- Jangho Architecture College, Northeastern University, Shenyang, China
Frequent mining activities can bring about problems such as soil erosion and environmental pollution, which are detrimental to the efficient use of land and the sustainable development of cities. Existing studies have paid little attention to mining areas and lack comparative analysis of landscape changes in multiple mining pits. In this paper, the main urban area of Anshan City, where the mining areas are concentrated, was used as the research area, and the Landsat TM/OLI surface reflectance (SR) data of the Google Earth Engine (GEE) platform and the random forest algorithm were used to map the land use in 2008, 2014, and 2020. On this basis, land use dynamics and landscape pattern indices were used to analyze the changes in land use and landscape patterns in the Anshan City area. In addition, a moving window method was combined to further analyze and compare the landscape changes between different pits. The results show that:1. From 2008 to 2020, the construction land in Anshan urban area continued to decline, the forest land continued to expand, and the construction land was shifted to the forest land and cultivated land. Mining land increased before 2014 and remained almost unchanged after 2014, which is in line with the actual situation. 2. During the study period, the landscape fragmentation degree and landscape heterogeneity in the urban area of Anshan kept increasing. The high value areas of landscape fragmentation were the urban-rural combination areas and the mining areas. Among them, the reclamation of Dagushan and Donganshan is better, while the reclamation of Anqian, Yanqianshan and Xiaolingzi mines needs to be strengthened. 3. The random forest algorithm based on GEE shows a high degree of accuracy for land use classification. The overall classification accuracy in 3 years exceeds 90% and the kappa coefficient exceeds 0.85. The study results can be used as an essential reference for optimizing the urban ecological environment and provide technical backing for the urbanization construction and rational use of land in Anshan City.
1 Introduction
Land use change is driven by human behavior and affects the structure and function of landscape ecosystems (Schmitt et al., 2010; Bajocco et al., 2012). Since the 1990s, land use change research has become one of the central issues in global environmental change research (Peng et al., 2008; Liu and Deng, 2010; Chang et al., 2018). Landscape pattern is the spatial distribution and arrangement of landscape elements (Liu et al., 2010), which represents the effects of different ecological processes acting at different spatial scales, and is the embodiment of landscape heterogeneity (Cheung et al., 2016; Feng et al., 2018). Changes in landscape patterns are an integrated reflection of the regional ecosystem as a result of a combination of human activities, natural and biological factors (Turner, 1990; Bürgi et al., 2004). Therefore, monitoring and analyzing the spatial and temporal evolutionary characteristics of land use and landscape patterns can help reveal the links between land use and landscape patterns, regulate the direction and speed of human activities, and provide important references for the rational use of land resources and the coordinated development of landscape types.
With the continuous advancement of RS and GIS, the application of RS and GIS to analyze the changing characteristics of landscape pattern and land use has become a research hotspot in geography, environmental science and other disciplines (Tekle and Hedlund, 2000; Groom et al., 2006; Shao and Wu, 2008; Du et al., 2012). The research includes the use of RS and machine learning methods to obtain long time series remote sensing to monitor the spatial and temporal evolution characteristics of land use and its driving factors (Gao et al., 2015; Thakkar et al., 2017), the use of Fragstats software and landscape pattern index to analyze landscape pattern changes (Huang et al., 2008; Liu et al., 2012) and the impact of land use and landscape pattern on the ecological environment (Singh et al., 2010; Jazouli et al., 2019; Tian et al., 2020). Tang et al. (2020) linked land use, landscape pattern and ecosystem service values and used Pearson correlation coefficients to explore the extent to which changes in land use and landscape pattern are associated with ecosystem services, finding that high intensity land use leads to degradation of ecosystem services. These studies have focused on ecologically important areas (Wang et al., 2009; Wan et al., 2015; Liu et al., 2018; Li et al., 2021a) and rapidly urbanizing areas (Deng et al., 2009; Hassan, 2017; Dadashpoor et al., 2019), but less on ecologically fragile urbanized areas such as mining areas and resource-based cities.
The exploitation of mineral resources has become the backbone of China’s sustained and stable economic and social development (Wang et al., 2016; Zhai et al., 2021). Frequent mining activities have changed the surface characteristics (Wu et al., 2021b), the integrity of the original landscape pattern has been damaged under human interference, and the landscape spatial structure has undergone dramatic changes, breaking the local ecological balance and damaging the regional ecological environment. At the same time, this has led to the degradation of urban ecology and aggravated environmental pollution (Xu et al., 2019; Takam Tiamgne et al., 2021; Wang et al., 2021; Yu et al., 2022), which has seriously affected the human settlement environment and restricted the green, healthy and sustainable development of cities (Yang et al., 2021). At present, environmental monitoring in mining areas mainly focuses on studies of vegetation cover changes in traditional mining areas (Lei et al., 2010; Liu et al., 2016b; Fang et al., 2019; Li et al., 2021b) and the characteristics of landscape use changes before and after mine reclamation (Townsend et al., 2009; Ge et al., 2010; Yang et al., 2018). Kuzevic et al. (2022) analyzed the vegetation cover of the Slovakian mining area containing four deposits using NDVI and further compared the vegetation changes in the four deposits using Forest Spatial Division Unit data. Zhang et al. (2020b) used multi-temporal remote sensing images and landscape pattern indexes to study the change characteristics of landscape pattern and land use in the Pingshuo mining area containing three open pits, and constructed a complex network to analyze the correlation between indexes, but did not further compare the landscape changes of the three open pits. Most mining and mineral resource cities have more than one pit, often multiple pits clustered together, and there are differences in landscape pattern changes between individual pits, so using the landscape pattern index to analyze overall trends does not reflect the changes in individual open pits. The moving window method produces a quantitative, spatially distributed metric. Raster mapping through moving windows allows specific changes in the landscape to be monitored both spatially and quantitatively (Hayes and Robeson, 2013).
However, when multi-temporal remote sensing images are used for land use mapping, it often takes a lot of time to download and process remote sensing images. The emergence of Google Earth Engine (GEE) has greatly promoted the research on land use by remote sensing. GEE not only provides massive satellite image datasets and geographic datasets (Kumar and Mutanga, 2018) but also provides API interfaces (Prasai et al., 2021), analysis algorithms and tools based on JavaScript and Python languages (Gorelick et al., 2017; Amani et al., 2020). Programming languages are directly used to analyze and process remote sensing data in GEE (Chang et al., 2018; Mutanga and Kumar, 2019), which avoids the tedious processes of data download, preprocessing, and image classification brought about by traditional remote sensing analysis models. Ang (Ang et al., 2021) and Pericak (Pericak et al., 2018) used GEE to draw land use maps for the Didipio mine and the Appalachian open-pit coal mine respectively. It was found that GEE can not only quickly and efficiently process remote sensing images of large areas over many years but also quantify land use change.
As a typical mineral resource city, Anshan City has abundant iron ore resources, and its iron ore reserves account for 52% of China’s total iron ore resources. Anshan iron ore is concentrated in the main urban area of Anshan City. According to statistics, the total area of the mining area in Anshan is about 50 km2 and the mining area in the main urban area of Anshan alone exceeds 30 km2, which means 60% of the iron ore in Anshan City is concentrated in the main urban area of Anshan. With the uninterrupted mining for a hundred years, a large area of dumps and tailings ponds have been formed in the main urban area of Anshan City, which not only wastes land resources, destroys geological landforms, but also brings many negative impacts to the urban and rural living environment of Anshan. Therefore, this paper uses GEE-based multi-temporal Landsat remote sensing data, combined with the moving window method and the landscape pattern index, to explore 1) the spatial and temporal evolutionary characteristics of land use and landscape patterns in the main urban area of Anshan. 2) Further analysis of landscape changes between different pits in Anshan. 3) The driving factors causing the changes. To provide reference for mine reclamation, rational land use and comprehensive environmental management in Anshan.
2 Data and methods
2.1 Study area
Anshan’s main urban area is the study area (122°4′-123°1′E, 40°5′-41°1′N), situated in the central part of Anshan City, Liaoning Province, bordering Liaoyang City, including Tiexi District, Tiedong District, Lishan District and Qianshan District. The urban area of Anshan is 796 km2. The population of Anshan reaches 1.45 million in 2020. Six major mining areas surround the main urban area of Anshan City. It can be seen from Figure 1 that they are the Qidashan mining area, Anqian mining area, Yanqianshan mining area, Dagushan mining area, Donganshan mining area and Xiaolingzi mining area. The Qidashan, Dagushan, Donganshan and Xiaolingzi mining areas are all composed of mining areas and tailings ponds. The Anqian mining area is composed of several small iron ore stopes, and the Yanqianshan is an independent mining area. These iron mines are concentrated in Anshan’s main urban area. The mining area in the urban area is about 34 km2, or 4.2% of the overall area of the main urban area. Continuous and uninterrupted mining activities have resulted in deepening mines, increasing the height of dumps, expanding tailings ponds and bringing mines closer to urban areas in Anshan, damaging the ecological environment of the city and restricting the sustainable development of the city.
2.2 Data source and processing
Data for the study are Landsat TM/OLI surface reflectance (SR) data, administrative division data and digital elevation data. Table 1 provides details of these data. On the basis of GEE platform, we obtained and processed: 1) Landsat TM/OLI surface reflectance (SR) data with a resolution of 30 m provided by USGS. Among them, the images in 2008 were Landsat five TM SR data, and those in 2014 and 2020 were Landsat eight OLI SR data. The SR data was preprocessed by geometric correction and atmospheric correction, and the data contained the image quality assessment (QA) band obtained by the FMASK algorithm (Qiu et al., 2018). The composite algorithm was used to stitch the SR data from January to December of the current year, and the median value was selected to synthesize the image with the smallest annual cloud cover. The QA band was then used to automatically mask clouds, snow and cloud shadows to remove clouds from images (Wahap and Shafri, 2020). The vector data of the study area was uploaded to GEE to crop the three-phase remote sensing images. The cloud-free images of the study area in 2008, 2014, and 2020 were obtained respectively. 2) NASA DEM data with a resolution of 30 m released by NASA LP DAAC. NASA DEM serves as an approach to reprocessing STRM data, improving its accuracy by incorporating auxiliary data from datasets such as STER GDEM.
2.3 Sample selection
Based on the Classification of Current Land Use Status (GB/T21010-2017) and with the land cover and landscape conditions of Anshan City taken into account, the land use types in the study area were categorized into five categories: mining land, construction land, forest land, water and cultivated land (Table 2). The training samples were selected on the basis of the features of five types of land use. This study used the hyperspectral satellite Sentinel-2A data with a resolution of 10 m, combined with the 2020 Landsat-8 OLI image, to select samples in 2020. The 2008 and 2014 samples were obtained by examining Google Earth Pro historical images and Landsat TM/OLI images. The number of sample points in 2008, 2014, and 2020 were 753, 601, and 906, respectively. 70% of the samples were randomly selected as training data and the rest as test data for accuracy evaluation.
In order to obtain classification results of higher accuracy, it is necessary to introduce feature variables. This study not only selected five spectral bands of blue, green, red, near-infrared, and short-wave infrared (SWIR 1) in the Landsat image, but also used the GEE platform to calculate with the normalized difference vegetation index (NDVI), enhanced vegetation index (EVI), bare soil index (BSI), normalized difference water index (NDWI) and index-based built-up index (IBI) (Kupidura, 2019; Cui et al., 2022; Fathololoumi et al., 2022). At the same time, DEM was introduced to account for the apparent variance related to topographical factors. The above 11 feature variables were selected and introduced to the original image to improve the accuracy of image classification.
2.4 Processes and methods
2.4.1 Land use classification based on random forest
Random forest is a machine learning algorithm consisting of multiple decision trees (Ghimire et al., 2013; Zhang and Yang, 2020). It uses the bootstrap resampling technique (Breiman, 2001b) to randomly choose
The construction of the decision tree in the random forest algorithm selects some samples and features randomly, which enhances the anti-noise performance of the method and avoids overfitting to a certain extent (Breiman, 2001a; Belgiu and Drăguţ, 2016). Moreover, the random forest adopts the bagging ensemble algorithm, and multiple independent decision trees make common decisions, which not only has high accuracy but also saves time (Schmidt et al., 2019). Classification using random forests in GEE requires setting five variables: the number of decision trees, the maximum number of leaf nodes in each tree, the randomization seed, the fraction of input to bag per tree, and the number of variables per split. In this study, the amount of feature variables for each split was the square root of the total amount of feature variables, the amount of decision trees was set to 100, the fraction of input to bag per tree was 0.8, and the rest of the parameters were default values. After the above parameters in GEE had been set, the land use map of Anshan urban area in 2008, 2014, and 2020 was obtained through random forest classification (Figure 2).
Random forest classification accuracy was evaluated by confusion matrix in GEE. Through the evaluation, overall classification accuracy and Kappa coefficient were educed. Among them, the Kappa coefficient is considered to be the most representative and comprehensive calculation accuracy index. If the Kappa coefficient exceeds 0.8, it means that the simulation is almost completely consistent (He et al., 2022a). Through the confusion matrix, the overall classification accuracy in 2008, 2014, and 2020 was 90.15%, 90.74%, and 91.94% respectively, and the Kappa coefficients were all above 0.85, indicating that the classification was efficient and of high accuracy.
2.4.2 Land use dynamic degree
The dynamic degree of land use is the rate of change of the area between various land use types over a certain period and reflects the degree of change in different land-use types over the study period. It is expressed as follows (Yang et al., 2022):
Where
2.4.3 Landscape pattern based on landscape pattern index
Landscape pattern index is commonly used to quantify landscape changes and it allows quantitative monitoring and analysis of evolution in the spatial structure of the landscape (Bai et al., 2008; Fan and Ding, 2016). Landscape pattern index can reflect landscape characteristics from three levels: landscape, class and patch. Based on the landscape conditions of Anshan City, the study chose to research the landscape pattern from the two levels of landscape and class. And Fragstats4.2 was used to compute the landscape index in 2008, 2014, and 2020 respectively.
Regarding the selection of the index, in view of the morphological complexity, fragmentation, agglomeration and diversity of the landscape pattern, seven indexes including patch density (PD), perimeter-area fractal dimension (PAFRAC), landscape shape index (LSI), largest patch index (LPI), Shannon’s diversity index (SHDI), contagion (CONTAG), and Shannon’s evenness index (SHEI) were chosen to quantify the landscape pattern of Anshan urban area. The specific calculation formula and meaning of each index are given in Table 3.
2.4.4 Landscape pattern based on moving window
Using a moving window to generate a raster map can be a better way to analyze the landscape pattern from a spatial perspective. A moving window is a window with a fixed radius. It starts to move from the upper left corner. Each time it moves by one grid, the landscape index value within the window range is calculated, and the value is assigned to the center grid of the window. Finally, a raster map that can reflect the landscape pattern is formed (Hagen-Zanker, 2016). In this method, the size of the window radius may affect the landscape pattern directly. When the window radius is too small, the landscape pattern index between adjacent pixels will have a significant difference, which will easily lead to distortion of the results. When the window radius is too large, some small areas with changes will be ignored, which is not conducive to research and analysis. Therefore, in this study, 500, 800, 1000, 1200, and 1500 m were set as the window radius in Fragstats4.2. It was found that when the window radius was 1000 m, the result was the most consistent with the landscape pattern of the area. So 1000 m was used as the moving window radius to generate the raster map.
3 Result analysis
3.1 Land use
3.1.1 Land use changes
Forest land, construction land and cultivated land are the main types of land use in the urban areas of Anshan, with the sum of the three areas exceeding 95% of the total land area. Construction land is mainly in the northern plains, namely Tiexi District, Tiedong District and Lishan District. The forest land is located in the southeast of the urban area, cultivated land in the southwest and northwest of it and mining land in the east and south of it. The proportion of water is small, mainly consisting of rivers, lakes and mining subsidence stagnant water.
The transfer direction and transfer quantity of various types of land in Anshan urban area were analyzed on the basis of Figure 3. Between 2008 and 2014, cultivated land and construction land declined, while forest land, mining land, and water increased (Table 4). Cultivated land was converted to construction land and forest land, of which 5.66% was converted to construction land and 3.44% to forest land. Construction land was converted to cultivated land, forest land and mining land, of which 8.44% was converted to cultivated land, 3.64% to forest land, and 0.87% to mining land. The increased area of forest land came from construction land and cultivated land. 0.87% of construction land and 0.75% of forest land were transformed to mining land, and the mining land increased. 0.37% of the construction land was converted into water, and the water area increased. From 2014 to 2020, construction land area decreased, forest land area grew, and cultivated land, mining land and water area remained almost unchanged (Table 5). Construction land was transformed to cultivated land and forest land, of which 6.7% was converted to cultivated land and 2.49% to forest land. The increased forest land area came from construction land and 2.61% of cultivated land.
In short, from 2008 to 2020, construction land area continued to decrease, and forest land area continued to increase, with a transfer of construction land to forest land and cultivated land. Water area increased significantly from 2008 to 2014 and remained unchanged from 2014 to 2020. Mining land area grew first and then remained almost unchanged, while cultivated land area decreased first and then remained unchanged.
3.1.2 Changes in land use dynamic degree
From Figure 4, it can be noticed that the annual change rate of cultivated land from 2008 to 2020 was −0.13%, indicating that there was nearly no change. The dynamic attitude of forest land, mining land and water were 0.84%, 1.61%, and 8.1% respectively, among which only the construction land was negative. The annual change rate of water is 8.1 times that of construction land, and water is the type with the most volatile degree of change. Comparing the degree of annual change of each land use type over different periods, it is found that the dynamics from 2008 to 2014 were relatively variable, with the rate of change for each land use type from 2014 to 2020 not exceeding 1%. Among them, the dynamic degree of construction land was negative, but the values for the first 6 years are approximately 2.2 times higher than those for the last 6 years. The annual change rate of forest land in the first 6 years was 1.15%, which was about 2.3 times that in the next 6 years. The dynamic degree of mining land in the first 6 years was 3.01%, which was approximately 16.7 times that in the following 6 years. The dynamic degree of water in 2008–2014 was 15.24%, while that in 2014–2020 was only 0.5%. The dynamic degree of the water in the first 6 years was about 30.5 times that of the latter years. The change of cultivated land was the least severe. The annual change rate of cultivated land was −0.28% from 2008 to 2014 and 0.02% from 2014 to 2020.
In conclusion, the dynamics of each land use type changed markedly during the first 6 years of the research period, with flat changes in the second 6 years. From 2008 to 2020, the most significant change was in water, followed by mining land, and cultivated land almost remained unchanged. The annual change rate of construction land remained negative.
3.2 Landscape pattern analysis
3.2.1 Comprehensive analysis at the class level
For the chosen seven landscape pattern indices, considered from the class level, the indices with similar results or high overlap were excluded, and PD, LSI, LPI, and PAFRAC were chosen to identify the landscape pattern characteristics of the study area at the class level (Figure 5).
1. From 2008 to 2014, the PD and LSI of water increased, but the PAFRAC remained almost unchanged. At this stage, the management of the Nansha River has achieved remarkable results, and the previously unconnected Nansha River is gradually being connected. At the same time, the development of mining activities has caused an increase in the accumulation of water in the subsidence. Secondly, with the urban construction and agricultural development, the number of ornamental lakes and rural reservoirs in the study area has increased. Water area increased and dispersed, and the agglomeration level decreased. At the same time, the fragmentation of water has increased under the impact of human activity. From 2014 to 2020, PD, LSI, and PAFRAC of water all decreased. During this period, the scattered rural reservoirs were gradually replaced by construction land, and with the continuous reconstruction of the Nansha River, the length of the Nansha River increased. The agglomeration degree of water rose, the degree of fragmentation declined, and the shape tended to be regular.
2. PD, LSI, and PAFRAC for construction land increased from 2008 to 2014. This period saw an increase in the fragmentation degree of construction land, a tendency for fragmented patches to become more complex in shape and a decrease in overall agglomeration. The reason is that the urbanization process of Anshan City was accelerating. According to the 2011 “Anshan City Master Plan,” the new urban land would expand in conformity with the development direction of the central urban area and spread mainly to the western Dadaowan New City and the southern Tanggang New City. The construction land has continued to expand to the west and south, not only in the central urban area, but also in the scattered townships and villages in the Qianshan Mountains. From 2014 to 2020, the PD and LSI of construction land decreased, and PAFRAC remained almost unchanged. During this period, the construction land expanded outwards, constantly encroaching on other surrounding lands. The original scattered construction land was connected into large pieces with the development of the city. At this time, the agglomeration of construction land increased and the degree of fragmentation decreased. In 2008, the LSI of construction land was the highest. At this time, construction land was the dominant type in the area. With the continuous reduction of construction land, forest land became the dominant type in Anshan urban area. The LSI and PAFRAC of construction land have always been the highest over the study period, indicating that construction land is the type with the lowest degree of aggregation and the most complex patch shape among the land types.
3. The LPI of forest land did not change much. From 2008 to 2020, the forest land continued to grow and gradually became the dominant type in this area. During the study period, PD, LSI, and PAFRAC of the woodland increased continuously. Due to the increase of park green space in urban construction as well as the policy of returning farmland to forest and mining area reclamation policy, part of the arable land and construction land have been converted to forest land and its area has increased. The forest land was less clustered, more fragmented and tended to be more complex in shape.
4. From 2008 to 2014, LSI, and PAFRAC of cultivated land increased, while LPI decreased. During this period, urbanization accelerated, the original cultivated land was replaced by construction land, and the degree of dominance of cultivated land was reduced. The concentrated cultivated land was divided by built-up land, the degree of agglomeration of cultivated land was reduced, and the shape and boundary became complex and irregular. Between 2014 and 2020, LSI and PAFRAC decreased and LPI rebounded. As a result of the policy, part of the cultivated land has been restored to forest land, and the cultivated land has been concentrated into pieces under the influence of agricultural activities, showing a state of agglomeration and distribution as a whole. The agglomeration degree has increased, and the boundaries have become regularized. The patch density of arable land continued to grow over the study period, indicating that fragmentation of arable land continued to rise.
5. The PD and LSI of mining land continued to increase over the study period. PAFRAC remained almost unchanged from 2008 to 2014 and increased from 2014 to 2020, demonstrating that the fragmentation of industrial and mining land was continuously increased due to human activities during the study period. After the implementation of closed-pit mine ecological management in Anshan City in 2014, some mining areas were reclaimed and converted into forest land, the degree of agglomeration was reduced, and the shape became complex and irregular.
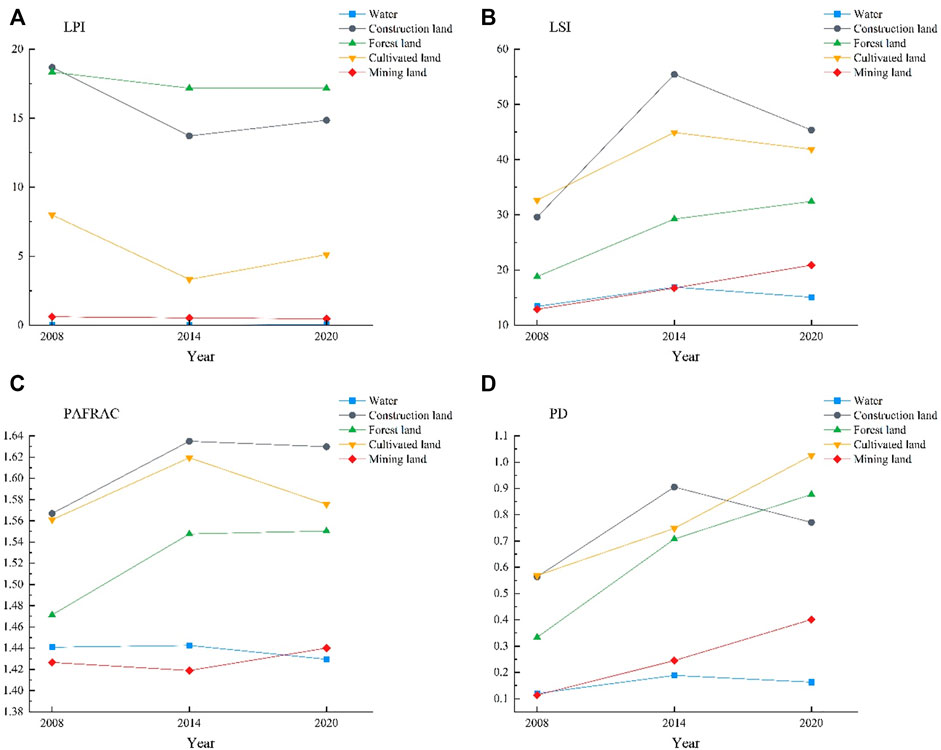
FIGURE 5. Landscape pattern index at class level. (A) change in largest patch index; (B) change in landscape shape index; (C) change in perimeter-area fractal dimension; (D) change in patch density.
3.2.2 Comprehensive analysis at the landscape level
From the landscape level, indexes that can reflect the characteristics of landscape aggregation, fragmentation and diversity were selected to study the landscape pattern of Anshan urban area (Table 6). From 2008 to 2020, PD, SHEI, and SHDI in Anshan urban area continued to increase, while CONTAG continued to decrease. In terms of the degree of change, each indicator changed more from 2008 to 2014, and slightly changed from 2014 to 2020. It is indicated that the degree of fragmentation and heterogeneity of landscape in Anshan urban area kept increasing over the study period. In particular, the degree of fragmentation and landscape heterogeneity increased significantly from 2008 to 2014.
3.2.3 Spatial level comparative analysis
The index calculation reveals that the overall fragmentation degree and landscape heterogeneity of Anshan urban area have been increased. However, due to the agglomeration of mines in the study area, it is impossible to compare the landscape pattern changes of different mining areas. Therefore, a raster map was drawn with a moving window to reflect landscape fragmentation and landscape heterogeneity in space and to compare the landscape pattern characteristics of different regions.
PD indicates the fragmentation degree of the landscape. The higher the value, the greater the degree of fragmentation. As can be seen from Figure 6, the areas with high PD values in 2008 are mainly the mining areas of Qidashan and Xiaolingzi, as well as the urban-rural areas in the southern part of the main city. Moreover, the high value areas are scattered and not connected to each other. Compared with 2008, the number of PD high-value areas increased significantly in 2014. The high-value areas are mainly divided into three areas: the urban-rural integration area in the western part of Qianshan District, the urban-rural integration area in the western part of Tiexi District, and areas where mining areas such as Anqian and Yanqianshan are concentrated. Anshan City expands to the west and the south. Both of these urban-rural areas are major areas of urban development with a fragmented distribution of arable land and building land. There is a high degree of fragmentation in both areas. With the development of mining activities, the degree of fragmentation in the Anqian mining area and the Yanqianshan mining area increased significantly, while the relative decline in the Qidashan and the Donganshan mining areas. The PD high-value areas in 2020 are similar to those in 2014, but they have expanded again compared to 2014. The high-value area surrounded the main urban area, and the original three high-value areas were connected into a ring. The reason is that Anshan City expanded from the central city to the surrounding areas and the landscape fragmentation degree around the main urban area increased during this period.
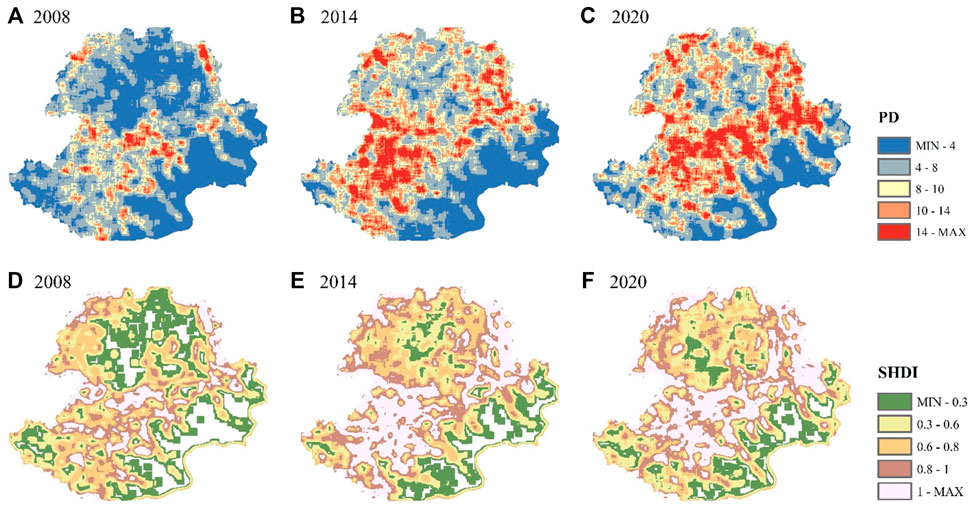
FIGURE 6. Raster map of landscape pattern in urban area of Anshan. (A) Patch density in 2008; (B) Patch density in 2014; (C) Patch density in 2020; (D) 2008 Shannon’s diversity index; (E) 2014 Shannon’s diversity Index; (F) 2020 Shannon’s diversity Index.
SHDI indicates landscape heterogeneity. The higher the value, the greater the heterogeneity. The high-value area of SHDI is nearly the same as the high-value area of PD. In 2008, the areas with high heterogeneity were mainly mining areas. In 2014, the areas with high SHDI values consisted of two parts: the urban-rural integration area in the west of Qianshan District and the mining agglomeration area in the east. With the constant development of Anshan City to the south and west, the original cultivated land and forest land have been replaced by construction land and water due to anthropogenic interventions. The landscape heterogeneity in the urban-rural integration area in the western part of Qianshan District has increased, the landscape richness has been improved, and the types have become diversified. Under the influence of mining activities, the mining area expanded and some workers built houses and farmland around the mining area, leading to the increase of landscape heterogeneity of the mining area. The high value areas in 2020 are similar to those in 2014, with increased landscape heterogeneity near the Dagushan mine, due to the implementation of mine reclamation in Anshan and the conversion of some industrial and mining land to forest land and construction land.
4 Discussion
4.1 Random forest classification optimization
With the continuous advancement of computer technology, machine learning technology is widely applied in remote sensing land use classification and mapping (Hansen et al., 2007; Liu et al., 2016a). Common classification methods include support vector machine (SVM), decision tree (DT), maximum likelihood classification (MLC), random forest (RF), etc. Yu et al. (2014) found that MLC is the most commonly used remote sensing classification method. Pal and Mather. (2006) compared the three methods of support vector machine, maximum likelihood classification and neural network and found that support vector machine accuracy is higher. Then Pal (2007) compared the support vector machine and the random forest, and found that the classification accuracy of the two methods is not much different, but the random forest needs to set fewer parameters. Lawrence and Moran (2015) compared SVM, RF, DT, and other six classification methods, and found that the classification accuracy of RF is higher than other classification methods.
Random forest has high classification accuracy. At the same time, in contrast to classification methods such as NN and SVM, this classification method can help to achieve the optimization of classification results by defining two parameters, the number of input feature variables and the number of decision trees (Rodriguez-Galiano et al., 2012; Phan et al., 2020). In this study, through the GEE platform, the random forest classifier was applied to classify remote sensing images, and the number of decision trees was adjusted in GEE to improve the classification accuracy. From 100 to 2000, 20 tests were carried out at intervals of 100 trees to observe the test accuracy (Figure 7). It is found that the test accuracy leveled off when the number of decision trees was 1700 in 2008, 200 in 2014, and 1,200 in 2020. In 2008, when the number of decision trees was changed from the initial 100 trees to 1700 trees, the classification accuracy increased by 1.6%. The optimized parameters were respectively input into the random forest classifier to improve the classification accuracy.
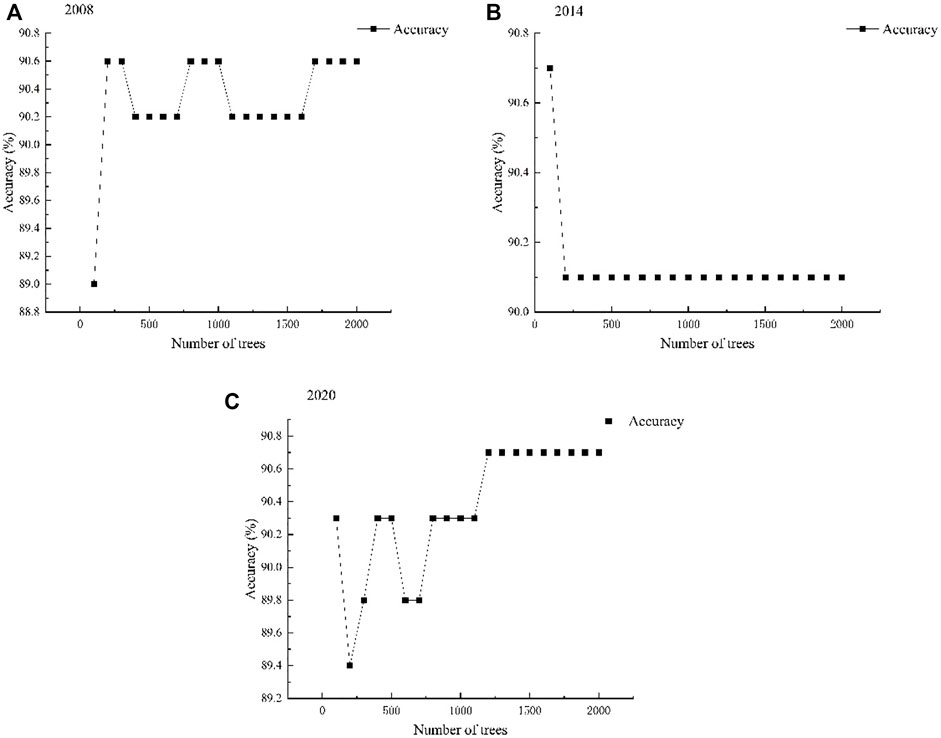
FIGURE 7. The effect of the number of decision trees on classification accuracy. (A) 2008; (B) 2014; (C) 2020.
4.2 Comparison of landscape changes in different pits
There are six large pits in the study area, and the extent of reclamation varies from mine to mine due to different mining and management patterns. Based on the spatial and temporal characteristics of the land use and landscape patterns of the six pits, it was found that the Donganshan and Dagushan mines were the most effective in terms of reclamation. These two mines have been significantly reduced in size. The Donganshan mine follows the principle of “greening while mining” and reclaims the open pit and tailings ponds, and the Yuemingshan tailings pond in the northeast of the mine and the Shannan tailings pond in the south have been effectively treated. The greening of the discharge site at the Dagushan mine has been effective and the mine area has been significantly reduced. The Donganshan and Dagushan mines have the lowest PD and SHDI values and the lowest degree of landscape fragmentation and landscape heterogeneity compared to the other mines. Reclamation of the Qidashan mine is generally effective, with no significant change in the size of the mine and consistently high PD and SHDI values, which have remained largely unchanged over the course of the study. The reclamation of the Yanqianshan, Anqian and Xiaolingzi mines is not satisfactory, as the size of these pits has increased to varying degrees due to ongoing mining activities, and PD and SHDI values have increased over time.
Based on the effectiveness of reclamation and changes in the landscape pattern, these pits are divided into three categories: 1. Mine areas with significant reclamation and low landscape fragmentation (Donganshan and Dagushan mines); 2. Mine areas with average reclamation and no increase in landscape fragmentation and heterogeneity (Qidashan mines); 3. Mine areas with unsatisfactory reclamation and increasing landscape fragmentation and heterogeneity (Yanqianshan, Anqian and Xiaolingzi mines). In the future management of mining areas in Anshan, there is an urgent need to strengthen the intensity of reclamation of Category three mining areas and improve the mining environment. Attention should be paid to changes in the landscape pattern of Category two mining areas to prevent an increase in landscape fragmentation. Continue to increase the area of greening and expand the scope of reclamation for Category one mining areas.
4.3 Factors affecting changes in land use and landscape patterns
Urbanization is one of the main drivers contributing to changes in land use and landscape patterns (Lambin et al., 2001; Deng et al., 2009; Wu et al., 2010). After the reform and opening up, urbanization in China has accelerated and a large number of people have flowed from rural areas to cities, leading to a rapid decrease in arable land and an increasing amount of land for construction (Deng et al., 2015; Zhang et al., 2020a). As Liaoyang City lies to the north of Anshan City, the urban development direction of Anshan City is mainly to the south, east and west. In 2008, Anshan City developed mainly to the south, hence the high PD value of the urban-rural area between the Donganshan and Dagushan mining areas. Urban roads are also the core areas of urban construction (Zhang et al., 2021). From 2008 to 2014, Anshan City continued to expand to the southwest along the Heida Line, and the PD value in the southwest area of Qianshan was relatively high. Between 2014 and 2020, the main city of Anshan expanded in all directions, with construction along the Anshan Ring Road, dominated by construction in the south and east. The main urban area of Anshan was surrounded by high value areas of PD and SHDI.
The evolution of land use and landscape patterns in Anshan City are obviously affected by policies. In 2007, Anshan City began to renovate the Nansha River, repairing bridge decks, building embankments and dams, regulating river water, and widening the river surface. Under the influence of river governance policies, the Nansha River basin has been connected and the water area increased. In 2014, Anshan City implemented the closed mine ecological management plan. Before 2014, with the development of mining activities, the fragmentation degree and landscape heterogeneity of the mining area increased. After 2014, mining activities in Anshan City were restricted, some mines were shifted from open pit to underground mining, and open-pit mines were gradually restored and managed. Among them, the belt rock dumping fields in the northern and central parts of the Qidashan mining area have been reclaimed, and some of them have been turned into forest land. The Dagushan and Donganshan mining areas have achieved remarkable results in reclamation, and the rock dumping fields and slopes have been treated. In 2015, Anshan City fully implemented the project of returning arable land to forest, and carried out afforestation in a planned way, and some cultivated land was changed into forest land from 2014 to 2020.
Mining activities affect land use and landscape patterns. Mining activities destroy land use and landscape structure, leading to landscape fragmentation and increased heterogeneity. At the same time, the mining industry attracts large numbers of people to the mining areas. Workers developed urban activities in the vicinity of the mines, and commercial facilities, housing, and public amenities near the mines increased.
Population also affects changes in land use and landscape patterns. According to the Anshan City Statistical Yearbook, the population of Anshan City was 1.473 million in 2008, 1.511 million in 2014, and 1.45 million in 2020. From 2008 to 2014, due to the urbanization of Anshan City and the development of mineral resources, a large number of people flowed into Anshan City, arable land decreased, and industrial and mining land increased. From 2014 to 2020, due to the transformation of the economic development model, Anshan City lost its population, the urban development slowed down, and the changes in various land use types were not obvious.
4.4 Limitations
This study has certain limitations. First, the analysis of land use and landscape pattern changes relies on the results of random forest classification. The study used Landsat data at a resolution of 30 m for land classification. At 30 m resolution, it is impossible to distinguish precisely various types of land use and then differentiate collapsed land, stope and dump in the mining area. In future research, high-resolution sentinel data can be used for land use classification, which may improve the accuracy of analysis and comparison studies on changes in subsidence, dumps and slopes in different mining areas. At the same time, this study selected 11 characteristic variables for random forest classification, which would generate a certain amount of data redundancy. In future research, characteristic variables with high correlation can be selected for training to improve classification efficiency. Secondly, the causes of variations in land use and landscape patterns are complex and diverse. The study only analyzed the impact of anthropogenic factors such as urbanization, policy, mining and population on change, and did not consider the impact of natural factors such as climate and soil on change, nor did it conduct a correlation analysis. The next step will be to conduct a principal components analysis of the drivers to clarify the main causes of change in both. Future research should focus on the evolution of land use and landscape patterns in mineral resource-based cities and areas where mining areas are concentrated, and further analyze the impact of change on regional ecosystems.
5 Conclusion
Building on the GEE platform, the study selected multi-temporal Landsat images and random forest classification algorithm to draw the land use map of Anshan urban area in 2008, 2014, and 2020. Using confusion matrices to verify the accuracy of classification. The results show that the overall classification accuracy in 3 years is more than 90% and the Kappa coefficient is more than 0.8. The classification accuracy is high. The dynamic land use attitude and the moving window method combined with the landscape pattern index were applied to analyze the spatial and temporal characteristics of land use and landscape patterns in Anshan City. The results of the study are as follows.
From 2008 to 2020, the change in land use in Anshan City District shows a continuous decline in construction land and a continuous growth in forest land, with a shift from construction land to forest land and cultivated land. The area of mining areas grew before 2014 but remained largely unchanged after mine management was carried out in Anshan City in 2014. During the study period, changes in the landscape pattern of the Anshan city area were characterized by increasing landscape fragmentation and landscape heterogeneity. The areas with high values of landscape fragmentation and heterogeneity in 2008 were the Qidashan mining area and the urban-rural combination area in the southern part of the main city. With urbanization and mining activities, the areas with high values of landscape fragmentation and landscape heterogeneity increased significantly. 2014 saw the development of Anshan City to the southwest, and the areas with severe landscape fragmentation were the urban-rural combination areas in the Qianshan District. During this phase, mining activities are frequent and landscape fragmentation is severe in the mining areas of Anshan Qian and the immediate mountain area. 2014–2020 sees little change in the landscape pattern of Anshan, but the city develops to the east, west and south, with the areas of severe landscape fragmentation being the combined urban-rural areas around the main urban area.
The six mining sites in the study area vary in their reclamation effectiveness. The best reclamation results were found at the Dagushan and Donganshan mines, where the mine area was significantly reduced and the landscape fragmentation was low. There is a need to continue to expand the greening area. The relatively good results of reclamation are in Qidashan, where the area of the mine and the degree of landscape fragmentation remain largely unchanged, but the degree of fragmentation is still high and the rate of reclamation needs to be accelerated. The least satisfactory results are found in the mining areas of Yanqianshan, Anqian and Xiaolingzi, where the size of the mining area is still increasing and the degree of landscape fragmentation continues to increase, requiring increased reclamation efforts.
Land use and landscape pattern changes in Anshan are influenced by urbanization, policies, population and mining activities. Between 2008 and 2014, Anshan experienced rapid urbanization, increased population, reduced arable land, increased industrial and mining land use, and a significant increase in landscape fragmentation. Since 2014, Anshan City has paid attention to environmental governance. Due to policies on returning farmland to forests, mining area restoration and watershed management, Anshan City set about planting trees, reclaiming mining areas and making rivers circulate. The landscape pattern and land use changes in Anshan City have slowed significantly since 2014.
Data availability statement
The original contributions presented in the study are included in the article/Supplementary Material, further inquiries can be directed to the corresponding authors.
Author contributions
YZ performed the data analysis and wrote the manuscript; YF and YZ contributed to the idea of the study; YF helped with the analysis through constructive discussions and provided valuable reviewer comments.
Funding
This work was supported by the National Natural Science Foundation of China under Grand 52074064.
Conflict of interest
The authors declare that the research was conducted in the absence of any commercial or financial relationships that could be construed as a potential conflict of interest.
Publisher’s note
All claims expressed in this article are solely those of the authors and do not necessarily represent those of their affiliated organizations, or those of the publisher, the editors and the reviewers. Any product that may be evaluated in this article, or claim that may be made by its manufacturer, is not guaranteed or endorsed by the publisher.
Supplementary material
The Supplementary Material for this article can be found online at: https://www.frontiersin.org/articles/10.3389/fenvs.2022.988346/full#supplementary-material
References
Amani, M., Ghorbanian, A., Ahmadi, S. A., Kakooei, M., Moghimi, A., Mirmazloumi, S. M., et al. (2020). Google earth engine cloud computing platform for remote sensing big data applications: A comprehensive review. IEEE J. Sel. Top. Appl. Earth Obs. Remote Sens. 13, 5326–5350. doi:10.1109/jstars.2020.3021052
Ang, M. L. E., Arts, D., Crawford, D., Labatos, B. V., Ngo, K. D., Owen, J. R., et al. (2021). Socio-environmental land cover time-series analysis of mining landscapes using Google Earth Engine and web-based mapping. Remote Sens. Appl. Soc. Environ. 21, 100458. doi:10.1016/j.rsase.2020.100458
Bai, J., Ouyang, H., Cui, B., Wang, Q., and Chen, H. (2008). Changes in landscape pattern of alpine wetlands on the Zoige Plateau in the past four decades. Acta Ecol. Sin. 28 (5), 2245–2252. doi:10.1016/s1872-2032(08)60046-3
Bajocco, S., De Angelis, A., Perini, L., Ferrara, A., and Salvati, L. (2012). The impact of land use/land cover changes on land degradation dynamics: A mediterranean case study. Environ. Manage. 49 (5), 980–989. doi:10.1007/s00267-012-9831-8
Belgiu, M., and Drăguţ, L. (2016). Random forest in remote sensing: A review of applications and future directions. ISPRS J. Photogrammetry Remote Sens. 114, 24–31. doi:10.1016/j.isprsjprs.2016.01.011
Breiman, L. (2001b). Using iterated bagging to debias regressions. Mach. Learn. 45 (3), 5–32. doi:10.1023/a:1010933404324
Bürgi, M., Hersperger, A. M., and Schneeberger, N. (2004). Driving forces of landscape change - current and new directions. Landsc. Ecol. 19 (8), 857–868. doi:10.1007/s10980-004-0245-8
Chang, Y., Hou, K., Li, X., Zhang, Y., and Chen, P. (2018). Review of land use and land cover change research progress. IOP Conf. Ser. Earth Environ. Sci. 113, 012087. doi:10.1088/1755-1315/113/1/012087
Cheung, A. K. L., Brierley, G., and O’Sullivan, D. (2016). Landscape structure and dynamics on the qinghai-Tibetan plateau. Ecol. Model. 339, 7–22. doi:10.1016/j.ecolmodel.2016.07.015
Cui, J., Zhu, M., Liang, Y., Qin, G., Li, J., and Liu, Y. (2022). Land use/land cover change and their driving factors in the yellow River basin of shandong Province based on Google earth engine from 2000 to 2020. ISPRS Int. J. Geoinf. 11 (3), 163. doi:10.3390/ijgi11030163
Dadashpoor, H., Azizi, P., and Moghadasi, M. (2019). Land use change, urbanization, and change in landscape pattern in a metropolitan area. Sci. Total Environ. 655, 707–719. doi:10.1016/j.scitotenv.2018.11.267
Deng, J. S., Wang, K., Hong, Y., and Qi, J. G. (2009). Spatio-temporal dynamics and evolution of land use change and landscape pattern in response to rapid urbanization. Landsc. Urban Plan. 92 (3-4), 187–198. doi:10.1016/j.landurbplan.2009.05.001
Deng, X., Huang, J., Rozelle, S., Zhang, J., and Li, Z. (2015). Impact of urbanization on cultivated land changes in China. Land Use Policy 45, 1–7. doi:10.1016/j.landusepol.2015.01.007
Du, P., Xia, J., Zhang, W., Tan, K., Liu, Y., and Liu, S. (2012). Multiple classifier system for remote sensing image classification: A review. Sensors (Basel) 12 (4), 4764–4792. doi:10.3390/s120404764
Fan, Q., and Ding, S. (2016). Landscape pattern changes at a county scale: A case study in fengqiu, henan Province, China from 1990 to 2013. Catena 137, 152–160. doi:10.1016/j.catena.2015.09.012
Fang, A., Dong, J., Cao, Z., Zhang, F., and Li, Y. (2019). Tempo-spatial variation of vegetation coverage and influencing factors of large-scale mining areas in eastern inner Mongolia, China. Int. J. Environ. Res. Public Health 17 (1), 47. doi:10.3390/ijerph17010047
Fathololoumi, S., Firozjaei, M. K., Li, H., and Biswas, A. (2022). Surface biophysical features fusion in remote sensing for improving land crop/cover classification accuracy. Sci. Total Environ. 156520, 156520. doi:10.1016/j.scitotenv.2022.156520
Feng, Y., Liu, Y., and Tong, X. (2018). Spatiotemporal variation of landscape patterns and their spatial determinants in Shanghai, China. Ecol. Indic. 87, 22–32. doi:10.1016/j.ecolind.2017.12.034
Gao, P., Niu, X., Wang, B., and Zheng, Y. (2015). Land use changes and its driving forces in hilly ecological restoration area based on gis and RS of northern China. Sci. Rep. 5, 11038. doi:10.1038/srep11038
Ge, H., Yi, Y., Yang, X., Yang, L., Su, D., and Ma, L. (2010). Study on ecological characteristic and reclamation in Xiangshui coal mining area, Guizhou, China. Int. J. Min. Reclam. Environ. 24 (1), 18–33. doi:10.1080/17480930903474774
Ghimire, B., Rogan, J., Galiano, V. R., Panday, P., and Neeti, N. (2013). An evaluation of bagging, boosting, and random forests for land-cover classification in cape cod, Massachusetts, USA. GIScience Remote Sens. 49 (5), 623–643. doi:10.2747/1548-1603.49.5.623
Gorelick, N., Hancher, M., Dixon, M., Ilyushchenko, S., Thau, D., and Moore, R. (2017). Google earth engine: Planetary-scale geospatial analysis for everyone. Remote Sens. Environ. 202, 18–27. doi:10.1016/j.rse.2017.06.031
Groom, G., Mücher, C. A., Ihse, M., and Wrbka, T. (2006). Remote sensing in landscape ecology: Experiences and perspectives in a European context. Landsc. Ecol. 21 (3), 391–408. doi:10.1007/s10980-004-4212-1
Hagen-Zanker, A. (2016). A computational framework for generalized moving windows and its application to landscape pattern analysis. Int. J. Appl. Earth Observation Geoinformation 44, 205–216. doi:10.1016/j.jag.2015.09.010
Hansen, M., Dubayah, R., and Defries, R. (2007). Classification trees: An alternative to traditional land cover classifiers. Int. J. Remote Sens. 17 (5), 1075–1081. doi:10.1080/01431169608949069
Hassan, M. M. (2017). Monitoring land use/land cover change, urban growth dynamics and landscape pattern analysis in five fastest urbanized cities in Bangladesh. Remote Sens. Appl. Soc. Environ. 7, 69–83. doi:10.1016/j.rsase.2017.07.001
Hayes, J. J., and Robeson, S. M. (2013). Spatial variability of landscape pattern change following a ponderosa pine wildfire in northeastern New Mexico, USA. Phys. Geogr. 30 (5), 410–429. doi:10.2747/0272-3646.30.5.410
He, F., Yang, J., Zhang, Y., Sun, D., Wang, L., Xiao, X., et al. (2022a). Offshore island connection line: A new perspective of coastal urban development boundary simulation and multi-scenario prediction. GIScience Remote Sens. 59 (1), 801–821. doi:10.1080/15481603.2022.2071056
He, Y., Oh, J., Lee, E., and Kim, Y. (2022b). Land cover and land use mapping of the east asian summer monsoon region from 1982 to 2015. Land 11 (3), 391. doi:10.3390/land11030391
Huang, J., Lin, J., and Tu, Z. (2008). Detecting spatiotemporal change of land use and landscape pattern in a coastal gulf region, southeast of China. Environ. Dev. Sustain. 12 (1), 35–48. doi:10.1007/s10668-008-9178-8
Jazouli, A. E., Barakat, A., Khellouk, R., Rais, J., and Baghdadi, M. E. (2019). Remote sensing and GIS techniques for prediction of land use land cover change effects on soil erosion in the high basin of the Oum Er Rbia River (Morocco). Remote Sens. Appl. Soc. Environ. 13, 361–374. doi:10.1016/j.rsase.2018.12.004
Kumar, L., and Mutanga, O. (2018). Google earth engine applications since inception: Usage, trends, and potential. Remote Sens. 10 (10), 1509. doi:10.3390/rs10101509
Kupidura, P. (2019). The comparison of different methods of texture analysis for their efficacy for land use classification in satellite imagery. Remote Sens. 11 (10), 1233. doi:10.3390/rs11101233
Kuzevic, S., Bobikova, D., and Kuzevicova, Z. (2022). Land cover and vegetation coverage changes in the mining area—a case study from Slovakia. Sustainability 14 (3), 1180. doi:10.3390/su14031180
Lambin, E. F., Turner, B. L., Geist, H. J., Agbola, S. B., Angelsen, A., Bruce, J. W., et al. (2001). The causes of land-use and land-cover change: Moving beyond the myths. Glob. Environ. Change 11 (4), 261–269. doi:10.1016/s0959-3780(01)00007-3
Lawrence, R. L., and Moran, C. J. (2015). The AmericaView classification methods accuracy comparison project: A rigorous approach for model selection. Remote Sens. Environ. 170, 115–120. doi:10.1016/j.rse.2015.09.008
Lei, S., Bian, Z., Daniels, J. L., and He, X. (2010). Spatio-temporal variation of vegetation in an arid and vulnerable coal mining region. Min. Sci. Technol. (China) 20 (3), 485–490. doi:10.1016/s1674-5264(09)60230-1
Li, P., Zuo, D., Xu, Z., Zhang, R., Han, Y., Sun, W., et al. (2021a). Dynamic changes of land use/cover and landscape pattern in a typical alpine river basin of the Qinghai‐Tibet Plateau, China. Land Degrad. Dev. 32 (15), 4327–4339. doi:10.1002/ldr.4039
Li, S., Wang, J., Zhang, M., and Tang, Q. (2021b). Characterizing and attributing the vegetation coverage changes in North Shanxi coal base of China from 1987 to 2020. Resour. Policy 74, 102331. doi:10.1016/j.resourpol.2021.102331
Liu, D., Hao, S., Liu, X., Li, B., He, S., and Warrington, D. N. (2012). Effects of land use classification on landscape metrics based on remote sensing and GIS. Environ. Earth Sci. 68 (8), 2229–2237. doi:10.1007/s12665-012-1905-7
Liu, J., and Deng, X. (2010). Progress of the research methodologies on the temporal and spatial process of LUCC. Chin. Sci. Bull. 55 (14), 1354–1362. doi:10.1007/s11434-009-0733-y
Liu, X., Li, X., Chen, Y., Tan, Z., Li, S., and Ai, B. (2010). A new landscape index for quantifying urban expansion using multi-temporal remotely sensed data. Landsc. Ecol. 25 (5), 671–682. doi:10.1007/s10980-010-9454-5
Liu, P., Choo, K.-K. R., Wang, L., and Huang, F. (2016a). SVM or deep learning? A comparative study on remote sensing image classification. Soft Comput. 21 (23), 7053–7065. doi:10.1007/s00500-016-2247-2
Liu, X., Zhou, W., and Bai, Z. (2016b). Vegetation coverage change and stability in large open-pit coal mine dumps in China during 1990–2015. Ecol. Eng. 95, 447–451. doi:10.1016/j.ecoleng.2016.06.051
Liu, X., An, Y., Dong, G., and Jiang, M. (2018). Land use and landscape pattern changes in the sanjiang plain, northeast China. Forests 9 (10), 637. doi:10.3390/f9100637
Magidi, J., Nhamo, L., Mpandeli, S., and Mabhaudhi, T. (2021). Application of the random forest classifier to map irrigated areas using Google earth engine. Remote Sens. 13 (5), 876. doi:10.3390/rs13050876
Mutanga, O., and Kumar, L. (2019). Google earth engine applications. Remote Sens. 11 (5), 591. doi:10.3390/rs11050591
Pal, M., and Mather, P. M. (2006). Support vector machines for classification in remote sensing. Int. J. Remote Sens. 26 (5), 1007–1011. doi:10.1080/01431160512331314083
Pal, M. (2007). Random forest classifier for remote sensing classification. Int. J. Remote Sens. 26 (1), 217–222. doi:10.1080/01431160412331269698
Peng, J., Wu, J., Yin, H., Li, Z., Chang, Q., and Mu, T. (2008). Rural land use change during 1986-2002 in lijiang, China, based on remote sensing and GIS data. Sensors (Basel) 8 (12), 8201–8223. doi:10.3390/s8128201
Pericak, A. A., Thomas, C. J., Kroodsma, D. A., Wasson, M. F., Ross, M. R. V., Clinton, N. E., et al. (2018). Mapping the yearly extent of surface coal mining in central appalachia using Landsat and Google earth engine. PLoS One 13 (7), e0197758. doi:10.1371/journal.pone.0197758
Phan, T. N., Kuch, V., and Lehnert, L. W. (2020). Land cover classification using Google earth engine and random forest classifier—the role of image composition. Remote Sens. 12 (15), 2411. doi:10.3390/rs12152411
Prasai, R., Schwertner, T. W., Mainali, K., Mathewson, H., Kafley, H., Thapa, S., et al. (2021). Application of Google earth engine python API and naip imagery for land use and land cover classification: A case study in Florida, USA. Ecol. Inf. 66, 101474. doi:10.1016/j.ecoinf.2021.101474
Qiu, S., Lin, Y., Shang, R., Zhang, J., Ma, L., and Zhu, Z. (2018). Making Landsat time series consistent: Evaluating and improving Landsat analysis ready data. Remote Sens. 11 (1), 51. doi:10.3390/rs11010051
Rodriguez-Galiano, V. F., Ghimire, B., Rogan, J., Chica-Olmo, M., and Rigol-Sanchez, J. P. (2012). An assessment of the effectiveness of a random forest classifier for land-cover classification. ISPRS J. Photogrammetry Remote Sens. 67, 93–104. doi:10.1016/j.isprsjprs.2011.11.002
Schmidt, J., Marques, M. R. G., Botti, S., and Marques, M. A. L. (2019). Recent advances and applications of machine learning in solid-state materials science. npj Comput. Mat. 5 (1), 83. doi:10.1038/s41524-019-0221-0
Schmitt, M., Bahn, M., Wohlfahrt, G., Tappeiner, U., and Cernusca, A. (2010). Land use affects the net ecosystem CO<sub>2</sub> exchange and its components in mountain grasslands. Biogeosciences 7 (8), 2297–2309. doi:10.5194/bg-7-2297-2010
Shao, G., and Wu, J. (2008). On the accuracy of landscape pattern analysis using remote sensing data. Landsc. Ecol. 23 (5), 505–511. doi:10.1007/s10980-008-9215-x
Singh, S., Singh, C., and Mukherjee, S. (2010). Impact of land-use and land-cover change on groundwater quality in the lower shiwalik hills: A remote sensing and GIS based approach. Open Geosci. 2 (2), 124–131. doi:10.2478/v10085-010-0003-x
Takam Tiamgne, X., Kalaba, F. K., and Nyirenda, V. R. (2021). Land use and cover change dynamics in Zambia's Solwezi copper mining district. Sci. Afr. 14, e01007. doi:10.1016/j.sciaf.2021.e01007
Tang, J., Li, Y., Cui, S., Xu, L., Ding, S., and Nie, W. (2020). Linking land-use change, landscape patterns, and ecosystem services in a coastal watershed of southeastern China. Glob. Ecol. Conservation 23, e01177. doi:10.1016/j.gecco.2020.e01177
Tekle, K., and Hedlund, L. (2000). Land cover changes between 1958 and 1986 in kalu district, southern wello, Ethiopia. Mt. Res. Dev. 20 (1), 42–51. doi:10.1659/0276-4741(2000)020[0042:Lccbai]2.0.Co;2
Thakkar, A. K., Desai, V. R., Patel, A., and Potdar, M. B. (2017). Post-classification corrections in improving the classification of Land Use/Land Cover of arid region using RS and GIS: The case of Arjuni watershed, Gujarat, India. Egypt. J. Remote Sens. Space Sci. 20 (1), 79–89. doi:10.1016/j.ejrs.2016.11.006
Tian, Y., Liu, B., Hu, Y., Xu, Q., Qu, M., and Xu, D. (2020). Spatio-temporal land-use changes and the response in landscape pattern to hemeroby in a resource-based city. ISPRS Int. J. Geoinf. 9 (1), 20. doi:10.3390/ijgi9010020
Townsend, P. A., Helmers, D. P., Kingdon, C. C., McNeil, B. E., de Beurs, K. M., and Eshleman, K. N. (2009). Changes in the extent of surface mining and reclamation in the Central Appalachians detected using a 1976–2006 Landsat time series. Remote Sens. Environ. 113 (1), 62–72. doi:10.1016/j.rse.2008.08.012
Turner, M. G. (1990). Spatial and temporal analysis of landscape patterns. Landsc. Ecol. 4 (1), 21–30. doi:10.1007/bf02573948
Wahap, N. A., and Shafri, H. Z. M. (2020). Utilization of Google earth engine (GEE) for land cover monitoring over klang valley, Malaysia. IOP Conf. Ser. Earth Environ. Sci. 540 (1), 012003. doi:10.1088/1755-1315/540/1/012003
Wan, L., Zhang, Y., Zhang, X., Qi, S., and Na, X. (2015). Comparison of land use/land cover change and landscape patterns in Honghe National Nature Reserve and the surrounding Jiansanjiang Region, China. Ecol. Indic. 51, 205–214. doi:10.1016/j.ecolind.2014.11.025
Wang, S., Zhao, Y., Yin, X. a., Yu, L., and Xu, F. (2009). Land use and landscape pattern changes in Nenjiang River basin during 1988–2002. Front. Earth Sci. China 4 (1), 33–41. doi:10.1007/s11707-010-0006-8
Wang, R., Cheng, J., Zhu, Y., and Xiong, W. (2016). Research on diversity of mineral resources carrying capacity in Chinese mining cities. Resour. Policy 47, 108–114. doi:10.1016/j.resourpol.2015.12.003
Wang, D., Huang, Z., Wang, Y., and Mao, J. (2021). Ecological security of mineral resource-based cities in China: Multidimensional measurements, spatiotemporal evolution, and comparisons of classifications. Ecol. Indic. 132, 108269. doi:10.1016/j.ecolind.2021.108269
Wu, Y., Zhang, X., and Shen, L. (2010). The impact of urbanization policy on land use change: A scenario analysis. Cities 28 (2), 147–159. doi:10.1016/j.cities.2010.11.002
Wu, H., Lin, A., Xing, X., Song, D., and Li, Y. (2021a). Identifying core driving factors of urban land use change from global land cover products and POI data using the random forest method. Int. J. Appl. Earth Observation Geoinformation 103, 102475. doi:10.1016/j.jag.2021.102475
Wu, J., Zhu, Q., Qiao, N., Wang, Z., Sha, W., Luo, K., et al. (2021b). Ecological risk assessment of coal mine area based on “source-sink” landscape theory – a case study of Pingshuo mining area. J. Clean. Prod. 295, 126371. doi:10.1016/j.jclepro.2021.126371
Xu, J., Zhao, H., Yin, P., Wu, L., and Li, G. (2019). Landscape ecological quality assessment and its dynamic change in coal mining area: A case study of peixian. Environ. Earth Sci. 78 (24), 708. doi:10.1007/s12665-019-8747-5
Yang, Y., Erskine, P. D., Lechner, A. M., Mulligan, D., Zhang, S., and Wang, Z. (2018). Detecting the dynamics of vegetation disturbance and recovery in surface mining area via Landsat imagery and LandTrendr algorithm. J. Clean. Prod. 178, 353–362. doi:10.1016/j.jclepro.2018.01.050
Yang, J., Yang, R., Chen, M.-H., Su, C.-H., Zhi, Y., and Xi, J. (2021). Effects of rural revitalization on rural tourism. J. Hosp. Tour. Manag. 47, 35–45. doi:10.1016/j.jhtm.2021.02.008
Yang, H., Zhong, X., Deng, S., and Nie, S. (2022). Impact of LUCC on landscape pattern in the yangtze River basin during 2001–2019. Ecol. Inf. 69, 101631. doi:10.1016/j.ecoinf.2022.101631
Yu, L., Liang, L., Wang, J., Zhao, Y., Cheng, Q., Hu, L., et al. (2014). Meta-discoveries from a synthesis of satellite-based land-cover mapping research. Int. J. Remote Sens. 35 (13), 4573–4588. doi:10.1080/01431161.2014.930206
Yu, H., Yang, J., Sun, D., Li, T., and Liu, Y. (2022). Spatial responses of ecosystem service value during the development of urban agglomerations. Land 11 (2), 165. doi:10.3390/land11020165
Zhai, M., Hu, R., Wang, Y., Jiang, S., Wang, R., Li, J., et al. (2021). Mineral resource science in China: Review and perspective. Geogr. Sustain. 2 (2), 107–114. doi:10.1016/j.geosus.2021.05.002
Zhang, F., and Yang, X. (2020). Improving land cover classification in an urbanized coastal area by random forests: The role of variable selection. Remote Sens. Environ. 251, 112105. doi:10.1016/j.rse.2020.112105
Zhang, D., Zhou, C., and Xu, W. (2020a). Spatial-temporal characteristics of primary and secondary educational resources for relocated children of migrant workers: The case of liaoning Province. Complexity 2020, 1–13. doi:10.1155/2020/7457109
Zhang, M., Wang, J., Li, S., Feng, D., and Cao, E. (2020b). Dynamic changes in landscape pattern in a large-scale opencast coal mine area from 1986 to 2015: A complex network approach. Catena 194, 104738. doi:10.1016/j.catena.2020.104738
Keywords: GEE, random forest, moving window, land use, landscape pattern, temporal and spatial evolution, anshan City
Citation: Fu Y and Zhang Y (2022) Research on temporal and spatial evolution of land use and landscape pattern in Anshan City based on GEE. Front. Environ. Sci. 10:988346. doi: 10.3389/fenvs.2022.988346
Received: 07 July 2022; Accepted: 05 August 2022;
Published: 30 August 2022.
Edited by:
Bing Xue, Institute of Applied Ecology (CAS), ChinaCopyright © 2022 Fu and Zhang. This is an open-access article distributed under the terms of the Creative Commons Attribution License (CC BY). The use, distribution or reproduction in other forums is permitted, provided the original author(s) and the copyright owner(s) are credited and that the original publication in this journal is cited, in accordance with accepted academic practice. No use, distribution or reproduction is permitted which does not comply with these terms.
*Correspondence: Yanhua Fu, fuyanhua@mail.neu.edu.cn; Yalin Zhang, 2001428@stu.neu.edu.cn