- 1College of Geography and Environmental Science, Northwest Normal University, Lanzhou, China
- 2Key Laboratory of Resource Environment and Sustainable Development of Oasis, Lanzhou, China
- 3School of Ecological and Environmental Science, East China Normal University, Shanghai, China
- 4College of Minning, Guizhou University, Guiyang, China
Studying the internal relationship between the innovation environment and the transformation development of resource-based cities from the perspective of coupling and coordination helps reveal the mechanism between them, which is of great significance to sustainable development of resource-based cities. This study reconstructs the index system for innovative environment and transformation development. We used the coupling coordination degree model, Epanechnikov function, and panel quantile regression model to analyze the coupling coordination level, dynamic evolution characteristics, and influencing factors of innovative environment and transformation development of resource-based cities in Gansu Province from 2009 to 2019. The study shows that the innovation environment index and the transformation development index of resource-based cities increased significantly during the study period. The innovation environment index of resource-based cities has been greatly improved, showing a dynamic evolution trend of rapid growth and development characteristics of “low-level, fast growth”. There are differences in the characteristics of transformation development in resource-based cities, but on the whole, it shows a trend of increasing fluctuation. The state of coupling coordination has changed from a severely unbalanced state to a moderately unbalanced state; some cities have turned to a low-level coordination state, and the overall trend is presented to optimize the process continuously. The quantile regression results show that the level of economic development and industrial structure has a significant positive effect on improving the coupling coordination degree of the two. The degree of government intervention has a negative impact. The level of capital investment has a negative effect at a high score but has no significant impact at a low score. This study tries to provide a scientific basis for formulating the policy of coordinated development between an innovation environment and transformation development of resource-based cities and also provides a reference for high-quality development of other resource-based cities.
1 Introduction
Resource-based cities are defined as cities built or developed into leading industries relying on the region’s exploitation and processing of natural resources, such as minerals and forests (Li et al., 2013; Yu et al., 2015; Chen and Zhang 2021). Since the founding of New China, resource-based cities, as an essential energy supply base in China, have made historic contributions to formation of the national industrialization development system and the map of industrialization development (Li and Dewan 2017; Li et al., 2020). Under economic globalization and dynamic population transfer, world GDP and CO2 emissions are moving eastward. Currently, China has shown a certain phenomenon of economic maturity, but the consumption efficiency of resources and energy is relatively high (Balsa-Barreiro et al., 2019), which puts pressure on sustainable development of resource-based cities. The report to the 19th National Congress of the Communist Party of China (2017) pointed out that China’s economic development has shifted to a stage of high-quality development (Deng et al., 2022), The key to high-quality development of resource-based cities is how to achieve the transformation and upgrading of industrial structure and effective transformation of old and new driving forces, transforming the original model of scale expansion led by natural resources and at the expense of the environment sacrifice into a model of high-quality development driven by technological innovation, changing from factor-driven development to innovation-driven development (Jiang et al., 2020) to overcome the instability problem in the development of resource-based cities. Resource-based cities have strong resource dependence and development inertia, and forming industrial development path dependence is easy (Zhang and Brouwer 2020), which leads to failure of resource-abundance cities to play a good “Resource Gospel” role in the high-quality development of the regional economy. On the contrary, the phenomenon of “Resource Curse”, which is negatively related to regional economic development, is likely to occur to a certain extent (Sachs and Warner 1995), causing regional resources to dry up, deteriorating ecological environment, and lack of economic industry development and sustainable urban development problems (Hooved 2012; Martinez-Fernandez et al., 2012). Also, the challenges and transformation of sustainable development of resource-based cities in China have become core issues (Chen et al., 2018). In addition, the essence of the economic transformation and development of resource-based cities is to guide the transformation of economic structure and reduce the dependence on resource-based cities’ industries (Kuai et al., 2015; Chen et al., 2018; Zhang et al., 2020). Transformation and upgrading of resource-based cities are not simply about economic development but the leapfrog development of regional economies caused by technological progress and institutional changes. Therefore, exploring a path for renewal and breakthroughs is an inevitable problem faced by resource-based cities.
The regional innovation environment plays a vital role in promoting regional industrial development or economic development of the whole region (Maurseth and Verspagen 2002; Hasan and Tucci 2010), such as building a high-quality regional innovation system, creating a suitable environment for regional technological innovation, and enhancing regional innovation capabilities, which are the fundamental ways to improve regional transformation development capabilities and regional competitiveness significantly (Gong 2021). A review of the successful transformation development experiences of resource-based cities in the world shows that regional transformation development cannot be separated from the construction and support of a regional innovation environment. As a supportive environment for innovation activities, the regional innovation environment promotes socio-economic development by expanding the regional innovation scale, increasing innovation activity quantity, and improving innovation efficiency, ultimately realizing urban transformation upgrading and sustainable development. Resource-based cities in the process of development need to rely on a regional innovation system and a regional innovation environment to constantly explore new economic growth points and growth poles, explore new models of regional development paths, and unlock realization of the creation of a new way of regional industry evolution, thus promoting the whole area into a new high-quality economic development orbit to achieve high-quality sustainable development.
The research on the innovation environment and transformation development of resource-based cities has become a hot topic in the academic circle with great achievements. Presently, some research studies on the innovation environment and transformation development of resource-based cities mainly focuses on the following aspects: 1) pay attention to the impact of the innovation environment on the transformation development process of resource-based cities. For example, the calculation of the innovation environment index shows that the urban innovation environment has a positive role in promoting the industrial transformation development of resource-based cities (Li and Lin 2018). Technological innovation improves resource utilization efficiency through technological progress, thus promoting the transformation development of resource-based cities (Porter 1991). Also, on this basis, some studies discuss the influence of the government institutional environment (Shao et al., 2021), science and technology environment (Xie et al., 2020), and network facility environment (Zhu et al., 2021) on the transformation development of resource-based cities. 2) Focus on evaluating innovation environment and transformation development level, collaborative development path, and countermeasures. For example, a comprehensive evaluation index system was constructed to measure the level of urban transformation development (Chen et al., 2018; Liu et al., 2021) and innovation environment (Chen and Zhang 2021). On this basis, some scholars combine the innovation environment and transformation development to explore the coupling process of resource-based cities (Chen and Zhang 2021), further putting forward synergistic development paths (Xing and Luo 2018; Wang Q. et al., 2021) and development countermeasures (Li et al., 2013; Yu et al., 2016; Yan et al., 2019). 3) Attribution analysis of regional transformation development level caused by innovation environment change in resource-based cities, and analysis of its influence effect and driving mechanism. For example, regional technological innovation plays a positive role in the green transformation development of resource-based cities, and technological innovation plays a more significant role in urban transformation development and promotes the technical innovation path of regional transformation (Xie et al., 2020). The regional innovation environment continues to promote regional economic development and plays a crucial role in upgrading urban industrial structure through technological innovation knowledge and a technological spillover effect (Doytch and Narayan 2016). The innovation environment can promote resource cities’ green industrial transformation by improving traditional industries’ production technology and resource utilization efficiency (Li and Lin 2018; Miao et al., 2018). Other studies have shown that the increase in revenue from the resource sector will cause innovators to prefer the resource sector over the R&D sector (Sachs and Warner 2001), and the crowding effect of innovation may reduce the efficiency of regional resource utilization (Sun et al., 2017), thus affecting regional transformation development. Jin et al. (2019) also confirmed that technological innovation restricts the green transformation development of regional industries. In summary, from the perspective of regional distribution, existing research on resource-based cities is mainly concentrated in the whole country, northeast China, and Shanxi Province. At the same time, there are few relevant research results on resource-based cities in northwest China, especially in Gansu province. Therefore, conducting relevant research on resource-based cities in Gansu province is significant.
The main purpose of this study is to demonstrate the coupling coordination between the innovation environment and transformation development of resource-based cities in Gansu Province from 2009 to 2019. The objectives are proposed at three levels: 1) a coupling mechanism between innovation environment and transformation development is analyzed. 2) The coupling coordination degree of resource-based cities in Gansu province is measured. 3) Explore the influencing factors of coupling coordination degree of resource-based cities in Gansu Province. Possible academic contributions to this study are as follows: The coupling mechanism of regional innovation environment and transformation development is expounded, and a mathematical model is used to explore the influencing factors of coupling coordination degree, which enriches the research on coupling coordination between innovation environment and transformation development of resource-based cities.
2 Coupling mechanism of regional innovation environment and transformation development
There is a close interaction between the regional innovation environment and regional transformation development (Appio et al., 2021). The regional innovation environment provides sufficient conditions for regional innovation development, is the main driving force for regional transformation development, and is critical to enhancing regional sustainable development (Fang et al., 2014) so that the regional economy can develop healthily and with high quality. Regional innovation environment refers to the sum of external factors that influence the various innovation activities of regional innovation subjects in a specific region in the process of innovation development. It mainly includes the planning and strategy of the state for regional innovation development; the diversified investment of the state in regional innovation development; and the social attitude to innovation behavior and so on. Based on the perspective of innovation environment elements, this study considers that the regional innovation environment is the concentrated embodiment of regional innovation development based on the infrastructure environment, driven by the human resource environment, guaranteed by the financial market environment, keyed by the technology service environment, and mainlined by the policy institutional environment. At the same time, the innovation environment is also a concrete representation of the leading power of emerging industries, the influence of scientific and technological achievements, and the gathering power of innovation resources. Regional transformation development is mainly reflected in three aspects: economy, society, and ecology, that is, to realize scientific control and high unity between economic development and environmental protection, natural ecology and human ecology, and people’s livelihood and social development in the complex system of economy–society–ecology (Figure 1).
The response degree of regional transformation development caused by the change in the regional innovation environment reflects the fit degree of the regional innovation environment for regional transformation development. The level of the regional innovation environment has been improved effectively, and the speed of regional transformation development has been fast, which reflects that regional transformation development has a solid response to improvement of the regional innovation environment, and vice versa. The regional innovation environment gathers innovative elements such as knowledge, capital, and technology in multiple ways and constantly forms new technologies and products in a region, which promotes high-level rationalization and modernization of regional industrial development and then promotes the development of regional transformation and upgrading. Regional transformation development can effectively promote the improvement of the regional innovation environment, and the regional innovation environment is the guaranteeing factor for improving regional innovation ability, which has a positive effect on the improvement of regional innovation ability. Regional transformation development can not only promote high-quality development of the regional economy but also provide the development foundation for the regional innovation environment, optimize the regional innovation environment, form the regional innovation atmosphere, improve the regional innovation output, and then feed the regional transformation development. Therefore, the regional innovation environment and regional transformation development promote each other and develop cooperatively.
On the one hand, the regional innovation environment can improve technical routes, workflows, and process equipment of the original production, thus forming a new industrial mode based on digitalization, intelligence, green, and security, extended by networking, personalization, and service. The regional innovation environment can effectively induce the emergence of new industries, new technologies, new forms of business, and new models and then promote regional transformation development. A regional innovation environment effectively aggregates innovation resources, accelerates the pace of regional transformation and upgrading, and elevates regional transformation development. In addition, the regional innovation environment can promote industry (chain) technology level to continuously improve, drive the deep integration of innovative technologies and traditional industries, and promote industry from low value-added links to high value-added links to ascend, laying a solid foundation maintenance industry (chain) stable industry and changing the development mode and path of regional industry. On the other hand, regional transformation development will stimulate the regional demand for the innovation of new technology and new methods, and the innovation demand cannot leave the fertile soil of the innovation environment. The surge in innovation demand will prompt the government and enterprises to invest a large amount of human, material, and financial resources in quantity and quality, which will play a positive role in promoting the transformation of old and new industrial driving forces and upgrading the economic structure. Regional transformation development can effectively improve the level of the regional innovation environment. The transformation development will put higher requirements on regional infrastructure, capital resources, institutions, and policies; will prompt the government to perfect relevant policy support; increase diversified capital investment; and improve the regional innovation environment. At the same time, regional transformation development will drive the accumulation of more talents, technology, capital, facilities, and other resources, providing a good development environment for regional innovation. Regional transformation development can improve regional innovation output, and its essence is to enhance the process of industrial evolution and change, aiming at product economic added value and product competitiveness. Therefore, resource-based cities should coordinate and integrate innovation environments and transformation development to make resource-based cities green and sustainable.
3 Study area and data source
3.1 Study area
Gansu province is a vital hinge province in northwest China and an important economic growth pole in western China. The GDP of Gansu province increased from 326.83 billion yuan in 2009 to 871.83 billion yuan in 2019, showing a fast economic growth rate. In terms of strategic position, it is an important transportation hub and a financial channel connecting central and western China with eastern China and numerous trunk railways and national highways. Gansu province is located at the intersection of the Qinghai–Tibet Plateau, Loess Plateau, and Inner Mongolia Plateau. The landforms within Gansu province are complex and diverse, including mountains, plateaus, plains, river valleys, deserts, and gobi; with complete types and staggered distribution, Gansu province is representative in terms of natural conditions. But, the economic development of resource-based cities in Gansu province is slow, and the transformation development is difficult. From 2009 to 2019, the GDP of resource-based cities in the province increased from 148.52 billion yuan in 2009 to 340.84 billion yuan in 2019, with an average annual growth rate of 11.77%. However, the proportion of resource-based cities in the province’s GDP decreased from 43.24% in 2009 to 39.09% in 2019. According to the National Sustainable Development Plan for Resource-Based Cities (2013–2020) issued by the State Council in December 2013 (Council 2013), Gansu Province involves seven resource-based cities, as shown in Figure 2.
3.2 Construction of the evaluation index system
The comprehensive evaluation index system of resource-based city innovation environments selects 23 evaluation indexes from five aspects: infrastructure environment, human resource environment, financial market environment, technology service environment, and policy institutional environment. The goal of the transformation development of resource-based cities is to get rid of excessive dependence of economic development on resources to achieve high-quality sustainable development of the cities. The transformation development situation mainly selects 13 evaluation indexes from three aspects: economic transformation development, social transformation development, and ecological transformation development. Finally, the comprehensive evaluation index system of the innovation environment and transformation development of resource-based cities is obtained (Table 1).
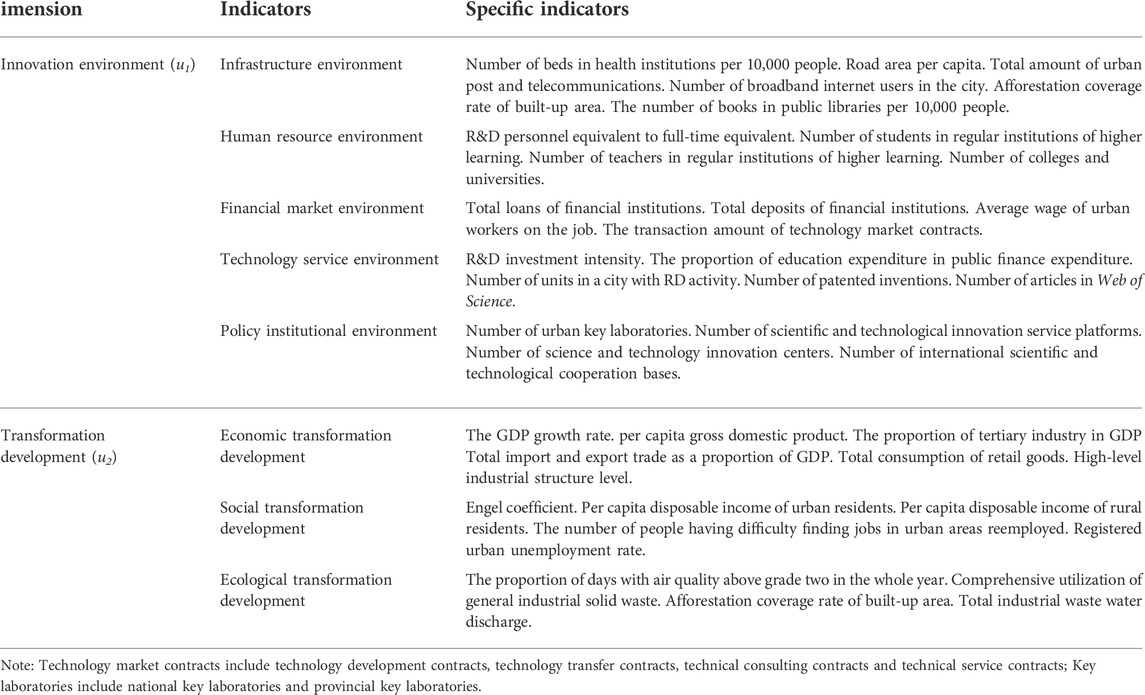
TABLE 1. Resource-based city innovation environment and transformation development evaluation index system.
3.3 Research methods
Figure 3 shows the analysis framework of this research. The theoretical basis of the coupling mechanism between innovation environment and transformation development is analyzed. The relevant data are collected and sorted, and a comprehensive evaluation index system is constructed. At the same time, the data are preliminarily processed by the standardized method. The entropy method calculates the subsystem index of the innovation environment and transformation development, and the coupling coordination degree model calculates the coupling coordination degree of innovation environment and transformation development. The Panel Quantile Regression model calculates the influencing factors of the coupling coordination degree of the innovation environment and transformation development.
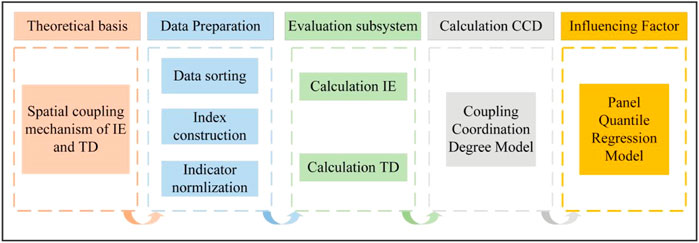
FIGURE 3. Analysis framework for evaluating coupling coordination degree between innovation environment and transformation development. Note: IE innovation environment), TD (transformation development), CCD (coupling coordination degree).
3.3.1 Entropy method
The entropy method is used to calculate the comprehensive measure of the innovation environment and transformation development of resource-based cities. The entropy method has the advantage of objective weighting, avoids the deviation caused by subjective factors to a certain extent, and can realistically reflect the importance of various indicators in the comprehensive indicators. The specific calculation process and steps can be found in the literature (Dong et al., 2021).
3.3.2 Coupling degree and coordination degree
The concept of “coupling” is borrowed from the concept of system coupling in physics, which is referred to by geoscience researchers as the relationship between two or more systems interacting and influencing each other (Liu et al., 2020; Yin et al., 2021). We used the coupling degree and coordination degree to explore the possible relationship between the innovation environment and transformation development of resource-based cities. The formula is as follows:
where C represents the coupling degree, u1 represents the innovation environment index, and u2 is the transformation development index. Because the coupling degree calculation method may produce the situation where the subsystem development level is low but the coupling degree is high, it cannot truly reflect the overall comprehensive coordination level. Therefore, coupling coordination degree is further introduced:
where D represents the coordination degree; T represents the comprehensive evaluation index of the two systems, and α and β represent the undetermined coefficient, and the sum is 1 (we assumed that the two systems are equally important; both α and β were assigned 1/2). Referring to previous research (Chen and Zhang 2021), the coupling degree and coordination degree were classified, as listed in Table 2.
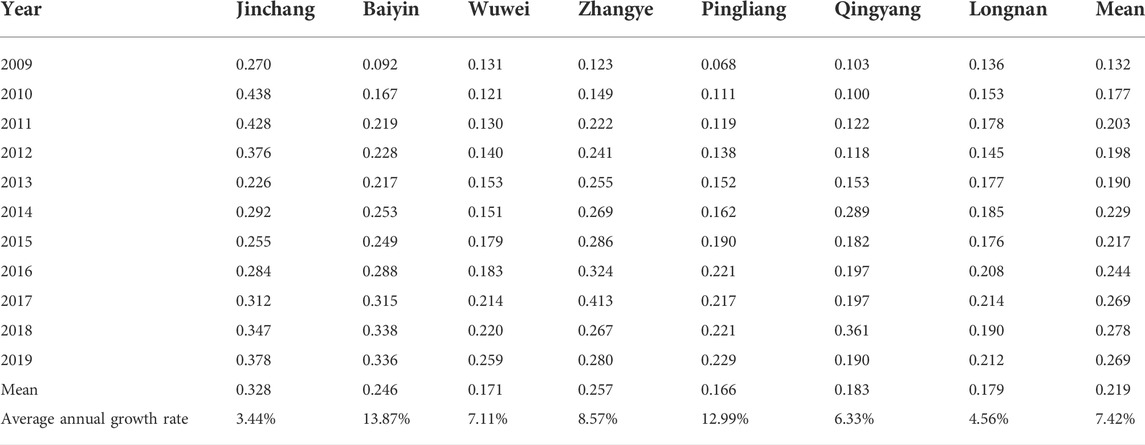
TABLE 4. Comprehensive scores of resource-based cities’ transformation development from 2009 to 2019.
3.3.3 Panel quantile regression model
In previous studies, scholars mainly focused on the influence of explanatory variables on the conditional expectation of the explained variables, which is actually the central tendency of describing the conditional distribution. However, this study focuses on the influence of explanatory variables on the whole conditional distribution, so the quantile regression model is selected to provide comprehensive information on the conditional distribution to reflect the overall picture of the entire conditional distribution and to explore the influencing factors of the coupling coordination degree between innovation environment and transformation development in resource-based cities. The panel quantile regression model was introduced by Koenker and Bassett Jr. (Koenker and Bassett, 1978) and became a comprehensive method for statistical analysis models in various fields (Koenker and Hallock 2001; Cheng et al., 2021). In this study, a panel quantile regression model was further introduced to estimate the linear relationship between explanatory variables and explained variables under different quantiles (Li et al., 2021; Salari et al., 2021). The traditional ordinary least squares regression model (OLS) can get the conditional expectation between explanatory variables and explained variables (Cheng et al., 2021). That is, it can provide a unique conclusion for all resource-based cities in Gansu Province. However, there are spatial differences in the degree of coupling coordination between the innovation environment and transformation development of resource-based cities in Gansu Province. Therefore, the quantile regression model is selected to investigate the influence of explanatory variables on cities with different degrees of coupling coordination. The formula is as follows:
In the formula, the left side of the equation is the τ conditional quantile of coupling coordination; Xit stands for the explanatory variable; τ stands for quantile, ranging from 0 to 1; β(τ) denotes the regression coefficient at the τ quantile; µi(τ) denotes the individual effect at the τ quantile; eit represents the model disturbance term.
3.4 Data sources
The research period of this study is 2009–2019, and the relevant data involved in this study are mainly obtained from The Statistical Yearbook of Chinese Cities, China Statistical Yearbook, China Urban Economic Yearbook, Gansu Development Yearbook, statistical yearbook of seven resource-based cities, and the Statistical Bulletin of National Economic and Social Development of corresponding cities. Some data were obtained from the Science and Technology Department of Gansu Province’s official website (https://kjt.gansu.gov.cn/). The missing data of some indicators in very few years were obtained by interpolation of the existing year’s data.
4 Results
4.1 Comprehensive evaluation of the innovation environment
On the time scale, the innovation environment index of resource-based cities in Gansu Province has greatly improved from 2009 to 2019, showing a dynamic evolution trend of rapid growth. With the increase of time, the innovation environment index of resource-based cities is unbalanced in the development process, and the gap among cities increases (Figure 4A). This is reflected in the fact that the innovation environment index scores for resource-based cities increased from 0.048 to 0.353 during the study period, and the average annual growth rate reached 21.52%. In 2009, the innovation environment gap of resource-based cities in Gansu province was small and basically in the same development stage. From 2009 to 2017, the growth of the innovation environment in all cities was relatively slow, with Zhangye City having the most significant growth range (0.277) and Longnan City having the smallest growth range (0.033). From 2017 to 2019, except for Qingyang and Baiyin, other cities’ innovation environment indexes realized a significant growth trend. The increasing range of Zhangye, Pingliang, and Longnan reached about 0.30, and the growing fields of Jinchang and Wuwei were 0.135 and 0.243, respectively. During this period, the gap in the innovation environment index of various cities gradually widened.
The innovation environment level of resource-based cities in Gansu province is not high, showing the development characteristics of “low level, fast growth.” The main reasons for this are that resource-based cities have a low level of economic and social development and relatively insufficient innovation development motivation, and it is challenging to create an improved regional innovation environment in terms of innovation element concentration and input. At the same time, the relatively backward infrastructure environment in the region also puts tremendous pressure on the improvement of the regional innovation environment, which results in the level of innovation development being low for a long time. In recent years, with the deepening of the innovation-driven development strategy, the central and local governments have increased efforts to create a regional innovation environment from various aspects, such as funds and policies, put forward to drive high-quality regional development through scientific and technological innovation, and actively improve regional innovation level so that resource-based cities in Gansu province usher in rapid development opportunities. In terms of regions, Zhangye has been actively implementing the innovation-driven development strategy in recent years, focusing on promoting regional scientific and technological progress and innovation level, and the comprehensive index of regional scientific and technical progress has been steadily increasing, and several high-tech enterprises have been identified as leading in the province, which has dramatically improved the innovation environment of the region in recent years. Baiyin and Qingyang are relatively rich in resources and have a relatively good industrial base, but the lack of diversified capital investment in innovation, science, and technology service environments to be further optimized makes the innovation environment of these two cities show a slow-growth trend. Other cities have also actively accelerated the construction of regional innovation environments and created an excellent regional innovation environment to promote local economic development, industrial transformation, and upgrading. For example, Jinchang has actively constructed an innovation city and demonstration area for the transfer and transformation of scientific and technological achievements, significantly improving the level of the regional innovation environment.
4.2 Comprehensive evaluation of transformation development
On the time scale, there are differences in the transformation development characteristics of resource-based cities from 2009 to 2019. However, in general, they show a fluctuating upward trend (Figure 4B). The score of the transformation development index of resource-based cities increased from 0.132 to 0.269 during the study period, with an average annual growth rate of 7.42%. The transformation development of Baiyin is the fastest, with a numerical increase from 0.092 in 2009 to 0.336 in 2019; its average annual growth rate is the largest; the growth rate is 13.87%. Pingliang followed with an annual growth rate of 12.99%. Wuwei, Zhangye, and Qingyang’s average annual growth rates are between 6% and 9%, which is in the middle. The transformation development index of Jinchang presents an “N” type, taking 2013 as the timing breakpoint, with the range of change in the early stage being significant and showing a sharp growth followed by a sharp decline and a relatively stable change in the late stage, presenting a trend of fluctuating growth. It is worth noting that Qingyang’s transformation development index fluctuates wildly and shows a downward trend after 2018. From the perspective of regional industrial structure, the typical “two, three, one” industrial structure in 2009 gradually changed to the coexistence pattern of “three, two, one” and “two, three, one” in 2019. The proportion of the output value of the primary and secondary industries showed a decreasing trend during the study period, while the ratio of the output value of the tertiary industry increased year by year. With time, the industrial structure of each city gradually changed from a lower level to a higher level. However, the output value of the secondary industry in Jinchang and Qingyang has always been in the first place for a long time, and the urban industrial structure is still dominated by the secondary industry. However, in Jinchang and Qingyang, the proportion of the output value of the secondary industry in the total GDP has always been the first, and the secondary sector still dominates the urban industrial structure.
Although the transformation development of resource-based cities in Gansu province fluctuates wildly, there is steady progress, and the overall trend is rising. The main reason is that, in recent years, resource-based cities in Gansu province have actively implemented innovation-driven strategies and industrial transformation and upgrading strategies, gradually promoting the coordinated development of various industries in the city and the effective use of natural resources, significantly improving the level of transformation development. The level of transformation development in Wuwei, Pingliang, and Longnan has increased steadily, mainly because these cities are relatively less reliant on resources. The industrial structure transformation was achieved earlier, forming an industrial structure development form dominated by the tertiary industry. At the same time, it also promotes rational division of labor and benign interaction of local industries through the centralized utilization and development of resources. Qingyang is rich in mineral, agricultural, and tourism resources, but in recent years, the quality of resources has declined, and mining is challenging. Meanwhile, it has always been an industrial city dominated by the secondary industry, and the urban transformation development fluctuates wildly, and it has entered the critical stage of transformation development. Jinchang has a more extensive, sizeable industrial foundation and good resource endowment, but it is also faced with excessive dependence on resources and a shortage of emerging industry development prominent question. For this, Jinchang puts forward the development idea of “two districts, two cities, and two integration,” which cultivates the new driving force of green development, gets rid of the dependence on path development, and speeds up the urban transformation and upgrading.
4.3 Coupling coordination analysis of innovation environment and transformation development
4.3.1 Coupling coordination degree analysis
The coupling coordination degree between the innovation environment and transformation development of all resource-based cities shows fluctuating growth during the study period. The coupling coordination state changes from a severe imbalance state to a moderate imbalance state. Some cities have changed to a low coordination state, and the overall trend of the continuous optimization process is shown in Figure 5. The starting point of the coupling coordination degree of each city is relatively low, but it shows a significant improvement during the study period. According to the increase in amplitude of coupling coordination degree, the rank from large to small is as follows: Zhangye > Pingliang > Baiyin > Longnan > Wuwei > Jinchang > Qingyang, and the overall increase amplitude was more than 66%, among which, Zhangye increased from 0.195 in 2009 to 0.457 in 2019, and the increase was the first among all cities, reaching 134.22%. Qingyang saw the smallest increase, from 0.181 in 2009 to 0.301 in 2019.
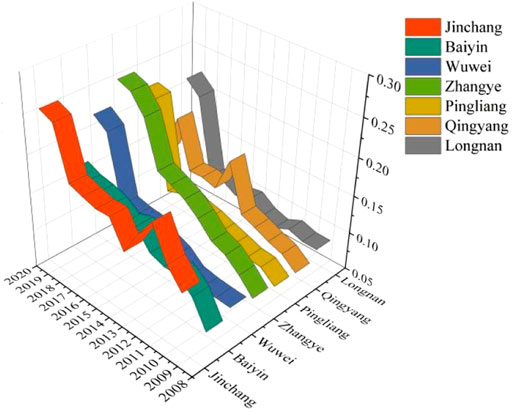
FIGURE 5. Time variation of coupling coordination degree between innovation environment and transformation development of resource-based cities.
4.3.2 Type of coupling coordination and analysis of shortboard
From the perspective of the long-term coordinated development of the system, it is essential to identify the shortcomings and influencing factors of the subsystem development balance. System Science Theory believes that development of composite systems does not require the complete synchronous development of subsystems. When the development difference of subsystems is within a certain buffer zone, it is considered that the development of subsystems tends to be synchronous, showing coordinated development. Based on the abovementioned considerations, this study classifies the coupling coordination degree and coordinated development type between innovation environment and transformation development of resource-based cities (Table 5). It can be seen from the figure that the coupling coordination degree between the innovation environment and transformation development of resource-based cities from 2009 to 2019 is in a moderate imbalance state in most cities. The short board form of coupling coordination between the two shows prominent stage characteristics, which is basically manifested as “synchronous development” or “moderate imbalance-innovation environment lag” development. Jinchang changed from “moderate imbalance-innovation environment lag” to “low coordination”. In 2017, Wuwei changed from “moderate imbalance” to “low coordination-transformation development lag”; Baiyin changed from “moderate imbalance” to “low coordination” in time series, but it always presents the shortboard of innovation environment lagging development. The main reason lies in the lack of innovation development motivation, innovation environment support, innovation talents, and capital investment in these cities, which leads to slow innovation development of these cities and lagging development of the innovation environment. Due to the limitations of natural resources, traffic location, and social and economic development level, the innovation environment and transformation development of Longnan City started late. Under the condition that the internal and external environment has not changed significantly, the development process of the two is difficult to present long-term synchronous development, and the coordinated development degree of the two is low.
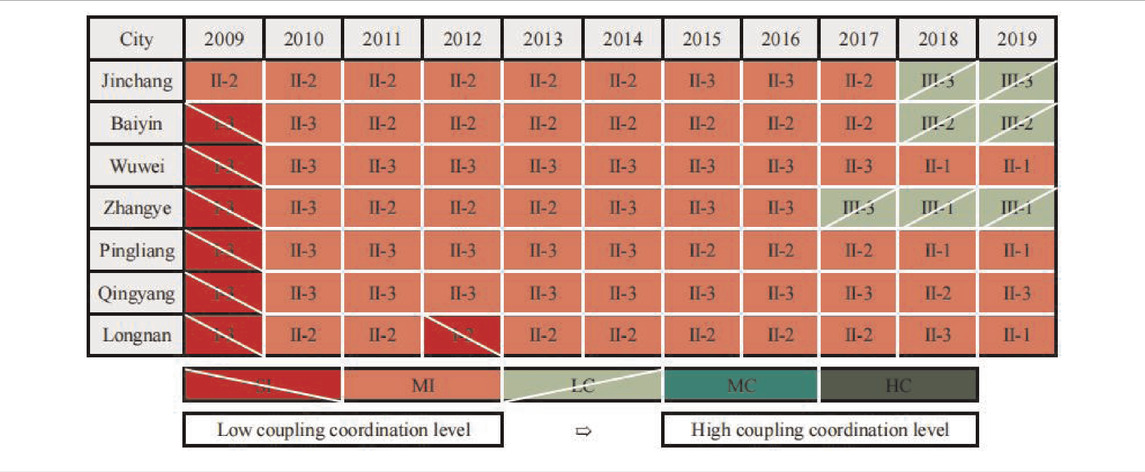
TABLE 5. Coupling coordination types of innovation environment and transformation development of resource-based cities in Gansu province from 2009 to 2019.
4.3.3 Dynamic evolution characteristics of coupling coordination degree
The Epanechnikov function was used to analyze the dynamic evolution characteristics of the coupling coordination degree between the innovation environment and the transformation development of resource-based cities in Gansu Province from 2009 to 2019. The time sections of 2009, 2011, 2013, 2015, 2017, and 2019 were selected as the research sections. Based on the kernel density distribution curve’s distribution location, morphology, and ductility, the dynamic evolution process of the coupling coordination degree from 2009 to 2019 was described in detail, and the kernel density curve was finally drawn (Figure 6). From the distribution position, the kernel density distribution curve shows a prominent right-shift characteristic, which indicates that the coupling coordination degree of innovation environment and transformation development of resource-based cities in Gansu province shows an increasing trend and keeps moving toward a high level. In terms of distribution morphology, the peak value of the kernel density curve experienced a “decline–rise” evolution trend, with the width continuously expanding, indicating that in the early stage of the study, the absolute gap within the region was further expanding. However, the absolute gap was narrowed in the later stage, and the central trend was strengthened. Regarding kurtosis of the kernel density distribution curve, the top of the kernel density curve in 2009 is relatively sharp and has a large kurtosis. The peak values of kernel density curves of coupling coordination degree in each year gradually slow down with time, indicating that the outliers of coupling coordination degree show a reducing trend with the increase of years. Regarding the kernel density distribution curve, the coupling coordination degree presents a “single-peak” distribution. In 2009, the coupling coordination degree was concentrated at about 0.18, and most cities (except Jinchang) were severely imbalanced, consistent with the previous research. In 2019, the coupling coordination degree of all cities was concentrated between 0.37 and 0.45, mainly distributed in Jinchang, Wuwei, Pingliang, and Zhangye.
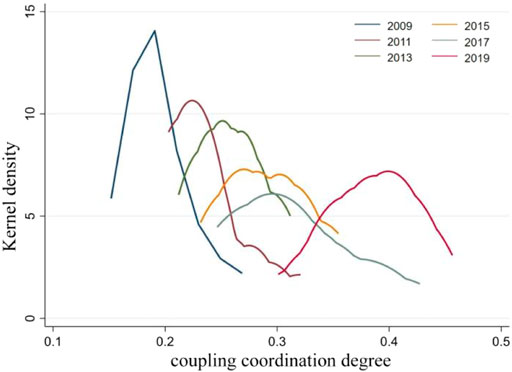
FIGURE 6. Core density curve of the coupling coordination degree between innovation environment and transformation development of resource-based cities in Gansu Province from 2009 to 2019.
4.4 Driving factor analysis
4.4.1 Identification of driving factors and variable selection
First, ITD was used to represent the coupling coordination degree between the innovation environment and transformation development of resource-based cities as the explained variable. Based on the abovementioned research and analysis, the influencing factors of the difference in the coupling coordination degree between the innovation environment and transformation development of resource-based cities are selected from four dimensions: economic development, industrial structure, capital investment, and government intervention (Table 6). 1) Economic development. The level of regional economic development is closely related to regional transformation (Wang L. et al., 2021). The level of economic development can affect the quality of the urban innovation environment and the process of transformation development and then affect the degree of synergistic development between the two. So, per capita gross domestic product is selected to represent the level of economic development. 2) Industrial structure. Relevant research results (Wang L. et al., 2021) show that scientific and technological innovation is of great significance to the optimization and upgrading of regional industrial structures, and the upgrading of regional industrial structures also has a significant impact on the improvement of regional scientific and technological innovation level and the quality of regional transformation development. Therefore, the proportion of the tertiary industry in GDP is selected as a proxy variable. 3) Capital investment. Referring to the neoclassical economic theory, an increase in capital investment can promote the improvement of urban economic aggregates, scientific and technological progress, and industrial transformation and upgrading. The social fixed asset investment ratio to GDP is selected as a proxy variable. 4) Government intervention. Government intervention can compensate for inadequacy of the regional economic market and the real economy. Moderate government intervention has significant positive effects on regional economic development, but excessive government intervention will also not be conducive to regional industrial restructuring (Zhang et al., 2018). So, the impact of government intervention on the urban innovation environment and transformation development depends on the situation. From the research perspective of this study, local government expenditure as a percentage of GDP is selected as a proxy variable. The more considerable local government expenditure as a percentage of GDP is, the higher the degree of government intervention is and the lower the role of the market plays. Natural logarithmic processing is carried out to analyze and study the coupling coordination degree between the innovation environment and transformation development of resource-based cities, per capita gross domestic product, and the proportion of tertiary industry in GDP.

TABLE 6. Indicator system for the coupled and coordinated development of innovation environment and transformation development in resource-based cities.
4.4.2 Quantile regrression analysis
As can be seen from Table 7, with the increase of quantiles, the quantile regression coefficient of the economic development level shows a trend of first decreasing and then increasing, which indicates that the influence of economic development on both ends of the conditional distribution of coupling coordination degree is more significant than that on the middle part. In other words, improving economic development significantly impacts cities with low or high coupling coordination degree between innovation environment and transformation development, while benefiting the middle part less. Specifically, for every 1% increase in the economic development level of resource-based cities, the level of coupling coordination at the 10, 40, and 90% quantiles increases by 0.203, 0.169, and 0.296%, respectively. The level of industrial structure promotes the improvement of the coupling coordination degrees between the innovation environment and transformation development of resource-based cities to a certain extent at each quantile, and all pass the 1% level test. The regression coefficient showed an increasing trend of fluctuation with the increase of quantile. This indicates that the industrial structure significantly influences the coupling coordination degree, and the influence degree is greater than that of the economic development level. To be specific, the coupling coordination degree of innovation environment and transformation development will increase by 0.543, 0.731, and 0.608% in cities at 10, 50, and 90% of the quantile when the level of industrial structure increases by 1%, indicating that the level of industrial structure will have a strong impact on the coupling coordination degree only within a certain floating range. The level of capital investment has different effects on different quantiles of coupling coordination degree. There is a positive correlation in the low quantiles, but it does not pass the significance test. In high quantiles, there is a negative correlation, and it passes the 1% significance test, the negative effect increased with the increase of quantile. The main reason may be that capital investment is a double-edged sword, and capital investment exceeding actual social demand will lead to slow regional economic development and will not show a linear growth relationship. Driven by capital investment, resource-based cities fail to promote advantages and eliminate disadvantages and fail to combine capital investment with a regional innovation environment and regional transformation development. The influence of government intervention degree on coupling coordination degree is negative, indicating that the stronger the government intervention degree, the worse the coupling coordination degree between innovation environment and transformation development of resource-based cities. As shown in Table 7, government intervention has no significant influence on coupling coordination degree at the 10 and 90% quantile. At the 20%–80% quantile, the regression coefficient increases first and then decreases and reaches its maximum at the 50% quantile, indicating that government intervention has the strongest influence on cities in the middle of the coupling coordination degree. The main reason is that the innovation environment of resource-based cities itself is low, and the transformation development is relatively tricky. As a result, the degree of government intervention does not match the development conditions of backward resource-based cities, which hinders the coordinated development degree of innovation environments and transformation development of resource-based cities. Figure 7 shows the differences in the coupling coordination degree of each explanatory factor in different quantiles from 2009 to 2019.
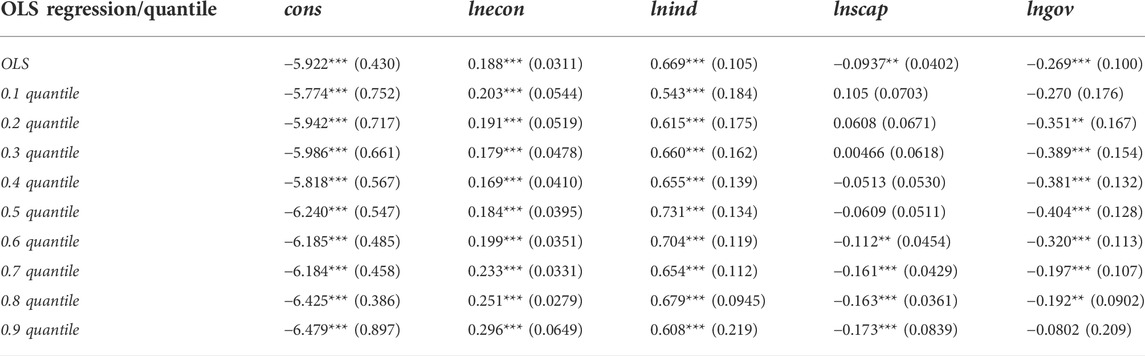
TABLE 7. Quantile regression and OLS regression results of coupled coordination degree driving factors.
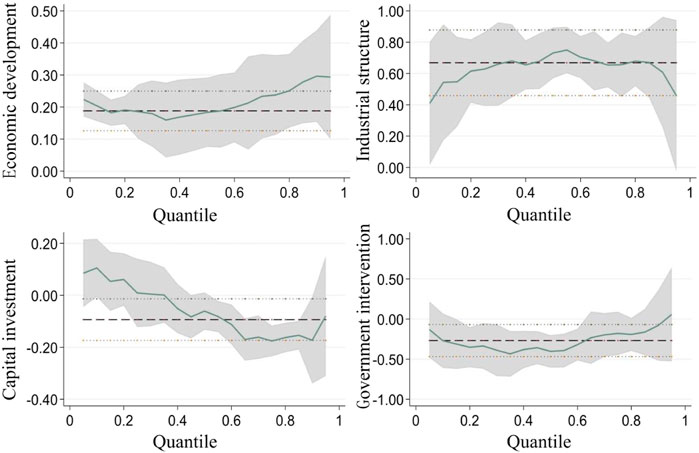
FIGURE 7. Differences in the coupling coordination degree of various explanatory factors in different quantile models.
5 Discussion
5.1 Policy recommendations
While improving the quality of the innovation environment in resource-based cities, innovation achievements should be transformed and applied to regional transformation development. Based on the current development situation, we should give full play to the advantages of urban resources and selectively develop industries with comparative advantages. At the same time, we will adhere to the direction of green, intelligent, and high-end industries; actively promote the extension of the industrial chain; and speed up the upgrading of traditional industries by centering on the advantages of featured minerals, petroleum, and other resources such as making full use of Jinchang’s nonferrous metal new material industry base, Qingyang, Pingliang’s oil and coal industry base, Zhangye’s big data cloud platform, as well as Baiyin’s Lanbai National Independent Innovation Demonstration Zone and Lanbai Science and Technology Innovation Reform Pilot Zone, strengthening the resource-based cities’ cooperation with well-known colleges and universities and research institutions, expanding and enhancing strategic emerging industries such as new energy and new materials, and realize their development in a concentrated, clustered, and intensive manner. Relying on regional, national, and provincial cultural tourism resources and cultural brands, we enlarge the comprehensive effect of the cultural tourism industry, promote in-depth integration of cultural tourism, and strive to build strategic pillar industries of cultural tourism to promote high-quality development of resource-based cities in transformation.
While accelerating the transformation development level of resource-based cities, efforts should be made to create a regional innovation environment. All resource-based cities take advantage of their geographical advantages to participate in major infrastructure construction actively and promote internal and external connectivity of infrastructure. For example, Qingyang and Pingliang should accelerate the formation of Ping-Qing metropolitan, actively integrate into the Guanzhong Plain urban agglomeration, and speed up intercity transportation facility interconnection. Longnan should actively integrate into the Chengdu–Chongqing urban agglomeration, deepen inter-provincial cooperation with Chengdu and other places, strengthen regional linkage development, and build new growth points and poles for regional development. Jinchang, Wuwei, Zhangye, and Baiyin actively integrated into the economic circle of Lanzhou and accelerated the planning and construction of high-grade highways and the layout planning of a high-speed intercity railway with Lanzhou, reducing the time cost and logistics cost of all cities and major cities and promoting the coordinated development of innovation environment optimization and industrial structure transformation. Encouraging research institutes and institutions of higher learning to set up research institutes in resource-dependent cities, introducing state-level key laboratories and technology engineering centers, encouraging local governments to build regional bases for technological venture capital and bases for entrepreneurship and innovation of small and medium-sized enterprises and selectively train high-level innovative scientific and technical personnel, and creating good innovation environment for the high-quality development of resource-based city industry transformation.
5.2 Research deficiencies and future research directions
The innovation environment and transformation development of resource-based cities discussed in this study are only discussed from the perspective of coupling coordination, but in fact, the internal relationship between the innovation environment and transformation development of resource-based cities is highly complex. In the future, it is necessary to comprehensively and systematically discuss the deep-seated essential problems existing in developing resource-based cities from a multi-directional and multi-perspective. Whether the relationship between an innovation environment and transformation development in resource-based cities in Gansu Province is universal needs to be tested by using other regions or provinces as examples, and it is necessary to explore further the mechanism of technology, talent, capital, and policy on the transformation development and upgrading development of resource-based cities, as well as the transformation development and upgrading development mode of resource-based cities under the background of the “3,060” dual carbon target.
6 Conclusions
1) During the study period, both the innovation environment index and the transformation development index of resource-based cities increased significantly. The innovation environment index of resource-based cities has been greatly improved, showing a dynamic evolution trend of rapid growth and showing the development characteristics of “low level, fast growth”. There are differences in the characteristics of transformation development resource-based cities, but on the whole, it shows a trend of increasing fluctuation. Although the fluctuation is significant, there is steady progress.
2) The coupling coordination degree of innovation environment and transformation development in various resource-based cities was significantly developed during the study period. The coupling coordination state shows the transformation from the severe imbalance state to the moderate imbalance state, and some cities have changed to the low coordination state. The overall trend is to optimize the process continuously. The process of the coupling coordination degree between innovation environment and transformation development in resource-based cities is not synchronous, and the short form of the coupling coordination between the two generally shows obvious stage characteristics, which is manifested as “synchronous development” or “moderate imbalance—innovation environment lag” development. The kernel density distribution curve showed an obvious right-shift characteristic, and the coupling coordination degree showed an increasing trend and continuously advanced to a high level.
3) Improving economic development significantly impacts the innovation environment and transformation development of resource-based cities with low and high coupling coordination degrees. Industrial structure promotes the coupling coordination between an innovation environment and the development of resource-based cities to a certain extent at each decimal point. Capital investment has different effects on different quartiles of coupling coordination degree, with positive correlation in low quartiles and negative correlation in high quartiles. The stronger the government’s intervention degree, the worse the coupling coordination degree between the innovation environment and transformation development of resource-based cities.
Data availability statement
The raw data supporting the conclusions of this article will be made available by the authors without undue reservation.
Author contributions
Junfeng Yin: conceptualization, methodology, software, visualization, writing—original draft, and writing—review and editing. Peiji Shi: conceptualization, writing review, and supervision. Haoyuan Feng, Huali Tong, and Meng Chao: writing—review and modified language.
Funding
This work was supported by the National Natural Science Foundation of China (Grant No. 41771130).
Conflict of interest
The authors declare that the research was conducted in the absence of any commercial or financial relationships that could be construed as a potential conflict of interest.
Publisher’s note
All claims expressed in this article are solely those of the authors and do not necessarily represent those of their affiliated organizations, or those of the publisher, the editors, and the reviewers. Any product that may be evaluated in this article, or claim that may be made by its manufacturer, is not guaranteed or endorsed by the publisher.
References
Appio, F. P., Frattini, F., Petruzzelli, A. M., and Neirotti, P. (2021). Digital transformation and innovation management: A synthesis of existing research and an agenda for future studies. J. Prod. Innov. Manage. 38, 4–20. doi:10.1111/jpim.12562
Balsa-Barreiro, J., Li, Y., Morales, A., and Pentland, A. S. (2019). Globalization and the shifting centers of gravity of world’s human dynamics: Implications for sustainability. J. Clean. Prod. 239, 117921–117923. 117917. doi:10.1016/j.jclepro.2019.117923
Chen, W., Shen, Y., and Wang, Y. (2018). Evaluation of economic transformation and upgrading of resource-based cities in Shaanxi province based on an improved TOPSIS method. Sustain. Cities Soc. 37, 232–240. doi:10.1016/j.scs.2017.11.019
Chen, Y., and Zhang, D. (2021). Multiscale assessment of the coupling coordination between innovation and economic development in resource-based cities: A case study of northeast China. J. Clean. Prod. 318, 128597. doi:10.1016/j.jclepro.2021.128597
Cheng, C., Ren, X., Dong, K., Dong, X., and Wang, Z. (2021). How does technological innovation mitigate CO2 emissions in OECD countries? Heterogeneous analysis using panel quantile regression. J. Environ. Manag. 280, 111818. doi:10.1016/j.jenvman.2020.111818
Council, T. S. (2013). The plan for the sustainable development of resource-based cities in China (2013–2020). China. Available online at: http://www.gov.cn/zwgk/-12/03/content_2540070.htm.
Deng, X., Liang, L., Wu, F., Wang, Z., and He, S. (2022). A review of the balance of regional development in China from the perspective of development geography. J. Geogr. Sci. 32 (1), 3–22. doi:10.1007/s11442-021-1930-0
Dong, L., Longwu, L., Zhenbo, W., Liangkan, C., and Faming, Z. (2021). Exploration of coupling effects in the economy–society–environment system in urban areas: Case study of the ebeiu river delta urban agglomeration. Ecol. Indic. 128, 107858. doi:10.1016/j.ecolind.2021.107858
Doytch, N., and Narayan, S. (2016). Does FDI influence renewable energy consumption? An analysis of sectoral FDI impact on renewable and non-renewable industrial energy consumption. Energy Econ. 54, 291–301. doi:10.1016/j.eneco.2015.12.010
Fang, C., Ma, H., Wang, Z., and Li, G. (2014). The sustainable development of innovative cities in China: Comprehensive assessment and future configuration. J. Geogr. Sci. 24 (6), 1095–1114. doi:10.1007/s11442-014-1141-z
Gong, C. (2021). Government R&D investment, knowledge accumulation, and regional innovation capability: Evidence of a threshold effect model from China. Complexity 2021, 8963237. doi:10.1155/2021/8963237
Hasan, I., and Tucci, C. L. (2010). The innovation–economic growth nexus: Global evidence. Res. Policy 39 (10), 1264–1276. doi:10.1016/j.respol.2010.07.005
Hoekveld, J. J. (2012). Time-space relations and the differences between shrinking regions. Built Environ. 38 (2), 179–195. doi:10.2148/benv.38.2.179
Jiang, Z., Lyu, P., Ye, L., and wenqian Zhou, Y. (2020). Green innovation transformation, economic sustainability and energy consumption during China’s new normal stage. J. Clean. Prod. 273, 123044. doi:10.1016/j.jclepro.2020.123044
Jin, W., Zhang, H.-q., Liu, S.-s., and Zhang, H.-b. (2019). Technological innovation, environmental regulation, and green total factor efficiency of industrial water resources. J. Clean. Prod. 211, 61–69. doi:10.1016/j.jclepro.2018.11.172
Koenker, R., and Bassett, G. (1978). Regression quantiles. Econometrica 46, 33–50. doi:10.2307/1913643
Koenker, R., and Hallock, K. F. (2001). Quantile regression. J. Econ. Perspect. 15 (4), 143–156. doi:10.1257/jep.15.4.143
Kuai, P., Li, W., Cheng, R., and Cheng, G. (2015). An application of system dynamics for evaluating planning alternatives to guide a green industrial transformation in a resource-based city. J. Clean. Prod. 104, 403–412. doi:10.1016/j.jclepro.2015.05.042
Li, B., and Dewan, H. (2017). Efficiency differences among China’s resource-based cities and their determinants. Resour. Policy 51, 31–38. doi:10.1016/j.resourpol.2016.11.003
Li, K., and Lin, B. (2018). How to promote energy efficiency through technological progress in China? Energy 143, 812–821. doi:10.1016/j.energy.2017.11.047
Li, H., Long, R., and Chen, H. (2013). Economic transition policies in Chinese resource-based cities: An overview of government efforts. Energy Policy 55, 251–260. doi:10.1016/j.enpol.2012.12.007
Li, H., Lo, K., and Zhang, P. (2020). Population shrinkage in resource-dependent cities in China: Processes, patterns and drivers. Chin. Geogr. Sci. 30 (1), 1–15. doi:10.1007/s11769-019-1077-4
Li, W., Elheddad, M., and Doytch, N. (2021). The impact of innovation on environmental quality: Evidence for the non-linear relationship of patents and CO2 emissions in China. J. Environ. Manag. 292, 112781. doi:10.1016/j.jenvman.2021.112781
Liu, H., Fang, C., and Fang, K. (2020). Coupled human and natural cube: A novel framework for analyzing the multiple interactions between humans and nature. J. Geogr. Sci. 30 (3), 355–377. doi:10.1007/s11442-020-1732-9
Liu, E. N., Wang, Y., Chen, W., Chen, W., and Ning, S. (2021). Evaluating the transformation of China’s resource-based cities: An integrated sequential weight and TOPSIS approach. Socio-Economic Plan. Sci. 77 (17), 101022. doi:10.1016/j.seps.2021.101022
Martinez‐Fernandez, C., Audirac, I., Fol, S., and Cunningham‐Sabot, E. (2012). Shrinking cities: Urban challenges of globalization. Int. J. Urban Reg. Res. 36 (2), 213–225. doi:10.1111/j.1468-2427.2011.01092.x
Maurseth, P. B., and Verspagen, B. (2002). Knowledge spillovers in ebei: A patent citations analysis. Scand. J. Econ. 104 (4), 531–545. doi:10.1111/1467-9442.00300
Miao, C., Fang, D., Sun, L., Luo, Q., and Yu, Q. (2018). Driving effect of technology innovation on energy utilization efficiency in strategic emerging industries. J. Clean. Prod. 170, 1177–1184. doi:10.1016/j.jclepro.2017.09.225
Porter, M. E. (1991). Towards a dynamic theory of strategy. Strateg. Manag. J. 12 (S2), 95–117. doi:10.1002/smj.4250121008
Sachs, D. J., and Warner, M. A. (2001). Natural resource and economic development: The curse of natural resources. Eur. Econ. Rev. 46, 827–838. doi:10.1016/s0014-2921(01)00125-8
Sachs, J. D., and Warner, A. (1995). Natural resource abundance and economic growth. USA: National bureau of economic research Cambridge, Mass.
Salari, T. E., Roumiani, A., and Kazemzadeh, E. (2021). Globalization, renewable energy consumption, and agricultural production impacts on ecological footprint in emerging countries: Using quantile regression approach. Environ. Sci. Pollut. Res., 1–15.
Shao, W., Yin, Y., Lu, S., and Taghizadeh-Hesary, F. (2021). Analysis of the upgrading effect of the industrial structure of environmental regulation—evidence from 113 cities in China. Front. Environ. Sci. 28 (36), 49627–49641. doi:10.3389/fenvs.2021.692478
Sun, D., Lu, D., Li, Y., Zhou, L., and Zhang, M. (2017). Energy abundance and China’s economic growth: 2000–2014. Chin. Geogr. Sci. 27 (5), 673–683. doi:10.1007/s11769-017-0901-y
Wang, L., Su, M., Kong, H., and Ma, Y. (2021a). The impact of marine technological innovation on the upgrade of China’s marine industrial structure. Ocean. Coast. Manag. 211, 105792. doi:10.1016/j.ocecoaman.2021.105792
Wang, Q., Liu, M., Tian, S., Yuan, X., Ma, Q., and Hao, H. (2021b). Evaluation and improvement path of ecosystem health for resource-based city: A case study in China. Ecol. Indic. 128, 107852. doi:10.1016/j.ecolind.2021.107852
Xie, W., Yan, T., Xia, S., and Chen, F. (2020). Innovation or introduction? The impact of technological progress sources on industrial green transformation of resource-based cities in China. Front. Energy Res. 8, 301. doi:10.3389/fenrg.2020.598141
Xing, M., and Luo, F. (2018). Comparative study on the optimization path of industrial value chain in China’s resource-based cities. Sustainability 10 (5), 1338. doi:10.3390/su10051338
Yan, D., Kong, Y., Ren, X., Shi, Y., and Chiang, S. (2019). The determinants of urban sustainability in Chinese resource-based cities: A panel quantile regression approach. Sci. total Environ. 686, 1210–1219. doi:10.1016/j.scitotenv.2019.05.386
Yin, J., Liu, H., Shi, P., and Zhang, W. (2021). Exploring coupling relationship between urban connection and high-quality development using the case of ebeiu-xining urban agglomeration. Complexity 2021, 1–12. doi:10.1155/2021/9933582
Yu, C., Li, H., Jia, X., and Li, Q. (2015). Improving resource utilization efficiency in China’s mineral resource-based cities: A case study of chengde, ebei province. Resour. Conservation Recycl. 94, 1–10. doi:10.1016/j.resconrec.2014.10.013
Yu, C., de Jong, M., and Cheng, B. (2016). Getting depleted resource-based cities back on their feet again–the example of Yichun in China. J. Clean. Prod. 134, 42–50. doi:10.1016/j.jclepro.2015.09.101
Zhang, H., Xiong, L., Li, L., and Zhang, S. (2018). Political incentives, transformation efficiency and resource-exhausted cities. J. Clean. Prod. 196, 1418–1428. doi:10.1016/j.jclepro.2018.06.093
Zhang, H., Shen, L., Zhong, S., and Elshkaki, A. (2020). Coal resource and industrial structure nexus in energy-rich area: The case of the contiguous area of Shanxi and Shaanxi Provinces, and Inner Mongolia Autonomous Region of China. Resour. Policy 66, 101646. doi:10.1016/j.resourpol.2020.101646
Zhang, Q., and Brouwer, R. (2020). Is China affected by the resource curse? A critical review of the Chinese literature. J. Policy Model. 42 (1), 133–152. doi:10.1016/j.jpolmod.2019.06.005
Keywords: innovation environment, transformation development, coupling mechanism, resource-based city, Gansu province
Citation: Yin J, Shi P, Feng H, Tong H and Chao M (2022) Dynamic evolution and influencing factors of coupling coordination between innovation environment and transformation development of resource-based cities in Gansu province, China. Front. Environ. Sci. 10:990238. doi: 10.3389/fenvs.2022.990238
Received: 09 July 2022; Accepted: 29 August 2022;
Published: 19 September 2022.
Edited by:
Jose Balsa-Barreiro, Massachusetts Institute of Technology, United StatesReviewed by:
Xiaodong Yang, Xinjiang University, ChinaFengtao Guang, China University of Geosciences Wuhan, China
Copyright © 2022 Yin, Shi, Feng, Tong and Chao. This is an open-access article distributed under the terms of the Creative Commons Attribution License (CC BY). The use, distribution or reproduction in other forums is permitted, provided the original author(s) and the copyright owner(s) are credited and that the original publication in this journal is cited, in accordance with accepted academic practice. No use, distribution or reproduction is permitted which does not comply with these terms.
*Correspondence: Peiji Shi, xbsdspj@163.com