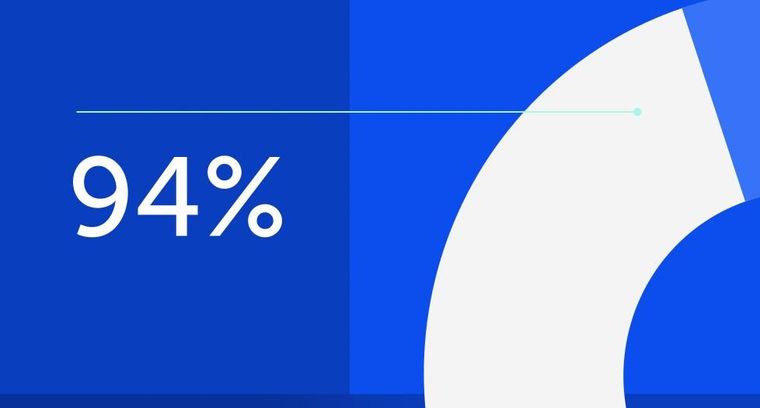
94% of researchers rate our articles as excellent or good
Learn more about the work of our research integrity team to safeguard the quality of each article we publish.
Find out more
ORIGINAL RESEARCH article
Front. Environ. Sci., 23 September 2022
Sec. Environmental Economics and Management
Volume 10 - 2022 | https://doi.org/10.3389/fenvs.2022.995984
This article is part of the Research TopicEconomic Development, Social Consequences, and Technological Innovation Under Climate Change COVID-19 Pandemic ConditionsView all 48 articles
Local government innovation support (LGIS) is an exogenous pulling force for high-quality economic development. Accurately identifying the mechanism by which LGIS affects green development remains practically and theoretically significant for building a long-term framework for driving green development. Based on provincial panel data (2010–2019), this paper uses the Super-SBM model to measure the green development efficiency (GDE) of different provinces in China. A dynamic panel GMM model is constructed to evaluate the significant relationship between LGIS and GDE and to explore the potential impact mechanism of other key factors such as fixed asset investment (FAI), environmental regulation (ER), industrial structure (IS), and foreign direct investment (FDI) on GDE. The estimation showed that LGIS had a positive impact on GDE. The data analysis indicated that GDE in the lag period significantly affected the GDE in the current period. More so, as per the results, the promoting effect of GDE in the second lag period gradually weakened due to the decline of LGIS and ER restrictions. The current model also revealed that FAI and ER inhibited the GDE progress, while IS and FAI had improved the state of GDE in the region. The research findings imply that China should further increase the government innovation expenditure, FDI utilization, and IS upgrade to promote high-quality economic development.
With the general improvement of people’s environmental awareness, more and more countries have begun to recognize the importance of ecological and environmental protection. It has become a common agenda of all countries to relieve the pressure on resources and the environment to realize sustainable economic development (Donald and Gordon, 2016). Recently, the local governments in China have been focusing on strategies to address environmental issues to improve green and sustainable development to an unprecedented level. In 2018, the Chinese government called for speeding up the formation of a green development model, reducing pollutant emissions from the source, and taking green development as a fundamental strategy to solve pollution (air, water, and land) and enhance the quality of the ecological environment. In 2019, the Chinese Government Work Report indicated that green development is an inevitable requirement for creating a modern economic system (Chen H et al., 2021; Chen and Sivakumar, 2021; Miao et al., 2022). At the same time, the Chinese government has stressed at many important domestic meetings to adhere to the new development philosophy and achieve sustained and sound economic development based on significantly improving quality and efficiency (Pan and Chen, 2021; Qiu et al., 2022a; Qin et al., 2022). The spirit of green development mandates coordination and unification of economic growth and environmental protection (Jari et al., 2018; Zhang et al., 2018; Li et al., 2019) to manage economic growth while addressing ecological constraints by improving energy utilization, reducing pollution emissions, promoting industrial transformation and upgrading (Luo et al., 2021), promoting economic growth, and improving environmental quality, is an important strategic choice to encourage high-quality development of economy in China (Na et al., 2020).
Green development has been continuously promoted in China, but many believe that the current state of overall green development still needs considerable improvements (e.g., Yang and Wen, 2017). Experts argue that regional green development can play a vital role in the improvement of GDE (Guo and Zhang, 2020; Qiu et al., 2020). Following the concept of economic development efficiency, resource input and environmental pollution are integral factors of GDE in China and are used as key indicators to measure regional green development (Li and Jing, 2019). With the decentralization of fiscal power in China, local governments have become the main players steering and implementing the central green development policy, promoting sustainable economic development, and institutionalizing regional green development policies (Li et al., 2020). They are responsible for guiding, regulating, and monitoring the efficiency of regional green development in their respective administrative units (Li, 2021) in the following ways: 1) effectively alleviate the shortage of R&D funds for local enterprises, universities, and scientific research institutions (Gao et al., 2022); 2) promote regional green technology innovation to provide financial support (Yang et al., 2022); 3) issue green development-related policies, e.g., environmental regulations (Guo and Chen, 2021; Hu et al., 2022); 4) formalize and integrate the environmental information disclosure system (Yang and Zhao, 2018) and green credit (Pei et al., 2018); 5) effectively standardize corporate behavior and provide policy support for their green technology innovation; 6) align green support measures to suit local conditions, e.g., the implementation of trade opening (Qi et al., 2022) and market-oriented reforms; 7) provide a good innovation environment for enterprises in the region to implement green technology innovation; 8) promote the construction of innovative cities (Wang H et al., 2022), low-carbon cities (Lan, 2021), smart cities (Du et al., 2020; Zhang and Zhong, 2022); 9) construct pilot green city areas for broader implementation. Despite the critical role of LGIS in facilitating regional and national GDE in China (Ding et al., 2022), most previous studies have predominantly restricted to antecedents or consequences of regional green technology innovation or specific policies and measures. However, the overall impact of government innovation on the regional green development efficiency in China remains largely unexplored.
In response to the above knowledge void, the main aim of this work is to explain the continuous promotion of government innovation construction and the continuous implementation of green development practices (Qiu et al., 2022b; Sheng et al., 2022). Firstly, the study systematically analyzes the influence mechanism of regional government innovation subsidies on GDE, as asserted in earlier studies (e.g., Jiang et al., 2021). From the perspective of government innovation governance and support, it generally evaluates the effect of LGIS and other key influencing factors (e.g., FAI, ER, IS, and FDI) on GDE improvement. Secondly, the paper uses two novel and robust estimators in the study: 1) the Super-SBM model to measure the efficiency of green development comprehensively; 2) the dynamic GMM model as the main analysis framework to empirically analyze the mechanism of government innovation support to improve the GDE. Third, taking into account the current situation of regional green development, the article also analyzes the heterogeneity of different regions and compares the differences between regions.
The following parts of this work are divided as follows. An overview of the study area and the academic literature on the interactions among the main study variables are given in Section 2. The details of the study methodology are discussed in Section 3. Section 4 provides empirical analysis based on the outcomes of various methods used in the study. The last section, Section 5, concludes the main study findings, offers policy implications, highlights limitations, and puts possible future directions forwards.
Government, market, and enterprise are the key components of a modern market economy system. For social transformation in China, government innovation can act as an essential tool for improving the ability, systems, and allocation of resources in the local administration. Local governments have adjusted the economic and administrative power of market allocation of resources, including financial subsidies, tax incentives, business license issuance, and investment restrictions (Tian et al., 2021a; Tian et al., 2021b; Tan et al., 2022). Of these expenditures, government financial science and technology expenditure is an important instrument for the government to participate in regional innovation activities. Previous literature on government innovation support is mainly divided into the following streams. The first stream, the connotation of government innovation, attempts to define the scope and objective of government innovation. For instance, He (2011) argued that the goal of government innovation is to establish a service-oriented government with political, economic, social, and cultural coordination and sustainable development, starting from the theory, system, personnel, and operation of government innovation. As a criterion for winning projects of the “Local Government Innovation Award,” the content of government innovation can be divided into four categories, i.e., political reforms, administrative reforms, public services, and social management. The content of government innovation can be roughly divided into two levels (Yan et al., 2021; Wang Q et al., 2022). One is the internal management of the government organization systems, whereas the other relates to the government’s management and services to society. The second stream of literature, the driving force and influencing factors of government innovation, unwraps the determinants of government innovation (Chen, 2015; Yu and Huang, 2017). Researchers have found that moderate decentralization ability (Wu and Wu, 2018) and performance appraisal system (Huang, 2017) are among the important factors promoting government innovation. The third stream, the influence mechanism of government innovation diffusion, explores the precise framework through which government innovation occurs in a certain region (e.g., Guo and Zhang, 2020; Yuan et al., 2022). The diffusion of government innovation refers to the process through which government innovation policies or projects are spread in a certain social system through certain channels. For example, Zhang et al. (2015) explained the driving force of government innovation diffusion from the perspective of neo-institutionalism theory and found that the driving force of government innovation diffusion has the characteristics of stages. Liu et al. (2021) studied the multi-factor combination path of the comprehensive promotion of LGIS.
Public policy experts argue that government-relevant policy formulation and resource allocation significantly impact the operation of enterprises (Xu, 2014; Han and Gao, 2018), encouraging enterprises to carry out innovative activities (Long et al., 2012). As an exogenous driving force to promote the high-quality development of the national economy, government innovation support is the management and service of the government to the society, and it has a basic, guiding, and stimulating effect on innovative economic activities. Through scientific and technological innovation subsidies, the government urges social entities to increase scientific and technological research investment and development, indirectly reduces the cost of enterprise research and development or makes up for the gap in research and development funds, eases the financial constraints of innovation activities, and encourages innovation activities in the region. Beyond that, government innovation can promote independent scientific research institutions, universities, and related enterprises to jointly conquer core technologies, strengthen industry-university-research cooperation, and promote the transition from technological imitation to cutting-edge technological innovation. Enterprises are further encouraged to conduct green (low-carbon/zero-carbon) technologies focused on research and development by reducing taxes and fees to promote green economy development. The key to improving the quality of green development is to develop green development efficiency (Yu et al., 2016). For China, green development efficiency is a critical indicator to measure the economy entering a stage of high-quality development in the new age.
Green development and green efficiency literature offer three main research directions: the concept of green development and green development efficiency. For instance, Pearce et al. (1996) define a green economy as “an economic growth model that will not lead to ecological crisis and social fragmentation.” As per Hu and Zhou (2014), the green development concept needs three key dimensions: function definition, mechanism analysis, and development strategy. The author added that green development emphasizes the relationship between economic, social, and natural systems. Liu (2017) divided the concept of green development into five dimensions: economic development, political construction, ecological environment, social development, cultural value. The author pointed out that green development is innovation-driven, high-level, sustainable, and improves people’s livelihood, harmonious and upward development. Secondly, the second literature theme is focused on the evaluation and evolution green development efficiency (in time and space). In this context, economists have constructed an evaluation index system for green development and green development efficiency based on the SBM model and its extensions to measure the green development efficiency of different areas (Yue and Xue, 2020; Shi and Lan, 2021), provinces, regions (Xu and Ouyang, 2022), economic belts (Chen J et al., 2021; Xiang et al., 2021), and urban agglomerations (Chen and Wu, 2021; Weng et al., 2022; Zhang et al., 2022). Based on the input-output theory, authors often incorporate resource consumption, environmental pollution, ecological benefits, and social benefits into the Super-SBM model to analyze the efficiency of the input-output allocation of economic, social, and environmental complex ecosystem elements, crucial factors of green economic efficiency. Among other methods, researchers usually predict green development efficiency through the Super-SBM model, directional distance function model, total factor productivity model or vector autoregressive model (VAR), and gray support vector-machine-regression model GM(1,1) (Cao, 2011; He et al., 2020) from a regional macro perspective to predict the green development efficiency of national inter-provincial, urban or rural, urban agglomerations. Chen et al. (2022) used the Super-SBM model and Malmquist index to measure the green development efficiency of the Chengdu-Chongqing economic circle from 2007 to 2019 based on dual perspectives. The authors analyzed the spatial-temporal evolution characteristics and influencing factors and then predicted green development efficiency using Went’s linear and seasonal exponential smoothing method.
Considering the stream of research on the influence mechanism and ascension path of green development efficiency based on the Tobit regression, Bootstrap truncation regression, and spatial autoregression models indicate that the level of economic development, technological innovation, and environmental regulations (Ma and Jin, 2022), green finance (Zhu et al., 2022), fiscal decentralization, ecological civilization pilot zones (Fan et al., 2022), and low-carbon cities affect the efficiency of green development, and specific progression paths (Wang et al., 2014; Song et al., 2018; Zhao et al., 2018; Wen, 2021). Guo et al. (2022) and others used the SBM-undesirable model to construct an input-output indicator system for green development efficiency in the Yellow River Basin. They conducted a spatial statistical analysis of the green development efficiency of the Yellow River Basin and its temporal and spatial pattern characteristics. Through different models (SE-SBM model, GML index and its decomposition index, and Tobit regression model), the above researchers have concluded that there is still room for improvement in green development efficiency in the sample area and the overall stage is characterized by a leap from low-level to high-level. The GML index revealed a fluctuating growth trend, where differences were found in the growth rate of local green development efficiency in China. The study further showed that urbanization and environmental policies promote regional green development, while industrial structure, degree of opening-up, and energy structure inhibit regional green development. Zirui (2021) used the DID model and the propensity matching score method to examine the influence mechanism of low-carbon pilot policies on regional GDE. The results demonstrated that the implementation of low-carbon pilot policies could significantly improve the level of regional GDE in Chinese provinces, and the effect of policies varies with different regions and cities.
Retrospectively, it is not difficult to find that government-led reforms and innovations, such as market-oriented reforms, low-carbon cities, ecological civilization pilot zones, green financial inclusion policies, and environmental information disclosure systems, play a significant effect on the regional green development efficiency. An important limitation of prior works resides in examining a specific policy or reform as the starting point and undermining the influence of government reform or innovation on the regional green development efficiency (as a whole). Therefore, in the case of unbalanced local government innovation levels and uncoordinated regional green development, it is indispensable to analyze the influence mechanism and improvement path of government innovation and its processes on green development efficiency from the national and regional perspectives. Exploring its impact on green development efficiency from the view of government governance innovation can enrich and supplement relevant research about the improvement path and impact mechanism of green development efficiency to a certain extent. The literature summary is shown in Table 1.
Keeping in view the mechanism of local government innovation support and green development efficiency, the innovation-driven development strategy has attracted significant attention from governments worldwide. Studies have shown that government innovation subsidies can effectively improve the efficiency of technological innovation, thereby promoting regional economic development (Jiang and Tan, 2020). Nevertheless, the existing research on local government innovation support mainly focuses on its relationship with economic development. From the economic development viewpoint, due to the externalities and high risks of science and technology, high investment, uncertainty, and other characteristics (Li and Yang, 2018), government science and technology funds can address the following issues: make up for the gap of corporate innovation funds; alleviate the difficulty of insufficient research and development funds; optimize the allocation of resources for scientific and technological innovation (Ye and Liu, 2018; Sung et al., 2022; Zhang and Ayele, 2022); improve economic development efficiency.
From a societal perspective, the essence of local government innovation support is to drive social development and maintain social stability. In the process of government innovation, society gradually grows and develops due to the availability of space for activities, rights protection, resource protection, and capacity protection (He, 2013). Ma et al. (2022) concluded that economic digitization reduced CO2 emissions in thirty Chinese provinces between 2006 and 2017. The negative impact of economic digitalization on CO2 emissions was more profound in provinces with higher R&D investment than those with lower R&D investments. From the perspective of environmental protection, increased government support for scientific and technological innovation can effectively improve energy-utilization technology and improve environmental quality to a certain extent (Qin and Yu, 2016; Zhou and Liu, 2021), and ultimately improve energy eco-efficiency (Wang et al., 2019; Song L et al., 2021). Among other researchers, Xie et al. (2018) observed a “U-shaped” nonlinear relationship between the LGIS and GDE. Only by increasing the support of scientific and technological innovation funds and making it transition to the right end of the “U-shaped” curve can the government facilitates the role of scientific and technological innovation in promoting ecological efficiency. The green development efficiency indicator emphasizes the symbiosis and interaction mechanism of the economic, social, and environmental systems (Liu et al., 2021). Therefore, through data envelopment analysis, this paper uses the Super-SBM model to build a green development-efficiency-index evaluation system, then uses the GMM model to inquire about the relationship between local government innovation support and green development efficiency.
Table 2 lists the summary of the data details.
The dependent variable, green development efficiency (GDE), was estimated using the data envelopment analysis method. The green development efficiency evaluation-index system was based on the input-output model: input indicators comprised physical capital, labor, and energy input; output indicators were divided into expected output and undesired output. The expected output was represented by the gross domestic product of each region, and the CO2 of each province was used to represent the unexpected output. Among them, the physical capital investment was measured by the capital stock, calculated by the fixed asset formation amount according to the perpetual inventory method, and the depreciation rate was taken as 9.6 percent, following Zhang et al. (2004). The GDP of each province took 2010 as the base period. The actual value was obtained after deflating according to the consumer price index of each region. Labor input represented the number of employees at the end of the year. The total energy consumption at the end of the past year was used to measure the energy input. The data on carbon dioxide emissions were compiled from the Carbon Emission Accounts and Datasets for Emerging Economies (CEADs) (Shan et al., 2016; Shan et al., 2018; Shan et al., 2020; Guan et al., 2021).
Kaoru (2001) proposed a slack-based efficiency evaluation model (Slack-Based Measure, SBM), introducing the amount of input and output slack into the objective function. When evaluating the efficiency of decision-making units in the traditional DEA method, there may be cases where the efficiency value of multiple decision-making units is 1, which cannot be further compared. Therefore, when there are multiple effective decision-making units, it cannot be considered for further comparison. Kaoru Tone Kaoru (2002) combines the advantages of the Super-DEA and SBM models. Unlike the traditional DEA model, the Super-SBM model can not only deal with the undesired output more properly, but also can make further comparisons in valid decision units. The Super-SBM model for this study was constructed as follows:
s.t.
In the above equation,
The independent variable, local government innovation support (LGIS), was developed by taking science and technology expenditure in local government financial expenditure as a proxy variable. Science and technology expenditure can promote the technological innovation of enterprises and other regional public departments to achieve the effect of energy saving and emission reduction. Innovative technology support and energy structure transformation improve the efficiency of green development and sustainable development in the region.
For control variables, the study accumulated important factors affecting the level of regional green development, including fixed asset investment (FAI), environmental regulation (ER), industrial structure (IS), and the level of foreign direct investment Utilization (FDI) (We and Hou, 2021). In China, FAI has created favorable conditions for regional infrastructure construction and economic development, thus impacting the efficiency of green development. The level of ER reflects the cost of regional pollution control. The more the government invests in pollution control and environmental protection, the more conducive it is to reducing undesired output (Wang and Zhang, 2018). This paper uses the investment in industrial pollution control as a proxy variable for the intensity of environmental regulation. The higher the proportion of secondary and tertiary industries in IS, the higher the concentration of industries in the region, which may have a crowding effect and impact the green development efficiency. The technology spillover brought by FDI not only helps local enterprises to improve the level of green production by imitating the innovation path but also stimulates the local enterprises for independent innovation, thereby contributing to the level of regional green development. It is measured by the amount of direct investment, in which the amount of foreign investment is deducted after conversion according to the annual exchange rate.
This paper used the logarithms of the dependent, independent, and control variables to control the nonlinear relationships and heteroscedasticity problems that may exist in the model test. The descriptive statistics of each variable are shown in Table 3.
Due to the long data time-span, this paper used the Augmented Dickey-Fuller (ADF) test to check the stationarity of all variables. The results in Table 4 (ADF test) supported the rejection of the null hypothesis, affirming no unit root and stationarity problems.
Panel data helps understand the dynamic behavior of the research object. As the individual unit is small and the time is long in the dynamic long panels, the deviation of the results of the dynamic panel estimation is small. A consistent estimate can be obtained by correcting the deviation. Provided that the number of object areas studied in this paper was greater than the length of time, the dynamic difference GMM model was selected. In this model, a consistent estimate is obtained in the following steps: 1) the first-order difference is used to eliminate the individual effects of the model; 2) appropriate instrument variables are found to eliminate the endogeneity of the model. Assuming that the impact of LGIS on GDE is inconsistent, it was conjectured that the GDE in the previous period would affect the current LGIS, which in turn would affect the current period GDE, as per prior concepts (He and An, 2019). The paper introduced the lag term of GDE as an instrumental variable. According to the provincial panel data structure, the specific model settings in this paper are as follows:
Where
The paper selected the dynamic panel system GMM model to test the nexus among the selected variables, GDE, LGIS, FAI, ER, IS, and FAI. In addition, since the model adds the lag term of GDE as an instrumental variable (L1 and L2), the GMM model can perform an over-identification test for instrumental variables (Arellano and Bond, 1991; David, 2009). As a consistent estimate, the premise of the system GMM estimate is that there is no autocorrelation in the disturbance term. This test is based on a two-step validation process: 1) the first-order autocorrelation for the first-order differences of the perturbation terms; 2) no second or higher-order autocorrelation for the differences in the perturbation terms (Arellano and Bond, 1991). The dynamic panel system GMM model results in Table 5 satisfied the pre-stated recommended criterion. Next, the Arellano–Bond test results showed that the perturbation term had first-order autocorrelation only, i.e., no second-order autocorrelation. The results of the Sargan test did not reject the null hypothesis, indicating that the instrumental variables were invalid, and the estimated results of the model were accurate.
As seen in Table 5, the effect of the GDE (previous period) on GDE (current period) was 0.72633. This result indicated that GDE improvements in the previous period contributed to enhancing the state of ER and technological innovation level, consequently making the impact of LGIS on economic development more evident. Also, this finding implies that continued LGIS, coupled with GDE in previous periods, has enabled improvements in current GDE in China, a view echoing previous research (Song L et al., 2021). The influence coefficient of the relationship between the second-lag GDE (L2) and the current-lag period GDE (L1) was 0.12511. This finding affirmed that the promotion effect of the former GDE period on the latter had begun to slow down. In line with Lin et al. (2022), this outcome supported that the GDE investment has declined recently. A possible explanation could be the volatility characteristics of ER restrictions and LGIS (Guo and Chen, 2021), which have caused slow progress in GDE and the gradual widening of the economic development gap across some regions. Under the current competitive atmosphere among local governments in China, the inferior performance of local governments lagging behind others in GDE in the second period will inhibit them from making GDE improvements in the current period. This finding contradicts preceding conclusions (He and An, 2019). As depicted in Table 5, LGIS significantly impacted GDE in local governments in China, as evidenced by the LGIS coefficient of 0.27494. Corroborating Hu and Wu’s (2019) assertions, this outcome supported that green and sustainable economic development and higher returns in China would require regional governments to continue to use technology expenditures to help upgrade and progress innovative technologies, thereby enhancing the regional GDE. Scientific and technological expenditures toward technological innovation by local governments in China have played a critical role in the technological transformation and upgrading of various industries (Gao and Li, 2021; Song M et al., 2021), decreasing the discharge of environmental pollutants in the region and improving GDE.
In Table 5, the data analysis showed the following results for the role of control variables. First, the coefficient of FAI affecting GDE was −0.02257, reflecting that economic development and construction in different regions have been achieved at the cost of environmental protection, undermining a focus on green and sustainable economic development. Zhang and Ma (2022) agree that although FAI creates an excellent external environment for improving enterprise productivity, excessive underutilized investments may generate a detrimental impact. Second, the estimates showed that ER had a significant and positive impact on GDE, as evident by the ER coefficient (0.01777). This outcome affirmed that the relevant environmental protection policies formulated by regional governments have led to considerable improvements in regional GDE, supporting the work of Lin et al. (2022). Sustainable technologies can enable enterprises to strengthen the economy, protect the environment, and upgrade the efficiency of green development. Besides, some scholars (cf. Huang and Wu, 2021; Qi et al., 2022) have established ER as a critical moderator in facilitating the positive impact of trade openness on GDE. Third, due to the “threshold effect” led by environmental regulations, the impact of trade liberalization on the efficiency of urban green development exhibits a “U-shaped” characteristic of first inhibition and then promotion. In the current context, the proportion of the secondary and tertiary industries in the regional GDP (IS) had a substantial promoting effect on regional GDE in Chinese regions, with a positive IS coefficient of 1.12644. Some experts (e.g., Zhu et al., 2019) argue that industrial structure optimization and coordinated expansion, industrial market force mechanism, and transformation of development methods are beneficial for constructing mechanisms for safe pollutant discharge. Zhao et al. (2016), based on findings on the influence of the two dimensions of industrial structure adjustment (advanced industrial structure and rationalized industrial structure), concluded that the interaction between human capital and industrial structure adjustment is regarded as an essential supporting condition for improving the GDE. The second and third industry synergistic agglomeration impact on green development has a practical promotion effect. Fourth, the results confirmed the positive effect of FDI on GDE (0.03755). This outcome suggested that increased utilization of foreign investment funds has contributed to local governments’ absorption of advanced green technologies. Also, it has helped in advancing production technologies and management models to promote the sustainable development and the improvement of GDE. Empirical studies have established that FDI can exert the spillover effect of technological innovation (Huang and Wu, 2021; Yue et al., 2022). Chinese regions opening up to the outside world can help them become the preferred investment destination for FDI, attract superior innovation infrastructure, resources, and high-quality human capital, and promote regional green development under the combined effect of FDI scale and quality.
Table 6 depicts the outputs of the dynamic panel difference GMM model adopted to test the robustness of the results. The robustness check indicated that the first-order lag term (0.72151) significantly improved the current GDE, benefiting from upsurges in LGIS, confirming prior findings (Gao and Li, 2021). With a decline in innovation input and the constraints of conditions, the GDE improvements in the second-order lag term (0.17087) began to weaken. Similarly, the fixed effects model showed that government innovation expenditures significantly contributed to the progress of GDE (0.27494). By comparing the results of the two estimators (i.e., the differential GMM model test and the system GMM model), it was concluded that no estimation bias existed, and the results of Table 5 were robust.
As there are disparities among different Chinese regions and the effect of various factors (e.g., geography, humanities, and policies), comparing provinces at different levels together may lead to biased test results, i.e., varying LGIS to GDE impact across the different regions in China (Hu and Wu, 2019). Thus, this paper divided China into three regions (East, Middle, and the West) based on province location to conduct a heterogeneity analysis. The results in Table 7 showed that the GDE of the second lag period in the East (0.63684) and Middle (1.08824) regions of China had significantly influenced the GDE of the current period. In other words, the geography, resources, and economic foundations of regions have played a significant role in assisting local governments in promoting green development in the East and Middle regions of China. Song M et al. (2021) also confirmed that the interaction among regional industrial upgrading, government innovation support, and energy eco-efficiency has nonlinear characteristics, even though government innovation support could promote the optimization and upgrading of regional industrial structure. The authors stated that energy eco-efficiency has a pulling effect on government innovation support in China. In contrast, the current analysis revealed that the GDE in the first period (L1 = 0.84392) in the West region was relatively weak in promoting GDE compared to the East and the Middle region, reflecting a more pronounced gap across regions. Yang et al. (2022) reported that capital mismatch had inhibited the further improvement of GDE in the Middle and East regions of China, while the West regions demonstrated weak progress. The results of the sub-regional tests indicated that the lagged first-order GDE in the East, Middle, and West regions have contributed to the current period.
Finally, the mean values of LGIS expenditure and GDE from 2010–2019 were taken as the sample to present the spatial distribution pattern of LGIS and GDE. The change characteristics were analyzed by combining ArcGIS mapping software. The results are shown in Figure 1. To summarise, government innovation expenditure shows an obvious distribution pattern in the East, middle, and West regions, moving from the Eastern coastal region to the interior. Benefiting from its location and solid economic foundation, the East region appears ahead of the inland region in government innovation expenditure, in which the Yangtze River Delta region has formed a clustering phenomenon. The distribution pattern of GDE resonates with the level of LGIS expenditure.
This study initially attempted to explain the interaction between LGIS, GDE, and GDE, along with FAI, ER, IS, and FAI as control variables. The Super-SBM model was used to measure the provincial-level regional GDE in China, including factors such as energy consumption, capital, labor input, and undesired output. The main techniques included the dynamic panel system GMM model (empirical analysis), the difference GMM model (robustness check and heterogeneity analysis), and the ArcGIS mapping software for mapping LGIS and GDE patterns across the different regions in China. First, the analysis showed that LGIS had improved the efficiency of regional green development across the East, Middle, and West regions of China. The analysis confirmed that innovative technologies application (i.e., increasing science and technology expenditure) had reduced undesired outputs, thereby improving the GDE in the regions. Unlike the first-order lag period, the positive effect of LGIS on GDE was significantly low in the second-order lag period due to the instability of local government science and technology spending and policy formulation. The current findings highlight that local governments are more inclined to rapid economic development rather than improving the quality of economic development and environmental protection.
Second, the current results offer a few possible explanations for why FAI had restricted the further improvement of GDE. For instance, local governments have increased the emission of pollutants in activities, including infrastructure construction, road renovations, and large-scale investments, consequently failing to GDE. Due to the “crowding out” effect, the local governments have supported and encouraged enterprises to invest more in energy conservation, emission reduction, and environmental protection, but inconsistent support has prompted short-term regional GDE. Enterprises cannot continue to invest more money to achieve the effect of energy conservation and emission reduction, which could inhibit regional GDE in the long run term. As factors, including ER, IS, and FDI, play a crucial role in the green development of different regions, local governments in China should pay more attention to these aspects and promote sustainable and healthy regional economic development by optimizing the policy system and enhancing institutional construction. Third, the data indicated that the effects of LGIS on GDE differ across different regions, i.e., LGIS has generated superior GDE outcomes in the economically developed East and Middle regions of China, but its effect in the economically deprived West regions is insignificant. The regional distribution pattern of LGIS level and GDE progress in China’s two economically developed regions (East and the West) are highly similar, unlike the East region, which significantly differs from the rest.
The following policy suggestions are put forward based on the current conclusion. Firstly, local governments are encouraged to build a green, low-carbon, circular, and government-led technological innovation economic system while simultaneously driving green public and private sector development. They should increase investment in corporate technology incubation and research and development, create a market-oriented green technology innovation system and achieve economic development. At the same time, local governments should introduce policies, frameworks, and mechanisms to protect the ecology and improve the efficiency of green development. All regions should strive to enhance the competitiveness of green and low-carbon industries. Secondly, local governments should strengthen ER, accelerate the establishment of legal systems and policy orientations for green production and consumption, and combine formal regulations with informal regulations to jointly promote the efficiency of green development. In all regions, provincial and city governments should consistently pursue reform and innovation support. They should promote the optimization and upgrading of IS while building a green and low-carbon modern industrial system to stimulate the vitality of urban green development. The technological spillover effect brought by FDI plays a crucial role in green development. The smart utilization of FDI should focus on achieving green and sustainable economic development and strengthening local enterprises through knowledge, technology, and skills. Thirdly, regional governments should implement differentiated green development policies and green transformation strategies according to local conditions, strengthen strategic thinking of regional integration, design and coordinate development plans, and fully release the green development potential empowered by LGIS.
The chief limitation of the article is that the study findings represent local governments in China, focusing on single-country and lack comparisons with other regions or countries. Cross-country comparisons in future studies are encouraged to unwarp the complexities in the LGIS-GDE nexus. Second, LGIS is a dynamic and complex process, so its effect (promote or inhibit) on GDE may involve changes in other factors (e.g., regional GDP, regional degree of openness in economies or society, per capita income, and economic policy) not included in this study. Thus, future researchers can explore these factors in the current model. Third, the study did not capture the mechanisms or pathways through which LGIS effect GDE in each region. The article only explains the linear relationship between some selected factors, i.e., FAI, ER, IS, FDI, and GDE. Future research can use nonlinear techniques to investigate LGIS-key factors-GDE nexus.
The datasets presented in this study can be found in online repositories. The names of the repository/repositories and accession number(s) can be found in the article/Supplementary Material.
SK: writing. UK: proofreading. MK: supervision. TS: basic idea, conceptualization, writing initial draft, methodology, and statistical software. XW: literature review, policy recommendations, and revision. YN: introduction, conclusion, and revision.
The authors declare that the research was conducted in the absence of any commercial or financial relationships that could be construed as a potential conflict of interest.
All claims expressed in this article are solely those of the authors and do not necessarily represent those of their affiliated organizations, or those of the publisher, the editors and the reviewers. Any product that may be evaluated in this article, or claim that may be made by its manufacturer, is not guaranteed or endorsed by the publisher.
Arellano, M., and Bond, S. (1991). Some tests of specification for panel data: Monte Carlo evidence and an application to employment equations. Rev. Econ. Stud. 58 (2), 277–297. doi:10.2307/2297968
Cao, M. (2011). Dynamic analysis and forecast of China’s energy economic efficiency. China’s Popul. Resour. Environ. 21 (04), 81–87. https://kns.cnki.net/kcms/detail/detail.aspx?FileName=ZGRZ201104012&DbName=CJFQ2011.
Chen H, H. J., Feng, W. Z., and Jiao, X. Y. (2021). The spatial and temporal pattern evolution of green development level and development efficiency of Beijing-Tianjin-Hebei cities. Ecol. Econ. 37 (04), 96–102. https://kns.cnki.net/kcms/detail/detail.aspx?FileName=STJJ202104017&DbName=DKFX2021.
Chen J, J., Wang, Q., and Huang, J. (2021). Motorcycle ban and traffic safety: Evidence from a quasi-experiment at Zhejiang, China. J. Adv. Transp. 2021, 1–13. doi:10.1155/2021/7552180
Chen, P. (2015). Three basic propositions of local government innovation. Adm. Manag. Reform (02), 40–44. doi:10.14150/j.cnki.1674-7453.2015.02.009
Chen, Y., and Sivakumar, V. (2021). Investigation of finance industry on risk awareness model and digital economic growth. Ann. Oper. Res., 1–22. doi:10.1007/s10479-021-04287-7
Chen, Y., Wen, C. H., and Shen, T. Y. (2022). Research on green development efficiency evaluation and spatial evolution of shuangcheng economic circle in chengdu-chongqing region. Resour. Environ. Yangtze River Basin 31 (05), 1137–1151. https://kns.cnki.net/kcms/detail/detail.aspx?FileName=CJLY202205017&DbName=DKFX2022.
Chen, Y., and Wu, J. (2021). The impact of regional integration on industrial green development efficiency and spatial differentiation: Evidence from the Yangtze River Delta urban agglomeration. Dongyue Lun. Cong 42 (10), 151–161. doi:10.15981/j.cnki.dongyueluncong.2021.10.017
David, R. (2009). How to do Xtabond2: An introduction to difference and system GMM in stata. Stata J. 1, 86–136. doi:10.1177/1536867X0900900106
Ding, J., Liu, B., and Shao, X. (2022). Spatial effects of industrial synergistic agglomeration and regional green development efficiency: Evidence from China. Energy Econ. 112, 106156. doi:10.1016/j.eneco.2022.106156
Donald, B., and Gordon, M. (2016). The urban informal economy, local inclusion and achieving a global green transformation. Habitat Int. 53, 97–105. doi:10.1016/j.habitatint.2015.11.002
Du, J. G., Wang, Y., and Zhao, A. W. (2020). Research on the impact and action mechanism of smart city construction on urban green development. Soft Sci. 34 (09), 59–64. doi:10.13956/j.ss.1001-8409.2020.09.09
Fan, Z. G., Deng, Z. K., Zhang, P. W., and Wu, Y. F. (2022). Green development efficiency measurement and influence mechanism in ecological civilization pilot area. Statistics Decis. 38 (05), 75–79. doi:10.13546/j.cnki.tjyjc.2022.05.014
Gao, X., He, Z. H., and Zhang, F. Y. (2022). How do government subsidies and environmental regulations enhance regional green technology innovation? research on linkage effect based on configuration perspective. Shanghai: Research and Development Management, 1–11. doi:10.13581/j.cnki.rdm.20211499
Gao, Z., and Li, M. (2021). The impact of institutional quality and government innovation support on the high-quality economic development of resource-based cities in the Yellow River Basin: From a supply-side perspective. Soft Sci. 35 (08), 121–127. doi:10.13956/j.ss.1001-8409.2021.08.18
Guan, Y., Shan, Y., Huang, L., Chen, H., Wang, D., and Klaus, H. (2021). Assessment to China’s recent emission pattern shifts. Earth's. Future 9. doi:10.1029/2021ef002241
Guo, A. J., and Zhang, N. (2020). Theoretical mechanism and empirical test of the impact of market-oriented reform on green development efficiency. China Popul. Resour. Environ. 30 (08), 118–127. https://kns.cnki.net/kcms/detail/detail.aspx?FileName=ZGRZ202008013&DbName=CJFQ2020.
Guo, F. Y., Gao, S. Q., Tong, L. J., and Ren, J. M. (2022). Temporal and spatial evolution characteristics and influencing factors of green development efficiency in the Yellow River Basin. Geogr. Res. 41 (01), 167–180. https://kns.cnki.net/kcms/detail/detail.aspx?FileName=DLYJ202201012&DbName=DKFX2022.
Guo, J. B., and Chen, F. L. (2021). Local government competition, environmental regulation and green development of urban agglomerations. Explor. Econ. Issues (01), 113–123.
Han, C., and Gao, S. X. (2018). Political strategy, breakthrough innovation and government innovation support: The moderating effect of technical capability and strategic flexibility. Contemp. Econ. Manag. 40 (09), 18–23. doi:10.13253/j.cnki.ddjjgl.2018.09.003
He, A., and An, M. (2019). Local government competition, environmental regulation and green development efficiency. China Popul. Resour. Environ. 29 (03), 21–30. https://kns.cnki.net/kcms/detail/detail.aspx?FileName=ZGRZ201903003&DbName=CJFQ2019.
He, Y., Guo, T., and Fang, F. (2020). Early warning research on urban sustainable development efficiency in Beijing-Tianjin-Hebei: Prediction based on grey support vector machine regression model. Ecol. Econ. 36 (09), 95–100. https://kns.cnki.net/kcms/detail/detail.aspx?FileName=STJJ202009016&DbName=DKFX2020.
He, Z. K. (2013). Coordinated governance of the state and society: From the perspective of local government innovation. Comp. Econ. Soc. Syst. 5, 109–116. https://kns.cnki.net/kcms/detail/detail.aspx?FileName=JJSH201305013&DbName=CJFQ2013.
He, Z. K. (2011). Trend analysis of Chinese government innovation——based on the quantitative research of the award-winning projects of the fifth “China local government innovation award”. J. Beijing Inst. Adm. (01), 1–8. doi:10.16365/j.cnki.11-4054/d.2011.01.009
Hu, A., and Zhou, S. (2014). Green development: Function definition, mechanism analysis and development strategy. China Popul. Resour. Environ. 24 (01), 14–20. https://kns.cnki.net/kcms/detail/detail.aspx?FileName=ZGRZ201401003&DbName=CJFQ2014.
Hu, S. L., Bao, H., Hao, J., and Zeng, G. (2022). Research on the impact of environmental regulation on urban green development in the Yangtze River Delta: An analysis of intermediary mechanism based on technological innovation. Nat. Resour. 37 (06), 1572–1585. doi:10.31497/zrzyxb.20220614
Hu, S., and Wu, H. (2019). Government innovation support and economic development quality: A comparative study from the perspective of ecology-social efficiency. Econ. Forum 12, 5–19. https://kns.cnki.net/kcms/detail/detail.aspx?FileName=JJLT201912002&DbName=CJFQ2019.
Huang, L., and Wu, C. (2021). Foreign investment, environmental regulation and the efficiency of urban green development in the Yangtze River Economic Belt. Reform 3, 94–110. https://kns.cnki.net/kcms/detail/detail.aspx?FileName=REFO202103008&DbName=CJFQ2021.
Huang, X. J. (2017). The mechanism and warning of the “innovation paradox” of local governments. Leadersh. Sci. 31, 54–56. doi:10.19572/j.cnki.ldkx.2017.31.026
Jari, L., Riina, A., Joonas, H., Sirkka, K., Sirpa, K., Riina, K., et al. (2018). Developing key indicators of green growth. Sust. Dev. 26 (1), 51–64. doi:10.1002/sd.1690
Jiang, Q., and Tan, Q. M. (2020). Government innovation subsidy and enterprise innovation and their configuration paths——based on the perspective of quality and efficiency. Soft Sci. 34 (04), 37–43. doi:10.13956/j.ss.1001-8409.2020.04.07
Jiang, Z., Zhao, X., and Zhou, J. (2021). Does the supervision mechanism promote the incentive effects of government innovation support on the R&D input of agricultural enterprises? IEEE Access 9, 3339–3359. doi:10.1109/access.2020.3047839
Kaoru, T. (2001). A slacks-based measure of efficiency in data envelopment analysis. Eur. J. Operational Res. 130 (3), 498–509. doi:10.1016/s0377-2217(99)00407-5
Kaoru, T. (2002). A slacks-based measure of super-efficiency in data envelopment analysis. Eur. J. Operational Res. 143 (1), 32–41. doi:10.1016/s0377-2217(01)00324-1
Kattel, R., and Mazzucato, M. (2018). Mission-oriented innovation policy and dynamic capabilities in the public sector. Indust. Corpor. Change 27 (5). doi:10.1093/icc/dty032
Lan, Z. R. (2021). Research on the heterogeneous impact of low-carbon city construction on regional green development efficiency. Nanjing, Jiangsu Province: Modern Economic Research, 101–110. doi:10.13891/j.cnki.mer.2021.06.01306
Li, J. J., and Jing, Y. J. (2019). Research on the spatial and temporal differentiation of green development efficiency evaluation based on SBM-GIS —Taking the central plains urban agglomeration as an example. Ecol. Econ. 35 (09), 94–101. https://kns.cnki.net/kcms/detail/detail.aspx?FileName=STJJ201909018&DbName=DKFX2019.
Li, S., Ying, Z., Zhang, H., Ge, G., and Liu, Q. (2019). Comprehensive assessment of urbanization coordination: A case study of jiangxi province, China. Chin. Geogr. Sci. 29 (3), 488–502. doi:10.1007/s11769-019-1021-7
Li, X. A. (2021). Environmental regulation, government subsidies and regional green technology innovation. Econ. Jingwei 38 (03), 14–23. doi:10.15931/j.cnki.1006-1096.2021.03.002
Li, Y., Wang, P., and Liang, J. W. (2020). Research on the impact of ecotourism demonstration areas on the green development of regional economy——based on the perspective of quasi-natural experiments. Explor. Econ. Issues (02), 21–30. https://kns.cnki.net/kcms/detail/detail.aspx?FileName=JJWS202002004&DbName=DKFX2020.
Li, Z., and Yang, S. Y. (2018). Fiscal decentralization, government innovation preference and regional innovation efficiency. Manag. World 34 (12), 29–42. doi:10.19744/j.cnki.11-1235/f.2018.0031
Lin, L. M., Lai, Y. B., Xie, J. L., and He, X. L. (2022). The impact of environmental regulation on urban green development efficiency: An empirical analysis based on super efficiency EBM model and system GMM model. J. Nanjing Univ. Technol. Soc. Sci. Ed., 1–13. https://kns.cnki.net/kcms/detail/detail.aspx?FileName=NJZS20220621002&DbName=CAPJ2022.
Liu, D. H. (2017). Scientific connotation and value orientation of green development concept. Jiangsu Soc. Sci. (03), 1–7. doi:10.13858/j.cnki.cn32-1312/c.2017.03.002
Liu, R. R., Chen, D., Yang, S. C., and Chen, Y. (2021). Evaluation of green development efficiency of the major cities in gansu province, China. Sustainability 13 (6), 3034. doi:10.3390/SU13063034
Long, J., Huang, X. J., and Yu, Z. Y. (2012). The influence of government support behavior on the innovation performance of SMEs: The role of service intermediaries. Sci. Res. 30 (05), 782–788. doi:10.16192/j.cnki.1003-2053.2012.05.011
Luo, G., Wang, X., Wang, L., and Guo, Y. (2021). The relationship between environmental regulations and green economic efficiency: A study based on the provinces in China. Int. J. Environ. Res. Public Health 18 (3), 889. doi:10.3390/IJERPH18030889
Ma, H., and Jin, Y. J. (2022). Environmental regulation, industrial agglomeration and industrial green development in the Yangtze River Economic Belt: Analysis based on adjustment effect and threshold effect. Res. Sci. Technol. Manag. 42 (06), 201–210.
Ma, Q., Tariq, M., Mahmood, H., and Khan, Z. (2022). The nexus between digital economy and carbon dioxide emissions in China: The moderating role of investments in research and development. Technol. Soc. 68, 101910. doi:10.1016/J.TECHSOC.2022.101910
Miao, R., Liu, Y., Wu, L., Wang, D., Liu, Y., Miao, Y., et al. (2022). Effects of long-term grazing exclusion on plant and soil properties vary with position in dune systems in the Horqin Sandy Land. Catena 209, 105860. doi:10.1016/j.catena.2021.105860
Na, Z., Jinqian, D., Fayyaz, A., and Muhammad, U. D. (2020). Local government competition and regional green development in China: The mediating role of environmental regulation. Int. J. Environ. Res. Public Health 10, 3485. doi:10.3390/ijerph17103485
Pan, D., and Chen, H. (2021). Border pollution reduction in China: The role of livestock environmental regulations. China Econ. Rev. 69, 101681. doi:10.1016/j.chieco.2021.101681
Pearce, D., Markandya, A., and Barbier, E. B. (1996). Blueprint for green economy. Beijing: Beijing Normal University Press.
Pei, Y., Xu, W. F., and Yang, G. Q. (2018). Green credit investment, green industry development and regional economic growth: Taking huzhou city, zhejiang province as an example. Zhejiang Soc. Sci. 3, 45–53. doi:10.14167/j.zjss.2018.03.005
Qi, Y. Y., Deng, X., and Ren, C. Q. (2022). Trade openness, environmental regulation and urban green development efficiency: Evidence from 282 cities in China from 2010 to 2018. Econ. Issues Explor. (05), 145–160. https://kns.cnki.net/kcms/detail/detail.aspx?FileName=JJWS202205010&DbName=DKFX2022.
Qin, X. L., and Yu, W. C. (2016). The impact of government investment in science and technology on the environmental effect of FDI: An empirical study based on the spatial correlation of 257 prefecture-level cities. J. Central Univ. Finance Econ. (10), 3–12. https://kns.cnki.net/kcms/detail/detail.aspx?FileName=ZYCY201610001&DbName=CJFQ2016.
Qin, Y., Xi, B., Sun, X., Zhang, H., Xue, C., and Wu, B. (2022). Methane emission reduction and biological characteristics of landfill cover soil amended with hydrophobic biochar. Front. Bioeng. Biotechnol. 10, 905466. doi:10.3389/fbioe.2022.905466
Qiu, F., Chen, Y., Tan, J., Liu, J., Zheng, Z., and Zhang, X. (2020). Spatial-temporal heterogeneity of green development efficiency and its influencing factors in growing metropolitan area: A case study for the xuzhou metropolitan area. Chin. Geogr. Sci. 30 (2), 352–365. doi:10.1007/s11769-020-1114-3
Qiu, L., He, L., Lu, H., and Liang, D. (2022a). Pumped hydropower storage potential and its contribution to hybrid renewable energy co-development: A case study in the qinghai-tibet plateau. J. Energy Storage 51, 104447. doi:10.1016/j.est.2022.104447
Qiu, L., He, L., Lu, H., and Liang, D. (2022b). Systematic potential analysis on renewable energy centralized co-development at high altitude: A case study in qinghai-tibet plateau. Energy Convers. Manag. 267, 115879. doi:10.1016/j.enconman.2022.115879
Shan, Y. L., Guan, D. B., Zheng, H., Ou, J., Li, Y., Meng, J., et al. (2018). China CO2 emission accounts 1997-2015. Sci. Data 5 (1), 170201. doi:10.1038/sdata.2017.201
Shan, Y. L., Huang, Q., Guan, D. B., and Hubacek, K. (2020). China CO2 emission accounts 2016-2017. Sci. Data 7 (1), 54. doi:10.1038/s41597-020-0393-y
Shan, Y. L., Liu, J. H., Liu, Z., Xu, X., Shao, S., Wang, P., et al. (2016). New provincial CO2 emission inventories in China based on apparent energy consumption data and updated emission factors. Appl. Energy 184, 742–750. doi:10.1016/j.apenergy.2016.03.073
Sheng, H., Zhang, Y., Wang, W., Shan, Z., Fang, Y., Lyu, W., et al. (2022). High confident evaluation for smart city services. Front. Environ. Sci. 1103. 950055. doi:10.3389/fenvs.2022.950055
Shi, Y. F., and Lan, X. Y. (2021). Research on the spatial network structure of green development efficiency of resource-based cities in the central region. Statistics Inf. Forum 36 (10), 86–98. https://kns.cnki.net/kcms/detail/detail.aspx?FileName=TJLT202110009&DbName=CJFQ2021.
Song L, M. L., Tao, W. L., and Weng, S. M. (2021). Research on the dynamic relationship between regional industrial upgrading, government innovation support and energy eco-efficiency: An empirical analysis of the Huaihe Ecological Economic Belt. J. China Univ. Geosciences ( Soc. Sci. Ed. 21 (04), 119–132. doi:10.16493/j.cnki.42-1627/c.2021.04.010
Song M, M., Tao, W., and Weng, S. (2021). Research on the dynamic relationship between regional industrial upgrading, government innovation support and energy eco-efficiency: An empirical analysis of the Huaihe Ecological Economic Belt. J. China Univ. Geosciences Soc. Sci. Ed. 21 (04), 119–132. doi:10.16493/j.cnki.42-1627/c.2021.04.010
Song, M., Du, J. T., and Hua Tan, K. (2018). Impact of fiscal decentralization on green total factor productivity. Int. J. Prod. Econ. 205, 359–367. doi:10.1016/j.ijpe.2018.09.019
Sung, B., Young Soh, J., and Gun Park, C. (2022). Comparing government support, firm heterogeneity, and inter-firm spillovers for productivity enhancement: Evidence from the Korean solar energy technology industry. Energy 246, 123250. doi:10.1016/J.ENERGY.2022.123250
Tan, Z., Zhu, H., He, X., Xi, B., Tian, Y., Sun, X., et al. (2022). Effect of ventilation quantity on electron transfer capacity and spectral characteristics of humic substances during sludge composting. Environ. Sci. Pollut. Res. Int., 1–16. doi:10.1007/s11356-022-20808-8
Tian, H., Qin, Y., Niu, Z., Wang, L., and Ge, S. (2021a). Summer maize mapping by compositing time series sentinel-1A imagery based on crop growth cycles. J. Indian Soc. Remote Sens. 49 (11), 2863–2874. doi:10.1007/s12524-021-01428-0
Tian, H., Wang, Y., Chen, T., Zhang, L., and Qin, Y. (2021b). Early-season mapping of winter crops using sentinel-2 optical imagery. Remote Sens. 13 (19), 3822. doi:10.3390/rs13193822
Wang, B., Tang, W. S., Wu, Y. R., and Zhang, N. (2014). Has urbanization improved the efficiency of China’s green development? Econ. Rev. (04), 38–49. doi:10.19361/j.er.2014.04.004
Wang, D. L., Wan, K. D., and Yang, J. Y. (2019). Ecological efficiency of coal cities in China: Evaluation and influence factors. Nat. Hazards (Dordr). 95 (1), 363–379. doi:10.1007/s11069-018-3441-6
Wang H, H., He, X. Y., and Xu, S. W. (2022). The influence mechanism of innovative city pilots on green innovation efficiency. China Popul. Resour. Environ. 32 (04), 105–114. https://kns.cnki.net/kcms/detail/detail.aspx?FileName=ZGRZ202204011&DbName=CJFQ2022.
Wang, J. R., and Zhang, Y. (2018). Environmental regulation, green technology innovation willingness and green technology innovation behavior. Sci. Res. 36 (02), 352–360. doi:10.16192/j.cnki.1003-2053.2018.02.018
Wang Q, Q., Sha, H., Cao, S., Zhao, B., Wang, G., and Zheng, P. (2022). Tourmaline enhanced methane yield via regulating microbial metabolic balance during anaerobic co-digestion of corn stover and cow manure. Bioresour. Technol. 359, 127470. doi:10.1016/j.biortech.2022.127470
We, i. L. L., and Hou, Y. Q. (2021). Research on the effect of specialized and diversified industrial agglomeration on regional green development. Manag. Rev. (10), 22–33. doi:10.14120/j.cnki.cn11-5057/f.2021.10.003
Wen, C. H. (2021). New work in the field of high-quality development of the Yangtze River economic belt: Review of “research on innovation-driven and green transformation development of the Yangtze River economic belt”. Resour. Environ. Yangtze River Basin 30 (05), 1284–1286. https://kns.cnki.net/kcms/detail/detail.aspx?FileName=CJLY202105025&DbName=DKFX2021.
Weng, Y. J., Wang, X. T., and Chen, S. J. (2022). Spatial-temporal differentiation and influencing mechanism of green development efficiency in three major urban agglomerations in Zhejiang Province, China. J. Appl. Ecol. 33 (02), 509–516. doi:10.13287/j.1001-9332.202202.014
Wu, R. C., and Wu, D. (2018). On local government innovation resilience. Jiangsu Soc. Sci. (01), 127–132. doi:10.13858/j.cnki.cn32-1312/c.2018.01.017
Xiang, Y. B., Wang, S. Y., and Deng, C. X. (2021). Spatial differentiation and driving factors of green development efficiency of chemical industry in the Yangtze River Economic Belt. Econ. Geogr. (04), 108–117. doi:10.15957/j.cnki.jjdl.2021.04.014
Xie, B., Shan, C. Y., and Zhang, C. H. (2018). Research on the impact of scientific and technological innovation and environmental regulation on regional ecological efficiency. Ecol. Econ. 34 (04), 86–92. https://kns.cnki.net/kcms/detail/detail.aspx?FileName=STJJ201804018&DbName=DKFX2018.
Xu, D., Lu, J. W., and Gu, Q. (2014). Organizational forms and multi-population dynamics: Economic transition in China. Adm. Sci. Q. 59 (3), 517–547. doi:10.1177/0001839214541866
Xu, Y., and Ouyang, W. H. (2022). Dynamic measurement and influence mechanism of urban green development level in jiangxi province. Resour. Environ. Yangtze River Basin 31 (05), 1152–1168. https://kns.cnki.net/kcms/detail/detail.aspx?FileName=CJLY202205018&DbName=DKFX2022.
Yan, L., Yin-He, S., Qian, Y., Zhi-Yu, S., Chun-Zi, W., and Zi-Yun, L. (2021). Method of reaching consensus on probability of food safety based on the integration of finite credible data on block chain. IEEE Access 9, 123764–123776. doi:10.1109/ACCESS.2021.3108178
Yang, W., and Zhao, J. (2018). Can government environmental information disclosure help improve the quality of the ecological environment? Econ. Manag. (08), 5–22. doi:10.19616/j.cnki.bmj.2018.08.001
Yang, Y. P., Wu, D., Xu, M., Yang, M. T., and Zou, W. J. (2022). Capital misallocation, technological innovation, and green development efficiency: Empirical analysis based on China provincial panel data. Environ. Sci. Pollut. Res. Int. doi:10.1007/s11356-022-20364-1
Yang, Z., and Wen, C. (2017). Evaluation of China’s green development efficiency and regional differences. Econ. Geogr. 37 (03), 10–18. doi:10.15957/j.cnki.jjdl.2017.03.002
Ye, X., and Liu, J. (2018). Government support, technological market development and technological innovation efficiency. Econ. Dyn. 07, 67–81. https://kns.cnki.net/kcms/detail/detail.aspx?FileName=JJXD201807008&DbName=CJFQ2018.
Yu, K. P. (2008). Thirty years of governance changes in China (1978-2008). J. Soc. Sci. 03, 5–17+159. https://kns.cnki.net/kcms/detail/detail.aspx?FileName=JLDB200803000&DbName=CJFQ2008.
Yu, J., and Huang, L. (2017). Dynamics of local government innovation in contemporary China: An analytical framework based on institutional change theory. Acad. Mon. 49 (02), 96–105. doi:10.19862/j.cnki.xsyk.2017.02.010
Yu, T., Song, Y., Hao, F., and Wang, W. (2016). Differences in economic efficiency and spatial pattern evolution of jilin province. Geoscience 07, 1066–1072. doi:10.13249/j.cnki.sgs.2016.07.013
Yuan, H., Zou, L., Luo, X., and Feng, Y. (2022). How does manufacturing agglomeration affect green development? A spatial and nonlinear perspective. Int. J. Environ. Res. Publ. Health 19 (16). doi:10.3390/IJERPH191610404
Yue, L., Cao, Y., and Ren, W. (2022). Foreign direct investment, heterogeneous innovation and green development efficiency. Int. Econ. Trade Explor. 38 (03), 68–81. doi:10.13687/j.cnki.gjjmts.2022.03.003
Yue, L., and Xue, D. (2020). Spatiotemporal change of urban green development efficiency in the Yellow River Basin and influencing factors. Resour. Sci. 42 (12), 2274–2284. doi:10.18402/resci.2020.12.02
Zhang, J., Chang, Y., Zhang, L., and Li, D. (2018). Do technological innovations promote urban green development? a spatial econometric analysis of 105 cities in China. J. Clean. Prod. 182, 395–403. doi:10.1016/j.jclepro.2018.02.067
Zhang, J., Wu, G., and Zhang, J. (2004). Estimation of China’s interprovincial physical capital stock: 1952 — 2000. Econ. Res. (10), 35–44. https://kns.cnki.net/kcms/detail/detail.aspx?FileName=JJYJ200410004&DbName=CJFQ2004.
Zhang, L., and Ma, L. (2022). Digital economy, industrial structure upgrade and total factor productivity. Statistics Decis. Mak. 38 (03), 5–10. doi:10.13546/j.cnki.tjyjc.2022.03.001
Zhang, P., Wu, J., and Wang, Y. (2015). The dynamic mechanism of government innovation diffusion: An interpretation based on the theory of new institutionalism——taking the construction of institutional effectiveness as an example. Acad. Forum (07), 20–24. doi:10.16524/j.45-1002.2015.07.014
Zhang, R., and Zhong, C. (2022). Smart city pilots, nearby pollution transfer and green and low-carbon development: New evidence from counties in China. China Popul. Resour. Environ. 32 (04), 91–104. https://kns.cnki.net/kcms/detail/detail.aspx?FileName=ZGRZ202204010&DbName=CJFQ2022.
Zhang, Y. T., and Ayele, E. Y. (2022). Factors affecting small and micro enterprise performance with the mediating effect of government support: Evidence from the amhara region Ethiopia. Sustainability 11, 6846. doi:10.3390/SU14116846
Zhang, Y., Zheng, H., and Ge, L. (2022). Research and analysis of green development efficiency measurement and synergistic improvement mechanism: Taking the central plains city cluster as an example. Ecol. Econ. 38 (01), 92–99. https://kns.cnki.net/kcms/detail/detail.aspx?FileName=STJJ202201009&DbName=DKFX2022.
Zhao, J., and Yang, W. (2017). Calculation of green development efficiency in various regions of China. Statistics Decis. (24), 115–120. doi:10.13546/j.cnki.tjyjc.2017.24.029
Zhao, L., Zhang, L., Xu, L., and Hu, M. (2016). The mechanism of human capital, industrial structure adjustment and green development efficiency. China Popul. Resour. Environ. 26 (11), 106–114. https://kns.cnki.net/kcms/detail/detail.aspx?FileName=ZGRZ201611014&DbName=CJFQ2016.
Zhao, X. M., Liu, C. J., and Yang, M. (2018). The effects of environmental regulation on China’s total factor productivity: An empirical study of carbon-intensive industries. J. Clean. Prod. 179, 325–334. doi:10.1016/j.jclepro.2018.01.100
Zhou, L., and Liu, J. (2021). The impact of government investment in science and technology on the green development of industrial enterprises under environmental regulation. Industrial Technol. Econ. 40 (01), 128–133. https://kns.cnki.net/kcms/detail/detail.aspx?FileName=GHZJ202101015&DbName=CJFQ2021.
Zhu, B. Z., Zhang, M. F., Zhou, Y. H., Wang, P., Sheng, J. C., He, K. J., et al. (2019). Exploring the effect of industrial structure adjustment on interprovincial green development efficiency in China: A novel integrated approach. Energy Policy 134, 110946. doi:10.1016/j.enpol.2019.110946
Zhu, M., Wang, K. L., and Tang, H. Y. (2022). Research on the spatial spillover effect of green finance development on ecological efficiency: Taking resource-based cities in the Yellow River basin as an example. Financial Dev. Res. (04), 55–62. doi:10.19647/j.cnki.37-1462/f.2022.04.009
Keywords: government innovation support, green development efficiency, regional analysis, GMM model, China
Citation: Khattak SI, Khan MK, Sun T, Khan U, Wang X and Niu Y (2022) Government innovation support for green development efficiency in China: A regional analysis of key factors based on the dynamic GMM model. Front. Environ. Sci. 10:995984. doi: 10.3389/fenvs.2022.995984
Received: 16 July 2022; Accepted: 26 August 2022;
Published: 23 September 2022.
Edited by:
Asif Razzaq, Ilma University, PakistanReviewed by:
Huaizhou Jin, Xiamen University, ChinaCopyright © 2022 Khattak, Khan, Sun, Khan, Wang and Niu. This is an open-access article distributed under the terms of the Creative Commons Attribution License (CC BY). The use, distribution or reproduction in other forums is permitted, provided the original author(s) and the copyright owner(s) are credited and that the original publication in this journal is cited, in accordance with accepted academic practice. No use, distribution or reproduction is permitted which does not comply with these terms.
*Correspondence: Muhammad Kamran Khan, bWtraGFuLmJ1aWNAYmFocmlhLmVkdS5waw==
Disclaimer: All claims expressed in this article are solely those of the authors and do not necessarily represent those of their affiliated organizations, or those of the publisher, the editors and the reviewers. Any product that may be evaluated in this article or claim that may be made by its manufacturer is not guaranteed or endorsed by the publisher.
Research integrity at Frontiers
Learn more about the work of our research integrity team to safeguard the quality of each article we publish.