- College of Economics and Management, Zhejiang Ocean University, Zhoushan, China
The environmental problems caused by excessive waste discharge are becoming more and more prominent, and how to deal with waste has become a problem that has to be done but is extremely costly. On the other hand, from a value chain perspective, waste has been focused on because of its potential value for conversion. Reverse logistics is an important means of turning waste into resources, solving environmental problems and promoting high-quality development, as well as an important reliance on enriching and improving the value chain system and further exploiting the value of products. However, the implementation of reverse logistics is a long-term, complex and dynamic process. Many factors need to be considered by the stakeholders when making decisions. With the help of the evolutionary game approach, this paper establishes a model based on the relationship between the government, enterprises (manufacturing enterprises) and logistics companies, and conducts simulation analysis to provide references for how the three parties implement or participate in reverse logistics. The results of the study show that: 1) The total amount of waste affects the evolutionary direction of enterprises’ decision choices, while the conversion rate of waste has a significant impact on the evolutionary trajectory (speed) of enterprises’ decision choices. 2) When the government’s regulation and punishment of the implementation of reverse logistics is greater, enterprises can choose to implement outsourcing reverse logistics to transfer the risk. 3) Logistics companies give certain discounts to attract enterprises to cooperate with them, but excessive discounts have different degrees of negative impact on the normal operation of both enterprises and logistics companies.
Introduction
Since the reform and opening up, the world has witnessed the rapid rise of economy in China, but the intensification of a series of pollution and resource wastage caused by the accelerated development (Zhang M. et al., 2018; Lu et al., 2020; Wang and Chen, 2022) has made the deterioration of the ecological environment one of the main costs of China’s high GDP growth. Environmental issues are becoming increasingly prominent, and the deepening conflict between economic development and environmental protection has drawn widespread attention from all walks of life, including the government. The 18th National Congress of the Communist Party of China incorporated “ecological civilization” into the overall layout of the five-in-one, highlighting the importance attached to environmental issues. However, strongly advocating environmental protection does not mean giving up economic development. From the perspective of integrating ecology and industry, the circular economy model, which mainly addresses waste reuse, provides a win-win development path for both ecology and economy (Lu and Hu, 2015; Wang G. et al., 2021). “The Proposal of the Central Committee of the Communist Party of China on Formulating the Fourteenth Five-Year Plan for National Economic and Social Development and the Visionary Goals for 2035,” published in November 2020, clearly states the need to comprehensively improve the efficiency of resource utilization, promote waste separation and reduction, and resource utilization, while accelerating the construction of a recycling system for used and waste materials (China, 2020). Recently, the Ministry of Ecology and Environment of the People’s Republic of China issued the “Implementation Plan for Reducing Pollution and Reducing Carbon Synergy,” which also proposed “promoting the recycling of construction waste” and “promoting the recycling of retired power batteries, photovoltaic modules, wind turbine blades and other new waste” (Environment et al., 2022). It further shows that the relationship between economic development and environmental protection is not irreconcilable and that by reproducing and reusing waste, we can effectively improve the efficiency of resource use and balance economic and environmental benefits (Ghisellini et al., 2016; Wang S. et al., 2022; Hansen et al., 2018).
Specifically, the problems caused by waste are acute: on the one hand, the direct or indirect pollution of land, water, and climate by waste can damage the human living environment (Laner et al., 2009; Islam, 2018; Guo et al., 2021), and waste also poses a potential threat to human health, such as odor pollution caused by volatile trace compounds released from municipal solid waste may negatively affect human health (Zhao et al., 2015). On the other hand, waste occupies land and is noteworthy (Li et al., 2020). Therefore, the recycling of waste has a strong practical significance. However, the current situation of waste recycling in China is not optimistic. Taking general industrial solid waste as an example, data from the China Environmental Statistics Yearbook (Figure 1) shows that from 2011 to 2019, the generation of general industrial solid waste showed an increasing trend, while on the other hand, its combined utilization rate showed a decreasing trend. To a certain extent, this shows that China’s recycling and processing capacity is slightly inadequate in spite of the large increase in the amount of waste, and also reflects the huge value of the waste hidden in the side. Therefore, reverse logistics as a necessary means to achieve waste recycling (Mishra and Singh, 2020a; Suzanne et al., 2020) should receive high attention to achieve efficient use of resources (Kirchherr et al., 2017).
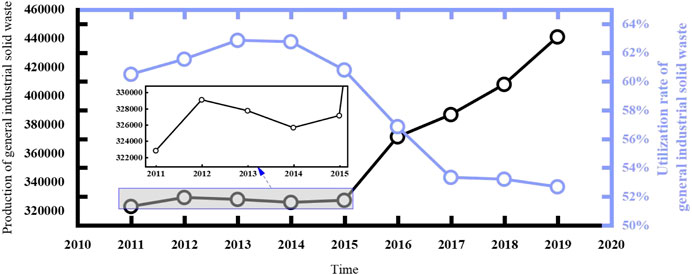
FIGURE 1. 2011–2019 China’s general industrial solid waste production and comprehensive utilization rate.
Reverse logistics, a concept first introduced by Stock (1992), is considered to be the core of the closed-loop supply chain, and it is clear that this concept is relative to forward logistics and can be combined with it to form a closed loop (Figure 2) (Kazemi et al., 2018). However, reverse logistics is never simply the reversal of forward logistics. It is more concerned with the reuse of products, which can be divided into two main types: 1) recycling and disposal of waste; 2) the return and repair of products (Govindan et al., 2015). In particular, the implementation of the first type of reverse logistics allows enterprises (in this case, manufacturing enterprises, hereinafter) to effectively ensure environmental benefits while balancing economic benefits (Cricelli et al., 2021). However, the characteristics of reverse logistics itself, such as the uncertainty of demand, time, and quality (Lieckens and Vandaele, 2011; Qiang et al., 2013; Zarbakhshnia et al., 2020) determines that its implementation must be a long-term, complex, and dynamic process.
In this regard, some scholars have proposed that reverse logistics can be analyzed by value chain (Dang and Duan, 2011). Value chain theory was first proposed by Porter (1985) and focuses on synergy and sharing effects, while being closely linked to the supply chain. However, value chain emphasizes the creation of value and benefits more than supply chain (Chi and Xuan, 2000; Wang and Song, 2017). Guo (2008) argues that reverse logistics value chain is the integration of reverse logistics value activities of enterprises with the goal of gaining competitive advantage, taking into account environmental and economic benefits. Based on this, the decisions related to the implementation of reverse logistics from the perspective of the value chain can be focused on two issues: the size of the value created by the waste and how to coordinate the many links in the chain in the reverse logistics process. That is, in the process of making decisions related to the implementation of reverse logistics, enterprises should not only consider their own operations, the value and nature of waste, but also pay attention to the behavioral decisions of other parties. In terms of the participants in reverse logistics, government, enterprises, logistics companies, and consumers (Agrawal et al., 2015; Liu C. et al., 2021; Sharif et al., 2012; Wu, 2021; Zhou et al., 2021) all play different roles in the process, and each party’s decisions, either large or small, have the effect of driving or hindering the development of reverse logistics to varying degrees. In particular, problems such as the crisis of trust and conflict of interest can also arise in cooperation between the government, enterprises and logistics companies (Giannakas and Fulton, 2000; Govindan et al., 2019; Govindan et al., 2015; Zhao and Bai, 2021). For example, in the real situation, the intention of government subsidies is to promote enterprises to implement reverse logistics, while the behavior of enterprises cheating subsidies really exists, and the specialized services of logistics companies can help enterprises avoid some problems, but even the waste has the risk of leakage (the operation of enterprises, product technology, etc.). Another example is that government regulation will have an impact on the choice of both the reverse logistics model and partners of enterprises.
It is extremely complicated for the government, enterprises and logistics companies to consider multiple factors in the process of making decisions related to whether to implement or participate in reverse logistics. Evolutionary game theory is based on the assumption of finite rationality and learning mechanisms to study the decision making of actors (Fan et al., 2021), and is known for solving multiple equilibrium problems in the decision making process compared to traditional game theory (Ji et al., 2019), and is widely used in many fields (Gu et al., 2019; Hosseini-Motlagh et al., 2020; Zhao and Liu, 2019; Xing et al., 2020; Wang S. et al., 2021; Zhao and Bai, 2021; Johari and Hosseini-Motlagh, 2022). Therefore, this paper selects the evolutionary game approach, based on the value chain perspective, to study the behavioral decision problem of implementing or participating in reverse logistics among the government, enterprises and logistics companies. To provide a reference for how to choose the implementation of reverse logistics as an effective way to convert waste into resources, so as to promote the sustainable development of reverse logistics and lay a solid foundation for the effective utilization of waste.
The main contributions of the article are as follows: 1) The economic and environmental benefits created by reverse logistics are explored from the perspective of value chain, and the government’s regulatory behavioral measures are introduced to analyze the influence relationship among the behavioral decisions of the government, enterprises and logistics companies. 2) From the perspective of the main parties, we systematically consider and summarize the driving and hindering factors that affect the parties’ choices to implement or participate in reverse logistics, and establish a tougher three-party evolutionary game model of reverse logistics. 3) It broadens the idea of applying and researching the three-party evolutionary game method in the field of reverse logistics.
Literature review
For each party, in the process of deciding whether to implement or participate in reverse logistics, the benefits are the main driving force, while the costs and risks are often a hindrance (Baptista et al., 2019; De Oliveira et al., 2021). Currently, scholars have conducted in-depth studies from different dimensions, such as economic, environmental, and social (Ramos et al., 2014; Zhang Y. et al., 2018), exploring the revenues and expenses of each party in the implementation of reverse logistics under different strategies or models (Álvarez-Gil et al., 2007; Genc and De Giovanni, 2018; Dhanorkar, 2019; Dominguez et al., 2020) and optimize them in order to achieve the maximum total benefits. Overall, it seems that the existing studies have focused on both one-way and two-sided factors that influence the choice of parties to participate in or implement reverse logistics.
One-way factors influencing stakeholders’ choices
The influencing factors sorted out in this section are divided into two main categories, one is simply the factors that promote the parties to choose to implement or participate in reverse logistics, and the other is simply the factors that hinder the parties to choose to implement or participate in reverse logistics. Economic benefits are one of the most powerful factors driving enterprises to choose to implement reverse logistics (Govindan et al., 2019), and most of such benefits are the value of the remanufactured waste and the hidden cost savings from a rational and efficient reverse logistics system (Datta, 2020; Gong and Zhang, 2022). Social benefits follow environmental benefits, and concern for and protection of the environment reflects the enterprise’s commitment to social responsibility (Gelderman et al., 2021; Usman et al., 2022), which is also the responsibility of the government (Ma and Zhang, 2020), which helps to enhance the image of both in the public mind. The costs paid can sometimes be a driving factor, such as the cost of incinerating or disposing of waste directly in landfills (Dijkgraaf and Vollebergh, 2004); the cost of maintaining facilities and equipment (Frisch et al., 2021) is one of the factors that motivate logistics companies to operate reverse logistics, and the high cost of environmental management can attract the government participation in reverse logistics while prompting enterprises to choose reverse logistics (Wang et al., 2018; Chu et al., 2019; Liu W. et al., 2021).
Reddy et al. (2020) considered the impact of carbon tax in the design of the reverse logistics network, indicating that the implementation of reverse logistics also comes at a certain environmental cost; similarly, Liu et al. (2014) pointed out that the process of remanufacturing also generates different levels of pollution, both providing new ideas for improving the overall environmental benefits; in terms of economic investment, the original construction of reverse logistics system requires a large amount of financial support (Abdulrahman et al., 2014; Zhang et al., 2020), and also requires certain cost investment in the subsequent operation process, such as material consumption in the remanufacturing process (Lv et al., 2021; Ullah et al., 2021; Andersen et al., 2022) and the cost of packaging the waste (Gámez Albán et al., 2015; Meherishi et al., 2021).
Two-sided factors influencing stakeholders’ choices
Some special factors have two sides, including three situations: (i) the factor drives the choice of some parties to implement or participate in reverse logistics but has a negative impact on the decisions of other parties. (ii) The factor produces opposite effects on the same participant at different stages. (iii) The role of this factor as an enabler or a hindrance depends on the overall quality of the reverse logistics system. We call the above situations “two-sided factors affecting the implementation or participation of the parties in reverse logistics”, and this section will provide a comprehensive overview of such factors:
(i) The volume discount given by the logistics company to the enterprise (Yu and Xiao, 2021) can be considered as the cost expenditure of the logistics company and part of the cost savings of the enterprise, and according to the doctrine of the “third source of profit” (Xize, 1970), it can even be interpreted as the revenue gained by enterprises. Government subsidies to enterprises are one of the important incentives for enterprises to implement reverse logistics (Feng et al., 2021; Heydari et al., 2017), and because enterprises may have a series of irregularities or even illegal behaviors such as subsidy fraud (Giannakas and Fulton, 2000), governmental regulation of firms becomes so necessary (Salmon et al., 2021; Wang Z. et al., 2022). Obviously, government incentives and penalties play a role in promoting and hindering the strategic choices of enterprises in reverse logistics, respectively, while the influence of incentives and penalties on the government’s own strategic choices is the opposite of that of enterprises.
(ii) An enterprise’s decision to implement reverse logistics is irreversible (Govindan et al., 2019). Once the system is in place, the cost of maintaining the facilities and equipment (Frisch et al., 2021) will drive enterprises to continue to develop reverse logistics. In contrast, combined with the long construction period of the reverse logistics system and the large original investment, the maintenance cost of the facilities and equipment before the construction of the system will prevent enterprises from choosing to establish a system to implement reverse logistics.
(iii) Anticipating that profitable reverse logistics projects may end up losing money due to issues such as risk shocks or improper operations (Lundin, 2012), a high-quality reverse logistics system should have more than just an efficient logistics network (Alshamsi and Diabat, 2018; Banguera et al., 2018; Van Engeland et al., 2020; Parchami and Behnamian, 2021), high-end facilities and equipment, and quality implementation solutions (Mishra and Singh, 2020a; Chan et al., 2020), reliable partners are also essential (Chen et al., 2021; Jauhar et al., 2021). Since mutual trust between parties is the cornerstone of cooperation (Paula et al., 2019), enterprises also need to consider the reputation of the logistics company and whether the waste contains trade secrets in the process of choosing a reverse logistics model.
Based on the above, it can be found that most of the existing studies focus on the impact of government subsidies and regulation on enterprises and their choices of logistics companies. Few studies have considered the interplay between the government’s regulatory behavior decisions and the cooperation strategies of enterprises and logistics companies under the constraints, and few studies have focused on issues such as the specific effects of multiple factors on the tripartite decisions of the government, enterprises, and logistics companies.
Basic assumptions and modeling
Basic assumptions
The game participants involved in the implementation of reverse logistics, i.e., the government, enterprises and logistics companies, are finite rational decision-makers. The probability of choosing a behavioral strategy for government participation in the evolutionary game of reverse logistics is x, where x ∈ (0,1). The probability of choosing a behavioral strategy for the enterprise to implement (mainly refers to the enterprise’s choice of self-operated or mixed mode) the evolutionary game of reverse logistics y, where y ∈ (0,1). The probability of choosing a behavioral strategy for a logistics company participation in the evolutionary game of reverse logistics is z, where z ∈ (0,1). Based on the relevant contents of the literature review as a theoretical basis, the following setting parameters are given in combination with the discussion in the introduction (Table 1):
Construction of payoff matrices for game participants
The payoff matrices are constructed based on the assumptions shown in the following tables (Tables 2–5):
Expected benefit functions of the game participants
(1) If the expected benefit of participating in reverse logistics implementation for the government is
Expected benefit of participating in reverse logistics implementation for government:
Expected benefit of not participating in reverse logistics implementation for government:
Average expected benefit for government:
(2) If the expected benefit of implementing reverse logistics for enterprises is
Expected benefits of implementing reverse logistics for enterprises:
Expected benefits of not implementing reverse logistics for enterprises:
Average expected benefits for enterprises:
(3) If the expected benefit of participating in reverse logistics implementation for logistics companies is
Expected benefits of participating in reverse logistics implementation for logistics companies:
Expected benefits of not participating in reverse logistics implementation for logistics companies:
Average expected benefits for logistics companies:
Asymptotic stability analysis of game participants
Since the game parties involved in the implementation of the reverse logistics decision are finite rational, and there are differences between individual behaviors, some of the game parties must continue to improve their strategies through a certain period of learning and accumulation, in order to obtain stable equilibrium results. Based on the above expected benefit function, the replicated dynamic equations of the participating game parties (government, enterprises, logistics companies) can be obtained.
Replicated dynamic equations and solutions of equilibrium points
The replicated dynamic equations for government, business, and logistics companies are as follows:
By associating the above 3 replicated dynamic equations, i.e.,
The solution gives:
The replicated dynamic equations for enterprises, logistics companies, and the government are derived as Eqs 16–18:
According to the theory related to evolutionary games, it can be obtained that the equilibrium points
Stability analysis of game participants
From Eq. 16 to Eq. 18, when
(1) Asymptotic stability analysis of the government
According to Eq. 16:
If
at this point, then there is
then there is
(2) Asymptotic stability analysis of enterprises
Similarly, According to Eq. 17:
at this point, then there is
then there is
(3) Asymptotic stability analysis of logistics companies
Similarly, According to Eq. 18:
at this point, then there is
then there is
Simulation analysis of the game participants
Through the above analysis, it can be seen that in the process of the evolutionary game, the strategic choices of government, enterprises and logistics companies influence each other and are closely linked. This paper uses MATLAB2020a software for simulation and analysis to make the evolution process visualized. Referring to existing related results (Gu et al., 2019; Ma and Zhang, 2020; Fan et al., 2021; Zhao and Bai, 2021), let the initial value α = 0.8, Ω = 0.8, β = 0.5, φ = 0.5, γ = 0.5, A = 1500, B = 100, S = 100, D1 = 60, D2 = 80, F = 100, G = 150, H = 50, I = 50, J = 20, L = 300, K = 60, M = 150, P = 150, C1 = 0.05, C2 = 0.2, C31 = 300, C32 = 500, W = 5.
Identification of the impact of total waste and conversion rate
The values of array I (initial values) and array II (let α = 0.5, A = 5,500, and the rest of initial values remain unchanged) are taken separately and simulated to evolve 100 times over time from different strategy combination points. By comparing the simulation results of the evolution of array I (Figure 3) and array II (Figure 4), it can be seen that the combination of evolutionary stabilization strategies of the system changes from (no implementation by enterprises, government participation, and logistics companies participation) to (implementation by enterprises, government participation, and logistics companies participation) with the increase of the value of α*A under the other conditions remain unchanged.
By comparing the simulation results in the above figures, it can be seen that the value of waste (α*A) can change the decision of reverse logistics of enterprises. However, the value of waste consists of two parameters, i.e., the conversion efficiency α and the total amount of waste A. Whether one of these parameters changes the choice of the enterprise, or both parameters change the choice of the enterprise, or only the combination of the two parameters changes the choice of the enterprise is to be further shown by with the initial values unchanged, let A = 7,000, A = 5,500, and A = 5,000 again respectively to obtain Figure 5A; with the rest of the initial values unchanged, let (A = 5,500, α = 0.9), (A = 5,500, α = 0.1), (A = 5,000, α = 0.9), and (A = 5,000, α = 0.1) respectively to obtain Figure 5B. As can be seen from Figure 5, the total amount of waste changes the evolutionary direction of the enterprise’s decisions in the condition that there is government control and cooperation with logistics companies, while the efficiency of waste conversion only has an impact on the evolutionary trajectory (speed).
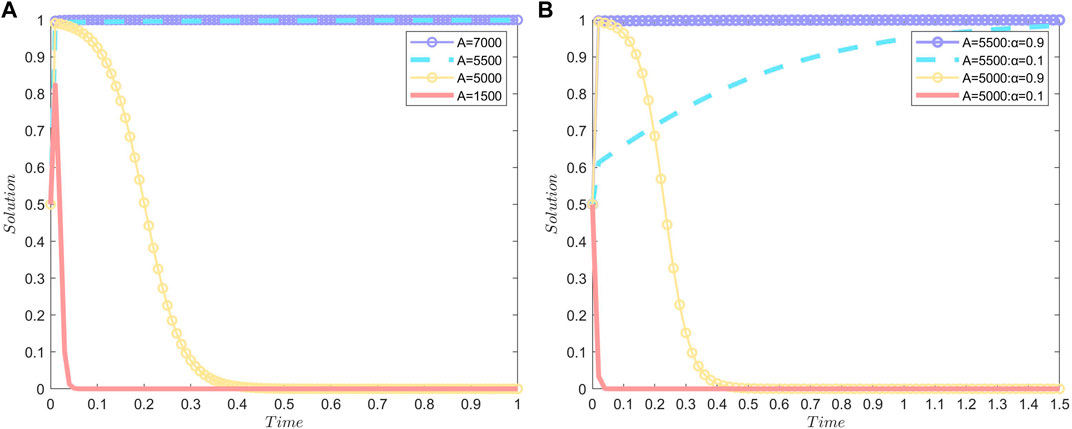
FIGURE 5. Comparison of the (A) impact of the total amount of waste (B) impact of the conversion effificiency of waste.
Analysis of the factors influencing the evolutionary trajectory (speed)
With the initial values unchanged, the impact of waste conversion rate on strategy selections was obtained by then making α = 0.5 and α = 0.2 again, respectively (Figure 6). In the process of evolution, when the efficiency of waste conversion increases, the probability of enterprises choosing to implement reverse logistics strategy increases, while the probability of logistics companies’ participation decreases, and the change in the probability of choice of all three parties is more obvious. Since improving the conversion rate of waste can bring more economic benefits to enterprises while enhancing environmental benefits, enterprises are more inclined to choose to implement reverse logistics. Therefore, in the stage of product design and life cycle assessment, it is important to consider the disposal method and conversion rate after it becomes waste for the implementation of enterprise reverse logistics.
With the initial values unchanged, the impact of the discount strength of the logistics companies on the strategy selections was obtained by then making Ω = 0.5 and Ω = 0.2 again, respectively (Figure 7). In the process of evolution, when the value of the discount from logistics companies to enterprises decreases, i.e., the discount increases, the probability of enterprises choosing to implement reverse logistics increases, the probability of logistics companies choosing to participate decreases, and the probability of the government choosing to participate increases. The position of logistics companies in the tripartite game is relatively weak, and they can increase the cooperation opportunities with enterprises by means of discounts, but the increase of discounts means the reduction of profits of logistics companies, which will increase the risk of losses. At the same time, the increase in discounts will also attract more attention from the government, as unusual discounts may be the result of unfair trade or monopolistic competition. Therefore, logistics companies must grasp the strength of the discount to enterprises, too low discount strength does not play a role in stimulating the enthusiasm of enterprises, while too high discounts are likely to combat their own enthusiasm at the same time to attract stricter government regulation of enterprises, causing unnecessary trouble for enterprises.
With the initial values unchanged, the impact of the strength of government regulation of enterprises on strategy selections was obtained by then making β = 0.8 and β = 0.2 again, respectively (Figure 8). In the process of evolution, when the government regulation of enterprises increases, the probability of enterprises choosing to implement reverse logistics decreases and the probability of logistics companies choosing to participate increases. Increased government regulation of enterprises will combat the enthusiasm of enterprises to implement reverse logistics, but it is also likely to promote cooperation between enterprises and logistics companies. Put another way, when the degree of government regulation becomes greater, enterprises can transfer or even avoid such risks by choosing to outsource reverse logistics.
With the initial values unchanged, the impact of the strength of the enterprise’s supervising the logistics company on the strategy selections was obtained by then making φ = 0.8 and φ = 0.2 again, respectively (Figure 9). In the process of evolution, when the enterprise’s supervision of logistics companies becomes stronger, the probability of logistics companies participating in reverse logistics decreases and the probability of enterprises implementing reverse logistics increases. The main reason for enterprises to supervise logistics companies is to prevent them from leaking secrets and carrying out irregularities. Among them, preventing logistics companies from leaking secrets is the focus of enterprise attention, and the content of leaks is mainly divided into two categories: one is the operation of the enterprise, and the second is the core technology of the enterprise’s products. Therefore, according to the characteristics of the waste itself and enterprises’ own operation, enterprises can choose several logistics companies for outsourcing, which makes the information related to the waste relatively decentralized and can prevent the operation of the enterprise from being leaked by the logistics companies. In addition, technically confidential waste can be classified differently from general waste. Implement self-operated reverse logistics for confidential waste, or significantly improve the supervision of logistics companies to reduce the risk caused by their leakage of secrets.
With the initial values unchanged, the impact of the proportion of enterprises’ self-operated reverse logistics waste on the strategy selections was obtained by then making γ = 0.8 and γ = 0.2 again, respectively (Figure 10). In the process of evolution, when the proportion of enterprises’ self-operated reverse logistics waste increases, the probability of enterprises implementing reverse logistics increases and the probability of logistics companies participating decreases. After the completion of the reverse logistics system, the unit cost of self-operated reverse logistics is smaller than that of outsourced reverse logistics in most cases. Under this premise, if the enterprise can grasp a higher percentage of waste that can carry out self-operated reverse logistics, the probability of enterprises implementing reverse logistics is increased, and the probability of logistics companies participating decreases.
Conclusion and discussion
Conclusion
Reverse logistics further enriches the system of the value chain and is an important way to convert waste into resources, solve environmental problems and explore the potential value of waste, so it is undoubtedly necessary to pay attention to and promote the development of reverse logistics. This paper sorts out the main factors affecting the decisions of the government, enterprises and logistics companies in reverse logistics, and selects the evolutionary game method to establish a tougher mathematical model and conduct simulation analysis, and reaches the following conclusion:
(1) When the government’s regulation of enterprises is low, a series of violations are prone to occur. However, when the regulation is high, it will inhibit the willingness of enterprises to implement reverse logistics on their own, but this also promotes the cooperation between enterprises and logistics companies to a certain extent.
(2) The considerable economic benefits brought by the conversion of waste into resources are the key to promoting the implementation of reverse logistics, along with the increase in the total value of waste, the willingness of enterprises to implement reverse logistics has also increased significantly. On the other hand, the implementation of reverse logistics to obtain environmental benefits is a social responsibility that enterprises need to undertake, and in the long run, it can also reduce the total cost of investment required for future environmental management.
(3) The professionalism of logistics companies can effectively cope with the complexity and risk of reverse logistics and play an indispensable role in assisting enterprises to implement reverse logistics. Especially for some enterprises difficult to grasp the nature of the special waste. The discounts given by logistics companies to enterprises can attract enterprises to choose to cooperate with them, but too high discounts strength is not conducive to the development of cooperation. In general, for logistics companies, their own reputation and professionalism are the bonds that maintain their cooperation with enterprises.
Discussion
Given that the total amount and conversion rate of waste have a greater impact on whether enterprises decide to implement reverse logistics, on the one hand, for enterprises with small waste production, they can choose to jointly operate reverse logistics to share the costs and benefits. On the other hand, most enterprises should evaluate the conversion rate of waste and should consider the final treatment stage at the initial product design stage, and effectively improve the conversion rate by improving the production process to maximize resource recycling.
In addition, trust is the basis of tripartite cooperation, too strict regulation not only means a considerable amount of investment but also will hinder the normal work, so both enterprises and logistics companies need to regulate their own behavior. Of course, reasonable regulation is necessary because it is an important guarantee to maintain a balanced market environment. When enterprises do not trust logistics companies (such as logistics companies have appeared to sell enterprises’ information or other behaviors known to enterprises) but have to choose outsourcing or mixed reverse logistics mode due to their capital, construction and special nature of waste products, they can reduce the risk by cooperating with several logistics companies at the same time, operating confidential waste reverse logistics on their own, and increasing the supervision to logistics companies.
On the other hand, several aspects of this paper need to be further solved in future research: 1) In terms of the relevant parties involved in reverse logistics, only the government, enterprises and logistics companies were selected for the study, without considering the impact of consumers’ choice and value orientation on the willingness of other parties and the development of reverse logistics. 2) Inventory-related issues involved in reverse logistics, such as the impact of remanufactured products on the inventory and sales of the enterprise’s original goods on the enterprises’ reverse logistics decisions are not considered.
Data availability statement
The original contributions presented in the study are included in the article/Supplementary Material, further inquiries can be directed to the corresponding author.
Author contributions
YH contributed to conceptualization, methodology, validation and supervised the research and writing-review and editing draft. FZ contributed to write and revise the manuscript.
Funding
This research was supported by the National Natural Science Foundation of China (Grant No. 42001235) and the Basic Research Projects of Zhejiang Universities (Grant No. 2021RD001).
Acknowledgments
We appreciate the valuable comments provided by the reviewers which greatly improved the quality of this paper.
Conflict of interest
The authors declare that the research was conducted in the absence of any commercial or financial relationships that could be construed as a potential conflict of interest.
Publisher’s note
All claims expressed in this article are solely those of the authors and do not necessarily represent those of their affiliated organizations, or those of the publisher, the editors and the reviewers. Any product that may be evaluated in this article, or claim that may be made by its manufacturer, is not guaranteed or endorsed by the publisher.
References
Abdulrahman, M. D., Gunasekaran, A., and Subramanian, N. (2014). Critical barriers in implementing reverse logistics in the Chinese manufacturing sectors. Int. J. Prod. Econ. 147, 460–471. doi:10.1016/j.ijpe.2012.08.003
Agrawal, V. V., Atasu, A., and van Ittersum, K. (2015). Remanufacturing, third-party competition, and consumers' perceived value of new products. Manag. Sci. 61 (1), 60–72. doi:10.1287/mnsc.2014.2099
Alshamsi, A., and Diabat, A. (2018). Large-scale reverse supply chain network design: An accelerated Benders decomposition algorithm. Comput. Ind. Eng. 124, 545–559. doi:10.1016/j.cie.2018.05.057
Álvarez-Gil, M. J., Berrone, P., Husillos, F. J., and Lado, N. (2007). Reverse logistics, stakeholders' influence, organizational slack, and managers' posture. J. Bus. Res. 60 (5), 463–473. doi:10.1016/j.jbusres.2006.12.004
Andersen, A.-L., Brunoe, T. D., Bockholt, M. T., Napoleone, A., Hemdrup Kristensen, J., Colli, M., et al. (2022). Changeable closed-loop manufacturing systems: Challenges in product take-back and evaluation of reconfigurable solutions. Int. J. Prod. Res., 1–20. doi:10.1080/00207543.2021.2017504
Banguera, L. A., Sepúlveda, J. M., Ternero, R., Vargas, M., and Vásquez, Ó. C. (2018). Reverse logistics network design under extended producer responsibility: The case of out-of-use tires in the Gran Santiago city of Chile. Int. J. Prod. Econ. 205, 193–200. doi:10.1016/j.ijpe.2018.09.006
Baptista, S., Barbosa-Póvoa, A. P., Escudero, L. F., Gomes, M. I., and Pizarro, C. (2019). On risk management of a two-stage stochastic mixed 0–1 model for the closed-loop supply chain design problem. Eur. J. Operational Res. 274 (1), 91–107. doi:10.1016/j.ejor.2018.09.041
Chan, C. K., Man, N., Fang, F., and Campbell, J. F. (2020). Supply chain coordination with reverse logistics: A vendor/recycler-buyer synchronized cycles model. Omega 95, 102090. doi:10.1016/j.omega.2019.07.006
Chen, Z.-S., Zhang, X., Govindan, K., Wang, X.-J., and Chin, K.-S. (2021). Third-party reverse logistics provider selection: A computational semantic analysis-based multi-perspective multi-attribute decision-making approach. Expert Syst. Appl. 166, 114051. doi:10.1016/j.eswa.2020.114051
Chi, X., and Xuan, G. (2000). Correct understanding the relation between supply chain and value chain. Industrial Eng. Manag. (04), 29–32. doi:10.19495/j.cnki.1007-5429.2000.04.007
China, T. t. C. C. o. t. C. P. o. (2020). Proposal of the central committee of the communist party of China on formulating the Fourteenth five-year plan for national economic and social development and the visionary goals for 2035. Available at: http://www.gov.cn/zhengce/2020-11/03/content_5556991.htm.
Chu, Z., Wang, W., Zhou, A., and Huang, W. C. (2019). Charging for municipal solid waste disposal in Beijing. Waste Manag. 94, 85–94. doi:10.1016/j.wasman.2019.05.051
Cricelli, L., Greco, M., and Grimaldi, M. (2021). An investigation on the effect of inter-organizational collaboration on reverse logistics. Int. J. Prod. Econ. 240, 108216. doi:10.1016/j.ijpe.2021.108216
Dang, G., and Duan, X. (2011). A VC anlaysis based study on enterprise reverse logistics cost control. Logist. Technol. 30 (19), 51–54. doi:10.3969/j.issn.1005-152X.2011.10.015
Datta, P. P. (2020). Hidden costs in different stages of advanced services – a multi-actor perspective of performance based contracts. J. Bus. Res. 121, 667–685. doi:10.1016/j.jbusres.2020.03.036
De Oliveira, U. R., Aparecida Neto, L., Abreu, P. A. F., and Fernandes, V. A. (2021). Risk management applied to the reverse logistics of solid waste. J. Clean. Prod. 296, 126517. doi:10.1016/j.jclepro.2021.126517
Dhanorkar, S. (2019). Environmental benefits of internet-enabled C2C closed-loop supply chains: A quasi-experimental study of craigslist. Manag. Sci. 65 (2), 660–680. doi:10.1287/mnsc.2017.2963
Dijkgraaf, E., and Vollebergh, H. R. J. (2004). Burn or bury? A social cost comparison of final waste disposal methods. Ecol. Econ. 50 (3-4), 233–247. doi:10.1016/j.ecolecon.2004.03.029
Dominguez, R., Cannella, S., Ponte, B., and Framinan, J. M. (2020). On the dynamics of closed-loop supply chains under remanufacturing lead time variability. Omega 97, 102106. doi:10.1016/j.omega.2019.102106
Environment, M. o. E. a., Commission, N. D. a. R., Technology, M. o. I. a. I., Development, M. o. H. a. U.-R., Transport, M. o., Development, M. o. A. a. R., et al. (2022). Notice on the issuance of the implementation plan for the synergistic reduction of pollution and carbon. Available at: http://www.mee.gov.cn/xxgk2018/xxgk/xxgk03/202206/t20220617_985879.html.
Fan, W., Wang, S., Gu, X., Zhou, Z., Zhao, Y., Huo, W., et al. (2021). Evolutionary game analysis on industrial pollution control of local government in China. J. Environ. Manag. 298, 113499. doi:10.1016/j.jenvman.2021.113499
Feng, D., Shen, C., and Pei, Z. (2021). Production decisions of a closed-loop supply chain considering remanufacturing and refurbishing under government subsidy. Sustain. Prod. Consum. 27, 2058–2074. doi:10.1016/j.spc.2021.04.034
Frisch, S., Hungerländer, P., Jellen, A., Primas, B., Steininger, S., Weinberger, D., et al. (2021). Solving a real-world locomotive scheduling problem with maintenance constraints. Transp. Res. Part B Methodol. 150, 386–409. doi:10.1016/j.trb.2021.06.017
Gámez Albán, H. M., Soto Cardona, O. C., Mejía Argueta, C., and Sarmiento, A. T. (2015). A cost-efficient method to optimize package size in emerging markets. Eur. J. Operational Res. 241 (3), 917–926. doi:10.1016/j.ejor.2014.09.020
Gelderman, C. J., Schijns, J., Lambrechts, W., and Vijgen, S. (2021). Green marketing as an environmental practice: The impact on green satisfaction and green loyalty in a business‐to‐business context. Bus. Strategy Environ. 30 (4), 2061–2076. doi:10.1002/bse.2732
Genc, T. S., and De Giovanni, P. (2018). Optimal return and rebate mechanism in a closed-loop supply chain game. Eur. J. Operational Res. 269 (2), 661–681. doi:10.1016/j.ejor.2018.01.057
Ghisellini, P., Cialani, C., and Ulgiati, S. (2016). A review on circular economy: The expected transition to a balanced interplay of environmental and economic systems. J. Clean. Prod. 114, 11–32. doi:10.1016/j.jclepro.2015.09.007
Giannakas, K., and Fulton, M. (2000). Efficient redistribution using quotas and subsidies in the presence of misrepresentation and cheating. Am. J. Agric. Econ. 82, 347–359. doi:10.1111/0002-9092.00030
Gong, H., and Zhang, Z.-H. (2022). Benders decomposition for the distributionally robust optimization of pricing and reverse logistics network design in remanufacturing systems. Eur. J. Operational Res. 297 (2), 496–510. doi:10.1016/j.ejor.2021.04.039
Govindan, K., Kadziński, M., Ehling, R., and Miebs, G. (2019). Selection of a sustainable third-party reverse logistics provider based on the robustness analysis of an outranking graph kernel conducted with ELECTRE I and SMAA. Omega 85, 1–15. doi:10.1016/j.omega.2018.05.007
Govindan, K., Soleimani, H., and Kannan, D. (2015). Reverse logistics and closed-loop supply chain: A comprehensive review to explore the future. Eur. J. Operational Res. 240 (3), 603–626. doi:10.1016/j.ejor.2014.07.012
Gu, W., Wei, L., Zhang, W., and Yan, X. (2019). Evolutionary game analysis of cooperation between natural resource- and energy-intensive companies in reverse logistics operations. Int. J. Prod. Econ. 218, 159–169. doi:10.1016/j.ijpe.2019.05.001
Guo, T. (2008). Research on optimization methods of reverse logistics value chain. (Doctor Degree). Harbin: Harbin Institute of Technology. Available from Cnki.
Guo, W., Xi, B., Huang, C., Li, J., Tang, Z., Li, W., et al. (2021). Solid waste management in China: Policy and driving factors in 2004–2019. Resour. Conservation Recycl. 173, 105727. doi:10.1016/j.resconrec.2021.105727
Hansen, M. H., Li, H., and Svarverud, R. (2018). Ecological civilization: Interpreting the Chinese past, projecting the global future. Glob. Environ. Change 53, 195–203. doi:10.1016/j.gloenvcha.2018.09.014
Heydari, J., Govindan, K., and Jafari, A. (2017). Reverse and closed loop supply chain coordination by considering government role. Transp. Res. Part D Transp. Environ. 52, 379–398. doi:10.1016/j.trd.2017.03.008
Hosseini-Motlagh, S.-M., Johari, M., and Zirakpourdehkordi, R. (2020). Grain production management to reduce global warming potential under financial constraints and time value of money using evolutionary game theory. Int. J. Prod. Res. 59 (17), 5108–5129. doi:10.1080/00207543.2020.1773562
Islam, K. M. N. (2018). Municipal solid waste to energy generation: An approach for enhancing climate co-benefits in the urban areas of Bangladesh. Renew. Sustain. Energy Rev. 81, 2472–2486. doi:10.1016/j.rser.2017.06.053
Jauhar, S. K., Amin, S. H., and Zolfagharinia, H. (2021). A proposed method for third-party reverse logistics partner selection and order allocation in the cellphone industry. Comput. Ind. Eng. 162, 107719. doi:10.1016/j.cie.2021.107719
Ji, S.-f., Zhao, D., and Luo, R.-j. (2019). Evolutionary game analysis on local governments and manufacturers' behavioral strategies: Impact of phasing out subsidies for new energy vehicles. Energy 189, 116064. doi:10.1016/j.energy.2019.116064
Johari, M., and Hosseini-Motlagh, S.-M. (2022). Evolutionary behaviors regarding pricing and payment-convenience strategies with uncertain risk. Eur. J. Operational Res. 297 (2), 600–614. doi:10.1016/j.ejor.2021.05.012
Kazemi, N., Modak, N. M., and Govindan, K. (2018). A review of reverse logistics and closed loop supply chain management studies published in IJPR: A bibliometric and content analysis. Int. J. Prod. Res. 57 (15-16), 4937–4960. doi:10.1080/00207543.2018.1471244
Kirchherr, J., Reike, D., and Hekkert, M. (2017). Conceptualizing the circular economy: An analysis of 114 definitions. Resour. Conservation Recycl. 127, 221–232. doi:10.1016/j.resconrec.2017.09.005
Laner, D., Fellner, J., and Brunner, P. H. (2009). Flooding of municipal solid waste landfills--an environmental hazard? Sci. Total Environ. 407 (12), 3674–3680. doi:10.1016/j.scitotenv.2009.03.006
Li, Q., Faramarzi, A., Zhang, S., Wang, Y., Hu, X., and Gholizadeh, M. (2020). Progress in catalytic pyrolysis of municipal solid waste. Energy Convers. Manag. 226, 120246. doi:10.1016/j.enconman.2020.113525
Lieckens, K., and Vandaele, N. (2011). Multi-level reverse logistics network design under uncertainty. Int. J. Prod. Res. 50 (1), 23–40. doi:10.1080/00207543.2011.571442
Liu, C., Dong, H., Cao, Y., Geng, Y., Li, H., Zhang, C., et al. (2021). Environmental damage cost assessment from municipal solid waste treatment based on LIME3 model. Waste Manag. 125, 249–256. doi:10.1016/j.wasman.2021.02.051
Liu, W., Long, S., Wei, S., Xie, D., Wang, J., Liu, X., et al. (2021). Smart logistics ecological cooperation with data sharing and platform empowerment: An examination with evolutionary game model. Int. J. Prod. Res., 1–21. doi:10.1080/00207543.2021.1925173
Liu, W.-w., Zhang, B., Li, Y.-z., He, Y.-m., and Zhang, H.-c. (2014). An environmentally friendly approach for contaminants removal using supercritical CO2 for remanufacturing industry. Appl. Surf. Sci. 292, 142–148. doi:10.1016/j.apsusc.2013.11.102
Lu, F., and Hu, P. (2015). Empirical analysis of relationships between operational influence factors and operational performance of industrial waste recycling network. Bus. Manag. J. 37 (12), 145–153. doi:10.19616/j.cnki.bmj.2015.12.018
Lu, X., Zhang, S., Xing, J., Wang, Y., Chen, W., Ding, D., et al. (2020). Progress of air pollution control in China and its challenges and opportunities in the ecological civilization era. Engineering 6 (12), 1423–1431. doi:10.1016/j.eng.2020.03.014
Lundin, J. F. (2012). Redesigning a closed-loop supply chain exposed to risks. Int. J. Prod. Econ. 140 (2), 596–603. doi:10.1016/j.ijpe.2011.01.010
Lv, Y., Li, C., Zhao, X., Li, L., and Li, J. (2021). A novel approach for remanufacturing process planning considering uncertain and fuzzy information. Front. Mech. Eng. 16 (3), 546–558. doi:10.1007/s11465-021-0639-1
Ma, L., and Zhang, L. (2020). Evolutionary game analysis of construction waste recycling management in China. Resour. Conservation Recycl. 161, 104863. doi:10.1016/j.resconrec.2020.104863
Meherishi, L., Narayana, S. A., and Ranjani, K. S. (2021). Integrated product and packaging decisions with secondary packaging returns and protective packaging management. Eur. J. Operational Res. 292 (3), 930–952. doi:10.1016/j.ejor.2020.11.022
Mishra, S., and Singh, S. P. (2020a). Designing dynamic reverse logistics network for post-sale service. Ann. Oper. Res. 310, 89–118. doi:10.1007/s10479-020-03710-9
Mishra, S., and Singh, S. P. (2020b). A stochastic disaster-resilient and sustainable reverse logistics model in big data environment. Ann. Oper. Res. doi:10.1007/s10479-020-03573-0
Parchami, A., and Behnamian, J. (2021). Lagrangian heuristic algorithm for green multi-product production routing problem with reverse logistics and remanufacturing. J. Manuf. Syst. 58, 33–43. doi:10.1016/j.jmsy.2020.11.013
Paula, I. C. d., Campos, E. A. R. d., Pagani, R. N., Guarnieri, P., and Kaviani, M. A. (2019). Are collaboration and trust sources for innovation in the reverse logistics? Insights from a systematic literature review. Supply Chain Manag. Int. J. 25 (2), 176–222. doi:10.1108/scm-03-2018-0129
Qiang, Q., Ke, K., Anderson, T., and Dong, J. (2013). The closed-loop supply chain network with competition, distribution channel investment, and uncertainties. Omega 41 (2), 186–194. doi:10.1016/j.omega.2011.08.011
Ramos, T. R. P., Gomes, M. I., and Barbosa-Póvoa, A. P. (2014). Planning a sustainable reverse logistics system: Balancing costs with environmental and social concerns. Omega 48, 60–74. doi:10.1016/j.omega.2013.11.006
Reddy, K. N., Kumar, A., Sarkis, J., and Tiwari, M. K. (2020). Effect of carbon tax on reverse logistics network design. Comput. Ind. Eng. 139, 106184. doi:10.1016/j.cie.2019.106184
Salmon, D., Babbitt, C. W., Babbitt, G. A., and Wilmer, C. E. (2021). A framework for modeling fraud in E-waste management. Resour. Conservation Recycl. 171, 105613. doi:10.1016/j.resconrec.2021.105613
Sharif, A. M., Irani, Z., Love, P. E. D., and Kamal, M. M. (2012). Evaluating reverse third-party logistics operations using a semi-fuzzy approach. Int. J. Prod. Res. 50 (9), 2515–2532. doi:10.1080/00207543.2011.581012
Suzanne, E., Absi, N., and Borodin, V. (2020). Towards circular economy in production planning: Challenges and opportunities. Eur. J. Operational Res. 287 (1), 168–190. doi:10.1016/j.ejor.2020.04.043
Ullah, M., Asghar, I., Zahid, M., Omair, M., AlArjani, A., Sarkar, B., et al. (2021). Ramification of remanufacturing in a sustainable three-echelon closed-loop supply chain management for returnable products. J. Clean. Prod. 290, 125609. doi:10.1016/j.jclepro.2020.125609
Usman Khizar, H. M., Iqbal, M. J., Khalid, J., and Adomako, S. (2022). Addressing the conceptualization and measurement challenges of sustainability orientation: A systematic review and research agenda. J. Bus. Res. 142, 718–743. doi:10.1016/j.jbusres.2022.01.029
Van Engeland, J., Beliën, J., De Boeck, L., and De Jaeger, S. (2020). Literature review: Strategic network optimization models in waste reverse supply chains. Omega 91, 102012. doi:10.1016/j.omega.2018.12.001
Wang, G., Chao, Y., and Chen, Z. (2021). Promoting developments of hydrogen powered vehicle and solar PV hydrogen production in China: A study based on evolutionary game theory method. Energy 237, 121649. doi:10.1016/j.energy.2021.121649
Wang, S., Lei, L., and Xing, L. (2021). Urban circular economy performance evaluation: A novel fully fuzzy data envelopment analysis with large datasets. J. Clean. Prod. 324, 129214. doi:10.1016/j.jclepro.2021.129214
Wang, S., and Chen, H. (2022). Could Chinese enterprises real benefit from embedding in global value chains? Environ. Dev. Sustain. doi:10.1007/s10668-022-02348-w
Wang, S., and Song, M. (2017). Influences of reverse outsourcing on green technological progress from the perspective of a global supply chain. Sci. Total Environ. 595, 201–208. doi:10.1016/j.scitotenv.2017.03.243
Wang, S., Wang, X., and Lu, B. (2022). Is resource abundance a curse for green economic growth? Evidence from developing countries. Resour. Policy 75, 102533. doi:10.1016/j.resourpol.2021.102533
Wang, Z., Duan, Y., and Huo, J. (2022). The impact of government intervention measures on recycling of waste electrical and electronic equipment in China considering consumer decision. Energy Policy 160, 112697. doi:10.1016/j.enpol.2021.112697
Wang, S., Yu, H., and Song, M. (2018). Assessing the efficiency of environmental regulations of large-scale enterprises based on extended fuzzy data envelopment analysis. Industrial Manag. Data Syst. 118 (2), 463–479. doi:10.1108/imds-08-2016-0327
Wu, C.-H. (2021). A dynamic perspective of government intervention in a competitive closed-loop supply chain. Eur. J. Operational Res. 294 (1), 122–137. doi:10.1016/j.ejor.2021.01.014
Xing, X.-H., Hu, Z.-H., and Luo, W.-P. (2020). Using evolutionary game theory to study governments and logistics companies’ strategies for avoiding broken cold chains. Ann. Oper. Res. doi:10.1007/s10479-020-03599-4
Xize, X. (1970). Circulation costs--the unseen third source of profit. Japan: Guangcun Publishing House.
Yu, Y., and Xiao, T. (2021). Analysis of cold-chain service outsourcing modes in a fresh agri-product supply chain. Transp. Res. Part E Logist. Transp. Rev. 148, 102264. doi:10.1016/j.tre.2021.102264
Zarbakhshnia, N., Kannan, D., Kiani Mavi, R., and Soleimani, H. (2020). A novel sustainable multi-objective optimization model for forward and reverse logistics system under demand uncertainty. Ann. Oper. Res. 295 (2), 843–880. doi:10.1007/s10479-020-03744-z
Zhang, M., Liu, Y., Wu, J., and Wang, T. (2018). Index system of urban resource and environment carrying capacity based on ecological civilization. Environ. Impact Assess. Rev. 68, 90–97. doi:10.1016/j.eiar.2017.11.002
Zhang, Y., Alshraideh, H., and Diabat, A. (2018). A stochastic reverse logistics production routing model with environmental considerations. Ann. Oper. Res. 271 (2), 1023–1044. doi:10.1007/s10479-018-3045-2
Zhang, Z.-c., Xu, H.-y., and Chen, K.-b. (2020). Operational decisions and financing strategies in a capital-constrained closed-loop supply chain. Int. J. Prod. Res. 59 (15), 4690–4710. doi:10.1080/00207543.2020.1770356
Zhao, T., and Liu, Z. (2019). A novel analysis of carbon capture and storage (CCS) technology adoption: An evolutionary game model between stakeholders. Energy 189, 116352. doi:10.1016/j.energy.2019.116352
Zhao, X., and Bai, X. (2021). How to motivate the producers' green innovation in WEEE recycling in China? - an analysis based on evolutionary game theory. Waste Manag. 122, 26–35. doi:10.1016/j.wasman.2020.12.027
Zhao, Y., Lu, W., and Wang, H. (2015). Volatile trace compounds released from municipal solid waste at the transfer stage: Evaluation of environmental impacts and odour pollution. J. Hazard. Mater. 300, 695–701. doi:10.1016/j.jhazmat.2015.07.081
Keywords: value chain, reverse logistics, environmental issues, evolutionary game, government regulation, third party logistics
Citation: Zhang F and He Y (2022) Study on the effective way to convert waste into resources—game analysis of reverse logistics implementation based on value chain. Front. Environ. Sci. 10:984837. doi: 10.3389/fenvs.2022.984837
Received: 02 July 2022; Accepted: 07 July 2022;
Published: 19 September 2022.
Edited by:
Shuhong Wang, Shandong University of Finance and Economics, ChinaReviewed by:
Chuanming Yang, Suzhou University of Science and Technology, ChinaXidong Yu, Nankai University, China
Copyright © 2022 Zhang and He. This is an open-access article distributed under the terms of the Creative Commons Attribution License (CC BY). The use, distribution or reproduction in other forums is permitted, provided the original author(s) and the copyright owner(s) are credited and that the original publication in this journal is cited, in accordance with accepted academic practice. No use, distribution or reproduction is permitted which does not comply with these terms.
*Correspondence: Yixiong He, aGV5aXhpb25nbWFpbEAxNjMuY29t