- 1Institute of Land Engineering and Technology, aanxi Provincial Land Engineering Construction Group Co., Xi’an, China
- 2Shaanxi Provincial Land Engineering Construction Group Co., Ltd., Xi’an, China
- 3School of Management, Xi’an Jiaotong University, Xi’an, China
The rapid development of high-standard farmland construction has triggered explosion of farmland cultivated land protection technologies in recent years. Contemporary, eco-friendly fertilization technology (EFFT) are rapidly becoming main force to achieve food security and promote the national ecological strategy. Hence, based on the extended stimulus-organism-response (S-O-R) theoretical analysis framework, this empirical research 295 field interview data of farmers in the high standard farmland grain main production area in Shaanxi Province, a developing country context, to analyze the mechanism of external incentives on farmers’ eco-friendly fertilization technology adoption behavior, further tested to examine the mediating effect of intrinsic perception and moderating effect of the family endowment. We applied the methodological approach, partial least square structural equation modeling (PLS-SEM), to test the hypothetical model. The results show that external incentives can effectively improve farmers’ technology adoptive behavior; internal perception has a significant positive effect on adoptive behavior, and it plays an intermediary role between external incentives and eco-friendly fertilization technology adoption behavior; family endowment has a significant positive effect on farmers’ technology adoption behavior, but the moderating effect of family endowment in external incentive-technology adoption behavior relationship is not significant. Therefore, we should choose appropriate and flexible government regulations, and give full play to the role of premium incentives, so as to improve the motivation of farmers to adopt eco-friendly fertilization technology.
1 Introduction
Reform and opening up for more than 40 years, China’s total agricultural economy can continue to increase, relying mainly on fertilizers, pesticides and other material factors such as a large number of inputs, but the high output behind the face of increasingly serious soil consolidation, pollution, agro-ecological degradation and other problems, soil productivity decline, the quality and safety of agricultural products under threat. (Yang and Li, 2000; Chen, 2007). Soil testing and formulated fertilization technology is an eco-friendly fertilization technology that can improve the green productivity and output quality of land (Wu et al., 2017). In recent years, the country has implemented a number of soil improvement measures and completed the top-level policy design (Liu et al., 2017). The report of the 19th National Congress clearly points out that “we will implement an action plan for soil pollution prevention, control and restoration, and promote green development in the countryside” (Li B. et al., 2020), and the “No. 1 Document” of the Central Government has also repeatedly mentioned the promotion of soil testing and formulated fertilization technology and the implementation of organic fertilizer replacement projects (Stewart et al., 2005; Meng et al., 2013). However, some research results in theoretical circles show that farmers’ eco-friendly fertilization technology adoption behavior is not optimistic, and there is a phenomenon of high willingness but low behavior (Adesemoye and Kloepper, 2009; Xin, 2022), the main crux of which is the obvious difference between farmers’ goals and government goals (Smith et al., 1999). The government, aiming to maximize the sustainable use of land resources and social welfare, tends to promote organic fertilizers with slow effect and low environmental impact to farmers. Rational farmers, aiming at increasing returns or reducing costs, focus more on short-term benefits and tend to use fast-acting chemical fertilizers, ignoring the agricultural surface pollution caused by over-application (Liu et al., 2019; Qi et al., 2021). Organic fertilizer is a labor-intensive production factor, characterized by slow fertilizer efficiency, large volume, and high dosage (Godfray and Garnett, 2014), and its application by farmers increases input costs and does not significantly improve agricultural yields (Jordan-Meille et al., 2012). In addition, soil testing and formulated fertilization technology is a typical public welfare technology with positive environmental externalities, but the lack of quality and price mechanism makes it difficult to compensate the overflowing social benefits through the market price of agricultural products (Marenya and Barrett, 2007), and farmers’ benefits are not effectively improved. The small-scale decentralized operation model will exist for a long time in the future, and it is important to explore how to improve the behavioral initiative of farmers to adopt conservation farming technologies, and whether external incentives can form an endogenous and long-term mechanism for farmers to adopt new technologies, i.e., to achieve cost savings and efficiency gains for farmers (Yan et al., 2008).
The current research on pro-environmental fertilizer application technology adoption behavior by scholars is mainly summarized into two views, one is that most of them use the planned behavior and its expansion model to analyze the influencing factors of farmers’ technology adoption behavior, forming a research paradigm with endogenous factors such as farmers’ individual characteristics, household characteristics, cognitive characteristics, and willingness to participate as the logical main line (Tey and Brindal, 2012; Daxini et al., 2018; Faruque-As-Sunny et al., 2018; Qi et al., 2021). Farmers’ endowments such as the education level of decision makers, the number of household laborers, the degree of part-time employment and the scale of land operation all have significant effects on the adoption of pro-environmental fertilizer application technology (Onwezen et al., 2013). Some studies have extended to the level of internal subjective factors such as individual cognition and psychological factors, and then penetrated into the fields of social psychology and organizational behavior. Dong et al. (2022) proposed a theoretical framework including “economic rationality” and “ecological rationality”, trying to find the behavioral logic of farmers’ conservation farming technology adoption from the internal psychological mechanism. Some scholars clearly distinguished the differences in psychological cognition and behavior of economic individuals, and the limited rationality caused by incomplete cognition and uncertainty in the external environment, and farmers’ motivation to adopt pro-environmental fertilizer application technology was insufficient. The difference in farmers’ green perceptions is one of the important reasons for the paradox of soil testing and formulated fertilization technology adoption intention and behavior (Liu et al., 2019; Li J. et al., 2020). Second, from the perspective of exogenous dynamics such as policy incentives and constraints, social networks and market resource allocation, Elmustapha et al. (2018) point out that government incentives have a greater impact on adoption intentions and decision behavior than farmers’ endowment characteristics. Mazvimavi and Twomlow (2009) and Bravo-Monroy et al. (2016) found that government subsidies, technology training, and agricultural returns were the key factors influencing the adoption decisions of surveyed farmers in choosing eco-friendly farmland soil management technologies. Government subsidies were more effective than controls in inducing proactive farmers’ adoption decisions, and information induction was more applicable to farmers with lower environmental awareness (Atari et al., 2009). Based on social capital theory, interpersonal relationships and social networks can significantly increase the adoption level of green production technologies by farmers (Yang et al., 2020). The market environment has a direct influence on farmers’ decision on soil testing and formulated fertilization technology application behavior mainly through information collection, quality testing, sales channels and prices (Smith and Siciliano, 2015).
The findings of existing studies provide important literature support for this paper. Many scholars believe that external variables such as farmers’ endowment resources (personal characteristics, family characteristics), external environment (market incentives and policy incentives), and internal psychological variables such as cognitive level and willingness to adopt are significant factors affecting farmers’ technology adoption. Bandura’s interaction model of human behavior and motivation theory suggest that behavior is not constrained by internal factors or external stimuli alone, but is determined by the interaction between the individual’s internal needs such as emotions and cognition and the environment, and is a process in which the external environment acts as an engine through internal factors (De Fano et al., 2019). As a rational economic person, farmers’ behavioral decision of technology adoption must be the result of coupled constraints of internal and external factors, and internal psychological motivation and external environmental changes jointly affect farmers’ behavioral decision, and the behavioral decision effect may also differ (Clark et al., 2003; Truelove et al., 2014). Based on the previous research results, this paper tries to conduct additional research in the following aspects: First, the existing literature mostly focuses on analyzing the influence of a single intrinsic factor or external environment on farmers’ behavior, and less incorporates internal factors such as family endowment, intrinsic perception and external incentives into a theoretical analysis framework to explore the path and logical mechanism of external incentives affecting farmers’ technology adoption behavior process. Second, most studies have used Logit regression model, stepwise regression, Bootstrap test and hierarchical regression to test the mediating and moderating effects of a variable step by step (Song et al., 2017), and structural equation model can deal with both the mediating effect of intrinsic perception and the moderating effect of family endowment. Based on the previous research results, this paper tries to conduct additional research in the following aspects: First, the existing literature mostly focuses on analyzing the influence of a single intrinsic factor or external environment on farmers’ behavior, and less incorporates internal factors such as family endowment, intrinsic perception and external incentives into a theoretical analysis framework to explore the path and logical mechanism of external incentives affecting farmers’ technology adoption behavior process. Second, most studies have used Logit regression model, stepwise regression, Bootstrap test and hierarchical regression to test the mediating and moderating effects of a variable step by step (Song et al., 2017), and structural equation model can deal with both the mediating effect of intrinsic perception and the moderating effect of family endowment. In short, this study aims to accomplish two main objectives:
• To explain the transmission mechanism through which external incentives influence farmers’ technology adoption behavior through intrinsic perceptions.
• To test whether there is a moderating effect of family endowments on external incentives to influence technology adoption behavior.
Based on the research objectives, the research questions underpin this quantitative study:
• Does each dimension of Stimulus-Organism-Response (S-O-R) (i.e., external incentives, intrinsic perception, and family endowment) have a positive impact on technology adoption Behavior?
• Does the family endowment moderate the relationship between external incentives and technology adoption behavior?
The significance of this study can be assessed from theoretical and practical perspectives. For theoretical contributions, this study expands on the Stimulus-Organism-Response (S-O-R) to advance the literature within the content of eco-friendly fertilization technology adoption behavior. Then, the empirical findings would enhance the knowledge of the statistical linkages in the context of Chinese agricultural technology. Furthermore, as previous empirical studies have shown the inconsistency relationships among the three main constructs, grounded on S-O-R theoretical framework, the introduction of family endowments as a moderator might provide some new evidence or justifications to the existing framework. The generated research framework also gives a comprehensive interpretation of the connections among the three dimensions of S-O-R, technology adoption Behavior, and the moderating role of family endowments.
For practical contributions, this research findings could be a valuable reference to the practitioners or government decision makers who intend to adopt eco-friendly fertilization technology adoption behavior. Farmers may be persuaded to focus their limited household endowments on specific external incentives that generate the highest levels of acceptance that match their household endowments. Besides, government or policymakers that relate to eco-friendly fertilization technology adoption behavior may look up the research outcomes as shreds of evidence to raise the interest of the agricultural players towards the adoption of eco-friendly technology.
2 Theoretical analysis and hypothesis
2.1 Theoretical model
The Stimulus-Organism-Response (S-O-R) theoretical model belongs to the domain of cognitive psychology, which specifically explains the influence of external environmental characteristics on individuals’ mental cognitive and emotional responses and later behavioral decisions, with emphasis on mental cognition as a mediating element, and provides an effective research paradigm for analyzing individual behavioral mechanisms (Kamboj et al., 2018). The stimulus (S) represents the characteristics of the external environment in which the individual lives, which has a stimulating effect on the organism (O) ‘s cognition and emotions, and the organism undergoes a series of psychological reaction processes that eventually manifest themselves as response (R) to internal or external behavior patterns (Vieira, 2013). The S-O-R model does not necessarily follow a strict “S-O″ and “O-R″ path of action, as external stimulus (S) can act directly on the mental processes of the organism (O) and can also directly produce behavioral responses (R). The S-O-R model has been applied to information systems and e-commerce, and the research involves the user experience and consumer purchase behavior of online social platforms. The paper is based on the analytical framework of S-O-R model, but considering that the S-O-R model cannot better reflect the influence path of farmers’ ability level on individual behavior, we borrow some ideas from the Motivation-Opportunity-Ability (MOA) model of behavioral organization theory. Ability (A) in the MOA model reflects the intrinsic possibility that an individual can engage in a certain behavior, and emphasizes that individual ability works together with Motivation (M) and Opportunity (O) to influence behavioral decisions (Ahmad et al., 2021). This paper incorporates the Ability (A) factor from MOA theory into the S-O-R theoretical model to construct an analytical framework for farmers’ adoption behavior of organic matter reclamation technology and further enhance the explanatory power of the model to reality.
Among the external environmental factors, external incentives are an important component, and changing the incentive structure and incentive level of agricultural production is a fundamental way to improve farmers’ fertilizer application technology and agricultural surface source pollution. Drawing on relevant research results (Pretty et al., 2001; Swinton et al., 2007; Bopp et al., 2019), external incentives mainly refer to incentives from three levels: government, market and society, and considering the principles of data availability, science and operability, external incentives are defined in this paper as two dimensions: policy incentives and market incentives. The policy incentives refer to the government-led technology promotion training and factor subsidies, while the market incentives refer to the market premium incentives brought by the “three products and one standard” and the brand effect to farmers. Among the internal factors, the adoption of technology by farmers is generally influenced by family endowment characteristics (Mendola, 2008), and the ability of family members largely determines whether farmers can overcome the operational challenges of green production technologies and cope with the high cost and riskiness of new technologies. Rogers’ innovation diffusion theory shows that an important influencing factor of technology diffusion is technology attributes, and there may be significant differences in the intrinsic perception of the same technology by different individual farmers, which significantly affects their new technology adoption behavior (Wonglimpiyarat and Yuberk, 2005). Therefore, this paper focuses on the perspective of farmers and constructs a conceptual model of farmers’ behavioral decision to adopt organic soil reclamation technology based on S-O-R extension theory, starting from family endowment, external incentives, and intrinsic motivation mechanisms. The specific theoretical analysis framework is shown in Figure 1.
2.2 Research hypothesis
2.2.1 Extrinsic incentives and technology adoption behavior
The impact of external incentives on farmers’ soil testing and formulated fertilization technology adoption behavior. While improving soil fertility, soil testing and formulated fertilization technology reduces agricultural non-point source pollution caused by chemical fertilizer application, which is typically characterized by high cost, high risk, and slow returns under positive spillovers over time. Small farmers generally have a risk aversion preference, lack the behavioral initiative to apply organic fertilizers that are slow to take effect and consume a lot of energy, and prefer to use chemical fertilizers to avoid the risk of crop yield reduction. External incentives can influence farmers’ soil testing and formulated fertilization technology adoption behavior by generating endogenous long-term mechanisms through two paths: loss aversion and benefit drive. On the one hand, the government compensates farmers appropriately by using technology promotion and implementation factor subsidies to reduce the input costs of green production technologies, which increases the potential benefits of farmers and enhances their endogenous motivation to adopt soil testing and formulated fertilization technology. On the other hand, according to the externality theory, the marginal private gain of farmers adopting the technology is smaller than the marginal social gain, resulting in a low level of economic incentives. The role of market incentive is to solve the problem of farmers’ distrust in market mechanism. “Three products and one standard” quality certification and brand building can make the premium effect appear, improve the expectation of the income of agricultural products, drive the farmers around the radiation to adopt soil testing and formulated fertilization technology, and promote the internalization of external effects to achieve the optimal allocation of resources. The higher the level of external incentives, the stronger the motivation of farmers to adopt soil testing and formulated fertilization technology. Thus, we propose the following hypothesis:
Hypothesis (H1): External incentives have a positive impact on farmers’ technology adoption behavior.
2.2.2 Intrinsic perception and technology adoption behavior
The influence of intrinsic perception on farmers’ soil testing and formulated fertilization technology adoption behavior. According to the theory of farmers’ behavior, under the competitive market mechanism, farmers’ behavioral decisions are fully rational and satisfy the principle of optimality. Whether a rational farmer accepts a new technology or decision depends on its technical characteristics and effects. The process of farmers’ perception and awareness of soil testing and formulated fertilization technology characteristics generally includes the assessment of technology usefulness, ease of use, and expected adoption costs, and intrinsic perception is a key psychological element for farmers to generate endogenous motivation to adopt green production technologies. In general, the stronger the intrinsic perception of the technology by farmers, the higher the likelihood of adopting soil testing and formulated fertilization technology. Thus, we propose the following hypothesis:
Hypothesis (H2): Farmers’ internal perception has a positive impact on technology adoption behavior.
2.2.3 Intrinsic perception, external incentives and formulated fertilization technology adoption behavior
The theory of behavioral economics believes that human decision-making behavior is uncertain, and the introduction of human psychological factors can scientifically and effectively analyze decision-making behavior. The basic point of the S-O-R theoretical model is that individuals will make corresponding behavioral responses to certain characteristics of the external environment, and the individual’s psychological cognition is the mediating factor that affects this process. Policy incentive tools or market incentives need to stimulate farmers’ subjective initiative by meeting the actual needs of farmers to increase income and efficiency, and internalize them into positive psychological perceptions, in order to further influence their technology adoption behavior. Thus, we propose the following hypothesis:
Hypothesis (H3): Intrinsic perception mediates between external incentives and soil testing and formulated fertilization technology adoption behavior.
2.2.4 Family endowment and technology adoption behavior
Family endowment is the factor resources and capabilities possessed by the whole household, which plays a significant role in individual behavioral choices and decisions. mmm technology will increase material input costs and labor costs in the short term, but the cost-benefit ratio cannot increase faster at the same time, and rational farmers will arrange their factor allocation behavior rationally according to their resource endowments such as labor, capital, and land. Therefore, individual and family characteristics and other endowment capabilities are the basic influencing factors of Dong et al. (2022)’s technology adoption, which determine farmers’ attitude and ability to adopt new technologies and ultimately affect the technology diffusion effect (Kong et al., 2021). Thus, we propose the following hypothesis:
Hypothesis (H4): The family endowment of farmers has a significant impact on technology adoption behavior.
2.2.5 Family endowment, external incentives and technology adoption behavior
Faced with the same external incentive, differences in family endowments lead to different responses from farmers and affect their soil testing and formulated fertilization technology adoption behavior. External incentives reflect the government’s long-term goal of improving land utilization and achieving green agricultural development, and do not directly improve farmers’ economic efficiency in a short period of time; farmers’ acceptance of external incentives depends on internal cognitive factors and preference factors. On the one hand, rich endowment resources mean that farmers have accumulated a large amount of production experience and knowledge, and have strong information acquisition and cognitive ability for policy incentives such as factor subsidies and technical training, which can reduce the learning cost of farmers and promote the effective transformation of farmers’ behavioral cognition into technical adoption. On the other hand, farmers with high family endowment have certain market risk tolerance and are willing to invest more costs to achieve high quality and good price for agricultural products, and are more likely to choose soil testing and formulated fertilization technology under market incentives. Therefore, the heterogeneity of family endowments can lead to a significant difference in the role of external incentives on soil testing and formulated fertilization technology adoption behavior. Thus, we propose the following hypothesis:
Hypothesis (H5): Family endowments play a moderating role in the relationship between external incentives and soil testing and formulated fertilization technology adoption behavior.
3 Methodology
3.1 Sample
China, the world’s most populous country, has the strictest cultivated land protection system for food security. In 2019, it issued a guideline on Strengthening the Construction of High-Standard farmland to Enhance the country’s ability to guarantee Food Security, which emphasized the use of environment-friendly fertilization technologies. The data in this paper come from a questionnaire survey conducted by the research team in Shaanxi Province from 2020 to 2021, and the research protocol uses a combination of stratified and random sampling to select the sample farmers. The first stage targeted grain-producing areas, i.e., sample counties were selected in the Guanzhong Plain and the Loess Plateau in northern Shaanxi based on factors such as rice grain and geographical location; the second stage aimed to find townships with large grain producers with the help of relevant agricultural departments in the sample counties; and the third stage was to find typical farmers in the selected townships. Finally, Yan’an, Yulin, Baoji, and Weinan cities in Shaanxi Province were selected as the sample areas. The group chose these study areas because, first, Shaanxi Province is located in the Guanzhong Plain region, backed by the Qinling Mountains, rich in water and fertile soil, and is an important grain-producing area in China. High-standard farmland projects are being built in recent years, which provides space for the development of soil testing and formulated fertilization technology. Second, according to previous research results, soil testing and formulated fertilization technology is more mature in the region and the sample data is more representative. The content of this survey mainly focused on the research of technology sources and communication, agricultural inputs and outputs, and ecological production behavior of environment-friendly arable land conservation technologies. A total of 325 questionnaires were obtained, and after eliminating invalid samples, 295 valid samples were obtained, with an effective rate of 90.8%.
3.2 Survey design
A questionnaire was designed, including 1) demographic characteristics; 2) evaluation of soil testing and formulated fertilization technology adoption predictors; and 3) evaluation of soil testing and formulated fertilization technology adoption implications. Each construct was measured using a validated research instrument developed by previous studies (modified to fit the research context) and based on the literature review findings and the theoretical foundation. A seven-point Likert scale was used to overcome measurement errors. The Likert scale ranges from “strongly disagree” (i.e., 1) to “strongly agree” (i.e., 7). Table 1 describes the used survey items.
3.3 Measures
Based on the existing research results, this paper selects four indicators of family endowment, such as planting scale, professional degree (the proportion of planting crop income in the total household income), total income and crop sales channel. The external incentives selected five indicators: organic matter soil improvement technology subsidy, the number of government-led technical training in the past year, whether the product has obtained the quality certification of “three products and one standard”, whether it has obtained the regional public brand, product brand, enterprise brand, etc. Intrinsic perception selects three indicators: usefulness awareness, ease of use perception and technology adoption cost. Six indicators were used to measure the technology adoption behavior (see Table 1).
All constructs were measured as mode A composites (Henseler and Schuberth, 2020). We analyzed the standard method to assess the quality of the information, which could improve the relationships between variables when collected from the same source. The analysis applying Harman’s single-factor test did not reveal that the variables were grouped into a single factor (Podsakoff and Organ, 1986), thus presenting no problems (Qalati et al., 2022).
3.4 Data analysis technique
Potential biases were considered in the survey, protocol design, and data analysis. Several approaches (e.g. direct contact by phone and assurance to share the results) were adopted to ensure the highest response rate and avoid a non-response bias (Frohlich and Westbrook, 2002). We used a partial least squares structural equation modelling (PLS-SEM) technique to analyse the data. This technique has been adopted because this process gives better results in the analysis of this type of exploratory study. This process can also analyse those data that are not normally distributed (Hair et al., 2012). This technique does not impose any sample restriction to conduct the survey. This process involves quantification of responses on a specific scale.
We tested our research model using partial least squares (PLS) and the SmartPLS package (v.3.3.3) (Ringle and Sarstedt, 2016). We used PLS for the following reasons. 1) The research model is complex, depending on the type of hypothesized relationships (direct, mediation, and moderation); 2) It has the advantage of not imposing distributional assumptions for the indicators, and 3) The constructs included are composite (Chin, 2010; Sarstedt et al., 2011). The model is also widely used to test theories using the dynamic capabilities approach (Ali et al., 2021; Ebrahimi et al., 2021; Frempong et al., 2021).
The application of the PLS technique involves several steps, including model fitting, applying a bootstrapping process (5,000 subsamples), following bootstrap-based exact fit texts for the estimated model (Henseler et al., 2016). Further, we evaluated the measurement model by analyzing the fit of the saturated model (Müller et al., 2018). Finally, the algebraic sign, magnitude, and statistical significance of the path coefficients were assessed and coefficients of determination (R2) were evaluated (Ali et al., 2018).
4 Results and analysis
4.1 Measurement model assessment
The reflective constructs were validated by testing internal consistency, composite reliability, convergent, and discriminant validity (Table 2 and Figure 2). To verify the internal consistency and composite reliability of the constructs, we verified that the value of Cronbach’s alpha and composite reliability indices exceeded 0.7 (Hair et al., 2011). This condition was valid for all the constructs. To test convergent validity, we verified that the average variance extracted (AVE) index was greater than 0.5. The lowest observed value (0.579) was substantially higher than this threshold. The discriminant validity of the reflective constructs was tested in three ways (Fornell and Larcker, 1981). The correlation matrix proved that the AVE was greater than the square correlation between each pair of latent constructs. These results suggest the validity of the reflective constructs used in our analysis and the adequacy of the items used as construct indicators. To determine the redundancy, the variance inflation factor (VIF) was used to test for multicollinearity among the different measurement variables, and the VIF values of the measurement models are shown in Table 1. The VIF values of all 18 observed variables were less than 5 (Hair et al., 2011; Ch’ng et al., 2021), indicating that there was no multicollinearity among the measurement variables. This study assesses discriminant validity using the Heterotrait-Monotrait (HTMT) ratio of correlations. As shown in Table 2, all HTMT values are below 0.85. Referring to Henseler et al. (2015), the results support the discriminant validity of the constructs. In sum, the robustness of measurement model was sound and appropriate for further structural equation modelling analyses.
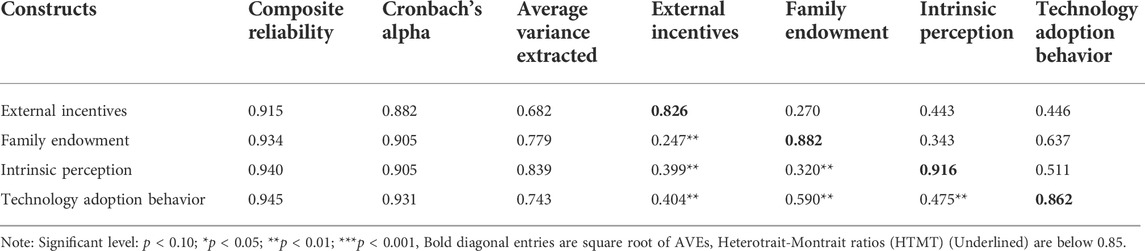
TABLE 2. Construct consistency, reliability, convergent and discriminant validity squared value of the AVE reported on the main diagonal of the correlation matrix.
4.2 Structural model assessment
As presented in Table 3, the relationships between technology adoption behavior (TAB) and among external incentives (EI), intrinsic perception (IP), and family endowment (FE), together with the relationship between EI and TAB, were found to be statistically significant. The moderating effects of FE on EI and TAB, was found to be not statistically significant. Specifically, EI, IP, and FE positively influenced on TAB at mean 0.184 (t = 2.741, p = 0.006**, CI = +/+), 0.244 (t = 4.925, p = 0.000***, CI = +/+), and 0.459 (t = 8.050, p = 0.000***, CI = +/+). Meanwhile, EI had a positive direct effect on IP at mean 0.399 (t = 6.546, p = 0.000***, CI = +/+). The relationship between EI and TAB became not significant under the moderating effect of FE at mean 0.040 (t = 0.532, p = 0.595NS, CI = −/+). Therefore, the results support hypotheses H1, H2, H3, and H4, but do not support H5.
The study assessed the model’s predictive power on the endogenous constructs using the coefficient of determination (R2). The results showed that the R-square value for construct IP was 0.159 (R2 adjusted = 0.156), TAB was 0.474 (R2 adjusted = 0.467). The R2 value ranges between 0 and 1, where values of 0.2, 0.5, and 0.75 indicate weak, moderate, and substantial effects, respectively (Hair et al., 2019). The R2 values for the present study’s model are moderate, with values 0.474 for TAB. Furthermore, the predictive relevance (Q2) of the endogenous latent variables were assured, as the IP and TAB were 0.118 (SSO = 885, SSE = 780.569) and 0.346 (SSO = 1,170, SSE = 1,157.585) respectively, which were all larger than zero (Hair et al., 2019). Figure 2 plots the results of the structural equation model calculations, and Table 3 shows the direct, indirect and total effect coefficients of external incentives, family endowments affecting intrinsic perceptions and farmers’ soil testing and formulated fertilization technology adoption behavior. These values can be considered as the predictive accuracy of the models among low, medium, and large (Hair et al., 2019). The analysis of the composite-based standardized root mean square residual (SRMR) yielded a value of 0.065, below the 0.10 threshold, which confirms the robustness of the model (Henseler et al., 2015).
4.3 Mediation model
To investigate the mediating effect of EI and TAB, the bootstrapping technique was employed to estimate the indirect effects. Table 3 and Figure 2 reveal that EI and IP positively and significantly affect TAB, directly and indirectly, with path coefficients of (β = 0.184, t = 2.741, p = 0.000***) and (β = 0.244, t = 4.925, p = 0.000***), respectively; therefore, H1 and H2 are supported. With regard to the estimation of the indirect effect of EI and TAB, the variance accounted for (VAF) formula was employed, where Total effect = Indirect effect + Direct effect. VAF values of 0.2<, 0.2–0.8, and >0.8 represent no mediation, partial mediation, and full mediation, respectively (Hair et al., 2019). The present study’s results evidence a 0.345 indirect effect of EI and TAB, respectively, which falls in the rage between 0.2 and 0.8, thus considered partial mediation.
4.4 Moderation model
This study tested the moderating role of EI and TAB as a complementary step for the structural model assessment and hypothesis testing. We did not find support for the moderating effect of FE on the relationship between EI and TAB (β = 0.040, t = 0.532, p = 0.595NS); therefore, H5 was not supported.
5 Discussion
The direct effect of external incentives on farmers’ technology adoption behavior. External incentives have a statistically significant positive effect on farmers’ technology adoption behavior at the 1% level with a path coefficient of 0.184, i.e., the more positive incentives farmers obtain, the more likely they are to adopt soil testing and formulated fertilization technology, and hypothesis H1 is verified. The incentive effect of government subsidies is to make up the difference between the extra cost of using the new technology and the expected benefit for farmers, and the more government subsidies, the more farmers are willing to adopt the new technology. The greater the number of technical trainings attended, the greater the chance for high-standard farmland grain farmers to receive direct technical services and support, and the lower the information cost of learning new technologies, which plays an important role in correcting farmers’ behavioral habits of excessive fertilizer application and raising their awareness of scientific fertilizer application. The “lemon effect” may prevent farmers from adding value to the high-quality agricultural products they produce, resulting in uncertain net returns. Market incentives can achieve market premiums for agricultural products, which is the interest driver for farmers to choose soil testing and formulated fertilization technology.
The effect of intrinsic perception on farmers’ technology adoption behavior. In Table 3, the path coefficient of intrinsic perception on adoption behavior was significant, and every 1 standard deviation increase in intrinsic perception would increase farmers’ technology adoption behavior by 0.244 standard deviations, and hypothesis H2 was verified. In the study, it was found that growers generally believed that the application of chemical fertilizers was fast and stable, while organic fertilizers and bacterial fertilizers were not as “powerful” as chemical fertilizers. Driven by the goal of profit maximization, some growers formed a preference for chemical fertilizers, and the stronger the perceived usefulness of the technology, the easier it was to master the new technology, and the lower the cost of technology adoption, the more motivated farmers were to adopt soil testing and formulated fertilization technology.
Intrinsic transmission mechanism of external incentives: the mediating role of intrinsic perceptions. The hypothesis H3 holds that external incentives have a significant positive effect on intrinsic perceptions and intrinsic perceptions have a significant positive effect on adoption behavior, and that intrinsic perceptions play a mediating role between external incentives and soil testing and formulated fertilization technology adoption behavior. This is fully consistent with the expected hypothesis of S-O-R theory. The coefficient of direct effect of external incentives on intrinsic perception is 0.399, the coefficient of direct effect of intrinsic perception on adoption behavior is 0.244, and the coefficient of indirect effect of external incentives on adoption behavior through intrinsic perception is 0.097. External incentives reduce the marginal cost of technology adoption to a certain extent, and make farmers fully realize that soil testing and formulated fertilization technology can bring long-term economic benefits to high-standard farming. The level of farmers’ intrinsic perceptions of technology adoption increased significantly, which eventually led to technology adoption behavior.
The effect of family endowment on farmers’ technology adoption behavior. The path coefficient of family endowment on adoption behavior is statistically significant at the 1% level, indicating that family endowment directly affects farmers’ technology adoption behavior, and hypothesis H4 is tested. Each 1 standard deviation increase in family endowment increased the adoption behavior of farmers by 0.459 standard deviations. Longer farming years and more experience of farmers imply more asset specificity of food on high standard farmland, more difficulty in switching to other crops, and more inclination to accept new technologies. Stable marketing channels make farmers pay more attention to the quality of high-standard farmland grain, creating conditions for the adoption of green production technologies. The application of organic fertilizer is a labor-intensive technology, and the lower the proportion of food income from high-standard farmland to total household income, the greater the opportunity cost for farmers to apply organic fertilizer and the less motivated they are to adopt soil testing and formulated fertilization technology. The more the scale effect generated by the expansion of planting scale can promote farmers’ choice of organic fertilizer application.
Inter-farmer variation: moderating role of family endowment. The interaction coefficient of external incentives and family endowment on adoption behavior is insignificant, indicating that family endowment does not play a moderating role in external incentives affecting farmers’ technology adoption behavior, i.e., family endowment heterogeneity does not lead to differential effects of external incentives on adoption behavior. The possible explanation for this is that in the historical process of rural revitalization and poverty alleviation, the government-led external incentives are universal policies, and the targets of implementation include high quality farmers with superior family endowments and poor households with poor resource endowments, and homogeneous and non-differentiated policy incentives cannot fundamentally stimulate different behavioral responses of farmers.
5.1 Theoretical implications
This study has been able to theorize the external incentives, intrinsic perception, and family endowment in farmers to unlock the development path of technology adoption behavior. This study has also theorized that such soil testing and formulated fertilization technology is compatible in the context of green development. For this, the help of a hybrid model, i.e., the integrated Stimulus-Organism-Response (S-O-R) model, has been taken. This hybrid model has been successfully used to explore farmers’ intention to adopt soil testing and formulated fertilization technology. Since few research studies are available to nurture the use of soil testing and formulated fertilization technology in farmers. This study provides a novel contribution in this context. This study has examined how psychological factor poses an impediment to adopting new technology in farmers, and it has been explained through this study how the S-O-R framework will help to interpret the situation. The reasons for using the S-O-R model have been explained in the theoretical background section. This study aims to explain what factors could influence the intention to adopt EAT, a component of green development. This study analyses the adoption of soil testing and formulated fertilization technology in farmers. In this context, this study could have used an updated standard adoption model. However, the study has ventured to select better-suited antecedents from S-O-R models.
5.2 Practical implications
This paper explains the mechanism of soil testing and formulated fertilization technology adoption behavior of grain growers in high-standard farmland to a certain extent, and mainly obtains the following three policy implications.
First, choose suitable and flexible incentives. The government’s “top-down” system supply will largely ignore the actual needs of farmers, so it is necessary to provide targeted policy supply, such as providing technical training at the initial stage of technology promotion, replacing classroom training with field experiments as far as possible, and accelerating the process of farmers’ mastery of new technology through “learning through work”.
Second, the higher management ability and stable economic income of farmers are the premise and guarantee of accepting new technology. The government in the implementation of the general policy of motivation at the same time, can’t ignore the audience heterogeneity characteristics of object, should focus on high-quality farmers tilt, to achieve the optimal allocation of factors, stimulate the radiating and driving function of the “new farmers".
Third, improve the intrinsic motivation of farmers to adopt technology. Farmers’ response to soil testing and formulated fertilization technology is indifferent and the adoption rate is low, which is due to their concern about the technology effect and adoption cost. We should give full play to the incentive effect of premium, motivate farmers to carry out product certification and brand registration, raise product prices, guarantee the economic interests and production enthusiasm of farmers participating in green production, and form an endogenous long-term mechanism for farmers to adopt green production technology.
5.3 Limitations and future research
Based on the S-O-R theory, this paper discusses the mechanism of EI,PE, FE on TAB, and draws beneficial conclusions, but there are still the following shortcomings: first, the influence of PE,EI, FE on TAB may have configuration effect, which can be further analyzed by fsQCA method in the future; Second, the samples are mainly from farmers, and different groups such as rural cooperatives or agricultural complexes can be considered. The universality of the research conclusions needs to be further tested. Third, the analysis of farmers’ technology acceptance behavior based on S-O-R needs to be further discussed through case studies.
6 Conclusion
In this study, family endowment, external motivation, internal perception and adoption behavior were incorporated into the extended S-O-R theoretical framework, and the data of 295 major high-standard farmland grain-producing areas in Shaanxi Province were used. The structural equation model of formative indicators was constructed based on partial least square method to estimate the direct influence of external incentives on farmers’ soil testing and formulated fertilization technology adoption behavior, as well as the mediating role of internal perception and the moderating role of family endowment. The research conclusions are as follows:
First, external incentives have a significant positive impact on farmers’ technology adoption behavior at the statistical level of 10%, that is, the more positive incentives farmers get, the more likely they are to adopt soil testing and formulated fertilization technology.
Second, the path coefficient of intrinsic perception on adoption behavior is significant, indicating that the latent variables of intrinsic perception, usability perception and technology adoption cost are the key factors determining adoption behavior. Internal perception plays a mediating role between external incentive and soil testing and formulated fertilization technology adoption behavior, which further confirms that in S-O-R theory, external environmental characteristics will act on internal perception and finally appear as farmers’ technology adoption behavior.
Third, the characteristics of farmers’ family endowment have a direct effect on soil testing and formulated fertilization technology adoption behavior. The interaction term between external incentives and family endowment has no significant path coefficient on soil testing and formulated fertilization technology adoption behavior, indicating that family endowment does not play a moderating role in the influence of external incentives on soil testing and formulated fertilization technology adoption behavior.
Data availability statement
The original contributions presented in the study are included in the article/Supplementary Material, further inquiries can be directed to the corresponding author.
Author contributions
HD, LL, JH, and HW: methodology and software. LZ, YD, and MD formal analysis. XC and YZ resources and data curation. XZ and LW: writing review and editing. HD: investigation. HD: writing original draft preparation, supervision and project administration. All authors have read and agreed to the published version of the manuscript.
Funding
Research on intelligent technology consultation service system driven by big data (2020ZDLGY09-05) AND Research, Development and Application of Dynamic Monitoring and Early Warning Technology for “Non-agriculturalization and Non-foodization” of Grain Fields (2022ZDLNY02-10).
Conflict of interest
HD, BW, JH, LL, HW, ZS, LZ, MD, XC and YZ were employed by Shaanxi Provincial Land Engineering Construction Group Co., Ltd.
Publisher’s note
All claims expressed in this article are solely those of the authors and do not necessarily represent those of their affiliated organizations, or those of the publisher, the editors and the reviewers. Any product that may be evaluated in this article, or claim that may be made by its manufacturer, is not guaranteed or endorsed by the publisher.
References
Adesemoye, A. O., and Kloepper, J. W. (2009). Plant–microbes interactions in enhanced fertilizer-use efficiency. Appl. Microbiol. Biotechnol. 85, 1–12. doi:10.1007/s00253-009-2196-0
Ahmad, B., Da, L., Asif, M. H., Irfan, M., Ali, S., and Akbar, M. I. U. D. (2021). Understanding the antecedents and consequences of service-sales ambidexterity: A motivation-opportunity-ability (MOA) framework. Sustainability 13, 9675. doi:10.3390/su13179675
Ali, F., Rasoolimanesh, S. M., Sarstedt, M., Ringle, C. M., and Ryu, K. (2018). An assessment of the use of partial least squares structural equation modeling (PLS-SEM) in hospitality research. Int. J. Contemp. Hosp. Mngt. 30, 514–538. doi:10.1108/IJCHM-10-2016-0568
Ali, S., Javed, H. M. U., and Danish, M. (2021). Adoption of green IT in Pakistan: a comparison of three competing models through model selection criteria using PLS-SEM. Environ. Sci. Pollut. Res. 28, 36174–36192. doi:10.1007/s11356-020-12163-3
Atari, D. O. A., Yiridoe, E. K., Smale, S., and Duinker, P. N. (2009). What motivates farmers to participate in the nova scotia environmental farm plan program? Evidence and environmental policy implications. J. Environ. Manag. 90, 1269–1279. doi:10.1016/j.jenvman.2008.07.006
Bopp, C., Engler, A., Poortvliet, P. M., and Jara-Rojas, R. (2019). The role of farmers’ intrinsic motivation in the effectiveness of policy incentives to promote sustainable agricultural practices. J. Environ. Manag. 244, 320–327. doi:10.1016/j.jenvman.2019.04.107
Bravo-Monroy, L., Potts, S. G., and Tzanopoulos, J. (2016). Drivers influencing farmer decisions for adopting organic or conventional coffee management practices. Food Policy 58, 49–61. doi:10.1016/j.foodpol.2015.11.003
Ch’ng, P.-C., Cheah, J., and Amran, A. (2021). Eco-innovation practices and sustainable business performance: The moderating effect of market turbulence in the Malaysian technology industry. J. Clean. Prod. 283, 124556. doi:10.1016/j.jclepro.2020.124556
Chen, J. (2007). Rapid urbanization in China: A real challenge to soil protection and food security. CATENA 69, 1–15. doi:10.1016/j.catena.2006.04.019
Chin, W. W. (2010). “How to write up and report PLS analyses,” in Handbook of partial least squares. Editors V. Esposito Vinzi, W. W. Chin, J. Henseler, and H. Wang (Berlin, Heidelberg: Springer Berlin Heidelberg), 655–690. doi:10.1007/978-3-540-32827-8_29
Clark, C. F., Kotchen, M. J., and Moore, M. R. (2003). Internal and external influences on pro-environmental behavior: Participation in a green electricity program. J. Environ. Psychol. 23, 237–246. doi:10.1016/S0272-4944(02)00105-6
Daxini, A., O’Donoghue, C., Ryan, M., Buckley, C., Barnes, A. P., and Daly, K. (2018). Which factors influence farmers’ intentions to adopt nutrient management planning? J. Environ. Manag. 224, 350–360. doi:10.1016/j.jenvman.2018.07.059
De Fano, A., Leshem, R., and Ben-Soussan, T. D. (2019). Creating an internal environment of cognitive and psycho-emotional well-being through an external movement-based environment: An overview of quadrato motor training. Int. J. Environ. Res. Public Health 16, 2160. doi:10.3390/ijerph16122160
Dong, H., Wang, H., and Han, J. (2022). Understanding ecological agricultural technology adoption in China using an integrated technology acceptance model—theory of planned behavior model. Front. Environ. Sci. 10, 927668. doi:10.3389/fenvs.2022.927668
Ebrahimi, P., Hamza, K. A., Gorgenyi-Hegyes, E., Zarea, H., and Fekete-Farkas, M. (2021). Consumer knowledge sharing behavior and consumer purchase behavior: Evidence from E-commerce and online retail in Hungary. Sustainability 13, 10375. doi:10.3390/su131810375
Elmustapha, H., Hoppe, T., and Bressers, H. (2018). Consumer renewable energy technology adoption decision-making; comparing models on perceived attributes and attitudinal constructs in the case of solar water heaters in Lebanon. J. Clean. Prod. 172, 347–357. doi:10.1016/j.jclepro.2017.10.131
Faruque-As-Sunny, , Huang, Z., and Karimanzira, T. T. P. (2018). Investigating key factors influencing farming decisions based on soil testing and fertilizer recommendation facilities (STFRF)—a case study on rural Bangladesh. Sustainability 10, 4331. doi:10.3390/su10114331
Fornell, C., and Larcker, D. F. (1981). Structural equation models with unobservable variables and measurement error: Algebra and statistics. J. Mark. Res. 18, 382–388. doi:10.1177/002224378101800313
Frempong, M. F., Mu, Y., Adu-Yeboah, S. S., Hossin, M. A., and Adu-Gyamfi, M. (2021). Corporate sustainability and firm performance: The role of green innovation capabilities and sustainability-oriented supplier–buyer relationship. Sustainability 13, 10414. doi:10.3390/su131810414
Frohlich, M. T., and Westbrook, R. (2002). Demand chain management in manufacturing and services: web-based integration, drivers and performance. J. Oper. Manag. 20, 729–745. doi:10.1016/S0272-6963(02)00037-2
Godfray, H. C. J., and Garnett, T. (2014). Food security and sustainable intensification. Phil. Trans. R. Soc. B 369, 20120273. doi:10.1098/rstb.2012.0273
Hair, J. F., Ringle, C. M., and Sarstedt, M. (2011). PLS-SEM: Indeed a silver bullet. J. Mark. Theory Pract. 19, 139–152. doi:10.2753/MTP1069-6679190202
Hair, J. F., Sarstedt, M., Ringle, C. M., and Mena, J. A. (2012). An assessment of the use of partial least squares structural equation modeling in marketing research. J. Acad. Mark. Sci. 40, 414–433. doi:10.1007/s11747-011-0261-6
Hair, J. F., Risher, J. J., Sarstedt, M., and Ringle, C. M. (2019). When to use and how to report the results of PLS-SEM. Eur. Bus. Rev. 31, 2–24. doi:10.1108/EBR-11-2018-0203
Henseler, J., and Schuberth, F. (2020). Using confirmatory composite analysis to assess emergent variables in business research. J. Bus. Res. 120, 147–156. doi:10.1016/j.jbusres.2020.07.026
Henseler, J., Ringle, C. M., and Sarstedt, M. (2015). A new criterion for assessing discriminant validity in variance-based structural equation modeling. J. Acad. Mark. Sci. 43, 115–135. doi:10.1007/s11747-014-0403-8
Henseler, J., Ringle, C. M., and Sarstedt, M. (2016). Testing measurement invariance of composites using partial least squares. Int. Mark. Rev. 33, 405–431. doi:10.1108/IMR-09-2014-0304
Jordan-Meille, L., Rubaek, G. H., Ehlert, P. A. I., Genot, V., Hofman, G., Goulding, K., et al. (2012). An overview of fertilizer-P recommendations in europe: soil testing, calibration and fertilizer recommendations: P fertilizer methods in europe. Soil Use Manag. 28, 419–435. doi:10.1111/j.1475-2743.2012.00453.x
Kamboj, S., Sarmah, B., Gupta, S., and Dwivedi, Y. (2018). Examining branding co-creation in brand communities on social media: Applying the paradigm of Stimulus-Organism-Response. Int. J. Inf. Manag. 39, 169–185. doi:10.1016/j.ijinfomgt.2017.12.001
Kong, F., Sun, S., and Wang, Y. (2021). Comprehensive understanding the disaster-causing mechanism, governance dilemma and targeted countermeasures of urban pluvial flooding in China. Water 13, 1762. doi:10.3390/w13131762
Li, B., Li, P., Zeng, X. C., Yu, W., Huang, Y. F., Wang, G. Q., et al. (2020a). Assessing the sustainability of phosphorus use in China: Flow patterns from 1980 to 2015. Sci. Total Environ. 704, 135305. doi:10.1016/j.scitotenv.2019.135305
Li, J., Feng, S., Luo, T., and Guan, Z. (2020b). What drives the adoption of sustainable production technology? Evidence from the large scale farming sector in east China. J. Clean. Prod. 257, 120611. doi:10.1016/j.jclepro.2020.120611
Liu, X., Zhao, C., and Song, W. (2017). Review of the evolution of cultivated land protection policies in the period following China’s reform and liberalization. Land Use Policy 67, 660–669. doi:10.1016/j.landusepol.2017.07.012
Liu, Y., Ruiz-Menjivar, J., Zhang, L., Zhang, J., and Swisher, M. E. (2019). Technical training and rice farmers’ adoption of low-carbon management practices: The case of soil testing and formulated fertilization technologies in Hubei, China. J. Clean. Prod. 226, 454–462. doi:10.1016/j.jclepro.2019.04.026
Marenya, P. P., and Barrett, C. B. (2007). Household-level determinants of adoption of improved natural resources management practices among smallholder farmers in Western Kenya. Food Policy 32, 515–536. doi:10.1016/j.foodpol.2006.10.002
Mazvimavi, K., and Twomlow, S. (2009). Socioeconomic and institutional factors influencing adoption of conservation farming by vulnerable households in Zimbabwe. Agric. Syst. 101, 20–29. doi:10.1016/j.agsy.2009.02.002
Mendola, M. (2008). Migration and technological change in rural households: Complements or substitutes? J. Dev. Econ. 85, 150–175. doi:10.1016/j.jdeveco.2006.07.003
Meng, Q., Hou, P., Wu, L., Chen, X., Cui, Z., and Zhang, F. (2013). Understanding production potentials and yield gaps in intensive maize production in China. Field Crops Res. 143, 91–97. doi:10.1016/j.fcr.2012.09.023
Müller, T., Schuberth, F., and Henseler, J. (2018). PLS path modeling – a confirmatory approach to study tourism technology and tourist behavior. JHTT 9, 249–266. doi:10.1108/JHTT-09-2017-0106
Onwezen, M. C., Antonides, G., and Bartels, J. (2013). The Norm Activation Model: An exploration of the functions of anticipated pride and guilt in pro-environmental behaviour. J. Econ. Psychol. 39, 141–153. doi:10.1016/j.joep.2013.07.005
Podsakoff, P. M., and Organ, D. W. (1986). Self-reports in organizational research: Problems and prospects. J. Manag. 12, 531–544. doi:10.1177/014920638601200408
Pretty, J., Brett, C., Gee, D., Hine, R., Mason, C., Morison, J., et al. (2001). Policy challenges and priorities for internalizing the externalities of modern agriculture. J. Environ. Plan. Manag. 44, 263–283. doi:10.1080/09640560123782
Qalati, S. A., Ostic, D., Sulaiman, M. A. B. A., Gopang, A. A., and Khan, A. (2022). Social media and SMEs’ performance in developing countries: Effects of technological-organizational-environmental factors on the adoption of social media. SAGE Open 12, 215824402210945. doi:10.1177/21582440221094594
Qi, X., Liang, F., Yuan, W., Zhang, T., and Li, J. (2021). Factors influencing farmers’ adoption of eco-friendly fertilization technology in grain production: An integrated spatial–econometric analysis in China. J. Clean. Prod. 310, 127536. doi:10.1016/j.jclepro.2021.127536
Rezaei, R., Safa, L., and Ganjkhanloo, M. M. (2020). Understanding farmers’ ecological conservation behavior regarding the use of integrated pest management- an application of the technology acceptance model. Glob. Ecol. Conserv. 22, e00941. doi:10.1016/j.gecco.2020.e00941
Ringle, C. M., and Sarstedt, M. (2016). Gain more insight from your PLS-SEM results: The importance-performance map analysis. IMDS 116, 1865–1886. doi:10.1108/IMDS-10-2015-0449
Sarstedt, M., Henseler, J., and Ringle, C. M. (2011). “Multigroup Analysis in partial least squares (PLS) path modeling: Alternative methods and empirical results,” in Advances in international marketing. Editors M. Sarstedt, M. Schwaiger, and C. R. Taylor (Emerald Group Publishing Limited), 195–218. doi:10.1108/S1474-7979(2011)0000022012
Smith, L. E. D., and Siciliano, G. (2015). A comprehensive review of constraints to improved management of fertilizers in China and mitigation of diffuse water pollution from agriculture. Agric. Ecosyst. Environ. 209, 15–25. doi:10.1016/j.agee.2015.02.016
Smith, V. H., Tilman, G. D., and Nekola, J. C. (1999). Eutrophication: impacts of excess nutrient inputs on freshwater, marine, and terrestrial ecosystems. Environ. Pollut. 100, 179–196. doi:10.1016/S0269-7491(99)00091-3
Song, G., Min, S., Lee, S., and Seo, Y. (2017). The effects of network reliance on opportunity recognition: A moderated mediation model of knowledge acquisition and entrepreneurial orientation. Technol. Forecast. Soc. Change 117, 98–107. doi:10.1016/j.techfore.2017.01.004
Stewart, W. M., Dibb, D. W., Johnston, A. E., and Smyth, T. J. (2005). The contribution of commercial fertilizer nutrients to food production. Agron. J. 97, 1–6. doi:10.2134/agronj2005.0001
Swinton, S. M., Lupi, F., Robertson, G. P., and Hamilton, S. K. (2007). Ecosystem services and agriculture: Cultivating agricultural ecosystems for diverse benefits. Ecol. Econ. 64, 245–252. doi:10.1016/j.ecolecon.2007.09.020
Tey, Y. S., and Brindal, M. (2012). Factors influencing the adoption of precision agricultural technologies: a review for policy implications. Precis. Agric. 13, 713–730. doi:10.1007/s11119-012-9273-6
Truelove, H. B., Carrico, A. R., Weber, E. U., Raimi, K. T., and Vandenbergh, M. P. (2014). Positive and negative spillover of pro-environmental behavior: An integrative review and theoretical framework. Glob. Environ. Change 29, 127–138. doi:10.1016/j.gloenvcha.2014.09.004
Vieira, V. A. (2013). Stimuli–organism-response framework: A meta-analytic review in the store environment. J. Bus. Res. 66, 1420–1426. doi:10.1016/j.jbusres.2012.05.009
Wonglimpiyarat, J., and Yuberk, N. (2005). In support of innovation management and Roger’s Innovation Diffusion theory. Gov. Inf. Q. 22, 411–422. doi:10.1016/j.giq.2005.05.005
Wu, Y., Shan, L., Guo, Z., and Peng, Y. (2017). Cultivated land protection policies in China facing 2030: dynamic balance system versus basic farmland zoning. Habitat Int. 69, 126–138. doi:10.1016/j.habitatint.2017.09.002
Xin, L. (2022). Chemical fertilizer rate, use efficiency and reduction of cereal crops in China, 1998–2018. J. Geogr. Sci. 32, 65–78. doi:10.1007/s11442-022-1936-2
Yan, X., Jin, J., He, P., and Liang, M. (2008). Recent advances on the technologies to increase fertilizer use efficiency. Agric. Sci. China 7, 469–479. doi:10.1016/S1671-2927(08)60091-7
Yang, H., and Li, X. (2000). Cultivated land and food supply in China. Land Use Policy 17, 73–88. doi:10.1016/S0264-8377(00)00008-9
Yang, Y., He, Y., and Li, Z. (2020). Social capital and the use of organic fertilizer: an empirical analysis of hubei Province in China. Environ. Sci. Pollut. Res. 27, 15211–15222. doi:10.1007/s11356-020-07973-4
Keywords: stimulus-organism-response (S-O-R), China, eco-friendly fertilization technology, intention to adopt, PLS-SEM
Citation: Dong H, Wang B, Han J, Luo L, Wang H, Sun Z, Zhang L, Dai M, Cheng X and Zhao Y (2022) Understanding farmers’ eco-friendly fertilization technology adoption behavior using an integrated S-O-R model: The case of soil testing and formulated fertilization technology in shaanxi, china. Front. Environ. Sci. 10:991255. doi: 10.3389/fenvs.2022.991255
Received: 11 July 2022; Accepted: 25 August 2022;
Published: 27 September 2022.
Edited by:
Asif Razzaq, Ilma University, PakistanReviewed by:
Sikandar Ali Qalati, Jiangsu University, ChinaApurbo Sarkar, The University of Queensland, Australia
Monica Lorena Sanchez Limon, Autonomous University of Tamaulipas, Mexico
Hua Lu, Jiangxi University of Finance and Economics, China
Copyright © 2022 Dong, Wang, Han, Luo, Wang, Sun, Zhang, Dai, Cheng and Zhao. This is an open-access article distributed under the terms of the Creative Commons Attribution License (CC BY). The use, distribution or reproduction in other forums is permitted, provided the original author(s) and the copyright owner(s) are credited and that the original publication in this journal is cited, in accordance with accepted academic practice. No use, distribution or reproduction is permitted which does not comply with these terms.
*Correspondence: Hao Dong, mail:donghao19@stu.xjtu.edu.cn; Jichang Han, hanjc_sxdj@126.com