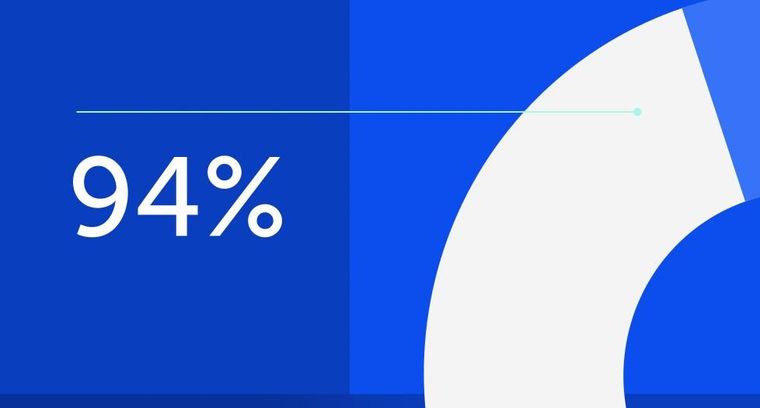
94% of researchers rate our articles as excellent or good
Learn more about the work of our research integrity team to safeguard the quality of each article we publish.
Find out more
ORIGINAL RESEARCH article
Front. Environ. Sci., 10 November 2022
Sec. Environmental Economics and Management
Volume 10 - 2022 | https://doi.org/10.3389/fenvs.2022.994323
This article is part of the Research TopicEconomic Development, Social Consequences, and Technological Innovation Under Climate Change COVID-19 Pandemic ConditionsView all 48 articles
Green technology innovation is an important driving force for the upgrading of the manufacturing value chain. The purpose of this study is to explore the effect of green technology innovation on the upgrading of the manufacturing value chain under institutional environments. To this aim, the panel data of 28 provinces in China from 2010 to 2019 and panel threshold regression model are applied. Empirical results show that the effect of green technology innovation on the upgrading of the manufacturing value chain is nonlinear under institutional environments. The effect of formal and informal institutional environments on the relationship between green technology innovation and the upgrading of the manufacturing value chain is also different. The positive effect of green technology innovation on the upgrading of the manufacturing value chain is best when the level of formal institutional environment is high and informal institutional environment is moderate. In addition, when we divide the sample into three regions (Eastern, Central, Western), the effect of green technology innovation on the upgrading of the manufacturing value chain under institutional environments shows regional heterogeneity. Hence, with this calculated optimal interval of institutional environments, the government can adjust and improve institutional environments so as to provide the most favorable institutional conditions for green technology innovation to promote the upgrading of the manufacturing value chain. This research is helpful for the government to make rational decisions according to the situation.
Constructing a powerful manufacturing country, promoting green transformation and upgrading the manufacturing industry are important development strategies of China at present. Under economic globalization, the key to strengthening the manufacturing industry is to promote the position of the manufacturing industry in the global value chain. However, developed countries have firmly occupied the high end of the global value chain with their advanced technology, abundant market resources and strong independent innovation ability, and China is facing the dilemma of “low-end locking” in the global value chain (Chen et al., 2019). Production and manufacturing links at the low end of the global value chain tend to consume a lot of energy and emit a lot of carbon dioxide (Ren et al., 2014; Kan et al., 2019), China’s resource and environmental carrying capacity is close to its limit, and it is unsustainable to sacrifice the environment for the development of manufacturing (Qu et al., 2020).
Green technology innovation can promote the industrial green transformation (Kemp and Never, 2017) and achieve “win-win” state between economic development and ecological protection (Sun et al., 2008; Schiederig et al., 2012). In this context, China has launched “Made in China 2025″ strategy and “Industrial Green Development Plan (2016–2020)", and the report of the 19th National Congress of the Communist Party of China also clearly proposed to “build a market-oriented green technology innovation system and strengthen the main position of green technology innovation in enterprises”, which aimed at driving the upgrading of the manufacturing value chain through green technology innovation and realizing the coordinated development of society, economy and ecology. However, green technology innovation has the positive externality of knowledge and technology spillover and the negative externality of production pollution. Society can benefit from the reduction of environmental pollution while enterprises bear the high risk and high investment of green technology innovation, so it is difficult to drive enterprises to carry out green technology innovation only by market mechanism (Wu et al., 2021). Some studies showed that policies can effectively promote green technology innovation, especially environmental regulations (Yang and Yang, 2015); social trust can reduce the occurrence of infringement or “free riding” to a certain extent, and alleviate the externality of green technology innovation (Yang et al., 2021); the media can guide the public to understand and evaluate the green innovation behavior of enterprises, which will exert public pressure on enterprises, and then force enterprises to pay attention to green innovation (Sun et al., 2021), all of which imply that we can improve formal institutions such as environmental regulation and informal institutions such as social trust and media attention to promote enterprises to carry out green innovation activities and then promote the upgrading of the manufacturing value chain. However, most of the researches on the relationship between green technology innovation, institutional environments and the upgrading of the manufacturing value chain focus on the exploration of the relationship between the two rather than bring institutional environments into the research framework of green technology innovation and the upgrading of the manufacturing value chain and explore the relationship between the three. For example, Yuan and Chen (2019) used GMM estimation to test the impact of environmental regulation on technological innovation and the impact of green technology innovation on the transformation and upgrading of manufacturing, and concluded that strict environmental regulation would promote pollution-intensive enterprises to engage in green technology innovation, and that the relationship between green technology innovation and the transformation and upgrading of manufacturing was U-shaped; Du et al. (2021) measured the heterogeneous effects of environmental regulation on green technology innovation and the industrial structure in 105 environmental monitoring cities in China, and concluded that when the level of economic development is low, environmental regulation will inhibit the development of green technology innovation. In addition, the advantage of the threshold model is that it can find the structural break points through real data simulation and perform statistical tests on it, which overcomes the subjectivity of selecting the threshold through group regression and cross model (Yang and Song, 2019).
Based on existing research results, this study attempts to fill the gap in the existing literature by incorporating the institutional environments into the research framework of green technology innovation and the upgrading of the manufacturing value chain, and further testing the effect of institutional environments on the relationship between green technology innovation and the upgrading of the manufacturing value chain. Specifically, based on the panel data of 28 provinces in China from 2010 to 2019, this study empirically investigate the driving effect of green technology innovation on the upgrading of the manufacturing value chain, and the effect of institutional environments on the relationship between green technology innovation and the upgrading of the manufacturing value chain. This study answers the following two questions: 1) Can green technology innovation effectively promote the upgrading of the manufacturing value chain? 2) How to adjust and improve the institutional environments to make green technology innovation better promote the upgrading of the manufacturing value chain? Does formal and informal institutional environments play different roles? Is there regional heterogeneity? This study can make the following contributions: firstly, because of the externalities of green technology innovation, it is difficult to promote enterprise to carry out green technology innovation only by the market mechanism. This study believes that the institutional environments plays an important role in the process of green technology innovation promoting the upgrading of the manufacturing value chain, and brings it into the research framework. Secondly, this study brings formal and informal institutional environments into the research framework at the same time, and compares the differences of their roles. Thirdly, it further divides the sample into three regions to explore the regional heterogeneity of effcet of green technology innovation on the upgrading of the manufacturing value chain under different institutional environments, aiming at providing the government with a rational basis for making decisions on regional green technology innovation policies and improving institutional environments. The rest of the study is organized as follows: Section 2 reviews relevant literature, Section 3 provides theoretical background and research hypothesis, Section 4 provides model settings and variable descriptions, Section 5 provides results and discussion, Section 6 provides conclusions and policy recommendations, and Section 7 is the limitations and future research directions.
With the development of green environmental protection and low-carbon life concepts, the effect of green technology innovation and institutional environments on the upgrading of the manufacturing value chain has gradually attracted the attention of scholars. Regarding the relationship between green technology innovation, institutional environments and the upgrading of the manufacturing value chain, scholars have mostly explored the effect of green technology innovation on the upgrading of the manufacturing value chain, the effect of institutional environments on green technology innovation and the effect of institutional environments on the upgrading of the manufacturing value chain.
Regarding the relationship between green technology innovation and the upgrading of the manufacturing value chain, there are two more representative views at home and abroad: one view is that green technology innovation can effectively promote the upgrading of the manufacturing value chain, stimulate changes in demand and play a decisive role in the upgrading of the industrial structure (Kaplinsky and Morris, 2000). This positive effect is mainly reflected in two aspects: the innovative compensation effect and the energy saving and emission reduction effect. The innovative compensation effect is mainly because green technology innovation can promote the upgrading of enterprise processes, products and functions by increasing production efficiency, improving or creating brand new products, and promoting the fame of brands (Yuan and Dai, 2017). The energy saving and emission reduction effect is mainly the improvement of enterprise’s green technology innovation ability that can promote the development and the use of various cleaner production processes, emission reduction technologies and green products, which can reduce energy consumption and pollution emissions, save environmental costs, thus improving enterprise profitability and promoting the upgrading of the value chain (Hua, 2011). Another view is that green technology innovation has a certain inhibitory effect on the upgrading of the manufacturing value chain, which is mainly embodied in the lock-in effect and crowding-out effect. The lock-in effect is mainly because in international competition, the developed countries, with their advantages in mastering core technology and resources and their position in the “high end” of the global value chain, threaten OEM enterprises in developing countries at the low end of the value chain with large transaction orders to maintain the production position of low cost, high energy consumption and high pollution. The lock-in effect has seriously inhibited the enterprises’ enthusiasm for green technology innovation in developing countries, making them locked in the low-end of manufacturing sector with high pollution and low technology for a long time (Yang and Lu, 1998; Zhong and Wang, 2000). The “crowding-out effect” is mainly because in the process of transforming from high pollution and low efficiency technology to low pollution and high efficiency green and clean technology, enterprises need to invest more money to transform existing equipment and technology, which will inevitably crowd out other development funds when they have limited funds. Therefore, the crowding-out effect will cause a decline of an enterprise’s production profits in a short period of time, which is not conducive to the upgrading of the value chain (Yuan and Shen, 2000).
In the above studies, most scholars have only investigated the unidirectional promotion or inhibitory relationship between green technology innovation and the upgrading of the manufacturing value chain, and only a few scholars have explored the bidirectional nonlinear relationship. For example, Song et al. (2021) empirically analyzed the impact of green technology innovation on the upgrading of the manufacturing value chain, and believed that green technology innovation has a U-shaped impact on the upgrading of the manufacturing value chain.
In the field of world economic research, in which the global value chain specialization is a hot topic, the importance of institutional factors has been repeatedly emphasized. Whether the institutional environments are good will affect a country’s role in the global value chain, and the institutional environments have a positive effect on the upgrading of the global value chain (Nunn, 2007; Feenstra et al., 2013). Good institutional environments can reduce the uncertainty in enterprises’ Research and Development (R&D), drive the development and application of high technology, and promote the continuous upgrading of value chain’s status (Tebaldi and Elmsile, 2013). Conversely, a country’s participation in the international division of the global value chain will also promote the improvement of its institutional quality (Jin et al., 2008). The definition of institutions in these studies mainly involves legal rules and trade agreements in contract execution and property rights protection (Hu and Zhang, 2015), which do not include institutional reforms targeting the internals of a country and tend to adopt a marketization index to measure the institutional environment (Shen and Wang, 2019; Zhao et al., 2019). However, the differences in characteristics between the index of marketization and technological innovation may lead to incorrect conclusions (Xu, 2018).
In recent years, with the introduction of a series of national environmental regulation policies, although some scholars have explored the relationship between environmental regulation and the upgrading of the value chain (Han and Yan, 2020), they have ignored the role of informal institutional environment. The effective implementation of formal institutions such as environmental regulation cannot be separated from the external supervision and governance mechanisms (Becker and Murphy, 1993). Therefore, it is difficult to comprehensively and deeply reflect the current institutional environments and its relationship with the upgrading of manufacturing value chains in China only from the perspective of formal environmental regulation.
Regarding the impact of the institutional environments on green technology innovation, domestic and foreign scholars have mostly explored it from the perspective of formal environmental regulation, but they have not formed a unified understanding. There are mainly the following viewpoints: firstly, moderate environmental regulation is conducive to promoting green technology innovation (Potter and Van der Linde, 1995; Lee et al., 2010); secondly, environmental regulation is detrimental to firms’ technological innovation (Gray and Shadbegian, 2003; Blind, 2012); thirdly, the relationship between environmental regulation and green technology innovation is nonlinear and environmental regulation has a U-shaped or inverted U-shaped impact on green technology innovation (Peng et al., 2017; Du et al., 2019). The reason why the above conclusions are inconsistent is that the research object and observation period are different, and the mechanism of different types of environmental regulation on green technology innovation is also different (Wang and Qi, 2016). At present, China’s formal institutions are not perfect enough to effectively promote enterprises to carry out green technology innovation (Yang et al., 2021), and informal institutions can complement and support formal institutions, thus improving the performance of formal institutions and promoting the realization of environmental governance goals (Wheeler and Afsah, 2000; Féres and Reynaud, 2012). Therefore, it is necessary to explore this in more depth from the dual perspectives of formal and informal institutional environments.
Institutional environments are composed of cognitive, normative, regulatory structures and activities (Scott, 1995). According to its nature, it can be divided into formal institutional environment and informal institutional environment. The formal institutional environment mainly includes political environment, legal environment, etc. While the informal institutional environment mainly includes culture, language, social ideology and public psychology (North, 1990). Formal environmental regulation is one of the institutional pressures imposed by the government on environmental behavior of enterprises, which can reflect the level of formal institutional environment in the region to a certain extent. Social trust and media attention are important elements of informal institutions, which have an important influence on enterprises’ adoption of clean technology, attention to environmental management and reduction of pollution emissions (Becker and Murphy, 1993; da Motta and Moreira, 2006; Zhao and Zhang, 2020).
Institutional theory argues that institutional environments in which enterprises are located will have an important impact on the behaviors of enterprises (Oliver, 1991), which implies that we can promote enterprises to carry out green innovation activities by adjusting and improving the institutional environments and then promote the upgrading of the manufacturing value chain.
Due to the high input cost and a certain risk in the early stage of green technology innovation, only a few enterprises with huge capital and strong R&D capacity will choose to try, which cannot drive the transformation and upgrading of industries. With a further increase in the intensity of the formal institutional environment, the deterrent effect of administrative punishment may make enterprises perceive the increase of illegal risks and costs, so enterprises will increase their investment in green technology innovation, improve production efficiency, cut production costs. The resulting profits can compensate or even offset the costs brought by environmental regulation, thereby generating innovative compensation effect and promoting industrial transformation. Therefore, the effect of green technology innovation on the upgrading of the manufacturing value chain changes with the formal institutional environment. And the level of formal institutional environment needs to reach a certain threshold before green technology innovation can promote the upgrading of the manufacturing value chain, that is, there is a non-linear relationship.
As media pay more attention to environmental issues, enterprises will increase investment in environmental protection to avoid damage to their public image, develop clean energy and green products, improve green innovation ability. In addition, a high level of social trust is more conducive to promoting enterprises to fulfil their social contract and obey environmental laws and regulations, which promotes the efficiency of the cooperation between enterprises and reduces the costs. Therefore, as the informal institutional environment improves, it will be good for green technology innovation to promote the upgrading of the manufacturing value chain. However, when the media pay too much attention to environmental issues, excessive public pressure may induce government’s administrative punishment, which causes a strong burden of legitimacy to enterprises. Thus, the green technology innovation activities of enterprises, and even the survival and development of enterprises will be affected, which is not conducive to promoting the upgrading of the manufacturing value chain. It can be seen that the effect of green technology innovation on the upgrading of the manufacturing value chain will be different due to the different levels of informal institutional environment. Accordingly, this study proposes hypothesis:
It is necessary to study the direct relationship between green technology innovation and the upgrading of the manufacturing value chain. Therefore, we construct model (1). According to the previous analysis, the relationship between green technology innovation and the upgrading of the manufacturing value chain may be non-linear, we refer to the practice of Zhao and Xi (2022) by adding the quadratic term of green technology innovation and constructing the following two-way fixed effects panel model:
In order to avoid generating errors by artificial grouping and to effectively judge the significance of the threshold value, this study use Hansen’s nonlinear panel threshold regression model to construct a piecewise function with the formal and informal institutional environments as threshold variables to verify whether green technology innovation has a nonlinear threshold effect on the upgrading of the manufacturing value chain. The multiple threshold panel model (taking a double threshold as an example) is as follows:
In the above models, i denotes the province, t denotes the year, umvc (upgrading of the manufacturing value chain) is the explanatory variable, gti (green technology innovation) is the explanatory variable, gti2 is the quadratic term of green technology innovation, ie (institutional environments) is the threshold variable (divided into formal and informal institutional environments), control denotes a set of control variables affecting the upgrading of the manufacturing value chain (human capital (hc), degree of openness (open) and financial development (fd)), I (∙) is an indicative function, γ is threshold values,
Upgrading of the manufacturing value chain (umvc). At present, most of the quantitative indicators of the upgrading of the manufacturing value chain are measured by the manufacturing profit margin, manufacturing profits and taxes, the status index of the value chain, the export technological level and the participation index. However, according to the “smiling curve” theory, most developed countries are in high-end segments of the global value chain with high-tech and high-value-added while developing countries are mainly in low-end segments of the global value chain with low-tech and low-value-added, such as simple production, assembly, processing and manufacturing. Therefore, according to the logic that the trade structure reflects the production structure, under the global production network system, the technical complexity of a country or region’s production and exports of products can reflect the position of a country or region in the global value chain. Based on the practice of Qiu et al. (2012), this study uses export complexity to measure the upgrading of the manufacturing value chain. The specific calculation formulas are as follows:
In model (4), PRODY denotes the industry export complexity; i denotes the province, k denotes the industry, Xik denotes the export value of industry k in region i, Xi denotes the export value of all industries in region i, and Yi denotes the per capita Gross Domestic Product (GDP) of region i. In model (5), MVC denotes the regional export complexity, Xin denotes the export value of year n in region i, Xink denotes the export value of industry k in region i and year n.
Green technology innovation (gti). Green total factor productivity considers both socio-economic factors such as capital, labor and desired output in an integrated manner, as well as resource and environmental factors such as energy consumption and undesired output, which has been widely used (Qu et al., 2020). Given that the traditional Data Envelopment Analysis (DEA) model does not consider slack variables and cannot distinguish effective decision units whose efficiency is all 1, Tone (2002) proposed the super Slack Based Measure-Data Envelopment Analysis (SBM-DEA) model based on the traditional DEA model. Thus, this study refers to the practice of Lv et al. (2021) by using the Global Malmquist-Luenberger (GML) index of super SBM-DEA model to calculate green total factor productivity and using green total factor productivity to characterize the level of green technology innovation in each province. In addition, this study measures green total factor productivity from two aspects of input and output. The specific input and output indicators are shown in Table 1.
Since GML only reflects the rate of change of green technology innovation, this study refer to the practice of Song et al. (2018) by assuming that the level of green technology innovation in the base period of 2009 is 1, and the level of green technology innovation in 2010 is equal to the level of green technology innovation in 2009 multiplied by the GML index, and so on for other years to obtain the level of green technology innovation in each province from 2010 to 2019.
Formal institutional environment (fie). At this stage, in order to restrict the environmental violations of enterprises, the Chinese government often uses environmental administrative penalties as the main means to impose sanctions on illegal enterprises in the process of implementing environmental regulations policies. In addition, sewage charge is the first negative market incentive tool adopted in China, which has a long implementation time, wide coverage and strong representativeness. The total number of environmental proposals of National People’s Congress (NPC) and Chinese People’s Political Consultative Conference (CPPCC) is an important form of public participation in environmental protection supervision, which represents the public’s attention to environmental issues to a certain extent.
Therefore, this study draws on the practices of Wang et al. (2020), Yi et al. (2019) and Wu et al. (2020) by taking the number of environmental administrative penalties, sewage charges and total environmental proposals of NPC and CPPCC in each province to characterize the formal institutional environment. In addition, the entropy method is used to combine the number of environmental administrative penalties, sewage charges and total environmental proposals of NPC and CPPCC in each province to comprehensively measure the formal institutional environment.
Informal institutional environment (iie). Most of study use a single index to measure it, and this study uses the entropy method to combine social trust and media attention together, which is more reasonable. Referring to the relevant research of Wang and Li (2017), Liu and Li (2019), survey data from the China General Social Survey (CGSS) are used to measure the level of provincial social trust. The following question was included in all previous surveys: “In general, do you agree that the vast majority of people in this society can be trusted?” Respondents were asked to choose the most appropriate answer from a list of five options ranging from “completely disagree” to “completely agree”. The five options are assigned values of 1, 2, 3, 4 and 5, respectively. The simple average of the respondents’ answers in the area was calculated as the level of regional social trust in the current year. The higher the score is, the higher the level of provincial social trust. Since survey data from 2014, 2016, 2018 and 2019 were unavailable during the sample period and social trust remains relatively stable for a certain period of time, the data from 2013 was used to measure the level of provincial social trust in 2014, the data from 2015 was used to measure the level of provincial social trust in 2016, and the data from 2017 were used to measure the level of provincial social trust in 2018–2019. Based on the practice of Xu et al. (2011), we use the number of environmental-related news reports in each province from 2009 to 2018 to reflect the degree of media attention in the area (considering the media lag, the 1-year lagged data are processed simultaneously with the data from 2010 to 2019). The data were collected from two authoritative official media outlets (News.com and People’s Daily Online) and two market-oriented online media outlets (Sina.com and Sohu.com). We conduct searches using keywords such as “ecological environment, environmental pollution, closure of polluting enterprises, environmental accountability, green development, environmental governance, environmental taxation and environmental insurance”, clean the data of related environmental reports, merge the reports with the same or similar contents, and finally obtain the number of environmental-related news reports in 28 provinces via manual sorting.
Regarding the selection of control variables, relevant studies have confirmed that human capital (hc), degree of openness (open), financial development (fd) have a certain impact on the upgrading of industry. Therefore, this study introduces these three variables as control variables to limit errors. As for the selection of the measurement indicators of the variables, human capital (hc) is measured by the average years of education in each province and calculated by the formula: [(primary school population *6 years)+ (junior middle school population *9 years)+(senior high school population *12 years)+(junior college population and above* 16 years)]/(population aged 6 years and above), degree of openness (open) is measured by the ratio of total imports and exports volume to the GDP in each province, financial development (fd) is measured by the ratio of loan balance of financial institutions to the GDP in each province.
Considering data continuity and availability, this study selects the panel data of 28 provinces from 2010 to 2019 for empirical evaluation. To obtain the data, China’s statistical yearbook, China’s science and technology statistical yearbook, China’s energy statistical yearbook, China’s environment yearbook, China’s tax yearbook, the China General Social Survey, the international trade research and decision support system of China’s research network, and the statistical yearbooks of various regions were consulted.
In order to eliminate the influence of heteroscedasticity, umvc and hc are processed with logarithm in this study. The descriptive statistical results of variables are shown in Table 2.
In order to avoid the existence of false regression, this study carries out unit root test on panel data before empirical analysis. The test results are shown in Table 3. It can be seen from Table 3 that p-value of LLC and PP-Fisher tests of the seven sequences are less than 0.05, so we can think all panel data are stable.
To avoid the interference of multiple multicollinearity, VIF (Variance Inflation Factor) method is used to diagnose the multicollinearity. The specific test results are shown in Table 4. It can be seen from Table 4 that VIFs of all variables are less than 5, indicating that there is no multicollinearity among the variables.
First, the relationship between green technology innovation and the upgrading of the manufacturing value chain is estimated. The regression results are shown in Table 5. In model 1, the coefficient of green technology innovation is negative. In model 2, the coefficient of gti is significantly negative, and the coefficient of gti2 is significantly positive. The possible reasons are as follows: the knowledge path and market dependence lead to the embedded dependence of China’s manufacturing industry in the global value chain, which means that manufacturing enterprises give priority to the use of low-cost technology in the value chain and refuse to implement high cost independent innovation (Hao and Zhang, 2016). Thus, enterprises may choose the scheme with the least economic loss to avoid the risk of green technology innovation, and cannot truly drive the industrial transformation and upgrading. With the gradual promotion of green technology innovation, the compensation effect produced by green technology innovation will reduce pollution costs while improving product quality and competitive advantage. When enterprises realize value-creating ability of green technology innovation, they will actively carry out green technology innovation, form a virtuous circle, and truly promote the upgrading and development of industry. Song et al. (2021) believed that there is a U-shaped relationship between green technology innovation and the upgrading of manufacturing value chain, that is, green technology innovation must exceed a certain threshold to promote the upgrading of the manufacturing value chain. Our results are consistent with Song et al. (2021).
The threshold model developed by Hansen assumes that the threshold variable is not endogenous. We draw on the practices of Yang et al. (2019) and Zhang et al. (2022) by using the Durbin Wu Hausmann chi-square test to tset the endogeneity of threshold variables, and it shows formal institutional environment (fie) and informal institutional environment (iie) are not endogenous (chi-sq value is 0.12 and 0.42, respectively; p-values is 0.7337 and 0.5147, respectively).
Results of the threshold effect significance test corresponding threshold estimations and the 95% confidence intervals using formal and informal institutional environments as the threshold variables are shown in Table 6. It can be seen from Table 6 that both the single threshold and the double threshold pass the significance test.
After completing the threshold effect significance test, we need to test the authenticity of the threshold estimation. When the upgrading of the manufacturing value chain is used as an dependent variable, the two thresholds estimated from the double threshold model of the formal institutional environment are 0.152 and 0.370, respectively. The corresponding likelihood ratio function graph is shown in Figure 1 and Figure 2. The figures show that the two threshold estimates of the formal institutional environment fall within the acceptable domain of the original hypothesis, indicating that the two threshold estimates are equal to the true value and pass the threshold authenticity test. The two thresholds estimated from the double threshold model of the informal institutional environment are 0.218 and 0.516, respectively. The corresponding likelihood ratio function graph is shown in Figure 3 and Figure 4. Similarly, we can know that the two threshold estimates of the informal institutional environment are equal to the true value and pass the threshold authenticity test.
The threshold regression model estimation results for green technology innovation and the upgrading of the manufacturing value chain obtained by using formal and informal institutional environments as the threshold variables are shown in Table 7. Thus, hypothesis is proved.
The results show that the relationship between green technology innovation and the upgrading of the manufacturing value chain is U-shaped under formal institutional environment. When the level of formal institutional environment is lower than 0.152, green technology innovation has a negative effect on the upgrading of the manufacturing value chain, but the effect is not significant. When the level of formal institutional environment ranges from 0.152 to 0.370, the effect of green technology innovation on the upgrading of the manufacturing value chain shift from negative to positive. This result shows that with the increase of the level of formal institutional environment, green technology innovation begins to promote the upgrading of the manufacturing value chain. When the level of formal institutional environment is greater than 0.370, the positive effect is enhanced. The possible reasons are as follows:
As green technology innovation is still in the preliminary stage of development, with strong economic externalities, as well as the characteristics of high investment and high risk, enterprises lack sufficient motivation to carry out it. As the level of formal institutional environment increases, in order to avoid the adverse impact of administrative penalties on production and operation, enterprises will improve the production process, develop new green products and increase the added value of products through green technology innovation, thus improving the competitiveness of enterprises and promoting the upgrading of the manufacturing value chain.
According to the threshold model estimation results in Table 7, there is a complex nonlinear relationship between green technology innovation and the upgrading of the manufacturing value chain under informal institutional environment. When the level of informal institutional environment is less than 0.218, green technology innovation has a positive effect on the upgrading of the manufacturing value chain, with a coefficient of 0.0880 significant at the 1% level. When the level of informal institutional environment ranges from 0.218 to 0.516, green technology innovation still significantly and positively promotes the upgrading of the manufacturing value chain. However, when the level of informal institutional environment is greater than 0.516, the positive effect diminishes to some extent. The possible reasons are as follows: after the events of environmental pollution are reported by the media, it will generate public opinion pressure on enterprises and force them to take corresponding improvement measures, so as to promote enterprises to implement green technology innovation. This is not only conducive to solving the public’s demands for environmental protection, but also helps enterprises achieve certain economic benefits through energy conservation and emission reduction. In addition, a high level of social trust is more conducive to promoting enterprises to fulfill their social contract and obey environmental laws and regulations, which promotes the efficiency of the cooperation between enterprises and reduces the transaction cost. However, when media pay too much attention to environmental issues, it will have a negative effect on the enterprise’s green technology innovation. This is mainly because the public will have a higher degree of acceptance of the negative information with the massive exposure of the negative news, which will seriously damage the social reputation and image of enterprises, and is not conductive to promoting green technology innovation and strategy of development.
In terms of control variables, the regression coefficient of financial development (fd) is significantly positive, indicating that financial development is conducive to promoting the upgrading of the manufacturing value chain, probably because a developed financial system can provide financial support for green innovation and relieve financial pressure. The regression coefficient of human capital (hc) is positive at 1% significance level, indicating that the cultivation of human capital has a positive effect on the upgrading of the manufacturing value chain, probably because high-quality human capital can provide solid intellectual support for the upgrading of the manufacturing value chain. The regression coefficient of degree of openness (open) is significantly negative, indicating that degree of openness has a negative effect on the upgrading of China’s manufacturing value chain at this stage, which may be related to the low technology content of China’s foreign trade products for a long time.
In order to further enhance the reliability of the research results, we used alternative measures of institutional environments to test the robustness. Considering the number of environmental petitions and the total number of environmental proposals of the NPC and CPPCC are both an important form of public participation in environmental protection supervision, which represent the public’s attention to environmental issues to a certain extent, we use the number of environmental petitions replace the total number of environmental proposals of the NPC and CPPCC to calculate the level of formal institutional environment. As for informal institutional environment, we have changed the calculation method of social trust. The original calculation method was to assign values of 1, 2, 3, 4 and 5 to the five options from “completely disagree” to “completely agree”, and the simple average of the respondents’ answers in the area was calculated as the level of regional social trust in the current year. Now, we draws on the practices of Liu and Li (2019) by using the proportion of “completely agree” and “relatively agree” in the total number of respondents in the area as the level of regional social trust. Robustness test results are shown in Table 8. The double threshold effect still exists and the coefficient symbol of each variable does not change significantly. Therefore, our empirical analysis is reliable and stable.
In order to further reveal the regional heterogeneity of the relationship between green technology innovation and the upgrading of the manufacturing value chain, and get more targeted research conclusions, this study divides the samples into three groups (including Eastern region, Central region, Western region). Based on the regional significance test and confidence intervals of institutional environments thresholds in Table 9, it is found that the single threshold of Eastern region and Western region, double threshold of Central region pass the significance test when taking formal institutional environment as the threshold variable. Similarly, the double threshold of Eastern region and Central region, the single threshold of Western region pass the significance test when taking informal institutional environment as the threshold variable.
TABLE 9. Regional significance test and confidence intervals of institutional environments thresholds.
The regional threshold regression results for green technology innovation and the upgrading of the manufacturing value chain obtained by using the formal institutional environment as the threshold variable are shown in Table 10. The results show that there is significant regional heterogeneity in the relationship between green technology innovation and the upgrading of the manufacturing value chain in Eastern region, Central regional and Western region. The details are presented as follows:
1) In Eastern region, green technology innovation has a positive effect on promoting the upgrading of the manufacturing value chain. When the level of formal institutional environment is greater than the first threshold value of 0.384, the promotion effect is further enhanced. 2) In Central region, green technology innovation has a U-shaped effcet on the upgrading of the manufacturing value chain. When the level of formal institutional environment is less than 0.103, green technology innovation will inhibit the upgrading of the manufacturing value chain. When the level of formal institutional environment is greater than 0.103, the effect of green technology innovation on the upgrading of the manufacturing value chain will shift from negative to positive. When the level of formal institutional environment is greater than the second threshold value of 0.226, the promotion effect is further enhanced. 3) In Western region, green technology innovation also has a U-shaped effect on the upgrading of the manufacturing value chain. When the level of formal institutional environment is less than 0.114, green technology innovation will inhibit the upgrading of the manufacturing value chain. When the level of formal institutional environment exceeds 0.114, the effect of green technology innovation on the upgrading of manufacturing value chain shift from negative to positive. The possible reasons are as follows: Eastern region has a more perfect environmental protection institutions and stronger enforcement of environmental regulation policies, so the formal institutional environment show a better effect. However, economic development in Central and Western region is relatively dropped behind. Considering the maintenance of enterprises’ competitive advantage, the protection of employment and the development of economy in this area, local governments may have a “weak enforcement” of environmental regulations. Therefore, the level of formal institutional environment in Central and Western region must exceed a certain threshold to promote green technology innovation and then realize the upgrading of the manufacturing value chain.
The regional threshold regression results for green technology innovation and the upgrading of the manufacturing value chain obtained by using the informal institutional environment as the threshold variable are presented as follows:
1) In Eastern region, the relationship between green technology innovation and the upgrading of the manufacturing value chain is nonlinear. When the level of informal institutional environment is less than 0.224, green technology innovation will promote the upgrading of the manufacturing value chain. When the level of informal institutional environment is between 0.224 and 0.494, the promotion effect will be enhanced. However, when the level of informal institutional environment is greater than 0.494, the promotion effect will be diminished. 2) In Central region, the relationship between green technology innovation and the upgrading of the manufacturing value chain is also nonlinear. When the level of informal institutional environment is less than 0.260, green technology innovation will promote the upgrading of the manufacturing value chain. When the level of informal institutional environment ranges from 0.260 to 0.407, the promotion effect will be enhanced. When the level of informal institutional environment is greater than 0.407, the promotion effect will be further enhanced. 3) In Western region, green technology innovation has a U-shaped effect on the upgrading of the manufacturing value chain. When the level of informal institutional environment is less than 0.153, green technology innovation will inhibit the upgrading of the manufacturing value chain. When the level of informal institutional environment exceeds 0.153, the effect of green technology innovation on the upgrading of the manufacturing value chain will shift from negative to positive. The possible reasons are as follows: Eastern region has a higher level of development, more access to resources, higher information transparency, and people are more sensitive to environmental issues. However, the ability to obtain resources is relatively weak in Central and Western regions, and the degree of information asymmetry is higher. In Eastern region, moderate media attention is conducive to promoting the implementation of policies and supervising the innovation behavior of enterprises, while excessive media attention may bring negative effect; in Central and Western regions, media attention can greatly alleviate information asymmetry, improve information transparency, alleviate “weak enforcement”, further promote enterprises’ innovation and then realize the upgrading of the manufacturing value chain.
With the development of globalization, China has increasingly participated in the international division of labor, and the scale of foreign trade is also expanding. However, China’s manufacturing industry has fell into the dilemma of “low-end locking” when the industry was integrated into the system of the division of labor in the global value chain. It has been indicated by some research that green technology innovation is an important driving force to promote the upgrading of manufacturing value chain. Due to the externalities of green technology innovation, it is difficult to promote enterprises to carry out green technology innovation activities only by the market mechanism. According to the institutional theory, the institutional environments in which an enterprise is located will have an important impact on its behavior. Therefore, what impact do institutional environments have on the relationship between green technology innovation and the upgrading of the manufacturing value chain? To this aim, the panel data of 28 provinces in China from 2010 to 2019 and panel threshold regression model are applied. The empirical results show that green technology innovation can promote the upgrading of the manufacturing value chain in the long run. There is a nonlinear relationship between green technology innovation and the upgrading of the manufacturing value chain under institutional environments, and the role of formal and informal institutional environments are different. In addition, when we divide the sample into three regions (Eastern, Central, Western), the effect of green technology innovation on the upgrading of the manufacturing value chain under institutional environments shows regional heterogeneity. These findings can help us to clarify the internal logic between the three variables and provide reference for the government to implement policies accurately. According to the above results, some policies can be implemented to promote enterprises to carry out green technology innovation and realize the upgrading of manufacturing value chain:
1) Restrict harmful non-green innovation activities. It can be seen from the above empirical results that green technology innovation can promote the upgrading of the manufacturing value chain in the long run. Therefore, while guiding enterprises to carry out green technology innovation, the government should make good use of the dual effect of institutional environments to supervise and restrict the non-green innovation activities that may cause negative externalities to the ecological environment.
2) Measure the performance of green technology innovation from a comprehensive perspective. Enterprises should not use simple income and expenditure to measure the benefits of green technology innovation. They should realize that the mode of high energy consumption and high pollution will not only have a negative impact on the environment, but also go against the sustainable development of itself. Therefore, they should actively carry out green innovation activities, which are not only beneficial to enterprises to gain market competitive advantage, but also can promote the transformation and upgrading of manufacturing industry.
3) Take effective actions to reduce the risk of uncertainty. Rising uncertainty is one of the prominent phenomenon of today’s modern economic systems, it not only affects some macroeconomic parameters (Işık et al., 2020), but also affects green innovation decision-making behavior of enterprises. Therefore, we should actively take the following measures: 1) the government should aim at the forefront of green technology innovation, focus on key areas, and publish a green technology demand directory to point out the direction for enterprises; 2) we should create an environment with high social trust, alleviate information asymmetry, reduce transaction costs, and strengthen the supervision of green technology innovation of enterprises; 3) the government should try to maintain consistency and continuity in formulating and implementing policies. At the same time, we must suit our measures to different conditions in terms of locality and time rather than “one size fits all” when adjust the regional institutional environment.
Due to various factors, this study still has the following limitations and the follow-up research can be improved from the following aspects: 1) Research object: This study mainly takes China as the research object without considering other countries. Future research can take other countries as the object to verify whether our conclusions are universal. 2) Sample size: This study mainly uses the data of 28 provinces in China from 2010 to 2019. Although it is enough to carry out the research in this study, future research can expand the time scope to make the research results more comprehensive. 3) Research perspective: Most of the previous studies are based on the perspective of formal environmental regulation to explore the relationship between the institutional environments, green technology innovation and the upgrading of the manufacturing value chain while ignore the possible impact of the informal institutional environment, thus making the research results not comprehensive. Although this study considers the perspective of informal institutional environment, it does not consider the synergy of the formal institutional environment and informal institutional environment. Future research can consider the synergy of the two to further enrich the Porter hypothesis and global value chain theory.
The original contributions presented in the study are included in the article/Supplementary Material, further inquiries can be directed to the corresponding author/s.
XW: Conceptualization, methodology, validation, supervision, project administration, writing—review and editing. JZ: Software formal analysis, data curation, writing—original draft preparation. QM: review and editing.
This research was funded by the National Social Science Fund of China (20BJY038).
The authors declare that the research was conducted in the absence of any commercial or financial relationships that could be construed as a potential conflict of interest.
All claims expressed in this article are solely those of the authors and do not necessarily represent those of their affiliated organizations, or those of the publisher, the editors and the reviewers. Any product that may be evaluated in this article, or claim that may be made by its manufacturer, is not guaranteed or endorsed by the publisher.
The Supplementary Material for this article can be found online at: https://www.frontiersin.org/articles/10.3389/fenvs.2022.994323/full#supplementary-material
Becker, G. S., and Murphy, K. M. (1993). A simple theory of advertising as a good or bad. Q. J. Econ. 108, 941–964. doi:10.2307/2118455
Blind, K. (2012). The influence of regulations on innovation: A quantitative assessment for OECD countries. Res. Policy 41, 391–400. doi:10.1016/j.respol.2011.08.008
Chen, L., Luo, S., and Zhao, T. (2019). Financial constraints, trade mode transition, and global value chain upgrading of Chinese firms. Sustainability 11, 4527. doi:10.3390/su11174527
da Motta, R. S., and Moreira, A. (2006). Efficiency and regulation in the sanitation sector in Brazil. Util. Policy 14, 185–195. doi:10.1016/j.jup.2006.03.002
Du, K., Cheng, Y., and Yao, X. (2021). Environmental regulation, green technology innovation, and industrial structure upgrading: The road to the green transformation of Chinese cities. Energy Econ. 98, 105247. doi:10.1016/j.eneco.2021.105247
Du, L. Z., Zhao, Y. H., Tao, K. T., and Lin, W. F. (2019). Compound effects of environmental regulation and governance transformation in enhancing green competitiveness. Econ. Res. J. 54, 106–120.
Feenstra, R. C., Hong, C., Ma, H., and Spencer, B. J. (2013). Contractual versus non-contractual trade: The role of institutions in China. J. Econ. Behav. Organ. 94, 281–294. doi:10.1016/j.jebo.2013.08.009
Féres, J., and Reynaud, A. (2012). Assessing the impact of formal and informal regulations on environmental and economic performance of Brazilian manufacturing firms. Environ. Resour. Econ. (Dordr). 52, 65–85. doi:10.1007/s10640-011-9520-8
Gray, W. B., and Shadbegian, R. J. (2003). Plant vintage, technology, and environmental regulation. J. Environ. Econ. Manage. 46, 384–402. doi:10.1016/s0095-0696(03)00031-7
Han, M. M., and Yan, D. (2020). Can environmental regulation enhance the status in the division of labour in global value chain? Empirical evidence from Chinese manufacturing industries. Commer. Res. 10, 33–41. doi:10.13902/j.cnki.syyj.2020.10.004
Hao, F. X., and Zhang, L. (2016). The impact of low-end lock-in on the upgrading of local industries in the global value chain. Sci. Res. Manage. (S1), 131–141. doi:10.19571/j.cnki.1000-2995.2016.s1.021
Hu, Z. L., and Zhang, Y. (2015). Does institutional quality improvement promote the position in GVC? World. Econ. Stud. 8, 19–26. doi:10.13516/j.cnki.wes.2015.08.003
Hua, Z. (2011). Empirical study on China's eco-innovation capability and lts lnfluencing factors: Based on DEA-malmquist productivity index. J. Technol. Econ. 30, 38–43.
Işık, C., Sirakaya-Turk, E., and Ongan, S. (2020). Testing the efficacy of the economic policy uncertainty index on tourism demand in USMCA: Theory and evidence. Tour. Econ. 26 (8), 1344–1357. doi:10.1177/1354816619888346
Jin, X. R., Ru, Y. X., and Wu, H. (2008). Institutions, firm productivity and inter-regional export differences in China. J. Manag. World. 11, 65–77. doi:10.19744/j.cnki.11-1235/f.2008.11.009
Kan, S. Y., Chen, B., and Chen, G. Q. (2019). Worldwide energy use across global supply chains: Decoupled from economic growth? Appl. Energy 250, 1235–1245. doi:10.1016/j.apenergy.2019.05.104
Kaplinsky, R., and Morris, M. (2000). A handbook for value chain research Brighton: University of Sussex, Institute of Development Studies.
Kemp, R., and Never, B. (2017). Green transition, industrial policy, and economic development. Oxf. Rev. Econ. Policy 33 (1), 66–84. doi:10.1093/oxrep/grw037
Lee, J., Veloso, F. M., Hounshell, D. A., and Rubin, E. S. (2010). Forcing technological change: A case of automobile emissions control technology development in the US. Technovation 30, 249–264. doi:10.1016/j.technovation.2009.12.003
Liu, X. X., and Li, M. H. (2019). The impact of social trust level on audit pricing: Empirical evidence based on CGSS data. Bus. Manag. J. 41, 143–161. doi:10.19616/j.cnki.bmj.2019.10.009
Lv, C., Shao, C., and Lee, C. C. (2021). Green technology innovation and financial development: Do environmental regulation and innovation output matter? Energy Econ. 98, 105237. doi:10.1016/j.eneco.2021.105237
North, D. C. (1990). Institutions, institutional change and economic performance. Cambridge: Cambridge University Press.
Nunn, N. (2007). Relationship-specificity, incomplete contracts, and the pattern of trade. Q. J. Econ. 122, 569–600. doi:10.1162/qjec.122.2.569
Oliver, C. (1991). Strategic responses to institutional processes. Acad. Manage. Rev. 16, 145–179. doi:10.5465/amr.1991.4279002
Peng, W., Cheng, F., and Lu, J. (2017). G-CSF treatment promotes apoptosis of autoreactive T cells to restrict the inflammatory cascade and accelerate recovery in experimental allergic encephalomyelitis. Exp. Neurol. 35, 73–84. doi:10.1016/j.expneurol.2016.10.008
Porter, M. E., and Van der Linde, C. (1995). Toward a new conception of the environment-competitiveness relationship. J. Econ. Perspect. 9, 97–118. doi:10.1257/jep.9.4.97
Qiu, B., Ye, L. F., and Sun, S. Q. (2012). An empirical study on the impact of participation in global production networks on the upgrading of China's manufacturing value chain: An analysis based on export complexity. China. Ind. Econ. 1, 57–67. doi:10.19581/j.cnki.ciejournal.2012.01.006
Qu, C. Y., Shao, J., and Cheng, Z. H. (2020). Can embedding in global value chain drive green growth in China’s manufacturing industry? J. Clean. Prod. 268, 121962. doi:10.1016/j.jclepro.2020.121962
Ren, S. G., Yuan, B. L., Ma, X., and Chen, X. H. (2014). International trade, FDI (foreign direct investment) and embodied CO2 emissions: A case study of chinas industrial sectors. China Econ. Rev. 28, 123–134. doi:10.1016/j.chieco.2014.01.003
Schiederig, T., Tietze, F., and Herstatt, C. (2012). Green innovation in technology and innovation management–an exploratory literature review. R&D Manage. 42, 180–192. doi:10.1111/j.1467-9310.2011.00672.x
Shen, Q., and Wang, S. P. (2019). Technological innovation, institutional innovation and efficiency analysis of lndustrial transformation and upgrading in central China. China. Soft. Sci. 4, 176–183.
Song, M., Du, J., and Tan, K. H. (2018). Impact of fiscal decentralization on green total factor productivity. Int. J. Prod. Econ. 205, 359–367. doi:10.1016/j.ijpe.2018.09.019
Song, P., Chen, Z., and Song, D. (2021). Can green technology innovation promote GVC of China's manufacturing industry? —empirical test based on WIOD data. Collect. Essays. Financ. Econ. 5, 3–13. doi:10.13762/j.cnki.cjlc.20210118.002
Sun, J. H., Xu, L. L., and Hu, J. (2021). The impact of informal environmental regulation on enterprise green technology innovation from the perspective of environmental responsibility- A mediated regulation model. J. Technol. Econ. 10, 10–22.
Sun, Y., Lu, Y., Wang, T., Ma, H., and He, G. (2008). Pattern of patent-based environmental technology innovation in China. Technol. Forecast. Soc. Change 75, 1032–1042. doi:10.1016/j.techfore.2007.09.004
Tebaldi, E., and Elmslie, B. (2013). Does institutional quality impact innovation? Evidence from cross-country patent grant data. Appl. Econ. 45, 887–900. doi:10.1080/00036846.2011.613777
Tone, K. (2002). A slacks-based measure of super-efficiency in data envelopment analysis. Eur. J. Oper. Res. 143, 32–41. doi:10.1016/s0377-2217(01)00324-1
Wang, B. B., and Qi, S. Z. (2016). Technological innovation effects of market-based and command-based policy instruments for energy conservation and emission reduction. Empirical evidence based on patent data of Chinese industrial industries. China. Ind. Econ. 6, 91–108. doi:10.19581/j.cnki.ciejournal.2016.06.008
Wang, Y., and Li, S. M. (2017). Does social trust enhance corporate M&A performance? J. Manag. World. 12, 125–140. doi:10.19744/j.cnki.11-1235/f.2017.12.013
Wang, Y., Li, Y. X., Ma, Z., and Song, J. B. (2020). Can environmental administrative punishment serve as an example? A study on the deterrent effect of environmental regulation from the perspective of peer impact. J. Manag. Sci. China. 23, 77–95.
Wheeler, B. L. B., and Afsah, S. (2000). Greening industry: New roles for communities, markets and governments. World bank policy research report New York: Oxford University Press.
Wu, L., Jia, X. Y., Wu, C., and Peng, J. C. (2020). Impact of heterogeneous environmental regulation on green total factors productivity. China. Popul. Resour. Environ. 30, 82–92.
Wu, W. H., Sheng, L. Y., Tang, F. C., Zhang, A. M., and Liu, J. (2021). A system dynamics model of green innovation and policy simulation with an application in Chinese manufacturing industry. Sustain. Prod. Consum. 28, 987–1005. doi:10.1016/j.spc.2021.07.007
Xu, H. (2018). Typical mechanisms of institutional environment affecting technological innovation: A theoretical interpretation and spatial test. Nankai. Econ. Stud. 5, 133–154. doi:10.14116/j.nkes.2018.05.008
Xu, L. P., Xin, Y., and Zhu, J. G. (2011). Media attention and fulfillment of social responsibility of listed companies: An empirical study based on wenchuan earth-quake donations. J. Manag. World. 3, 135–143. doi:10.19744/j.cnki.11-1235/f.2011.03.014
Yang, F. M., and Lu, Y. (1998). Research on combined incentives of green technology innovation. Sci. Res. Manag. 1, 41–45. doi:10.19571/j.cnki.1000-2995.1998.01.009
Yang, F., and Yang, M. (2015). Analysis on China's eco-innovations: Regulation context, intertemporal change and regional differences. Eur. J. Oper. Res. 247 (3), 1003–1012. doi:10.1016/j.ejor.2015.07.029
Yang, Q., and Song, D. (2019). How does environmental regulation break the resource curse: Theoretical and empirical study on China. Resour. Policy 64, 101480. doi:10.1016/j.resourpol.2019.101480
Yang, X., He, L., Xia, Y., and Chen, Y. (2019). Effect of government subsidies on renewable energy investments: The threshold effect. Energy Policy 132, 156–166. doi:10.1016/j.enpol.2019.05.039
Yang, Y., Liu, D. S., Zhang, L. X., and Yin, Y. K. (2021). Social trust and green technology innovation: Evidence from listed firms in China. Sustainability 13, 4828. doi:10.3390/su13094828
Yi, M., Fang, X. M., Wen, L., Guang, F. G., and Zhang, Y. (2019). The heterogeneous effects of different environmental policy instruments on green technology innovation. Int. J. Environ. Res. Public Health 16, 4660. doi:10.3390/ijerph16234660
Yuan, L., and Shen, Y. T. (2000). On green technology innovation. Sci. Technol. Prog. Policy. 17, 64–65.
Yuan, Y. J., and Chen, Z. (2019). Green technology innovation and the transformation and upgrading of China's manufacturing industry. Stud. Sci. Sci. 37, 1902–1911. doi:10.16192/j.cnki.1003-2053.2019.10.020
Yuan, Y. J., and Dai, N. (2017). Manufacture industry upgrading path of China based on green technology innovation. Sci. Technol. Manag. 19, 8–15. doi:10.16315/j.stm.2017.01.002
Zhang, B. S., Wang, T. L., and Wang, X. H. (2021). The relationship between government investment in science and technology, R&D personnel scale and output of basic research in universities—Threshold regression analysis based on provincial panel data. Forum. Sci. Technol. China. 4, 55–63+74. doi:10.13580/j.cnki.fstc.2021.04.007
Zhang, H., Zhang, J., and Song, J. (2022). Analysis of the threshold effect of agricultural industrial agglomeration and industrial structure upgrading on sustainable agricultural development in China. J. Clean. Prod. 341, 130818. doi:10.1016/j.jclepro.2022.130818
Zhao, L., and Zhang, L. (2020). The impact of media attention on firms' green technology innovation: The moderating role of marketization level. Manag. Rev. 32, 132–141. doi:10.14120/j.cnki.cn11-5057/f.2020.09.011
Zhao, X., and Xi, Y. (2022). Threshold effects of urban population size and industrial structure on CO2 emissions in China. Front. Env. Sci. 492. doi:10.3389/fenvs.2022.894442
Zhao, Y. H., Zhang, Z., Feng, T. W., and Tao, K. T. (2019). Big data development, institutional environment and government governance efficiency. J. Manag. World 35, 119–132. doi:10.19744/j.cnki.11-1235/f.2019.0152
Keywords: green technology innovation, the upgrading of the manufacturing value chain, formal institutional environment, threshold effect, informal institutional environment
Citation: Wang X, Zhou J and Maitlo Q (2022) Effect of green technology innovation on the upgrading of the manufacturing value chain: Evidence from China. Front. Environ. Sci. 10:994323. doi: 10.3389/fenvs.2022.994323
Received: 14 July 2022; Accepted: 20 October 2022;
Published: 10 November 2022.
Edited by:
Cem Işık, Anadolu University, TurkeyReviewed by:
Mohamed Chakroun, German University of Technology in Oman, OmanCopyright © 2022 Wang, Zhou and Maitlo. This is an open-access article distributed under the terms of the Creative Commons Attribution License (CC BY). The use, distribution or reproduction in other forums is permitted, provided the original author(s) and the copyright owner(s) are credited and that the original publication in this journal is cited, in accordance with accepted academic practice. No use, distribution or reproduction is permitted which does not comply with these terms.
*Correspondence: Jun Zhou, MzA0Njk2QHdodXQuZWR1LmNu; Qamaruddin Maitlo, cWFtYXIubWFpdGxvQGliYS1zdWsuZWR1LnBr
Disclaimer: All claims expressed in this article are solely those of the authors and do not necessarily represent those of their affiliated organizations, or those of the publisher, the editors and the reviewers. Any product that may be evaluated in this article or claim that may be made by its manufacturer is not guaranteed or endorsed by the publisher.
Research integrity at Frontiers
Learn more about the work of our research integrity team to safeguard the quality of each article we publish.