the intensity and scope of such effect across different stages (early-middle) of development in different cities in China (Cheng et al., 2017).
Per the traditional neoclassical economic theory, ER produces a cost effect of compliance, inhibiting enterprises from actively carrying out TI (Altman, 2001). The revisionist school represented by Porter believes that ER generates innovation compensation effects. ER effectively incites firms to pursue innovation (scientific or technological) and develop green technologies, processes, and products for the following reasons: 1) enhance enterprise core competitiveness; 2) partially or entirely offset economist costs generated by environmental regulations (Song et al., 2022a; Chen Y. et al., 2022). Only when the innovation’s compensation effect (led by environmental regulation) exceeds the cost-effectiveness of compliance can enterprises get the opportunity to promote their transformation and upgrading through green TI, which unifies the Porter hypothesis and traditional neoclassical economic theory (Liu X. et al., 2022). At present, the current literature on the topic is dominated by the following three main viewpoints. The first perspective, built on the compensation cost theory, advocates that environment-related laws and legislation inhibit green TI. Extra funds for waste disposal (in response to ER) drain out the capital investment of firms for TI (Qiao et al., 2022). Yuan (2022) analyzed A-share listed data (2011–2019) to conclude that environmental protection tax significantly inhibited the green TI of firms based in Shanghai and Shenzhen, China. Compared to the non-state-owned enterprises, the environmental protection tax significantly inhibited the green TI ability of state-owned enterprises.
The second perspective, based on the tenets of the innovation compensation theory, proposes that ER promotes green TI (Song et al., 2022b; Chen L. et al., 2022). The increasing intensity of ER can indirectly stimulate enterprises to enhance scientific and TI, offsetting the cost of pollution prevention and control, thereby promoting TI (Lanoie et al., 2008). Drawing from the strong Porter hypothesis, Jaffe and Palmer (1997) state that stimulating enterprises to innovate in production technology can benefit enterprises in two ways. While the benefits obtained by ER can significantly offset the cost of improving the environment, they can simultaneously trigger an innovation compensation effect, enabling enterprises to yield sustainable development dividends. Strict and efficient ER and high-intensity R&D investment, coupled with independent R&D at the core, can generate a high level of regional green TI (Gao et al., 2022). Based on the uncertainty theory, the third perspective considers the ER-green TI nexus as non-linear, claiming no significant relationship exists between the two factors. Lu (2022) used the panel data of thirty provinces in China (2011–2019) to support the U-shaped connection between green TI and ER.
This paper selected the panel data of thirty provinces in China, covering a period from 2005 to 2019. Tibet data were excluded from the sample due to missing items. Table 1 shows the data sources, descriptions, annotations, and variables.
In GGIP, the independent variable, was adopted in this study, measured by the sum of green patents. Previous studies (e.g., Pei et al., 2019) have used the sum of the number of utility models (granted) and invention patents as the measurement index. A high index number reflects that GTI is high. The natural logarithm processing of the index was used to obtain GGIP.
Advanced industrial structure (ln TS), a proxy of ISU, was adopted as the dependent variable. Even though most researchers, following Clark’s Law, have used the proportion of non-agricultural output value as the measure of the ISU, it fails to reflect the new trend, i.e., service-oriented economy. The information technology revolution after the 1970s greatly impacted the industrial structure of major industrialized countries. The servitization of the economic structure driven by informatization is a key feature of the ISU, in that tertiary industries have grown faster than secondary industries in the economic servitization. Following the work of Gan et al. (2011), the paper adopted the tertiary-secondary industry output ratio as a measure of the advanced industrial structure. This indicator reflects the servitization tendency of the economic structure and indicates whether the industrial structure is developing in the direction of servitization. An increase in the advanced industrial structure value represents the economy moving toward superior servitization and the industry structure improving.
This study integrated environmental regulation (ER) into the model as the threshold variable. Following the approach of Ye et al. (2018), Hao and Zhang, (2016), and Ren et al. (2020), the current study incorporated the emissions of three industrial wastes to obtain the intensity of comprehensive ER. A high ER value indicates high pollution emissions, while a weak ER intensity denotes low pollution. ER was computed in the following way. First, the steps involved standardizing the industrial wastewater discharge per unit of output value, the industrial SO2 discharge per unit of output value, and the industrial smoke and dust discharge per unit of output value, as seen in Eq. 1 below:\
Where,
As depicted in Eq. 2, the weight of various pollutants was computed as follows:
Where,
As shown in Eq. 2, the comprehensive index of environmental regulations for the city i was calculated as follows:
Following Hansen (1999), Eq. 4 reflect the basic model for testing the effect of government green innovation preference on industrial structure upgrading, whereas Eq. 5 depicts the panel threshold regression with ER as the threshold variable.
Where, i = region; t = year;
The paper incorporated different control variables, including financial input (FN), human capital level (lnHC), financial development (FD), regional economic development (lnGDP), degree of opening to the outside world (lnOP), and infrastructure (lnINF)—for the following reasons. Firstly, the quantity and quality of human capital directly determine the success or failure of upgrading the industry at the local and national levels. Secondly, financial support and financial investments provide a certain financial guarantee for ISU (TS) by providing necessary economic support for ISU (TS). Thirdly, the level of regional economic development facilitates ISU (TS), determining whether the ISU (TS) can be realized. Fourthly, the degree of opening to the outside world (lnOP) is reflected in the absorption of advanced technologies and experience from developed countries and international trade of products, thereby affecting ISU (TS) to varying degrees. Fifthly, infrastructure (lnINF) provides the basic material guarantee for ISU (TS).
The descriptive statistics of each variable are illustrated in Table 2. The mean value, standard deviation, maximum value, and minimum value of ISU (TS) was 1.174, 0.65, 5.234, and 0.527, respectively, reflecting a large gap in different provinces. The GGIP (LNGR) average was 6.798. More so, the standard deviation was 1.657. The min-max values were 0.693–10.436, indicating that the innovation level was largely unbalanced during the study period in different regions of China.
The unit root and stationarity test results provided empirical ground for the stationary of all variables at the first difference level (depicted in Table 3).
Preliminary Hausman test results offered support for the application of regression analysis with fixed (FE)- and random effect (RE) model as a reference, as shown in Table 4. The two models corroborated the inhibitory effect of GGIP (lnGR) on ISU (TS) in China (i.e., RE = −0.0752 and FE = −0.1757), supporting the FE model outputs of Li and Yang (2015). A logical explanation is that government grants more green patents to the secondary industries, stimulating a market change from tertiary to secondary industry. This shift effects GGIP (lnGR) in a manner that (rather than supporting) it inhibits the advancement of ISU (TS) to the next stage. Consistent with the previous studies (e.g., Liu & Wang, 2021), the FE coefficient indicated the following effects of different variables on ISU (TS) at 1% significance: insignificant (financial investments, FN = 0.0449, Ln GDP = 0.449); negative (infrastructure, ln INF = -0.1962, degree of openness, lnOP = -0.0809), and positive (human capital level, lnHC = 0.3690).
The threshold regression results with ER as the threshold variable are shown in Table 5. The model only passed the single-threshold test (threshold value = 0.0041), reflecting an inverted U-shaped effect of ER on ISU (TS). As seen below, GGIP (lnGR coefficient = 0.212, 1% significance level) promoted ISU (TS) when the ER value was less than 0.0041. In contrast, GGIP (lnGR coefficient = −0.0648) disrupted ISU (TS) when the ER value exceeded 0.0041. The threshold predictions implied that technological advancement and cost reduction (through patents and other tolls) in the early stages of ER implementation had facilitated industrial transformation and upgrading (ISU) in China. The model outputs signaled that the government restrained the development of polluting industries through various measures (e.g., ER taxation and pollution fines) after reaching a certain threshold in the development of environmental protection technology and formalization of ER laws, consequently slowing down the new round of development and progress of ISU.
The robustness check results are given below in Table 6. The robustness check of the research results was carried out in the following two ways. Method one comprised change of the time interval (cf. Zhang et al., 2022), while the explanatory variables was replaced in Method 2. Instead of using the sum of invention patents and utility model patents for GGIP (lnGR), ISU (TS) was regressed with the replacement of authorized invention patents. Overall, the signs and coefficient valves of all variables in the two methods of the robustness test were in line with Table 4, indicating that the model fitting and construction of regression results were robust.
As the policy environment and industrial structure vary among different regions in the vast territory of China, the sample was divided into resource-based and non-resource-based regions according to the differences in regional industrial structure and resource endowments. Resource-based regions included Hebei, Shanxi, Inner Mongolia, Liaoning, Jilin, Heilongjiang, Sichuan, Guizhou, Yunnan, Shaanxi, and Xinjiang, while the remaining provinces were considered non-resource-based regions. As reported in Table 7, GGIP had a stronger inhibitory effect on the ISU (TS) in non-resource-based regions (lnGR = −0.2327) than resource-based regions (lnGR = = −0.0912) of China. For control factors, government financial expenditure (FN) and the degree of opening (lnOP) had a significant controlling role in the resource-based regions, yet the impact of these factors on ISU (TS) was weak.
The key objective of this article was to measure the impact of GGIP on ISU under different levels of ER using Chinese provincial panel data from 2005 to 2019. All the variables in the study passed the unit root test and stationarity testing. The regression analysis suggested that GGIP prevented ISU in Chinese regions, while FN and GDP had insignificant effect on ISU, infrastructure and OP had a negative effect on ISU, and HC positively impacted ISU. The main regression method, with ER as a threshold variable, confirmed the non-linear inverted U-shaped relationship between GGIP and ISU through the coefficient of the quadratic term (e.g., Zhang et al., 2022). The threshold regression delineated that the ER rigidity controlled the inhibitory role of GGIP in improving ISU. More so, the heterogeneity analysis demonstrated non-resource-based region and resource-based region differed in the intensity of inhibitory influence of GGIP on ISU, as the inhibitory effect was relatively more significant in non-resource-based regions.
The paper proposes the following recommendations according to the current findings. First, the government should create a conducive atmosphere and continuously improve the level of TI. Regional governments can enhance emphasis on basic research and original innovation to provide a source for GTI progress by continuously expanding the R&D frontiers in different regions; deepening the protection of intellectual property rights in GTI; by actively introducing market mechanisms to build a platform for the transformation of GTI projects to promote advanced technology for enhancing the development of enterprises; by mobilizing the role of ER in promoting ISU. Second, the current findings assert the need for simultaneous development of GTI and ER. The central and local governments should introduce a balanced approach in terms of implementation, rules, incentives, and penalties, considering the restraining effect of GTI on ISU. Third, the current findings indicate that the rationalization of the industrial structure should be realized in cooperation with ER. With nascent insight into the dynamics of ER, the rapid expansion of the tertiary industry in China could be harmful. Under the self-regulating action of the market, the government should give good play to the dispatching role of government regulations so that the industrial structure can be upgraded at an appropriate speed and the industrial structure can be rationalized appropriately in a systematic manner. Fourth, the current findings call for promulgation of indigenous policies tailored to local conditions to prevent the inhibitory effects of GGIP on ISU in different regions, particularly non-resource-based regions. It is necessary to provide corresponding industrial policy support to realize the adaptive development and balance between resource-based and non-resource-based regions. While achieving green development, the government should support the industrial policy of various regions and achieve coordinated and high-quality development.
Although the paper brings fresh insight into regional innovations in China, it has the following shortcomings. First, the current findings are limited in representing the interaction among GGIP and ISU of provincial governments in thirty provinces of China. Second, the paper explored the impact of GGIP on the rationalization of ISU in addition to ISU. As the results were not ideal, they were excluded. It appears that GTI has not sufficiently affected the rationalization of the industrial structure, yet its significant impact on the rationalization of the industrial structure cannot be underestimated in other parts of the world. Third, it is assumed that the province panel data cannot fully reflect the effect of GGIP on industrial structure. Given that prefecture-level city or micro-enterprise data may be able to make the research more representative (Long et al., 2017), follow-up research is expected to bring fresh insight.
The original contributions presented in the study are included in the article/Supplementary Material, further inquiries can be directed to the corresponding author.
SK: Supervision, conceptualization, Editing. AK: Proofreading MK: Supervision JL: Introduction part, preparation ZP: Literature review part, preparation CL: Data handling, methodology, statistical software.
The authors declare that the research was conducted in the absence of any commercial or financial relationships that could be construed as a potential conflict of interest.
All claims expressed in this article are solely those of the authors and do not necessarily represent those of their affiliated organizations, or those of the publisher, the editors and the reviewers. Any product that may be evaluated in this article, or claim that may be made by its manufacturer, is not guaranteed or endorsed by the publisher.
Ali, S., Yan, Q., Elavarasan, R. M., Irfan, M., Abbasi, K. R., and Işik, C. (2021). Modeling biogas technology adoption in Pakistan: A way forward for sustainable research of energy. doi:10.21203/rs.3.rs-994158/v1
Altman, M. (2001). When green isn't mean: Economic theory and the heuristics of the impact of environmental regulations on competitiveness and opportunity cost. Ecol. Econ. 36 (1), 31–44. doi:10.1016/s0921-8009(00)00211-1
Alvarado, R., Tillaguango, B., Murshed, M., Ochoa-Moreno, S., Rehman, A., Işık, C., et al. (2022). Impact of the informal economy on the ecological footprint: The role of urban concentration and globalization. Econ. Analysis Policy 75, 750–767. doi:10.1016/j.eap.2022.07.001
Baig, I. A., Irfan, M., Salam, M. A., and Işik, C. (2022). Addressing the effect of meteorological factors and agricultural subsidy on agricultural productivity in India: A roadmap towards environmental sustainability. doi:10.21203/rs.3.rs-1776797/v1
Blair, B. F., and Hite, D. (2005). The impact of environmental regulations on the industry structure of landfills. Growth Change 36 (4), 529–550. doi:10.1111/j.1468-2257.2005.00293.x
Brandt, L., and Thun, E. (2010). The fight for the middle: Upgrading, competition, and industrial development in China. World Dev. 38 (11), 1555–1574. doi:10.1016/j.worlddev.2010.05.003
Cai, Y., and Wang, H. (2018). Research on the influence mechanism of innovation input on industrial structure upgrade——analysis based on quantile regression. Inq. into Econ. Issues (01), 138–146. (in Chinese).
Chen, J., Wang, Q., Huang, J., and Chen, X. (2021). Motorcycle ban and traffic safety: Evidence from a quasi-experiment at zhejiang, China. J. Adv. Transp. 2021, 1–13. doi:10.1155/2021/7552180
Chen, L., Li, W., Yuan, K., and Zhang, X. (2022). Can informal environmental regulation promote industrial structure upgrading? Evidence from China. Appl. Econ. 54 (19), 2161–2180. doi:10.1080/00036846.2021.1985073
Chen, Y., Kumara, E. K., and Sivakumar, V. (2021). Investigation of Finance industry on risk awareness model and digital economic growth. Ann. Oper. Res. doi:10.1007/s10479-021-04287-7
Chen, Y., Yao, Z., and Zhong, K. (2022). Do environmental regulations of carbon emissions and air pollution foster green technology innovation: Evidence from China's prefecture-level cities. J. Clean. Prod. 350, 131537. doi:10.1016/j.jclepro.2022.131537
Cheng, Z., Li, L., and Liu, J. (2017). Environmental regulation and industrial structure upgrading——Based on dynamic spatial econometric analysis of Chinese cities' data. Forum Sci. Technol. China (02), 66–72. (in Chinese). doi:10.13580/j.cnki.fstc.2017.02.010
Cole, M. A., Elliott, R. J. R., and Shimamoto, K. (2005). Industrial characteristics, environmental regulations and air pollution: An analysis of the UK manufacturing sector. J. Environ. Econ. Manag. 50 (1), 121–143. doi:10.1016/j.jeem.2004.08.001
Deng, L., and Zhao, Y. (2022). Investment lag, financially constraints and company value—evidence from China. Emerg. Mark. Finance Trade 58, 3034–3047. doi:10.1080/1540496X.2021.2025047
Dong, B., Xu, Y., and Fan, X. (2020). How to achieve a win-win situation between economic growth and carbon emission reduction: Empirical evidence from the perspective of industrial structure upgrading. Environ. Sci. Pollut. Res. 27, 43829–43844. doi:10.1007/s11356-020-09883-x
Du, K., Cheng, Y., and Yao, X. (2021). Environmental regulation, green technology innovation, and industrial structure upgrading: The road to the green transformation of Chinese cities. Energy Econ. 98, 105247. doi:10.1016/j.eneco.2021.105247
Endrikat, J., Guenther, E., and Hoppe, H. (2014). Making sense of conflicting empirical findings: A meta-analytic review of the relationship between corporate environmental and financial performance. Eur. Manag. J. 32 (5), 735–751. doi:10.1016/j.emj.2013.12.004
Fu, H., Mao, Y., and Song, L. (2013). Empirical analysis on the effect of innovation on advancement of industrial structure process——based on the panel datas of provinces from 2000 to 2011. China Ind. Econ. (09), 56–68. (in Chinese). doi:10.19581/j.cnki.ciejournal.2013.09.006
Gan, C., Zheng, R., and Yu, D. (2011). An empirical study on the effect of industrial structure on economic growth and fluctuations in China. Econ. Res. J. 46 (05), 4–16. (in Chinese).
Gao, K., and Yuan, Y. (2022). Government intervention, spillover effect and urban innovation performance: Empirical evidence from national innovative city pilot policy in China. Technol. Soc. 70, 102035. doi:10.1016/j.techsoc.2022.102035
Gao, S., Wei, Y., and Chen, W. (2012). Effects of environmental regulation on the structure optimization and performance of manufacturing industry in Wuxi City. J. Lake Sci. 24 (01), 17–26. (in Chinese). doi:10.18307/2012.0103
Gao, X., He, Z., and Zhang, F. (2022). How to improve region-level green technology innovation by government subsidies and environmental regulations? ——a research on co-movement effect from configuration perspective. R&D Manag., 1–11. (in Chinese). doi:10.13581/j.cnki.rdm.20211499
Gray, W. B., and Shadbegian, R. J. (1995). Pollution abatement costs, regulation, and plant-level productivity. Natl. Bureau Econ. Res., 123–127. doi:10.4324/9781315188010-13
Guo, R., and Yuan, Y. (2020). Environmental regulation, R&D subsidy and industrial structure upgrading. Stud. Sci. Sci. 38 (12), 2140–2149. (in Chinese).
Han, Y., and Ma, L. (2020). Research on the relationship between the change of industrial structural and economic growth under the stage of high-quality development in China: Based on the logical frame of structure-efficiency-speed. Res. Econ. Manag. 41 (12), 28–40. (in Chinese). doi:10.16192/j.cnki.1003-2053.2020.12.004
Hao, S., and Zhang, Y. (2016). Research on the impact of environmental regulation on economic agglomeration - based on the perspective of new economic geography. Soft Sci. (04), 27–30. doi:10.13956/j.ss.1001-8409.2016.04.06
He, Y., and Zeng, Y. (2013). How open & indigenous innovation affects industries international competitiveness: An empirical study on Chinese manufacturing industries based on the panel data from the year 2000 to 2010. Sci. Sci. Manag. S.& Trans. 34 (03), 13–22. (in Chinese). doi:10.13502/j.cnki.issn1000-7636.2020.12.003
Hsieh, C., and Klenow, P. J. (2009). Misallocation and manufacturing TFP in China and India. Q. J. Econ. 124 (4), 1403–1448. doi:10.1162/qjec.2009.124.4.1403
Hussain, I., Rehman, A., and Işık, C. (2022). Using an asymmetrical technique to assess the impacts of CO2 emissions on agricultural fruits in Pakistan. Environ. Sci. Pollut. Res. 29 (13), 19378–19389. doi:10.1007/s11356-021-16835-6
Işık, C., Ongan, S., Bulut, U., Karakaya, S., Irfan, M., Alvarado, R., et al. (2022). Reinvestigating the Environmental Kuznets Curve (EKC) hypothesis by a composite model constructed on the Armey curve hypothesis with government spending for the US States. Environ. Sci. Pollut. Res. 29 (11), 16472–16483. doi:10.1007/s11356-021-16720-2
Jaffe, A. B., and Palmer, K. (1997). Environmental regulation and innovation: A panel data study. Rev. Econ. Stat. 79 (4), 610–619. doi:10.1162/003465397557196
Ji, L. (2018). Impact of technological innovation on upgrade of China's manufacturing industry: Based on adjustment of financing constraints. J. Technol. Econ. 37 (11), 30–36. (in Chinese).
Jiang, M., Luo, S., and Zhou, G. (2020). Financial development, OFDI spillovers and upgrading of industrial structure. Technol. Forecast. Soc. Change 155, 119974. doi:10.1016/j.techfore.2020.119974
Jiang, S., and Ji, M. (2019). Analysis of the influence of technological innovation on spatial transmission path of the industrial structure. Sci. Technol. Manag. Res. 39 (13), 15–23. (in Chinese).
Kheder, S. B., and Zugravu, N. (2012). Environmental regulation and French firms location abroad: An economic geography model in an international comparative study. Ecol. Econ. 77, 48–61. doi:10.1016/j.ecolecon.2011.10.005
Lanoie, P., Patry, M., and Lajeunesse, R. (2008). Environmental regulation and productivity: Testing the porter hypothesis. J. Product. Anal. 30 (2), 121–128. (in Chinese). doi:10.1007/s11123-008-0108-4
Li, H., and Zou, Q. (2018). Environmental regulation, resource endowments and urban industrial transformation: Comparative analysis of resource-based cities and non-resource-based cities. Econ. Res. J. 53 (11), 182–198.
Li, K., and Lin, B. (2017). Economic growth model, structural transformation, and green productivity in China. Appl. Energy 187, 489–500. doi:10.1016/j.apenergy.2016.11.075
Li, T., and Dong, H. (2018). Empirical analysis on the relationship between technological innovation and industrial structure based on LSTAR model. China Soft Sci. (06), 151–162. (in Chinese).
Li, Z., and Yang, S. (2015). Innovation input, industrial structure and economic growth. Seek. Truth 42 (04), 61–67. (in Chinese).
Liao, H., Yang, L., Ma, H., and Zheng, J. (2020). Technology import, secondary innovation, and industrial structure optimization: A potential innovation strategy for China. Pac. Econ. Rev. 25 (2), 145–160. doi:10.1111/1468-0106.12326
Liu, S., Liu, Y., Wang, C., and Dang, X. (2022). The distribution characteristics and human health risks of high- fluorine groundwater in coastal plain: A case study in southern laizhou bay, China. Front. Environ. Sci. 10. doi:10.3389/fenvs.2022.901637
Liu, X., Dong, J., Ji, K., Li, X., and Xu, S. (2022). Investigating the ‘short pain’ and ‘long gain’ effect of environmental regulation on financial performance: Evidence from Chinese listed polluting firms. Sustainability 14 (4), 2412. doi:10.3390/su14042412
Liu, Z., Wang, F., Han, M., Tian, M., Deng, L., Meng, N., et al. (2021). Bone marrow-derived mesenchymal stem cells inhibit CD8+ T cell immune responses via PD-1/PD-L1 pathway in multiple myeloma.. Clin. Exp. Immunol. 38 (04), 53–62. (in Chinese). doi:10.1111/cei.13594
Long, X., Chen, Y., Du, J., Oh, K., Han, I., and Yan, J. (2017). The effect of environmental innovation behavior on economic and environmental performance of 182 Chinese firms. J. Clean. Prod. 166, 1274–1282. doi:10.1016/j.jclepro.2017.08.070
Lu, J. (2007). International environmental regulations and the forced upgrade of industry technologies. J. Int. Trade (07), 71–76. (in Chinese).
Lu, Q. (2022). Research on the impact of environmental regulation on green technology innovation: Based on the regulatory role of the digital economy. Value Eng. 41 (19), 1–3. (in Chinese).
Luo, Z., and Qi, B. (2021). The effects of environmental regulation on industrial transfer and upgradingu and the banking synergistic development: Evidence from water pollution control in the Yangtze river basin. Econ. Res. J. 56 (02), 174–189. (in Chinese).
Ma, Q., and Yuan, D. (2004). Technological innovation and the evolution of industrial structure. Soc. Sci. J. (02), 27–31. (in Chinese).
Martić, M., and Savić, G. (2001). An application of DEA for comparative analysis and ranking of regions in Serbia with regards to social-economic development. Eur. J. Operational Res. 132 (2), 343–356. doi:10.1016/s0377-2217(00)00156-9
Mazzucato, M. (2016). From market fixing to market-creating: A new framework for innovation policy. Industry Innovation 23 (2), 140–156. doi:10.1080/13662716.2016.1146124
Miao, R., Liu, Y., Wu, L., Wang, D., Liu, Y., Miao, Y., et al. (2022). Effects of long-term grazing exclusion on plant and soil properties vary with position in dune systems in the Horqin Sandy Land. CATENA 209, 105860. doi:10.1016/j.catena.2021.105860
Mieg, H. A. (2012). Sustainability and innovation in urban development: Concept and case. Sust. Dev. 20 (4), 251–263. doi:10.1002/sd.471
Ngai, L. R., and Pissarides, C. A. (2007). Structural change in a multisector model of growth. Am. Econ. Rev. 97 (1), 429–443. (in Chinese). doi:10.1257/000282807780323460
Nilssen, M. (2019). To the smart city and beyond? Developing a typology of smart urban innovation. Technol. Forecast. Soc. Change 142, 98–104. doi:10.1016/j.techfore.2018.07.060
Pan, D., and Chen, H. (2021). Border pollution reduction in China: The role of livestock environmental regulations. China Econ. Rev. 69, 101681. doi:10.1016/j.chieco.2021.101681
Pan, H., Yang, T., Jin, Y., Dall'Erba, S., and Hewings, G. (2020). Understanding heterogeneous spatial production externalities as a missing link between land-use planning and urban economic futures. Reg. Stud. 55 (1), 90–100. doi:10.1080/00343404.2019.1701186
Pei, X., Jiang, A., Ye, Y., and Wang, F. (2019). Private investment, environmental regulation and green technology innovation -- Analysis of spatial Dubin model based on 11 provinces and cities in the Changjiang River economic belt. Sci. Technol. Prog. Policy 36 (08), 44–51. (in Chinese).
Pickles, J. (2006). Trade liberalization, industrial upgrading, and regionalization in the global clothing industry. Environ. Plan. A 38 (12), 2201–2206. doi:10.1068/a3812ed
Qiao, S., Zhao, D., Guo, Z., and Tao, Z. (2022). Factor price distortions, environmental regulation and innovation efficiency: An empirical study on China's power enterprises. Energy Policy 164, 112887. doi:10.1016/j.enpol.2022.112887
Qu, R., and Wang, Y. (2002). Environmental protection: An important means to enhance international competitiveness. Commer. Res. (20), 84–85. (in Chinese). doi:10.13902/j.cnki.syyj.2002.20.036
Quan, Q., Liang, W., Yan, D., and Lei, J. (2022). Influences of joint action of natural and social factors on atmospheric process of hydrological cycle in Inner Mongolia, China. Urban Clim. 41, 101043. doi:10.1016/j.uclim.2021.101043
Ren, X. S., Liu, Y. J., and Zhao, G. H. (2020). Impact of economic agglomeration on carbon emission intensity and transmission mechanism. China Population-Resources Environ. (04), 95–106.
Sarwar, S., Shahzad, U., Chang, D., and Tang, B. (2019). Economic and non-economic sector reforms in carbon mitigation: Empirical evidence from Chinese provinces. Struct. Change Econ. Dyn. 49, 146–154. (in Chinese). doi:10.1016/j.strueco.2019.01.003
Shadbegian, R. J., and Gray, W. B. (2004). Pollution abatement expenditures and plant-level productivity: A production function approach. Ecol. Econ. 54 (2), 196–208. doi:10.1016/j.ecolecon.2004.12.029
Sheng, H., Zhang, Y., Wang, W., Shan, Z., Fang, Y., Lyu, W., et al. (2022). High confident evaluation for smart city services. Front. Environ. Sci. 10. doi:10.3389/fenvs.2022.950055
Song, M., Peng, L., Shang, Y., and Zhao, X. (2022a). Green technology progress and total factor productivity of resource-based enterprises: A perspective of technical compensation of environmental regulation. Technol. Forecast. Soc. Change 174, 121276. doi:10.1016/j.techfore.2021.121276
Song, M., Peng, L., Shang, Y., and Zhao, X. (2022b). Green technology progress and total factor productivity of resource-based enterprises: A perspective of technical compensation of environmental regulation. Technol. Forecast. Soc. Change 174, 121276. doi:10.1016/j.techfore.2021.121276
Su, J., Su, K., and Wang, S. (2021). Does the digital economy promote industrial structural upgrading?—a test of mediating effects based on heterogeneous technological innovation. Sustainability 13 (18), 10105. doi:10.3390/su131810105
Sun, Y., Mu, H., Fan, H., Hou, X., and Zhang, Z. (2020). Research on heterogeneous linkage effects of environmental regulations on the upgrading of industrial structures. J. Industrial Technol. Econ. 39 (04), 89–95. (in Chinese).
Tao, C. Q., Xu, M., and Yu, J. J. (2020). Government innovation investment, interregional linkages and industrial structure upgrading -- an empirical analysis of 283 cities in China. Statistics Inf. Forum 35 (07), 89–100. (in Chinese).
Varum, C. A., Cibrão, B., Morgado, A., and Costa, J. (2009). R&D, structural change and productivity: The role of high and medium-high technology industries. Econ. Apl. 13 (4), 399–424. doi:10.1590/s1413-80502009000400003
Wang, H., Cui, H., and Zhao, Q. (2020). Effect of green technology innovation on green total factor productivity in China: Evidence from spatial durbin model analysis. J. Clean. Prod. 288, 125624. doi:10.1016/j.jclepro.2020.125624
Wang, L., Wang, Z., and Ma, Y. (2021). Heterogeneous environmental regulation and industrial structure upgrading: Evidence from China. Environ. Sci. Pollut. Res. 29 (9), 13369–13385. doi:10.1007/s11356-021-16591-7
Wang, M., Li, Y., Li, J., and Wang, Z. (2021). Green process innovation, green product innovation and its economic performance improvement paths: A survey and structural model. J. Environ. Manag. 297, 113282. doi:10.1016/j.jenvman.2021.113282
Wang, X., and Wang, Q. (2021). Research on the impact of green finance on the upgrading of China's regional industrial structure from the perspective of sustainable development. Resour. Policy 74, 102436. doi:10.1016/j.resourpol.2021.102436
Wei, L., Zhang, H., and Zhang, Y. (2021). The impact of technological innovation and industrial structure upgrading on new urbanization: An empirical analysis based on panel quantile regression. Mod. Econ. 12 (10), 1463–1485. doi:10.4236/me.2021.1210075
Wu, M., Xu, C., and Tang, L. (2019). Environmental regulation and manufacturing's upgrading ——Mechanism and empirical test. Reform Econ. Syst. (01), 135–139. (in Chinese).
Wu, Y., and Zhu, W. (2021). The role of CSR engagement in customer-company identification and behavioral intention during the COVID-19 pandemic. Front. Psychol. 12, 721410. doi:10.3389/fpsyg.2021.721410
Xue, Y. (2016). The industrial structure effect of environmental regulation: Theoretical and empirical analysis. J. Statistics Inf. 31 (08), 39–46. (in Chinese).
Yan, Z., Zou, B., Du, K., and Li, K. (2020). Do renewable energy technology innovations promote China's green productivity growth? Fresh evidence from partially linear functional-coefficient models. Energy Econ. 90, 104842. doi:10.1016/j.eneco.2020.104842
Yao, L., Li, J., and Li, J. (2020). Urban innovation and intercity patent collaboration: A network analysis of China’s national innovation system. Technol. Forecast. Soc. Change 160, 120185. doi:10.1016/j.techfore.2020.120185
Yao, Y., Pan, H., Cui, X., and Wang, Z. (2022). Do compact cities have higher efficiencies of agglomeration economies? A dynamic panel model with compactness indicators. Land Use Policy 115, 106005. doi:10.1016/j.landusepol.2022.106005
Ye, Q., Zeng, G., Dai, S., and Wang, F. (2018). Research on the effects of different policy tools on China's emissions reduction innovation: Based on the panel data of 285 prefecture-level municipalities. China Popul. Resour. Environ. 28 (02), 115–122. (in Chinese).
Yin, K., Zhang, R., Jin, X., and Yu, L. (2022). Research and optimization of the coupling and coordination of environmental regulation, Technological Innovation, and Green Development. Sustainability 14 (1), 501. doi:10.3390/su14010501
Yuan, D. (2022). Impact of environmental protection tax on green technology innovation ability of enterprises: Based on empirical analysis of listed companies in Shanghai and Shenzhen. J. Yibin Univ., 1–10. (in Chinese).
Zhang, D., Rong, Z., and Ji, Q. (2019). Green innovation and firm performance: Evidence from listed companies in China. Resour. Conserv. Recycl. 144, 48–55. doi:10.1016/j.resconrec.2019.01.023
Zhang, T., Li, Z., and Cui, J. (2022). Green finance, environmental regulation and industrial structure optimization. J. Shanxi Univ. Finance Econ. 44 (06), 84–98. (in Chinese). doi:10.3390/su14010501
Zhang, Y., Sun, J., Yang, Z., and Wang, Y. (2020). Critical success factors of green innovation: Technology, organization and environment readiness. J. Clean. Prod. 264, 121701. doi:10.1016/j.jclepro.2020.121701
Zhao, S., Jiang, Y., and Wang, S. (2019). Innovation stages, knowledge spillover, and green economy development: Moderating role of absorptive capacity and environmental regulation. Environ. Sci. Pollut. Res. 26 (24), 25312–25325. doi:10.1007/s11356-019-05777-9
Zhong, Z., Chen, Z., and He, L. (2022). Technological innovation, industrial structural change and carbon emission transferring via trade-------An agent-based modeling approach. Technovation 110, 102350. doi:10.1016/j.technovation.2021.102350
Zhou, K., Zhang, B., and Gu, Z. (2016). The impact of scientific and technological innovation on industrial upgrading——Empirical analysis based on provincial panel data of China. J. Industrial Technol. Econ. 35 (08), 85–92. (in Chinese).
Zhou, K. Z., and Li, C. B. (2012). How knowledge affects radical innovation: Knowledge base, market knowledge acquisition, and internal knowledge sharing. Strateg. Manag. J. 33 (9), 1090–1102. doi:10.1002/smj.1959
Zhou, Y. (2018). Human capital, institutional quality and industrial upgrading: Global insights from industrial data. Econ. Change Restruct. 51 (1), 1–27. doi:10.1007/s10644-016-9194-x
Keywords: government green innovation preference, environmental regulation, industrial structure upgrade, threshold effect, innovation, China
Citation: Khattak SI, Khan AM, Khan MK, Li C, Liu J and Pi Z (2022) Do regional government green innovation preferences promote industrial structure upgradation in China? Econometric assessment based on the environmental regulation threshold effect model. Front. Environ. Sci. 10:995990. doi: 10.3389/fenvs.2022.995990
Received: 16 July 2022; Accepted: 16 August 2022;
Published: 15 September 2022.
Edited by:
Cem Işık, Anadolu University, TurkeyReviewed by:
Nudrat Fatima, Beijing Technology and Business University, ChinaCopyright © 2022 Khattak, Khan, Khan, Li, Liu and Pi. This is an open-access article distributed under the terms of the Creative Commons Attribution License (CC BY). The use, distribution or reproduction in other forums is permitted, provided the original author(s) and the copyright owner(s) are credited and that the original publication in this journal is cited, in accordance with accepted academic practice. No use, distribution or reproduction is permitted which does not comply with these terms.
*Correspondence: Muhammad Kamran Khan, bWtraGFuLmJ1aWNAYmFocmlhLmVkdS5waw==
Disclaimer: All claims expressed in this article are solely those of the authors and do not necessarily represent those of their affiliated organizations, or those of the publisher, the editors and the reviewers. Any product that may be evaluated in this article or claim that may be made by its manufacturer is not guaranteed or endorsed by the publisher.
Research integrity at Frontiers
Learn more about the work of our research integrity team to safeguard the quality of each article we publish.