- 1State Key Laboratory of Water Environment Simulation, School of Environment, Beijing Normal University, Beijing, China
- 2Guangdong Provincial Key Laboratory of Water Quality Improvement and Ecological Restoration for Watersheds, School of Ecology, Environment and Resources, Guangdong University of Technology, Guangzhou, China
- 3Research Center for Eco-environmental Engineering, Dongguan University of Technology, Dongguan, China
Assessing food web structural properties and energy fluxes under changing hydrological regimes and water quality reveals the temporal patterns of ecosystem dynamics in shallow lakes. Here, we studied northern China’s largest shallow lake (Lake Baiyangdian) using quantitative food web models for five representative years (1958, 1980, 1993, 2009, and 2019). We analyzed the temporal patterns of food web structure and function by combining a Bayesian isotope mixing model with a food web energetics model. We further examined the temporal changes of unweighted and weighted food web topological attributes. Lake Baiyangdian changed from a detritus-based into a phytoplankton-based food web based on the relative contributions of basal food sources and energy flux distributions. The trophic position of fingerlings, large omnivorous fish, and carnivorous fish decreased with increasing eutrophication. The highest energy fluxes were from detritus to zooplankton and mollusks in 1958, from detritus and phytoplankton to zooplankton in 1980, 1993, and 2009, and from phytoplankton to zooplankton and detritus to mollusks in 2019. The highest total energy flux was in 1993, followed by 2019, with the lowest value in 1958. Unweighted food web metrics showed similar patterns. We observed more pronounced temporal variability in the node- and link-weighted food web metrics than in the unweighted metrics. In addition, hydrological factors (threshold, duration, reversals between high, and low water levels), eutrophication, and some water quality factors (chemical oxygen demand, dissolved oxygen, and pH) played important roles in the temporal changes of food web dynamics in Lake Baiyangdian. Our findings demonstrate the importance of integrating unweighted and weighted indicators to holistically comprehend how highly aggregated food webs respond to changing hydrological regimes and water quality, thereby improving management and restoration of shallow lake ecosystems.
1 Introduction
Food webs describe the trophic interactions among species or trophic groups in an ecosystem (Lynam et al., 2017; Bartley et al., 2019). Their structures reflect the effects of external disturbance, community history, and resource availability and types, thereby offering insights that can guide ecosystem management and conservation (Thompson et al., 2012; Harvey et al., 2017). However, most temporal food web studies have focused on the differences in before and after scenarios (Yletyinen et al., 2016; Ibarra-García et al., 2020) or on changes in the historical food web structure without accounting for the current state (Kong et al., 2016; Xu et al., 2016). If the management objective is to alter the food web structure to promote the ecosystem’s recovery from degradation, it is necessary to study the current food web and how it reached its current state before it is possible to identify effective restoration measures (Ushio et al., 2018; Griffith et al., 2019; Olivier et al., 2019).
In fact, the food web structure is intimately related to ecosystem functioning (Thompson et al., 2012; Hines et al., 2015). Clarifying the relationship between food web structure and ecosystem functioning is a key to understanding and predicting how an ecosystem responds to environmental change (Duffy et al., 2007; Ives et al., 2019). There is a growing interest in analyzing the temporal patterns of food web dynamics as well as linking changes in food web structure to changes in ecosystem functioning (Olivier et al., 2019; Ibarra-García et al., 2020; Kortsch et al., 2021). The energy fluxes can be regarded as a universal currency for ecosystem functioning because they describe the rate of energy flow among species or trophic groups (Barnes et al., 2018; Jochum and Eisenhauer, 2022). The food web energetics approach (Barnes et al., 2018; Gauzens et al., 2019; Jochum and Eisenhauer, 2022) combines food-web theory (Paine, 1980) with metabolic theory (Brown et al., 2004), and thereby provides a powerful tool for assessing energy fluxes. What’s more, it permits quantification of critical ecosystem processes by summing all energy fluxes from animals, autotrophs, and detritus to their respective consumers (Kortsch et al., 2021; Li et al., 2021; Jochum and Eisenhauer, 2022).
Topological analyses are useful for summarizing the structural properties of food webs by extracting ecologically meaningful information (Xu et al., 2016). For instance, Dunne et al. (2002) showed that a food web with higher complexity, defined as higher connectance (the proportion of potential links in a food web that are realized), is more robust against species loss. However, most studies of structural properties were based on unweighted food webs (also known as binary- or topology-based food webs), which only contain information about the presence and absence of trophic links between species or trophic groups (Mor et al., 2018; Kortsch et al., 2019; Ru et al., 2020). This approach assumes a static food web, and ignores the temporal variability in trophic interactions, as well as the asymmetry of energy flux distributions, and may therefore overestimate some structural properties (Banašek-Richter et al., 2009; Poisot et al., 2015). Furthermore, most research on unweighted food webs suggests that food web metrics are essentially invariant over long time scales (Olesen et al., 2011; Trøjelsgaard and Olesen, 2016; Olivier et al., 2019). In contrast, weighted food webs offer an opportunity to capture subtle temporal fluctuations in the food web structure and function through changes in species dominance or energy fluxes rather than through changes in species richness and numbers of trophic links (Boit and Gaedke, 2014; Olivier et al., 2019; Kortsch et al., 2021; Jochum and Eisenhauer, 2022). Previous studies demonstrated this difference between food web metrics calculated from unweighted and weighted food web approaches, and although the binary food web structure may appear unchanged, the weighted food web structure and function may have changed considerably, or vice versa (Bersier et al., 2002; Banašek-Richter et al., 2009). In addition, multiple aspects of food web metrics may highlight complementary patterns of temporal variability in food web dynamics associated with different ecosystem processes (Boit and Gaedke, 2014; Olivier et al., 2019; Kortsch et al., 2021). Therefore, to understand how food web structure and function change over time, unweighted metrics (topology-based) and weighted metrics (node-weighted by biomass and link-weighted by energy fluxes) should be combined in food web research because each group of metrics provides different insights.
Understanding the underlying environmental drivers that govern the temporal variability of food web structures and functions is vital to guiding the management and conservation of shallow lake ecosystems (Trøjelsgaard and Olesen, 2016; Danet et al., 2021). Driven by anthropogenic or natural changes such as dam construction and climate change, the hydrological regimes and water quality of shallow lakes are profoundly modified from their natural condition (Marino et al., 2017; Li et al., 2019; Yan et al., 2020). As a consequence, community dynamics and various ecosystem processes have been significantly degraded (Evtimova and Donohue, 2014). In addition to adversely affecting the food web structure and ecosystem functioning, these changes can damage the associated ecosystem services that support humanity (Yang and Yang, 2014a; Evtimova and Donohue, 2016; Jackson et al., 2016; Gu et al., 2019; Zhao et al., 2019; Zhang et al., 2020). For example, Marino et al. (2017) found that the degree of hydrological stability (i.e., the magnitude of the water fluctuations) affects food web structure indirectly through changes in predator–prey interactions. Lake Chaohu has suffered from gradual eutrophication since the 1950s, and the food web has evolved progressively towards a simplified structure (Gu et al., 2011; Kong et al., 2016). Moreover, a given environmental factor may elicit different responses in different metrics of the food web structure and different ecosystem functions. In particular, eutrophication may increase an ecosystem’s productivity and energy by stimulating the rapid growth of some basal organisms, such as phytoplankton, but may simultaneously decrease the trophic position of top predators and the food chain length (Post, 2002a; Xu et al., 2016). Hence, it is necessary to more fully understand how changes in hydrological regimes and water quality could influence food web structure and function (Ives et al., 2019).
In the present research, we selected Lake Baiyangdian, the largest shallow lake in northern China, as a case study. We aimed to 1) describe food web structure and energy fluxes in Lake Baiyangdian between 1958 and 2019 through analyzing some representative years and several sources (historical and novel data); 2) compare unweighted and weighted food web approaches to describe the food web’s temporal dynamics using data obtained in Lake Baiyangdian in five representative years; and 3) determine whether the hydrological regimes and some water quality factors can explain the temporal trends of food web dynamics in Lake Baiyangdian. We hypothesized that the weighted food web approach could reveal valuable insights that are not revealed by the traditional unweighted food web approach, and that temporal variation in food web structure and function are related to changes in above mentioned environmental factors.
2 Materials and methods
2.1 Study area
Lake Baiyangdian (38°44′N to 38°59′N, 115°45′E to 116°07′E) is located near the center of Hebei Province. It is the largest freshwater lake in northern China, covering an area of around 366 km2 at the mean surface level (Figure 1). Moreover, it is the main surface water source for the Xiong’an New Area and plays an important role in maintaining ecosystem functioning and constructing a more ecologically sustainable society. The lake lies in relatively flat terrain, with an elevation range of about 5.2–6.0 m for the lake’s bottom. The macrophytes in the lake consist mainly of reeds (Phragmites australis). The lake plays a vital role in providing habitat for plant and bird species, protection against floods, water purification, and providing amenities and recreational opportunities for the residents of northern China. The dominant water source is surface runoff from precipitation, which averages 560 mm per year, of which 80% falls between June and September. However, the potential evaporation of 1,369 mm is much higher than precipitation.
Before 1960, Lake Baiyangdian was dominated by natural flows, but since then, dams and reservoirs have been constructed upstream for flood prevention, crop irrigation, and human consumption (Yang and Tian, 2009; Yang and Yang, 2014b; Wang et al., 2021). The resulting hydrological alterations have decreased natural flows into the lake to almost zero, which vastly reduced the water area and water level of the lake (Yang et al., 2006). Moreover, Lake Baiyangdian’s watershed experienced severe drought, leading to further shrinkage of the lake, which nearly dried up from 1984 to 1988 (Song et al., 2018). Subsequently, heavy rainfall during a short period contributed to the filling of Lake Baiyangdian in July 1988. To mitigate the stress created by a shortage of water resources, the local government has intervened. From 1997 onwards, water from other sources (e.g., discharges of treated domestic wastewater, transfers from reservoirs in other watersheds) has been used to sustain the lake’s environmental flows, with more than 50 releases (Yang and Yang, 2014b). Due to the construction of the Xiong’an New Area in 2017 (Yuan et al., 2017), multiple sufficient artificial ecological water replenishments have promoted water level recovery in Lake Baiyangdian. Despite these inflows, the lake has become a semi-closed water body. Currently, the Fu River, Baigouyin River, and Xiaoyi River are the only three rivers that still flow into the lake. The variable frequency, quantity, and quality of the inflows have profoundly altered the hydrological regimes and water quality of Lake Baiyangdian. Consequently, changes have occurred in Lake Baiyangdian’s ecosystem. We have built five quantitative food webs for Lake Baiyangdian, representative of 1958, 1980, 1993, 2009, and 2019. The reasons we focus on these 5 years in the present study are that they represent five distinct stages in the development of the lake ecosystem mentioned above, and the historical years correspond to the times when intensive investigations were conducted in Lake Baiyangdian, with relatively abundant data available in the literature.
2.2 Historical data collection
Trophic groups are defined as groups of taxa that share the same or similar predators and prey in a food web. It is widely accepted in food web studies, where it reduces the methodological biases associated with uneven resolution of taxa within and among food webs (Williams Richard and Martinez, 2000; Dunne et al., 2002; Li et al., 2021). Based on previous studies of the lake’s food web structure (Yang, 2011; Zhang et al., 2022), the food web comprises 12 trophic groups (Table 1). We obtained historical biomass data for living trophic groups from the unpublished investigation reports of the Institute of Zoology of the Chinese Academy of Sciences (1958), the Department of Biology of Hebei University (1975), the Hebei Fisheries School (1980), and the Environmental Science Research Institute of Baoding (1993). We also referred to the research literature to supplement the historical biomass data in 1958, 1980, and 1993 (Jin, 1995; Yang et al., 2010; Zhang et al., 2022). We obtained biomass data for 2009 from four field surveys carried out from August 2009 to July 2010 by our research group and the published articles (Yang, 2011; Yang et al., 2014). Supplementary Table S1 shows the biomass of each trophic group in the first four representative historical years. We defined the theoretically possible feeding links among the 12 trophic groups from published information, qualitative records, and stable isotope analysis performed for consumers (Xu et al., 2016; Laigle et al., 2018; Olivier et al., 2019). The dietary preferences of consumer trophic groups in historical representative years (i.e., 1958, 1980, 1993, and 2009) were gathered from the gut content analysis in published reports and studies (Yang, 2011; Yang et al., 2014) of Lake Baiyangdian by our research group, and also referred to other relevant studies on Lake Baiyangdian (Ma et al., 2011; Zhang et al., 2022). In addition, daily water level data from 1958 to 2019 were derived by the Water Conservancy Office of Baoding City, Hebei Province. Water quality data for four historical years (i.e., 1958, 1980, 1993, and 2009) were provided by the Baoding Environmental Monitoring Center and Municipal Environmental Protection Bureau. Seasonal variations (i.e., spring, summer, and autumn) were considered, and we collected 6 samples of water quality data for each study year.
2.3 Sample collection
We performed field investigations in July and November 2018 and April 2019 (hereafter, “2019”). To improve the comparability of the measured data and the historical dataset, we chose the same sampling sites used in the historical reports (Figure 1). We obtained 6 water samples to quantify the water quality in 2019. Each sample consisted of 14 subsamples that were collected within 14 sampling sites. We also sampled the detritus, phytoplankton, submerged macrophytes, zooplankton, meiofauna, mollusks, and fish in each sampling site during the surveys.
2.3.1 Water sampling
We measured the water temperature (Tw), dissolved oxygen content (DO), and pH in the field using a multiparameter handheld probe (YSI Professional Plus; YSI, Yellow Springs, OH, United States). In addition, we collected 2-L water samples from the surface to a depth of 0.5 m below the surface on each sampling date, and stored the samples on ice until analysis. We measured other parameters including total phosphorus (TP), soluble reactive phosphorus (SRP), ammonium (NH4+), total nitrogen (TN), nitrite-nitrate (NO2−+NO3−), and chemical oxygen demand (COD) according to standard protocols (CBEP, 2002).
2.3.2 Biological sampling
We collected samples of detritus, phytoplankton, submerged macrophytes, zooplankton, meiofauna, mollusks, and fish on the same dates and locations used for the water sampling. Water samples of detritus (1 L), phytoplankton (5 L), and zooplankton (10 L) were collected from the surface water (to a depth of 0.5 m) with a Plexiglass water collector. Subsequently, we pre-filtered the phytoplankton and zooplankton samples by passing the samples through 200-mesh and 125-mesh plankton nets, respectively, and then concentrated them into 50 ml. To obtain samples for stable isotope analysis of the detritus, phytoplankton, and zooplankton, we passed the concentrated samples through pre-combusted (450°C for 6 h) and pre-weighed Whatman GF/F filters (glass microfiber filters; 0.45-μm pore size) under a moderate vacuum using a standard filtration system.
We collected green leaves of the submerged macrophytes by hand and washed them with distilled water to remove epiphytes. For the meiofauna and mollusk samples, we used a Van Veen grab with a mouth area of 1/16 m2, whose contents we washed through a 35-mesh filter to extract the meiofauna and mollusks and preserved the organisms in 75% ethanol. We used the whole body of the meiofauna and the muscle tissue of the mollusks for the stable isotope determination.
For the fish community, we used a multi-mesh gillnet with mesh sizes ranging from 5 to 55 mm and an overall size of 1.5 × 30 m (Mao et al., 2014), which we installed beside a ground cage. The ground cage had a mesh size of 5 mm and was 25 m long, and was partitioned into 20 sections with 10-cm openings at the front and at the back for fish to enter the trap. All fish sampling started in the late afternoon (approx. 18:00 h) and ended the following morning (approx. 06:00 h), for a total of 12 h. The duration was chosen to limit the number of fish caught per net (Prchalová et al., 2011). For each species, we chose three representative specimens, and used their muscle tissue for the stable isotope analysis. All samples were oven-dried at 60°C to a constant weight and then stored individually in a dry and ventilated place. The biomasses of trophic groups in 2019 are shown in Supplementary Table S1.
2.4 Stable isotope analysis and bayesian isotope mixing model
The stable isotope samples were crushed to a fine homogeneous powder using a ball mill. Subsamples were then pressed into ultra-pure tin capsules and analyzed using an Elementar Vario Micro-Cube elemental analyzer (Flash EA1112; Thermo Scientific, Monza, Italy) coupled with a continuous-flow isotope-ratio mass spectrometer (Delta V Advantage, Thermo Scientific, Dreieich, Germany) (Careddu et al., 2015; Hansen et al., 2018). The elemental analyzer and spectrometer were recalibrated after every 10 measurements following the manufacturer’s directions. The obtained carbon (C) and nitrogen (N) stable isotope ratios (13C:12C and 15N:14N) were expressed in δ units (deviations in ‰ from Vienna Pee Dee Belemnite (VPDB) for C and atmospheric N2 for N) according to the following equations:
All samples were analyzed twice. Measurement errors were typically smaller than ±0.1‰ for both δ13C and δ15N. The values (mean ± SD) of δ13C and δ15N of the 2019 samples are listed in Supplementary Table S2.
To elucidate the contributions of the different potential food sources to consumer trophic groups in Lake Baiyangdian in 2019, we used the Stable Isotope Analysis in R (SIAR) model (Parnell et al., 2008) with version 4.1.2 of the R software (www.r-project.org). The SIAR model is based on a Bayesian method and is fitted using a standard Markov-chain Monte Carlo method with Metropolis–Hastings steps, and we used it to generate plausible simulations and estimate the source proportions using a Dirichlet prior distribution (Parnell et al., 2010). All the potential sources for each consumer trophic group were included in the Bayesian mixing model calculations. We applied trophic enrichment factors of 0.4 ± 1.3‰ (mean ± SD) for carbon and 3.4 ± 1.0‰ for nitrogen in our model (Post, 2002b). The SIAR model performed a total of 500 000 iterations, with the first 50 000 used as the burn-in period. The output of the model was a density distribution function for plausible values of the dietary composition in terms of the proportion of the total diet accounted for by each diet item (Parnell et al., 2010). We considered a feeding link to be effective only if the lower limit of the 50% confidence interval for the contribution of each food source to a given consumer diet exceeded 5% (Careddu et al., 2015; Bentivoglio et al., 2016). On this basis, we compared the relative contribution of food sources to the food web in 2019 and the other four representative historical years.
We calculated the trophic position of the consumer trophic groups based on their dietary preferences. Producers and detritus were defined as trophic position 1, and the consumer trophic position was weighted based on the dietary preference:
where TPj is the trophic position of trophic group j; TPij is the trophic position of the food source i of trophic group j; and Pij is the relative proportion of food source i in the diet of trophic group j.
2.5 Energy fluxes among trophic groups
We calculated the energy fluxes among the trophic groups in Lake Baiyangdian’s food web for each study year, with the trophic links assigned using the food web energetics approach (Barnes et al., 2018; Gauzens et al., 2019). This approach estimates how much energy flux is needed to maintain the survival of the present community. Assuming a steady-state for the ecosystem, the model calculates energy fluxes in a top-down manner; that is, all energetic losses of each trophic group (estimated based on consumption of a resource by organisms at higher trophic levels and metabolic losses) are balanced by energetic gains after accounting for the assimilation efficiency. In other words, this means first calculating the energy flux at the top trophic level, where predation losses equal zero, then calculating the energy flux at lower trophic levels based on the losses to consumers at higher trophic levels, and iterating this process until the lowest trophic level is reached.
We defined food source preferences based on the collected quantitative diet data for the historical representative years (i.e., 1958, 1980, 1993, and 2009) and the outputs of the SIAR model for 2019. To account for differences in food source quality, we defined the assimilation efficiencies for each food source type: 0.906 for animals, 0.545 for plants, and 0.158 for detritus (Lang et al., 2017; Gauzens et al., 2019). Body mass estimates for each trophic group are specific to Lake Baiyangdian and derived from local biomonitoring data in 2019. The output is a metric of the total energy fluxes through the food web, as well as a matrix of energy fluxes for the trophic links among trophic groups (Kortsch et al., 2021). Energy flux calculations were performed using version 0.2.0 of the fluxweb package (https://cran.r-project.org/web/packages/fluxweb/index.html) for the R software.
2.6 Food web metrics
To characterize the temporal patterns of Lake Baiyangdian’s food web structure, we selected three common unweighted, topology-based metrics: connectance (C), generality (G), and vulnerability (V). We also selected six weighted metrics: the node-weighted connectance (nwC), generality (nwG), and vulnerability (nwV), and the link-weighted connectance (lwC), generality (lwG), and vulnerability (lwV). These metrics describe the complexity and horizontal dimensions of the food webs. The calculation of unweighted metrics only considered species occurrences. Connectance (C = L/S2, with L and S being the number of links and nodes, respectively) represents the number of links that exist divided by the total number of possible trophic links (Dunne et al., 2002). The generality and vulnerability properties represent the mean number of food sources per consumer and the mean number of consumers per food source, respectively (Schoener, 1989; Kortsch et al., 2015). As in the unweighted food web metrics, the node- and link-weighted metrics capture changes in the biomass and energy flux distribution among the trophic groups (Bersier et al., 2002; Olivier et al., 2019; Kortsch et al., 2021).
We also used the energy fluxes among the trophic groups to quantify ecosystem functioning. We considered six ecosystem functioning metrics (i.e., detritivory, phytoplanktivory, herbivory, zooplanktivory, benthivory, and piscivory) that were calculated as the sum of energy fluxes from detritus, phytoplankton, submerged macrophytes, zooplankton, benthos, and fish for their consumers. Here, we present each ecosystem functioning metric as a percentage of the total energy flux to the food web in each study year.
2.7 Statistical analysis
We conducted statistical analyses using version 26.0 of the SPSS software (https://www.ibm.com/analytics/spss-statistics-software). We used one-way ANOVA to identify significant temporal differences if the water quality variables satisfied the assumption of homogeneity of variance; if the result was significant at p < 0.05, we used least-significant difference (LSD) tests for pairwise comparison of variables between study years. We used Kruskal–Wallis tests to test for temporal differences if the water quality variables did not meet the assumption of homogeneity of variance; if the result was significant at p < 0.05, indicating significant differences among variables, we used the Mann–Whitney U test with Bonferroni’s correction to adjust the p values for multiple comparisons.
We used the indicators of hydrologic alteration (IHA) method (Richter et al., 1996) to calculate the values of hydrological regime factors in each study year. Then, we performed a principal component analysis (PCA) on the hydrological indicators and water quality factors to investigate their temporal dynamics using SPSS. We performed partial least-squares regression (PLSR) with SPSS and the pls package for the R software (https://cran.r-project.org/web/packages/pls/index.html) to determine the relationship between environmental factors and food web metrics. In addition, we used the variable importance in projection (VIP) and regression coefficient (RC) values from PLSR to finely screen the most important environmental factors according to their explanatory power. In our study, we considered hydrological and water quality factors with VIP ≥ 1 to be important to the food web structure, whereas those with VIP < 1 were of minor importance (Wold, 1995; Onderka et al., 2012; Zhao et al., 2020).
3 Results
3.1 Quantitative food webs in lake baiyangdian
Figure 2 illustrates the relative contributions of the various potential food sources to the diets of consumers in 2019, which we estimated using the SIAR model. We found that the zooplankton diet was composed of detritus and phytoplankton in all study years (Figure 2, Supplementary Figure S1), but phytoplankton replaced detritus as the dominant food source in 2019 (Figure 2). Meiofauna and mollusks both functioned primarily as detritivores (75.7% ± 7.7% and 63.8% ± 3.0%, respectively) in all study years. The contribution of submerged macrophytes to the diet of herbivorous fish was greater than 95% until 2019, when ingested phytoplankton (32.3%) and zooplankton (22.8%) also became significant components. Filter-feeding fish mostly consumed phytoplankton and zooplankton in all study years. Fingerlings showed a dietary preference for zooplankton (39.2% ± 1.1%) in 1958, 1980, and 1993, but shifted to a phytoplankton preference (30.7%) in 2009 and a submerged macrophytes preference (27.9%) in 2019. Small omnivorous fish initially fed mainly on submerged macrophytes, but the proportion decreased from 57.4% in 1958 to 28.5% in 2019. Large omnivorous fish had a dietary preference for mollusks in the first four historical representative years but changed to a more even preference for diverse food sources in 2019. Fingerlings and small omnivorous fish comprised a major contribution to the diet of carnivorous fish during the first 4 years, accounting for 36.1% ± 1.9% and 25.6% ± 7.08% of the total, respectively; however, in 2019, the proportions of fingerlings, small omnivorous fish, and filter-feeding fish were 21.4, 20.6, and 18.6%, respectively.
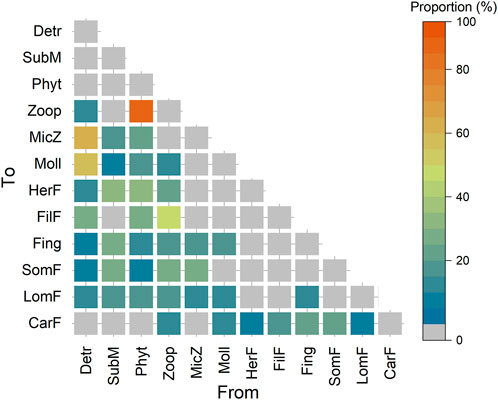
FIGURE 2. The relative contributions of the food sources to consumers in 2019. Data for the other four representative historical years are presented in Supplementary Figure S1. Abbreviations of trophic groups: CarF, carnivorous fish; Detr, detritus; FilF, filter-feeding fish; Fing, fingerlings; HerF, herbivorous fish; LomF, large omnivorous fish; MicZ, meiofauna; Moll, mollusks; Phyt, phytoplankton; SomF, small omnivorous fish; SubM, submerged macrophytes; Zoop, zooplankton.
We observed large interannual variations in the relative contributions of basal food sources to the food web (Supplementary Figure S2). For the first 4 years, detritus and submerged macrophytes were the main food sources in the food webs, accounting for averages of 30.1 and 22.1% of the total, respectively. However, the contributions of detritus and submerged macrophytes decreased to 21.4 and 15.2% in 2019, respectively. Furthermore, the contribution of phytoplankton increased from an average of 15.7% in the first three study years to 23.7% in 2009 and then became the main food source in 2019, with a contribution of 25.3%. Together, these results demonstrate a shift of the food web from detritus-based to phytoplankton-based from 1958 to 2019.
The trophic position of the trophic groups ranged from 1 (detritus, submerged macrophytes, and phytoplankton) to an average of 3.34 ± 0.13 (carnivorous fish) (Figure 3). The trophic positions of zooplankton, meiofauna, and mollusks were relatively stable, with an average value of 2.02 ± 0.03. The carnivorous fish occupied the top trophic position, but their trophic position decreased from 3.51 in 1958 to 3.21 in 2019. This was followed by large omnivorous fish (2.60 ± 0.07) and fingerlings (2.54 ± 0.10). The highest trophic positions for small omnivorous, filter-feeding, and herbivorous fish in 2019 were 2.56, 2.46, and 2.23, respectively. The lowest trophic positions for herbivorous fish, fingerlings, and small and large omnivorous fish occurred in 2009, but these values recovered slightly in 2019.
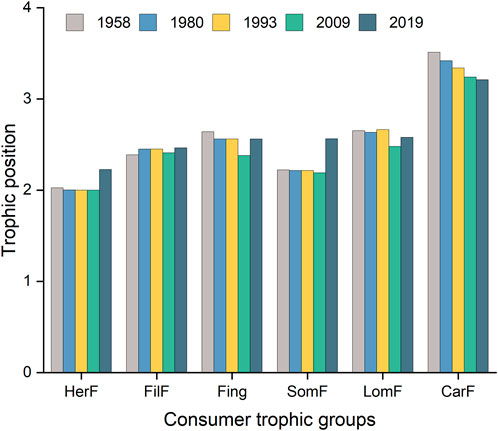
FIGURE 3. Temporal changes in the trophic positions of the six fish consumer trophic groups: CarF, carnivorous fish; FilF, filter-feeding fish; Fing, fingerlings; HerF, herbivorous fish; LomF, large omnivorous fish; SomF, small omnivorous fish.
Figure 4 illustrates the temporal changes in energy fluxes from food sources to their consumers in the food webs in the five study years. A low proportion (25.6–28.6%) of these energy fluxes was ingested by the consumer trophic groups, and a higher proportion (71.4–75.4%) of these energy fluxes entered the detritus pool. The highest total energy fluxes in the food webs were observed in 1993 (376.06 g m−2 yr−1), followed by in 2019 (227.34 g m−2 yr−1), with the lowest value in 1958 (107.18 g m−2 yr−1). The ingestion by primary consumers (zooplankton, meiofauna, and mollusks) accounted for more than 90% of the total ingestion of consumers in all study years. Among the energy fluxes along specific trophic links, the energy fluxes from detritus to zooplankton (35.70 g m−2 yr−1) and mollusks (35.24 g m−2 yr−1) were highest in 1958. The highest values characterized energy fluxes of trophic links from detritus to zooplankton, followed by values from phytoplankton to zooplankton, in three study years (1980, 1993, and 2009). Energy fluxes from phytoplankton to zooplankton (88.55 g m−2 yr−1) and from detritus to mollusks (67.98 g m−2 yr−1) were higher than those of other trophic links in 2019. Supplementary Figure S3 shows the temporal variability in flux-based ecosystem functioning metrics. The proportion of detritivorous flows increased first and then decreased over time, with the highest and lowest values observed in 1993 (75.2%) and 2019 (36.5%), respectively. The percentage of planktivorous flows showed an increasing trend, from 22.0% in 1958 to 24.7% in 2009, and became the main energy flow (49.5%) in 2019. In addition, the proportions of herbivorous, zooplanktivorous, benthivorous, and piscivorous flows in food webs showed a trend of first decreasing and then increasing over time, which were relatively higher in 2019 and 1958 than in the other three study years.
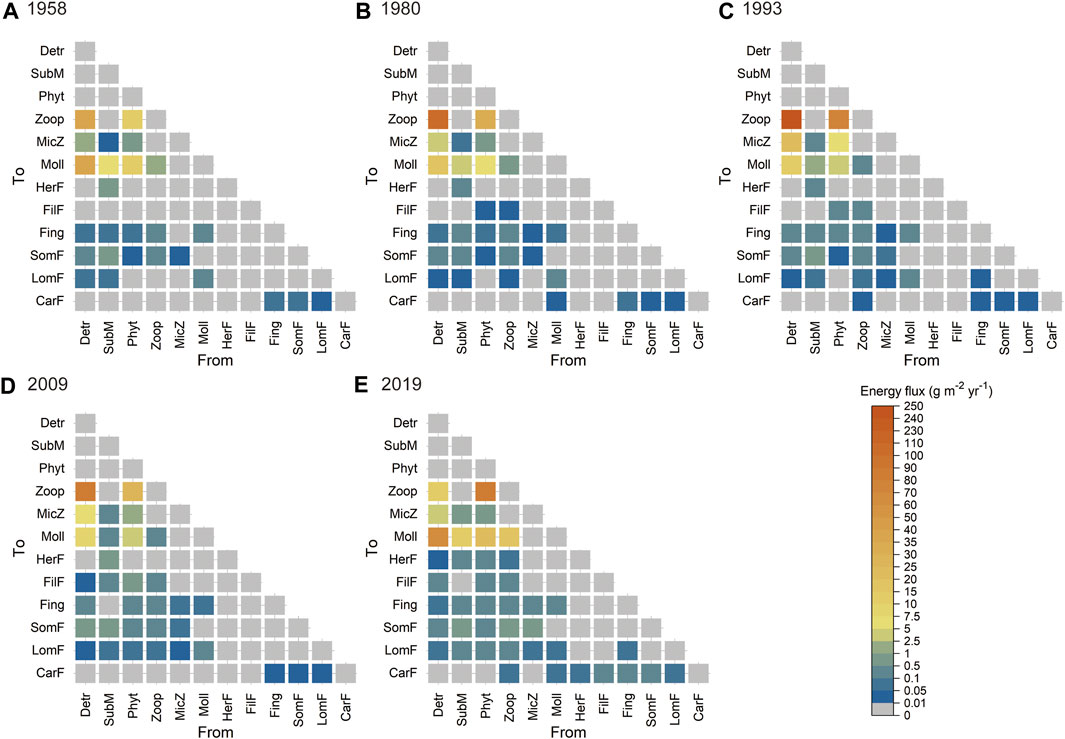
FIGURE 4. Distribution of energy fluxes from food sources to their consumers in the food webs for five representative years: (A) 1958, (B) 1980, (C) 1993, (D) 2009, and (E) 2019, represented as heat maps. Colour intensity represents the energy flux from the trophic groups in the column to the trophic groups in the row. Abbreviations of trophic groups: CarF, carnivorous fish; Detr, detritus; FilF, filter-feeding fish; Fing, fingerlings; HerF, herbivorous fish; LomF, large omnivorous fish; MicZ, meiofauna; Moll, mollusks; Phyt, phytoplankton; SomF, small omnivorous fish; SubM, submerged macrophytes; Zoop, zooplankton.
The different types of food web metrics (i.e., unweighted, node-, and link-weighted) displayed temporal variability and complementary dynamics from 1958 to 2019 (Table 2). Three unweighted food web metrics (C, G, and V) showed similar trends, with the highest values in 1980 and 2019. The highest and lowest node-weighted connectance (nwC) values were observed in 2019 and 1980, respectively. Link-weighted connectance (lwC) was highest in 1958, after which it decreased to its lowest value in 1993. Node-weighted generality (nwG) and link-weighted generality (lwG) both decreased initially and then increased, with the highest values observed in 1958 and 2019, respectively. In contrast, node-weighted vulnerability (nwV) first increased and then decreased, with the highest and lowest values in 1980 and 2019, respectively. Link-weighted vulnerability (lwV) was highest in 1958, with all other values much lower.
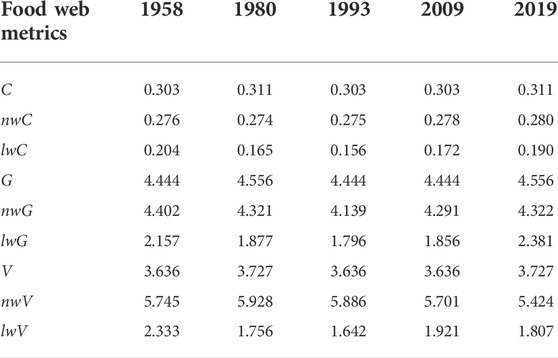
TABLE 2. Temporal changes in the unweighted and weighted (including biomass-based and flux-based) food web structure metrics. Values are the unweighted, link-weighted (lw), and node-weighted (nw) connectance (C), generality (G), and vulnerability (V).
3.2 Relationships between water environmental factors and metrics of food web structure and function
The extreme maximum and minimum water levels were highest in 1958, followed by 2019 and 1980, respectively (Supplementary Table S3). The amplitudes of the water level fluctuation represent the difference between the maximum and minimum water levels for a given period, and they were highest in 1958 and lowest in 2019. The highest pulse duration was reported in 2009, whereas the lowest frequency of hydrologic reversals was observed in 2019. The threshold of water level pulses decreased gradually from 1958 to 2009, and then partially recovered in 2019. Since hydrological indicators of the 1-day, 3-days, 7-days, 30-days, and 90-days scale water level were significantly positively correlated with each other (Supplementary Figure S4), we have focused on the 7-days scale in our descriptions, since this period is broadly representative of the other periods. Supplementary Figure S5 and Supplementary Table S4 show the PCA results for the hydrological indicators. The first two principal component axes (i.e., PC1 and PC2) account for 81.2% of the variation in hydrological regime indicators of five representative years, 56.9% and 24.3% respectively. PC1 was strongly positively associated with HPT (factor loading: 0.986), WLMaxd7 (0.981), LPT (0.910), WLMind7 (0.886), and WLAmpd7 (0.758). PC2 was strongly positively associated with HPD (0.935) and LPD (0.851).
Table 3 shows the values (mean ± SD) of the water quality variables for the five study years. All variables except for DO differed significantly among the five study years (p < 0.05). Lake Baiyangdian generally showed slightly alkaline water, with the mean pH value ranging from 7.96 to 8.72 in the five representative years, but with increasing acidity over time. Tw was highest in 2009, when it was significantly higher than in 1958 and 1980. Serious eutrophication occurred in Lake Baiyangdian, with average TN and TP concentrations ranging from 0.70 mg/L to 3.75 mg/L and from 0.03 mg/L to 0.34 mg/L, respectively, with both values increasing from 1958 to 2009, then decreasing. In addition, NO2−+NO3− was much higher in 2019 and 2009 than in the other 3 years, and this difference was significant; except for a decrease in 1993, NO2−+NO3− increased significantly from 1958 to 2019. The highest concentrations of SRP, TN, TP, and NH4+ were all observed in 2009. The average COD ranged from 12.0 mg/L to 59.2 mg/L, and increased to a maximum in 1993, and although it decreased thereafter, it remained much higher than in 1958. The PCA captures the relationship and associations in the dynamics among water quality factors in the five study years (Figure 5, Supplementary Table S5). The first two principal components (i.e., PC1 and PC2) together explained 62.8% of the variance, 43.0% and 19.8% respectively. PC1 was positively correlated with SRP (0.889), NH4+ (0.867), TN (0.827), TP (0.802), and Tw (0.730). NO2−+NO3− (−0.735) showed a negative loading on PC2, while COD (0.688) and pH (0.607) were positively correlated with this dimension. Furthermore, PCA arranged the five representative years into three groups according to the gradient of nutrient concentrations (i.e., SRP, NH4+, TN, TP, and NO2−+NO3−): 1958 and 1980 with low nutrients, 1993 and 2019 with relatively high nutrients, and 2009 with high nutrients.
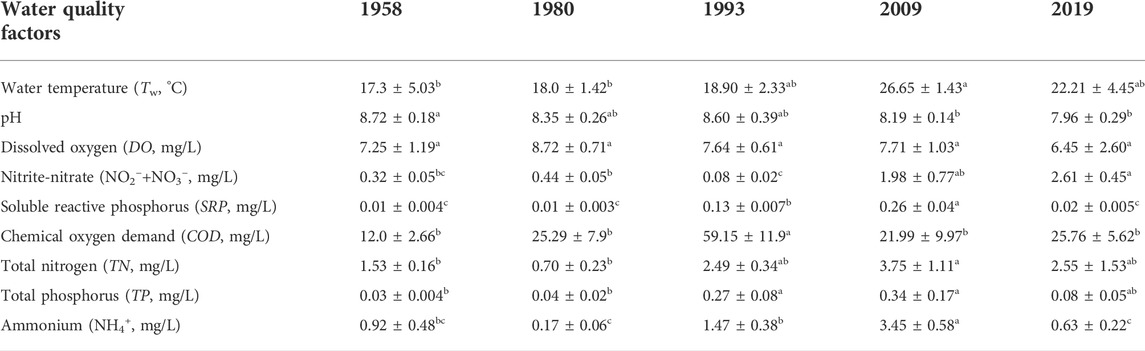
TABLE 3. Mean and standard deviation (mean ± SD) for the water quality variables in the five study years. Values of a variable followed by different letters (a, b, c) differed significantly between study years (one-way ANOVA followed by LSD test or Kruskal–Wallis test followed by Mann-Whitney U test, p < 0.05).
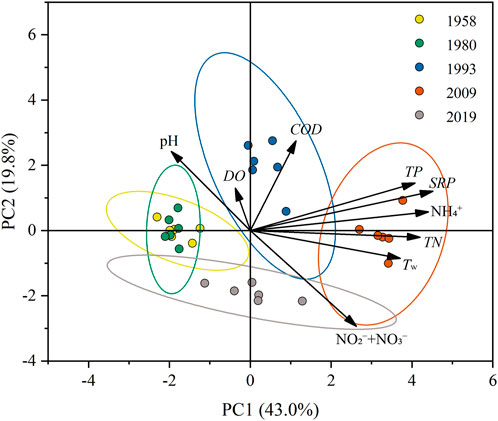
FIGURE 5. Biplot of principal component analysis (PCA) summarizing the variations in water quality factors of five representative years.
We detected minor variations (i.e., most of them are similar) in values of the unweighted food web metrics over time. Therefore, these unweighted metrics were not appropriate for further analyses. We used the VIP (Table 4) and RC (Supplementary Table S6) values from the PLSR to select the most important environmental factors that affected the food web structure and function. nwC and nwV were mainly affected by NO2−+NO3−, NumOfRev, and DO, whereas the RC values showed correlations with opposite signs for these two weighted metrics. For node-weighted generality (nwG), the highest VIP was for COD (1.936), followed by HPD and HPT (1.684 and 1.252, respectively). Link-weighted connectance (lwC) and vulnerability (lwV) were both negatively correlated with COD (VIP = 1.486 and 1.613, respectively), and positively correlated with the threshold (LPT and HPT) and duration (LPD and HPD) of the water level pulse, respectively. Link-weighted generality (lwG) was negatively correlated with DO, and positively associated with NO2−+NO3−, LPT, and WLMind7. In addition, detritivory, phytoplanktivory, and zooplanktivory were mainly affected by NO2−+NO3−, DO, pH, and NumOfRev, whereas the RC values showed correlations with opposite signs for detritivory and the other two metrics. Herbivory was positively correlated with the threshold (LPT and HPT) of the water level pulse and WLMind7, and negatively correlated with DO. Moreover, benthivory and piscivory were both negatively correlated with DO (VIP = 1.821 and 1.551, respectively), and positively correlated with LPT and NO2−+NO3−.
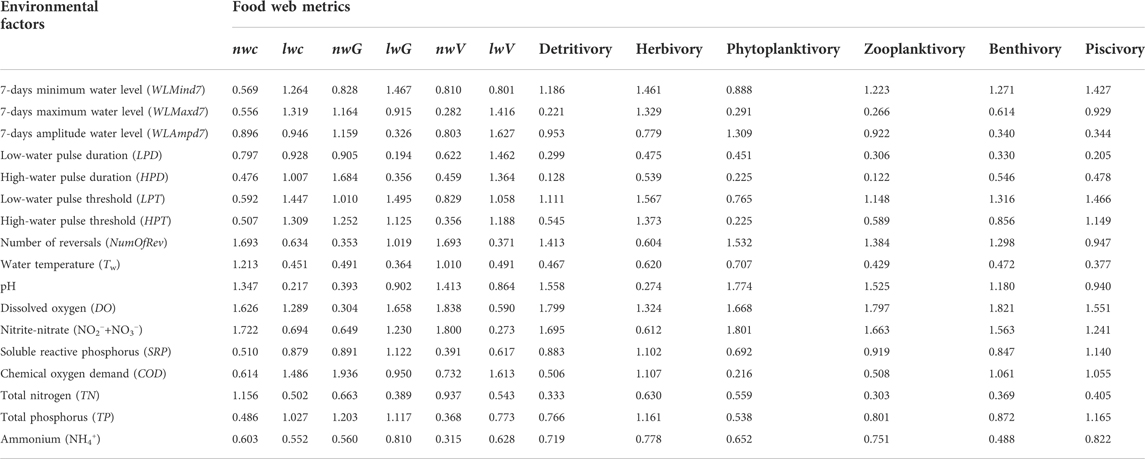
TABLE 4. Mean values of the variable importance in the projection (VIP) obtained via partial least-squares regression. Metrics are the node-weighted (nw) and link-weighted (lw) connectance (C), generality (G), and vulnerability (V), and six flow-based ecosystem functioning metrics.
4 Discussion
4.1 Temporal dynamics of the food web structure and function
In recent decades, Lake Baiyangdian has faced severe eutrophication (Zhao et al., 2010; Zeng et al., 2021). Nutrient over-enrichment associated with anthropogenic activities has accelerated eutrophication since the 1980s, leading to a high abundance of phytoplankton (Bumpers et al., 2017; Yang et al., 2021). Rogers et al. (2020) reported that grazing might have a negligible impact on phytoplankton dynamics at high phytoplankton concentrations. In addition, nutrient enrichment often alters the elemental composition of phytoplankton (Sterner and Elser, 2002; Burson et al., 2016), and often improves its nutritional quality for potential consumers. The higher nutritional enrichment of phytoplankton relative to detritus (Guo et al., 2016; Thorp and Bowes, 2017), and the associated increase in phytoplankton production, can turn detritus-based into phytoplankton-based food webs (Power et al., 2013; Mor et al., 2018), which is what happened in our study. It is acknowledged that eutrophication promotes changes in primary productivity that could potentially affect trophic interactions and alter the dietary preferences of consumers (Bumpers et al., 2017; Zhang et al., 2018; van der Lee et al., 2021). In addition, temporal changes in dietary preferences of consumers among five representative years may also be attributed to the difference in methodologies between the gut content analysis and the stable isotope analysis. For example, in a review paper on diet tracing methods in ecology, it was concluded that these two approaches measure the diet at various stages during ingestion and assimilation, and the relative dietary contribution can vary substantially (Nielsen et al., 2018). Furthermore, the contributions of detritus were the highest with averages of 30.1% in the first four study years. This may be due to the fact that the taxonomic impediment of identifying partially digested material by the gut content analysis can render some components unknown and classify them as detritus (Nielsen et al., 2018). Recently, stable carbon isotopes values of subfossils have been successfully tracked the food sources of consumers over a long-time scale (Cheng et al., 2020), which may provide an opportunity for temporal studies of highly resolved food webs.
Consumers may change their dietary preferences to take advantage of multiple food sources based on the relative availability or nutritional quality of the resources (de Carvalho et al., 2019), and this may cause changes in their trophic positions and hence in the food web structure (Rooney and McCann, 2012; McMeans et al., 2019). In the present study, three fish trophic groups (i.e., fingerlings, large omnivorous fish, and carnivorous fish) shifted their dietary preferences toward food resources with higher nutritional quality until 2009 (fingerlings and large omnivorous fish) and until 2019 (carnivorous fish), and their trophic position decreased accordingly. Similarly, some previous studies concluded that omnivorous consumers might lower their trophic position by adjusting their diet from consumption of animal resources to increased consumption of plant resources with high nutritional quality when both resources are offered simultaneously (Zhang et al., 2018; van der Lee et al., 2021). In addition, lower trophic positions of omnivorous and carnivorous fish have been reported during the wet season in tropical floodplains, leading to increased consumption of plants or invertebrates (Blanchette et al., 2014; McMeans et al., 2019). The trophic position of the top predator or the maximum trophic position of species in a food web is conceptually similar to food chain length (Briand and Cohen, 1987). Carnivorous fish consistently occupied the highest trophic position in all five representative years, and the decrease in its trophic position over time implies a shortening of the food chain length between the top and bottom of Lake Baiyangdian’s food webs, which may be caused by increased trophic omnivory and reduced dietary specialization at any or all trophic levels (Post et al., 2000; Post and Takimoto, 2007). Furthermore, the dietary preferences of consumers can shape biomass ratios between different trophic levels (McCauley et al., 2018; Burian et al., 2020). Our results showed that the biomass of trophic groups was unequally distributed across the food webs, and decreased with increasing trophic level, which is consistent with previous studies (Trebilco et al., 2013; Hatton et al., 2015).
Any changes in dietary preferences can affect energy fluxes because different food sources have different concentrations of carbon and nutrients, which leads to different assimilation efficiencies (Sterner and Elser, 2002; Jochum and Eisenhauer, 2022). The highest total energy flux in the food web was observed in 1993, followed by 2019, with the lowest in 1958. This is related to the biomass pools of phytoplankton, submerged macrophytes, and detritus, as well as the biomass flow from these pools to primary consumers. The efficiency of the energy fluxes ingested by the consumers was low, and a considerable proportion of the energy fluxes directly entered the detritus pool, where the biomass and its contained energy served as fuel for ecosystem recycling in Lake Baiyangdian’s food webs, which agrees with Zeng et al. (2021). According to Barnes et al. (2018), trophic complementarity can be an important mechanism for promoting ecosystem functioning in food webs, where greater consumer diversity reduces competition for food sources, thereby increasing the total energy fluxes to consumers (Poisot et al., 2013; Peralta et al., 2014).
In addition, the distribution of energy flux is important for determining food web stability. The ingestion by primary consumers (zooplankton, meiofauna, and mollusks) accounted for more than 90% of the total ingestion of consumers in our study. The bottom-heavy food web energetics structure (i.e., larger energy fluxes at lower trophic levels) may confer greater food web stability (Rip and McCan, 2011; Barnes et al., 2018). Zooplankton and mollusks, which dominated the total energy fluxes in Lake Baiyangdian, were strongly connected to the phytoplankton and detritus energy pathways. Both energy channels can convey significant energy fluxes from basal food sources to consumers at higher trophic levels, thereby potentially stabilizing food webs (Ward et al., 2015; Mougi, 2020). However, the primary consumers that feed on more nutritious phytoplankton can use less energetically costly mechanisms to meet their nutrient uptake, assimilation, and retention needs (Sardans et al., 2012; Teurlincx et al., 2017).
4.2 Changes in unweighted and weighted food web metrics
Although the food webs displayed large differences in the underlying dietary preferences and energy flux distribution in 1980 and 2019, or in 1958, 1993, and 2009, they showed similar values for the unweighted food web metrics. This suggests that unweighted food web metrics are inadequate predictors of highly aggregated food web structures and functions, since they are limited to a static view, and are more sensitive to changes in species and link richness (Kortsch et al., 2021). However, some studies indicated that changes in community composition did not always trigger changes in the food web structure (Yletyinen et al., 2016; Griffith et al., 2019; Frelat et al., 2022). Moreover, previous studies concluded that macroscopic unweighted food web descriptors may be invariant at a broader temporal scale (spanning years to millennia), whereas strong dynamics were observed at finer temporal scales (from hours to months) (Fründ et al., 2011; Trøjelsgaard and Olesen, 2016; CaraDonna et al., 2021). We observed more pronounced temporal variability in the weighted (node- and link-weighted) food web metrics than in the unweighted food web metrics in all study years, which can be related to the fact that unweighted and weighted food web metrics likely reflect different underlying ecosystem processes (Olivier et al., 2019; Kortsch et al., 2021; Frelat et al., 2022). Previous studies show that weighted food web metrics can capture the associated consequences of energy flux distribution and thus predict ecosystem functioning (Kortsch et al., 2021). In our study, three weighted metrics (i.e., lwC, lwG, and nwG) showed the same trend of decreasing first and then increasing over time as most metrics of ecosystem functioning (i.e., herbivory, zooplanktivory, benthivory, and piscivory), while the detritivory showed the opposite trend. Some studies of temporal changes of node-weighted food web metrics suggest that food web structure was influenced by changes in species dominance (Olivier et al., 2019). Node-weighted connectance (nwC) was highest in 2019, which can be explained by the high biomass of highly connected nodes, such as mollusks and omnivorous fish, that reflect dominance of the food web by particular trophic groups in 2019.
Node-weighted vulnerability (nwV) was always much higher than unweighted vulnerability (V) in our study, which was consistent with previous studies (Olivier et al., 2019; Kortsch et al., 2021). We also found that link-weighted metrics had lower values than the corresponding unweighted metrics in Lake Baiyangdian’s food webs. Similar results were observed in a previous study (Kortsch et al., 2021), which indicates that energy fluxes are not equally distributed in food webs, but rather are skewed towards a few strong fluxes and many weak fluxes. Link-weighted connectance (lwC) showed higher values in 1958 and 2019, which was related to the fact that several trophic groups with many interactions also had relatively high and even inflows and outflows, and this increases link-weighted food web complexity. Together these results indicate that an approach that considers the dominant species, their dietary preferences, and the energy flux distribution will challenge the conclusion about temporal changes in food web structures based only on unweighted metrics (Scotti et al., 2009; Kortsch et al., 2021; López-López et al., 2022). However, in other studies, weighted metrics were similar (Frelat et al., 2022) or complementary (Kortsch et al., 2021) to unweighted metrics in their ability to describe temporal changes in the food web structure. Therefore, it is necessary to integrate unweighted, node-weighted, and link-weighted metrics to identify the complementary temporal patterns of food web dynamics, since this provides better support for management decisions.
4.3 Effect of environmental factors on food web dynamics
The changing hydrological regimes and water quality factors that we observed can explain the temporal trends of food web dynamics. Disentangling their relationships is crucial for guiding conservation and restoration strategies in shallow lakes. Studies of ecosystem processes in shallow lakes have generally shown that water level fluctuations are a dominant force and that many other environmental factors vary concurrently with these fluctuations (Kolding and van Zwieten, 2012; Jeppesen et al., 2015). In our study, the low- and high-water level pulse thresholds (i.e., LPT and HPT) were (after COD) the hydrological regime indicators that most strongly affected the link-weighted connectance (lwC). They were positively associated with link-weighted food web complexity. Values of LPT and HPT represent variable conditions that provide a broader range of hydrologic niches that can provide conditions suitable for organisms at different trophic positions (Yan et al., 2020; Zheng et al., 2020). The number of water level reversals (NumOfRev) was negatively associated with node-weighted connectance (nwC). Frequent water level fluctuations may prevent the establishment and development of organisms, thereby decreasing biodiversity and food web complexity.
Eutrophication due to excessive nutrient loading is a common stressor for changes in food web structure and function in shallow lakes (Paerl et al., 2011). It affects the relative availability of basal resources, which further affects consumers at higher trophic positions (Bumpers et al., 2017). Eutrophication also can homogenize the composition of lake benthic assemblages (Olden et al., 2004; Donohue et al., 2009). Moreover, lakes exposed to prolonged eutrophication are prone to hypoxia, and subsequent fish kills (Bullerjahn et al., 2016). Our results showed that the biomass of phytoplankton increased from 1958 to 2009, which coincided with the intensive eutrophication in Lake Baiyangdian. As a result, most of the consumer trophic groups shifted in the direction of phytoplankton, and the food web then became more dependent on algae-based energy channels. Meanwhile, the food chain length declined from 1958 to 2009, which mean the food web structure of Lake Baiyangdian was simplified even though eutrophication brought sufficient nutrient into the ecosystem. Similar results have been reported in Lake Taihu with long-term eutrophication (Xu et al., 2016). The overall water quality of Lake Baiyangdian was better in 2019 than in 2009 and 1993, which is likely to be related to a series of governance policies and restoration projects, including a prohibition of fish breeding, water diversion projects, wastewater treatment in villages, and dredging key channels (Zhu et al., 2019; Wang et al., 2021). The highest lwC value was in 1958, followed by 2019, whereas nwC was highest in 2019, followed by 2009. Taken together, the restoration of hydrological regimes and water quality improvements contributed to improving the lake’s food web complexity by increasing the node- and link-weighted connectance in 2019. In addition, previous studies showed that most biological communities might be suppressed when a lake became eutrophication, leading to a decline in the total energy fluxes of the ecosystem (Kong et al., 2016; Wang et al., 2020). This finding is not evident in our study, as we observe the highest total energy fluxes in 1993, followed by in 2019, and the lowest value in 1958.
5 Conclusion
We confirmed our research hypotheses that the food web structure and function would vary over time in response to changes in hydrological regimes, eutrophication, and some related water quality factors. Our results revealed temporal variability of the food web structure and function in a shallow lake in northern China using both unweighted and weighted approaches and food web energetics approach. The lake changed from a detritus-based food web into a phytoplankton-based web based on the relative contributions of the basal food sources and the energy flux distribution. Fingerlings, large omnivorous fish, and carnivorous fish shifted their dietary preferences towards food sources with higher nutritional quality in the latter three study years, and their trophic position decreased accordingly. The link-weighted metrics had lower values than the corresponding unweighted topology-based metrics, which indicates that energy fluxes were not equally distributed, but rather were skewed towards a few strong fluxes and many weak fluxes. We observed more pronounced temporal variability in the weighted (node- and link-weighted) food web metrics than the unweighted food web metrics, and this may be related to the fact that unweighted and weighted food web metrics reflect different underlying ecosystem processes. Furthermore, our study demonstrated that restoring the lake’s hydrological regimes and improving the water quality contributed to improving the food web complexity and function, with increased node- and link-weighted connectance and most of the flow-based ecosystem functioning metrics values in 2019. Overall, our results suggested that studies of weighted food web metrics will complement the information provided by classical topology-based (unweighted) metrics. Our approach can therefore provide useful data to support the management and restoration of the structure and functions of shallow lake ecosystems. In future research, it will be necessary to find ways to integrate the effects of additional hydrologic and water quality metrics (e.g., the differences between long- and short-term indicators, heavy metal concentrations in the water and sediments) and to expand our analysis to spatiotemporally highly resolved food webs to improve lake ecosystem management.
Data availability statement
The original contributions presented in the study are included in the article/Supplementary Material, further inquiries can be directed to the corresponding author.
Author contributions
WY: Conceptualization, Resources, Supervision, Data analysis—original draft, Writing—review and editing. XF: Investigation, Methodology, Visualization, Data analysis—original draft, Writing—original draft. XL: Data analysis—original draft, Writing—review and editing. YY: Data analysis—original draft, Writing—review and editing.
Funding
This study was financially supported by the National Natural Science Foundation of China (No. 52079006), the National Key R&D Program of China (No. 2017YFC0404505), and the Beijing Advanced Innovation Program for Land Surface Science.
Acknowledgments
We thank Geoffrey Hart for providing language help during the writing of this paper.
Conflict of interest
The authors declare that the research was conducted in the absence of any commercial or financial relationships that could be construed as a potential conflict of interest.
Publisher’s note
All claims expressed in this article are solely those of the authors and do not necessarily represent those of their affiliated organizations, or those of the publisher, the editors and the reviewers. Any product that may be evaluated in this article, or claim that may be made by its manufacturer, is not guaranteed or endorsed by the publisher.
Supplementary material
The Supplementary Material for this article can be found online at: https://www.frontiersin.org/articles/10.3389/fenvs.2022.987600/full#supplementary-material.
References
Banašek-Richter, C., Bersier, L. F., Cattin, M. F., Baltensperger, R., Gabriel, J. P., Merz, Y., et al. (2009). Complexity in quantitative food webs. Ecology 90, 1470–1477. doi:10.1890/08-2207.1
Barnes, A. D., Jochum, M., Lefcheck, J. S., Eisenhauer, N., Scherber, C., O’Connor, M. I., et al. (2018). Energy flux: The link between multitrophic biodiversity and ecosystem functioning. Trends Ecol. Evol. 33, 186–197. doi:10.1016/j.tree.2017.12.007
Bartley, T. J., McCann, K. S., Bieg, C., Cazelles, K., Granados, M., Guzzo, M. M., et al. (2019). Food web rewiring in a changing world. Nat. Ecol. Evol. 3, 345–354. doi:10.1038/s41559-018-0772-3
Bentivoglio, F., Calizza, E., Rossi, D., Carlino, P., Careddu, G., Rossi, L., et al. (2016). Site-scale isotopic variations along a river course help localize drainage basin influence on river food webs. Hydrobiologia 770, 257–272. doi:10.1007/s10750-015-2597-2
Bersier, L. F., Banašek-Richter, C., and Cattin, M. F. (2002). Quantitative descriptors of food-web matrices. Ecology 83, 2394–2407. doi:10.1890/0012-9658(2002)083[2394:QDOFWM]2.0.CO;2
Blanchette, M. L., Davis, A. M., Jardine, T. D., and Pearson, R. G. (2014). Omnivory and opportunism characterize food webs in a large dry-tropics river system. Freshw. Sci. 33, 142–158. doi:10.1086/674632
Boit, A., and Gaedke, U. (2014). Benchmarking successional progress in a quantitative food web. PloS One 9, e90404. doi:10.1371/journal.pone.0090404
Briand, F., and Cohen, J. E. (1987). Environmental correlates of food chain length. Science 238, 956–960. doi:10.1126/science.3672136
Brown, J. H., Gillooly, J. F., Allen, A. P., Savage, V. M., and West, G. B. (2004). Toward a metabolic theory of ecology. Ecology 85, 1771–1789. doi:10.1890/03-9000
Bullerjahn, G. S., McKay, R. M., Davis, T. W., Baker, D. B., Boyer, G. L., D’Anglada, L. V., et al. (2016). Global solutions to regional problems: Collecting global expertise to address the problem of harmful cyanobacterial blooms. A Lake Erie case study. Harmful Algae 54, 223–238. doi:10.1016/j.hal.2016.01.003
Bumpers, P. M., Rosemond, A. D., Maerz, J. C., and Benstead, J. P. (2017). Experimental nutrient enrichment of forest streams increases energy flow to predators along greener food-web pathways. Freshw. Biol. 62, 1794–1805. doi:10.1111/fwb.12992
Burian, A., Nielsen, J. M., and Winder, M. (2020). Food quantity–quality interactions and their impact on consumer behavior and trophic transfer. Ecol. Monogr. 90, 1–19. doi:10.1002/ecm.1395
Burson, A., Stomp, M., Akil, L., Brussaard, C. P. D., and Huisman, J. (2016). Unbalanced reduction of nutrient loads has created an offshore gradient from phosphorus to nitrogen limitation in the North Sea. Limnol. Oceanogr. 61, 869–888. doi:10.1002/lno.10257
CaraDonna, P. J., Burkle, L. A., Schwarz, B., Resasco, J., Knight, T. M., Benadi, G., et al. (2021). Seeing through the static: The temporal dimension of plant–animal mutualistic interactions. Ecol. Lett. 24, 149–161. doi:10.1111/ele.13623
Careddu, G., Costantini, M. L., Calizza, E., Carlino, P., Bentivoglio, F., Orlandi, L., et al. (2015). Effects of terrestrial input on macrobenthic food webs of coastal sea are detected by stable isotope analysis in Gaeta Gulf. Estuar. Coast. Shelf Sci. 154, 158–168. doi:10.1016/j.ecss.2015.01.013
Cheng, L., Kattel, G., Xue, B., Yao, S., Li, L., and Liu, J. (2020). Application of subfossil Bosmina and its δ13C values in tracing the long-term food web dynamics of shallow eutrophic lakes: A case in Taihu lake, southeast China. Sci. Total Environ. 730, 138909. doi:10.1016/j.scitotenv.2020.138909
China Bureau of Environmental Protection (Cbep), (2002). Methods for monitoring and analysis of 616 water and wastewater. fourth ed. Beijing: China Environmental Science Press. (in Chinese).
Danet, A., Mouchet, M., Bonnaffé, W., Thébault, E., and Fontaine, C. (2021). Species richness and food-web structure jointly drive community biomass and its temporal stability in fish communities. Ecol. Lett. 24, 2364–2377. doi:10.1111/ele.13857
de Carvalho, D. R., de Castro, D. M. P., Callisto, M., Chaves, A. J. de M., Moreira, M. Z., and Pompeu, P. S. (2019). Stable isotopes and stomach content analyses indicate omnivorous habits and opportunistic feeding behavior of an invasive fish. Aquat. Ecol. 53, 365–381. doi:10.1007/s10452-019-09695-3
Donohue, I., Jackson, A. L., Pusch, M. T., and Irvine, K. (2009). Nutrient enrichment homo- genizes lake benthic assemblages at local and regional scales. Ecology 90, 3470–3477. doi:10.1890/09-0415.1
Duffy, J. E., Cardinale, B. J., France, K. E., McIntyre, P. B., Thébault, E., and Loreau, M. (2007). The functional role of biodiversity in ecosystems: Incorporating trophic complexity. Ecol. Lett. 10, 522–538. doi:10.1111/j.1461-0248.2007.01037.x
Dunne, J. A., Williams, R. J., and Martinez, N. D. (2002). Network structure and biodiversity loss in food webs: Robustness increases with connectance. Ecol. Lett. 5, 558–567. doi:10.1046/j.1461-0248.2002.00354.x
Evtimova, V. V., and Donohue, I. (2014). Quantifying ecological responses to amplified water level fluctuations in standing waters: An experimental approach. J. Appl. Ecol. 51, 1282–1291. doi:10.1111/1365-2664.12297
Evtimova, V. V., and Donohue, I. (2016). Water-level fluctuations regulate the structure and functioning of natural lakes. Freshw. Biol. 61, 251–264. doi:10.1111/fwb.12699
Frelat, R., Kortsch, S., Kröncke, I., Neumann, H., Nordström, M. C., Olivier, P. E. N., et al. (2022). Food web structure and community composition: A comparison across space and time in the north sea. Ecography 2022 (2). doi:10.1111/ecog.05945
Fründ, J., Dormann, C. F., and Tscharntke, T. (2011). Linné’s floral clock is slow without pollinators—Flower closure and plant-pollinator interaction webs. Ecol. Lett. 14, 896–904. doi:10.1111/j.1461-0248.2011.01654.x
Gauzens, B., Barnes, A., Giling, D. P., Hines, J., Jochum, M., Lefcheck, J. S., et al. (2019). fluxweb: an R package to easily estimate energy fluxes in food webs. Methods Ecol. Evol. 10, 270–279. doi:10.1111/2041-210X.13109
Griffith, G. P., Hop, H., Vihtakari, M., Wold, A., Kalhagen, K., and Gabrielsen, G. W. (2019). Ecological resilience of Arctic marine food webs to climate change. Nat. Clim. Chang. 9, 868–872. doi:10.1038/s41558-019-0601-y
Gu, B., Schelske, C. L., and Waters, M. N. (2011). Patterns and controls of seasonal variability of carbon stable isotopes of particulate organic matter in lakes. Oecologia 165, 1083–1094. doi:10.1007/s00442-010-1888-6
Gu, X. D., Li, N., Liu, W. L., and Xia, B. (2019). Soil moisture in relation to Lake fluctuations in a river-lake-basin system: A case study of the poyang lake region, China. Ecol. Indic. 104, 306–312. doi:10.1016/j.ecolind.2019.05.018
Guo, F., Kainz, M. J., Sheldon, F., and Bunn, S. E. (2016). The importance of high-quality algal food sources in stream food webs - current status and future perspectives. Freshw. Biol. 61, 815–831. doi:10.1111/fwb.12755
Hansen, A. G., Gardner, J. R., Connelly, K. A., Polacek, M., and Beauchamp, D. A. (2018). Trophic compression of lake food webs under hydrologic disturbance. Ecosphere 9 (6), e02304. doi:10.1002/ecs2.2304
Harvey, E., Gounand, I., Ward, C. L., and Altermatt, F. (2017). Bridging ecology and conservation: From ecological networks to ecosystem function. J. Appl. Ecol. 54, 371–379. doi:10.1111/1365-2664.12769
Hatton, I. A., McCann, K. S., Fryxell, J. M., Davies, T. J., Smerlak, M., Sinclair, A. R. E., et al. (2015). The predator-prey power law: Biomass scaling across terrestrial and aquatic biomes. Science 349 (6252), aac6284. doi:10.1126/science.aac6284
Hines, J., van der Putten, W. H., De Deyn, G. B., Wagg, C., Voigt, W., Mulder, C., et al. (2015). Towards an integration of biodiversity–ecosystem functioning and food web theory to evaluate relationships between multiple ecosystem services. Adv. Ecol. Res. 53, 161–199. doi:10.1016/bs.aecr.2015.09.001
Ibarra-García, E. C., Abarca-Arenas, L. G., Ortiz, M., and Rodríguez-Zaragoza, F. A. (2020). Impact of hurricane dean on chinchorro bank coral reef (western caribbean): Temporal variation in the food web structure. Ecol. Indic. 118, 106712. doi:10.1016/j.ecolind.2020.106712
Ives, J. T., McMeans, B. C., McCann, K. S., Fisk, A. T., Johnson, T. B., Bunnell, D. B., et al. (2019). Food-web structure and ecosystem function in the laurentian great lakes—Toward a conceptual model. Freshw. Biol. 64, 1–23. doi:10.1111/fwb.13203
Jackson, M. C., Loewen, C. J. G., Vinebrooke, R. D., and Chimimba, C. T. (2016). Net effects of multiple stressors in freshwater ecosystems: A meta-analysis. Glob. Change Biol. 22, 180–189. doi:10.1111/gcb.13028
Jeppesen, E., Brucet, S., Naselli-Flores, L., Papastergiadou, E., Stefanidis, K., Nõges, T., et al. (2015). Ecological impacts of global warming and water abstraction on lakes and reservoirs due to changes in water level and related changes in salinity. Hydrobiologia 750, 201–227. doi:10.1007/s10750-014-2169-x
Jochum, M., and Eisenhauer, N. (2022). Out of the dark: Using energy flux to connect above- and belowground communities and ecosystem functioning. Eur. J. Soil Sci. 73, 1–11. doi:10.1111/ejss.13154
Kolding, J., and van Zwieten, P. A. M. (2012). Relative lake level fluctuations and their influence on productivity and resilience in tropical lakes and reservoirs. Fish. Res. 116, 99–109. doi:10.1016/j.fishres.2011.11.008
Kong, X. Z., He, W., Liu, W. X., Yang, B., Xu, F. L., Jørgensen, S. E., et al. (2016). Changes in food web structure and ecosystem functioning of a large, shallow Chinese lake during the 1950s, 1980s and 2000s. Ecol. Modell. 319, 31–41. doi:10.1016/j.ecolmodel.2015.06.045
Kortsch, S., Frelat, R., Pecuchet, L., Olivier, P., Putnis, I., Bonsdorff, E., et al. (2021). Disentangling temporal food web dynamics facilitates understanding of ecosystem functioning. J. Anim. Ecol. 90, 1205–1216. doi:10.1111/1365-2656.13447
Kortsch, S., Primicerio, R., Aschan, M., Lind, S., Dolgov, A. V., and Planque, B. (2019). Food-web structure varies along environmental gradients in a high-latitude marine ecosystem. Ecography 42, 295–308. doi:10.1111/ecog.03443
Kortsch, S., Primicerio, R., Fossheim, M., Dolgov, A. V., and Aschan, M. (2015). Climate change alters the structure of arctic marine food webs due to poleward shifts of boreal generalists. Proc. R. Soc. B 282, 20151546. doi:10.1098/rspb.2015.1546
Laigle, I., Aubin, I., Digel, C., Brose, U., Boulangeat, I., and Gravel, D. (2018). Species traits as drivers of food web structure. Oikos 127, 316–326. doi:10.1111/oik.04712
Lang, B., Ehnes, R. B., Brose, U., and Rall, B. C. (2017). Temperature and consumer type dependencies of energy flows in natural communities. Oikos 126, 1717–1725. doi:10.1111/oik.04419
Li, X. X., Yang, W., Sun, T., and Gaedke, U. (2021). Quantitative food web structure and ecosystem functions in a warm-temperate seagrass bed. Mar. Biol. 168, 74–17. doi:10.1007/s00227-021-03878-z
Li, Y. K., Qian, F. W., Silbernagel, J., and Larson, H. (2019). Community structure, abundance variation and population trends of waterbirds in relation to water level fluctuation in Poyang Lake. J. Gt. Lakes. Res. 45, 976–985. doi:10.1016/j.jglr.2019.08.002
López-López, L., Genner, M. J., Tarling, G. A., Saunders, R. A., and O’Gorman, E. J. (2022). Ecological networks in the scotia sea: Structural changes across latitude and depth. Ecosystems 25, 457–470. doi:10.1007/s10021-021-00665-1
Lynam, C. P., Llope, M., Möllmann, C., Helaouët, P., Bayliss-Brown, G. A., and Stenseth, N. C. (2017). Interaction between top-down and bottom-up control in marine food webs. Proc. Natl. Acad. Sci. U. S. A. 114, 1952–1957. doi:10.1073/pnas.1621037114
Ma, X., Liu, C., Liu, L., Wang, Y., Wang, J., and Zhang, Y. (2011). Study on the food web of fish in baiyangdian lake based diet analysis. J. Hydroecology 32, 85–90. (In Chinese).
Mao, Z. G., Gu, X. H., Zeng, Q. F., and Pan, G. (2014). Seasonal and spatial variations of the food web structure in a shallow eutrophic lake assessed by stable isotope analysis. Fish. Sci. 80, 1045–1056. doi:10.1007/s12562-014-0771-5
Marino, N. A. C., Srivastava, D. S., MacDonald, A. A. M., Leal, J. S., Campos, A. B. A., and Farjalla, V. F. (2017). Rainfall and hydrological stability alter the impact of top predators on food web structure and function. Glob. Change Biol. 23, 673–685. doi:10.1111/gcb.13399
McCauley, D. J., Gellner, G., Martinez, N. D., Williams, R. J., Sandin, S. A., Micheli, F., et al. (2018). On the prevalence and dynamics of inverted trophic pyramids and otherwise top-heavy communities. Ecol. Lett. 21, 439–454. doi:10.1111/ele.12900
McMeans, B. C., Kadoya, T., Pool, T. K., Holtgrieve, G. W., Lek, S., Kong, H., et al. (2019). Consumer trophic positions respond variably to seasonally fluctuating environments. Ecology 100 (2), e02570. doi:10.1002/ecy.2570
Mor, J. R., Ruhí, A., Tornés, E., Valcárcel, H., Muñoz, I., and Sabater, S. (2018). Dam regulation and riverine food-web structure in a Mediterranean river. Sci. Total Environ. 625, 301–310. doi:10.1016/j.scitotenv.2017.12.296
Mougi, A. (2020). Coupling of green and Brown food webs and ecosystem stability. Ecol. Evol. 10, 9192–9199. doi:10.1002/ece3.6586
Nielsen, J. M., Clare, E. L., Hayden, B., Brett, M. T., and Kratina, P. (2018). Diet tracing in ecology: Method comparison and selection. Methods Ecol. Evol. 9, 278–291. doi:10.1111/2041-210X.12869
Olden, J. D., Poff, N. L. R., Douglas, M. R., Douglas, M. E., and Fausch, K. D. (2004). Ecological and evolutionary consequences of biotic homogenization. Trends Ecol. Evol. 19, 18–24. doi:10.1016/j.tree.2003.09.010
Olesen, J. M., Stefanescu, C., and Traveset, A. (2011). Strong, long-term temporal dynamics of an ecological network. PLoS One 6, e26455–6. doi:10.1371/journal.pone.0026455
Olivier, P., Frelat, R., Bonsdorff, E., Kortsch, S., Kröncke, I., Möllmann, C., et al. (2019). Exploring the temporal variability of a food web using long-term biomonitoring data. Ecography 42, 2107–2121. doi:10.1111/ecog.04461
Onderka, M., Wrede, S., Rodný, M., Pfister, L., Hoffmann, L., and Krein, A. (2012). Hydrogeologic and landscape controls of dissolved inorganic nitrogen (DIN) and dissolved silica (DSi) fluxes in heterogeneous catchments. J. Hydrol. X. 450–451, 36–47. doi:10.1016/j.jhydrol.2012.05.035
Paerl, H. W., Xu, H., McCarthy, M. J., Zhu, G., Qin, B., Li, Y., et al. (2011). Controlling harmful cyanobacterial blooms in a hyper-eutrophic lake (Lake Taihu, China): The need for a dual nutrient (N & P) management strategy. Water Res. 45, 1973–1983. doi:10.1016/j.watres.2010.09.018
Paine, R. T. (1980). Food webs: Linkage, interaction strength and community infrastructure. J. Anim. Ecol. 49, 666–685. doi:10.2307/4220
Parnell, A. C., Haslett, J., Allen, J. R. M., Buck, C. E., and Huntley, B. (2008). A flexible approach to assessing synchroneity of past events using Bayesian reconstructions of sedimentation history. Quat. Sci. Rev. 27, 1872–1885. doi:10.1016/j.quascirev.2008.07.009
Parnell, A. C., Inger, R., Bearhop, S., and Jackson, A. L. (2010). Source partitioning using stable isotopes: Coping with too much variation. PLoS One 5, e9672–5. doi:10.1371/journal.pone.0009672
Peralta, G., Frost, C. M., Rand, T. A., Didham, R. K., and Tylianakis, J. M. (2014). Complementarity and redundancy of interactions enhance attack rates and spatial stability in host–parasitoid food webs. Ecology 95, 1888–1896. doi:10.1890/13-1569.1
Poisot, T., Mouquet, N., and Gravel, D. (2013). Trophic complementarity drives the biodiversity–ecosystem functioning relationship in food webs. Ecol. Lett. 16, 853–861. doi:10.1111/ele.12118
Poisot, T., Stouffer, D. B., and Gravel, D. (2015). Beyond species: Why ecological interaction networks vary through space and time. Oikos 124, 243–251. doi:10.1111/oik.01719
Post, D. M., Pace, M. L., and Halrston, N. G. (2000). Ecosystem size determines food-chain length in lakes. Nature 405, 1047–1049. doi:10.1038/35016565
Post, D. M., and Takimoto, G. (2007). Proximate structural mechanisms for variation in food-chain length. Oikos 116, 775–782. doi:10.1111/j.0030-1299.2007.15552.x
Post, D. M. (2002a). The long and short of food-chain length. Trends Ecol. Evol. 17, 269–277. doi:10.1016/S0169-5347(02)02455-2
Post, D. M. (2002b). Using stable isotopes to estimate trophic position: Models, methods, and assumptions. Ecology 83, 703–718. doi:10.1890/0012-9658(2002)083[0703:USITET]2.0.CO;2
Power, M. E., Holomuzki, J. R., and Lowe, R. L. (2013). Food webs in Mediterranean rivers. Hydrobiologia 719, 119–136. doi:10.1007/s10750-013-1510-0
Prchalová, M., Mrkvička, T., Peterka, J., Čech, M., Berec, L., and Kubečka, J. (2011). A model of gillnet catch in relation to the catchable biomass, saturation, soak time and sampling period. Fish. Res. 107, 201–209. doi:10.1016/j.fishres.2010.10.021
Richter, B. D., Baumgartner, J. V., Powell, J., and Braun, D. P. (1996). A method for assessing hydrologic alteration within ecosystems. Conserv. Biol. 10, 1163–1174. doi:10.1046/j.1523-1739.1996.10041163.x
Rip, J. M. K., and McCann, K. S. (2011). Cross-ecosystem differences in stability and the principle of energy flux. Ecol. Lett. 14, 733–740. doi:10.1111/j.1461-0248.2011.01636.x
Rogers, T. L., Munch, S. B., Stewart, S. D., Palkovacs, E. P., Giron-Nava, A., Matsuzaki, S. S., et al. (2020). Trophic control changes with season and nutrient loading in lakes. Ecol. Lett. 23, 1287–1297. doi:10.1111/ele.13532
Rooney, N., and McCann, K. S. (2012). Integrating food web diversity, structure and stability. Trends Ecol. Evol. 27, 40–46. doi:10.1016/j.tree.2011.09.001
Ru, H. J., Li, Y. F., Sheng, Q., Zhong, L. Q., and Ni, Z. H. (2020). River damming affects energy flow and food web structure: A case study from a subtropical large river. Hydrobiologia 847, 679–695. doi:10.1007/s10750-019-04130-y
Sardans, J., Rivas-Ubach, A., and Peñuelas, J. (2012). The elemental stoichiometry of aquatic and terrestrial ecosystems and its relationships with organismic lifestyle and ecosystem structure and function: A review and perspectives. Biogeochemistry 111, 1–39. doi:10.1007/s10533-011-9640-9
Schoener, T. W. (1989). Food webs from the small to the large: The robert H. MacArthur award lecture. Ecology 70, 1559–1589. doi:10.2307/1938088
Scotti, M., Bondavalli, C., Bodini, A., and Allesina, S. (2009). Using trophic hierarchy to understand food web structure. Oikos 118, 1695–1702. doi:10.1111/j.1600-0706.2009.17073.x
Song, C. Q., Ke, L. H., Pan, H., Zhan, S. G., Liu, K., and Ma, R. H. (2018). Long-term surface water changes and driving cause in xiong’an, China: From dense landsat time series images and synthetic analysis. Sci. Bull. (Beijing). 63, 708–716. doi:10.1016/j.scib.2018.05.002
Sterner, R. W., and Elser, J. J. (2002). Ecological stoichiometry. Princeton, New Jersey, USA: Princeton University Press.
Teurlincx, S., Velthuis, M., Seroka, D., Govaert, L., van Donk, E., Van de Waal, D. B., et al. (2017). Species sorting and stoichiometric plasticity control community C:P ratio of first-order aquatic consumers. Ecol. Lett. 20, 751–760. doi:10.1111/ele.12773
Thompson, R. M., Brose, U., Dunne, J. A., Hall, R. O., Hladyz, S., Kitching, R. L., et al. (2012). Food webs: Reconciling the structure and function of biodiversity. Trends Ecol. Evol. 27, 689–697. doi:10.1016/j.tree.2012.08.005
Thorp, J. H., and Bowes, R. E. (2017). Carbon sources in riverine food webs: New evidence from amino acid isotope techniques. Ecosystems 20, 1029–1041. doi:10.1007/s10021-016-0091-y
Trebilco, R., Baum, J. K., Salomon, A. K., and Dulvy, N. K. (2013). Ecosystem ecology: Size-based constraints on the pyramids of life. Trends Ecol. Evol. 28, 423–431. doi:10.1016/j.tree.2013.03.008
Trøjelsgaard, K., and Olesen, J. M. (2016). Ecological networks in motion: Micro- and macroscopic variability across scales. Funct. Ecol. 30, 1926–1935. doi:10.1111/1365-2435.12710
Ushio, M., Hsieh, C. H., Masuda, R., Deyle, E. R., Ye, H., Chang, C. W., et al. (2018). Fluctuating interaction network and time-varying stability of a natural fish community. Nature 554, 360–363. doi:10.1038/nature25504
van der Lee, G. H., Vonk, J. A., Verdonschot, R. C. M., Kraak, M. H. S., Verdonschot, P. F. M., and Huisman, J. (2021). Eutrophication induces shifts in the trophic position of invertebrates in aquatic food webs. Ecology 102, e03275–13. doi:10.1002/ecy.3275
Wang, M. X., Yao, Y., Shen, Q., Gao, H. J., Li, J. S., Zhang, F. F., et al. (2021). Time-series analysis of surface-water quality in xiong’an new area, 2016–2019. J. Indian Soc. Remote Sens. 49, 857–872. doi:10.1007/s12524-020-01264-8
Wang, S. C., Liu, X., Liu, Y., and Wang, H. (2020). Benthic-pelagic coupling in lake energetic food webs. Ecol. Modell. 417, 108928. doi:10.1016/j.ecolmodel.2019.108928
Ward, C. L., McCann, K. S., and Rooney, N. (2015). HSS revisited: Multi-channel processes mediate trophic control across a productivity gradient. Ecol. Lett. 18, 1190–1197. doi:10.1111/ele.12498
Williams Richard, J., and Martinez, N. D. (2000). Simple rules yield complex food webs. Nature 404, 180–183. doi:10.1038/35004572
Wold, S. 1995. “Chemometric methods in molecular design methods and principles in medicinal chemistry,” in PLS for multivariate linear modeling, H. van deWaterbeemd, Ed., pp.195–218. Verlag-Chemie,Weinheim, Germany.
Xu, D. L., Cai, Y., Jiang, H., Wu, X. Q., Leng, X., and An, S. Q. (2016). Variations of food web structure and energy availability of shallow lake with long-term eutrophication: A case study from Lake Taihu, China. Clean. Soil Air Water 44, 1306–1314. doi:10.1002/clen.201300837
Yang, Y., Chen, H., and Yang, Z. F. (2010). Assessing changes of trophic interactions during once anthropogenic water supplement in Baiyangdian Lake. International Conference on Ecological Informatics and Ecosystem Conservation (ISEIS), 2. Beijing: Procedia Environmental Sciences, 1169–1179.
Yan, S. J., Wang, X., Zhang, Y. L., Liu, D., Yi, Y. J., Li, C. H., et al. (2020). A hybrid PCA–GAM model for investigating the spatiotemporal impacts of water level fluctuations on the diversity of benthic macroinvertebrates in Baiyangdian Lake, North China. Ecol. Indic. 116, 106459. doi:10.1016/j.ecolind.2020.106459
Yang, J., Strokal, M., Kroeze, C., Chen, X. J., Bai, Z. H., Li, H. B., et al. (2021). Seasonal river export of nitrogen to guanting and baiyangdian lakes in the hai He basin. J. Geophys. Res. Biogeosci. 126, 1–20. doi:10.1029/2020JG005689
Yang, W., and Yang, Z. F. (2014b). Effects of long-term environmental flow releases on the restoration and preservation of Baiyangdian Lake, a regulated Chinese freshwater lake. Hydrobiologia 730, 79–91. doi:10.1007/s10750-014-1823-7
Yang, W., and Yang, Z. F. (2014a). Integrating ecosystem-service tradeoffs into environmental flows decisions for Baiyangdian Lake. Ecol. Eng. 71, 539–550. doi:10.1016/j.ecoleng.2014.07.065
Yang, Y. H., and Tian, F. (2009). Abrupt change of runoff and its major driving factors in Haihe River Catchment, China. J. Hydrol. X. 374, 373–383. doi:10.1016/j.jhydrol.2009.06.040
Yang, Y. (2011). Study on ecological water demand based on the dynamics of food web structure and function in the Baiyangdian wetland. China: Beijing Normal University.
Yang, Y., Yin, X. A., Chen, H., and Yang, Z. F. (2014). Determining water level management strategies for lake protection at the ecosystem level. Hydrobiologia 738, 111–127. doi:10.1007/s10750-014-1923-4
Yang, Y., Yin, X. A., and Yang, Z. F. (2016). Environmental flow management strategies based on the integration of water quantity and quality, a case study of the Baiyangdian Wetland, China. Ecol. Eng. 96, 150–161. doi:10.1016/j.ecoleng.2015.12.018
Yletyinen, J., Bodin, Ö., Weigel, B., Nordström, M. C., Bonsdorff, E., and Blenckner, T. (2016). Regime shifts in marine communities: A complex systems perspective on food web dynamics. Proc. R. Soc. B 283 (1825), 20152569. doi:10.1098/rspb.2015.2569
Yuan, R. Q., Wang, S. Q., Wang, P., Song, X. F., and Tang, C. Y. (2017). Changes in flow and chemistry of groundwater heavily affected by human impacts in the Baiyangdian catchment of the North China Plain. Environ. Earth Sci. 76, 571. doi:10.1007/s12665-017-6918-9
Zeng, Y., Zhao, Y. W., and Qi, Z. F. (2021). Evaluating the ecological state of Chinese Lake Baiyangdian (BYD) based on ecological network analysis. Ecol. Indic. 127, 107788. doi:10.1016/j.ecolind.2021.107788
Zhang, J. H., Huang, T., Chen, L., Zhu, D. Z., Zhu, L. L., Feng, L. J., et al. (2020). Impact of the three gorges reservoir on the hydrologic regime of the river-lake system in the middle yangtze river. J. Clean. Prod. 258, 121004. doi:10.1016/j.jclepro.2020.121004
Zhang, P., Van Den Berg, R. F., Van Leeuwen, C. H. A., Blonk, B. A., and Bakker, E. S. (2018). Aquatic omnivores shift their trophic position towards increased plant consumption as plant stoichiometry becomes more similar to their body stoichiometry. PLoS One 13, e0204116–13. doi:10.1371/journal.pone.0204116
Zhang, X. X., Yi, Y. J., and Yang, Z. F. (2022). The long-term changes in food web structure and ecosystem functioning of a shallow lake: Implications for the lake management. J. Environ. Manage. 301, 113804. doi:10.1016/j.jenvman.2021.113804
Zhao, C. S., Yang, Y., Yang, S. T., Xiang, H., Ge, Y. R., Zhang, Z. S., et al. (2020). Effects of spatial variation in water quality and hydrological factors on environmental flows. Sci. Total Environ. 728, 138695. doi:10.1016/j.scitotenv.2020.138695
Zhao, C. S., Yang, Y., Yang, S. T., Xiang, H., Wang, F., Chen, X., et al. (2019). Impact of spatial variations in water quality and hydrological factors on the food-web structure in urban aquatic environments. Water Res. 153, 121–133. doi:10.1016/j.watres.2019.01.015
Zhao, Y., Yang, Z. F., and Li, Y. X. (2010). Investigation of water pollution in baiyangdian lake, China. Procedia Environ. Sci. 2, 737–748. doi:10.1016/j.proenv.2010.10.085
Zheng, L. I., Zhan, P. F., Xu, J. Y., Xu, L. G., Tan, Z. Q., and Wang, X. L. (2020). Aquatic vegetation dynamics in two pit lakes related to interannual water level fluctuation. Hydrol. Process. 34, 2645–2659. doi:10.1002/hyp.13757
Keywords: food web structure, ecosystem functioning, energy fluxes, temporal dynamics, hydrological regimes, eutrophication
Citation: Yang W, Fu X, Li X and Yang Y (2022) Temporal changes of the food web structure and function driven by changes in hydrological regimes and water quality in a large shallow lake. Front. Environ. Sci. 10:987600. doi: 10.3389/fenvs.2022.987600
Received: 06 July 2022; Accepted: 15 August 2022;
Published: 09 September 2022.
Edited by:
Celso Santos, Federal University of Paraíba, BrazilReviewed by:
Naoto F. Ishikawa, Japan Agency for Marine-Earth Science and Technology (JAMSTEC), JapanDiego Frau, National Institute of Limnology (INALI), Argentina
Copyright © 2022 Yang, Fu, Li and Yang. This is an open-access article distributed under the terms of the Creative Commons Attribution License (CC BY). The use, distribution or reproduction in other forums is permitted, provided the original author(s) and the copyright owner(s) are credited and that the original publication in this journal is cited, in accordance with accepted academic practice. No use, distribution or reproduction is permitted which does not comply with these terms.
*Correspondence: Wei Yang, yangwei@bnu.edu.cn