- 1School of Mathematics and Statistics, Guangxi Normal University, Guilin, China
- 2School of Business, University Sains Malaysia, Penang, Malaysia
- 3Center for Applied Mathematics of Guangxi, Guangxi Normal University, Guilin, China
- 4School of Economic Information Engineering, Southwestern University of Finance and Economics, Chengdu, China
Green technology innovation is an important means to help reduce carbon emissions. Most of the current researches focus on the evaluation of green technology innovation and its relationship with economic factors, while ignoring its relationship with financial factors. In fact, financial development is an important driving force for further improving the efficiency of technological innovation, especially in developing countries. China, which has put forward the “double carbon” strategy (achieve peak carbon dioxide emission and carbon neutrality) in recent years, has a lot of interaction between green technology innovation and financial development, which is a good research sample. Based on the provincial panel data of 30 provinces in China from 2003 to 2020, this paper follows the research steps: 1) From the perspective of carbon emissions, we use the non-radial super-efficiency SBM-ML model to measure the total factor productivity of green technology innovation. 2) We analyze the temporal and spatial changes of green technology innovation and financial agglomeration in each province. 3) We establish a spatial simultaneous model of generalized three-stage least squares to study the intrinsic relationship between green technology innovation and financial agglomeration. The results show that: 1) The financial agglomeration level of each province is basically stable due to the fixedness of the financial core area, but the development speed of green technology innovation in the southeast coastal area is significantly higher than that in the inland area. 2) The interaction between green technology innovation and financial agglomeration has a nonlinear mechanism of “low-level inhibition and high-level promotion,” that is, low-level financial agglomeration has an inhibitory effect on green technology innovation, and as the level of financial agglomeration increases, its impact on green technology innovation gradually develops positively, and vice versa. 3) The green technology innovation and financial agglomeration in the surrounding provinces have positive and significant promoting effects on the green technology innovation and financial agglomeration in the province, but the impact of green technology innovation is significantly stronger than that of financial agglomeration. Finally, suggestions are put forward for further reducing carbon emissions, realizing the sustainable economic growth and approaching goals of “carbon peaking and carbon neutrality.”
Introduction
China is currently on the road of rapid economic and social development, and its economic level is rapidly improving year by year. But a series of environmental problems also follow, the most important of which is carbon emissions. Since 2006, the annual carbon emissions of China have surpassed the United States which ranked the first above the world. In 2014, China surpassed Russia to become the world’s second largest carbon emitting economy group, and the carbon dioxide emissions in China were 13.9 billion tons in 2020, accounting for 30% of the global total carbon emissions. However, it is still far from the peak and put huge pressure on China to reduce carbon emissions. Not only is China’s carbon emission problem more prominent, but looking at the global greenhouse gas emissions, according to data released by the Netherlands Environmental Assessment Agency in 2020: Since 2010, the total global greenhouse gas emissions have increased by an average of 1.4% per year. In 2019, a record high was reached, with total emissions excluding land use change reaching 52.4 billion tons of carbon dioxide equivalent, 44 and 59% higher than in 2000 and 1990, respectively, and global per capita greenhouse gas emissions reached a total of 6.8 tons of carbon dioxide. If the 5.5 gigatons of carbon dioxide equivalent emitted by land-use change are included, the global total is as high as 59.1 gigatons. Therefore, how to implement comprehensive energy conservation and emission reduction is a top priority. Even though China is an energy superpower, its structure of energy is single and the industrial structure is mainly dominated by “extensive and inefficient” enterprises, which is basically rely on fossil energy such as coal. However, the main cause of increasing carbon emissions annually and the growing air pollution is the direct combustion of fossil energy (Zheng et al., 2016). Faced with the current situation of global warming and high green-house gas emissions, General Secretary Xi Jinping has delivered important speeches many times in influential international conferences such as the Biodiversity Summit, and participated in planning and proposing the “30•60” strategic goals, that is, “China strives to reach a peak in carbon emissions by 2030 and achieve net zero carbon emissions by 2060.” However, the key to realize the vision of carbon neutrality as soon as possible and accelerate the green transformation of China’s industrial structure is to continue to innovate green low-carbon, zero-carbon and even negative-carbon technologies (Shi and Lai, 2013).
The focus of existing literature on green technology research mainly concentrates on the measurement of green technology innovation indicators and its direct or indirect impact on regional carbon emissions and economic development. Many scholars use patent indicators such as the number of green technology innovation patents granted or scientific and technological input indicators such as R&D expenditure to measure a region’s green technology innovation capability (Faber and Frenken, 2009; Hall and Helmers, 2013), but Chen et al. (2021) pointed out that the difference between green technology innovation for traditional technological innovation, the goal should be to achieve sustainable economic development, while green technology innovation is constrained by multiple systems such as education level, economic level, and even natural environment. But in China, where the environment is increasingly severe, environmental impact is a factor that needs to be paid attention to. That’s why a reasonable evaluation system should be constructed to describe the development of green technology. So far, the total factor productivity of green technology innovation calculated by the multi-index evaluation system has been gradually used by scholars. On the basis of the continuous updating and optimization of the calculation methods of green technology innovation indicators, many scholars have further considered its impact on regional development. Ye et al. (2021) et al. studied the green technology innovation of the Yangtze River Economic Belt in China. Through empirical analysis, it was shown that the impact of green technology innovation on the green development of the Yangtze River Economic Belt is heterogeneous. In particular, it has played a very important role in promoting the green development of the lower reaches of the Yangtze River. Meirun et al. (2021) started from both environmental and economic aspects, and pointed out the important practical significance and economic value of green technology innovation. Du et al. (2019) and Liu et al. (2016) proceeded from the “dual carbon” strategy and empirically analyzed the relationship between green technological innovation and carbon emission efficiency. Various studies have shown that green technology innovation is of great significance to reducing carbon emission intensity and promoting green and sustainable economic development.
Based on the existing research on green technology innovation, Xie et al. (2021) found that although financial agglomeration in Chinese cities will promote green total factor productivity, it has a negative impact on the green total factor productivity of surrounding cities based on the empirical space Durbin model. Has inhibitory effect. Before that, Muhsin (2011) had discovered a complex spatial relationship between financial agglomeration and technological progress, and Xie et al. (2021) were graduate students who extended their research to sustainable development. Further, Liu D. et al. (2021) analyzed the rural data of 31 provinces in China, and pointed out that China’s rural financial development and agricultural industry agglomeration have a significant role in promoting agricultural technology innovation and have spatial correlations. Taking the Guangdong-Hong Kong Greater Bay Area as an example, Guo and Wang (2018) pointed out that financial agglomeration also has significant spatial autocorrelation on technological innovation, but the overall impact of financial agglomeration on technological innovation is not significant. From the above literature analysis, it can be seen that the results of various studies on the relationship between financial agglomeration and green technology progress are inconsistent and incomplete. All studies do not consider financial agglomeration and green technology innovation as endogenous variables to each other, consider the interaction between the two, let alone study the spatial relationship of the interaction (Hirsch-Kreinsen, 2011), but these kinds of dynamic influence and development change is exactly what is necessary to further deepen the research. If this demand can be realized and researched in depth, it will contribute to the sustainable development of many regions.
The main purpose of this paper is to deeply study the interaction and spatial influence between China’s provincial financial agglomeration and green technology innovation as endogenous variables. In summary, the contributions of this study are of three points: 1) From the perspective of carbon emissions, we construct an evaluation system of green technology innovation and use the non-radial super-efficiency SBM-ML model to measure the total factor productivity of green technology innovation; 2) We analyze the temporal and spatial changes of green technology innovation and financial agglomeration; 3) We establish a spatial simultaneous model of generalized three-stage least squares estimation to study the intrinsic relationship between green technology innovation and financial agglomeration. By combining green technology innovation and financial agglomeration, we carry out empirical analysis on the spatial attributes of the two and their interaction, study their overall operating mechanism, and analyze the reasons for the differences in development between regions.
Theoretical mechanisms and research hypothesis
Interaction between green technology innovation and financial agglomeration
In the context of China’s continuous increase in carbon emissions, low-carbon technology has gradually attracted attention from all walks of life, and its status in green technology has become more and more important. Meanwhile, green technology and finance are important influencing factors to achieve China’s carbon peak and carbon neutrality goals, and there are many internal mechanisms and influence mechanisms between them. Although Liu et al. (2022) have carried out some research in this field, there are still many problems to be explored. Therefore, Ye and Cheng (2019) empirically analyzed its impact on the efficiency of green technology innovation from the perspective of the financial ecological environment. The results show that the financial ecological environment and its components can effectively promote the efficiency of green technology innovation. Vigorously developing green technology is an inevitable choice to achieve a “win-win” situation of environmental performance and economic performance. Through empirical analysis, Li et al. (2020) clearly pointed out that financial development and environmental policies in Shandong Province have an important impact on green technology innovation. Not only that, financial development will have varying degrees of impact on the development of green technology due to the continuous strictness of regional environmental policies, which indicates that the impact of regional financial development on green technology innovation is not linear, and will produce a periodic difference depending on the changes of region, time or surrounding conditions. Further, Wen et al. (2021) started from the perspective of nonlinear effects and used the panel threshold model and the spatial Durbin model to verify that the financial agglomeration in the Yangtze River Delta region has a nonlinear direct influence on technological innovation and an “inverted U-shaped” spatial spillover effect (Hao et al., 2022). It can be seen that when financial development reaches different stages and is dynamically affected by different factors, the impact on technological innovation will change. At the same time, financial agglomeration is an inevitable stage in the development of regional financial undertakings (Kindleberger, 1973). Therefore, this thesis proposes two hypotheses H1 and H2.
H1: Financial agglomeration has a positive impact on green technology innovation.
H2: The total effect between green technology innovation and financial agglomeration is nonlinear.
Spillover effect mechanism of green technology innovation and financial agglomeration
Spatial spillover effect refers to the influence effect that a certain indicator in a region produces or promotes or inhibits the surrounding areas with geographical and economic significance in the process of development. Some studies have pointed out that financial development has a significant positive spillover effect under the influence of multiple factors (Xie et al., 2020). Wang et al. (2004) analyzed the spatial simultaneous model and concluded that there is a significant spatial effect between financial agglomeration and green development. Green development in surrounding areas can promote local green development, but financial agglomeration in surrounding cities is not conducive to improving local financial agglomeration. In addition, there is an obvious interactive spatial spillover effect between green development and financial agglomeration in each city: financial agglomeration in surrounding areas has a significant positive impact on local green development, and in turn, green development has an inhibitory effect on financial agglomeration. Wang H. et al. (2021) took the province as the research unit and found that the progress of green technology in the surrounding provinces has a negative impact on the economic development of the province. Obviously, the research results of many scholars are not consistent. From the similarity study of Xue et al. (2022) and Zhao et al. (2021), it can be seen that although the two articles are aimed at the carbon emission efficiency of digital finance, Xue et al. (2022) conducted a study on 278 prefecture-level cities in China and pointed out that the development of digital finance has played a significant role in promoting carbon emission efficiency in various regions of China. However, Zhao et al. (2021) took 30 provinces in China as an example, through research and analysis: the impact of China’s digital finance on carbon emissions presents an “inverted U-shaped” state. When the level of digital finance has not reached the threshold, the development of digital finance will significantly promote carbon emissions and aggravate regional pollution. In addition, digital finance has the greatest impact on eastern China, showing spatial heterogeneity of impact. Comparing the differences in the conclusions of the two articles, it can be found that even when faced with the same problem, when there are differences in the research unit and research scope, the results are often different. Based on the above analysis and the research ideas of this thesis, the following hypotheses are proposed:
H3: Financial agglomeration in neighboring provinces has a positive impact on financial agglomeration and green technology innovation in the province, but it may not be significant or at a low level.
H4: The green technology innovation in neighboring provinces has a significant positive impact on the province’s financial agglomeration and green technology innovation.
Methodology and data
Main research methods
SBM-ML model
The SBM model proposed by Tone (2004) is one of the main methods for analyzing static production efficiency. The SBM model can effectively overcome the slack problem of input and output variables, so that the efficiency evaluation problem when there are undesired outputs in the output factors can be obtained. solved. The Malmquist-Luenberger (ML) index based on the SBM model can well reflect the green rate and innovation changes (Wang et al., 2019). Based on the idea of the set average value, the SBM-ML index of the adjacent reference from t to t+1 is constructed, the SBM-ML index of the 2-year adjacent reference is as follows (Li and Chen, 2021; Sun et al., 2021):
This paper takes each province in China as a production decision-making unit. Assuming that there are N kinds of inputs
Among them,
Furthermore, the concept of intertemporal dynamics is introduced, and the idea of geometric mean is used for reference, and the SBM-ML index for 2 years adjacent to t and t+1 is constructed as follows:
The
In order to measure whether geographically adjacent provinces have similar industrial attribute values, analyze the overall spatial association and spatial difference of the research objects from the perspective of spatial correlation, and judge whether there is a spatial relationship and change law, this thesis uses the
Where
Spatial simultaneous model
Considering whether financial agglomeration and regional green innovation have significant spatial spillover effects and regional interaction effects, considering only the impact of financial agglomeration on green innovation and ignoring the reverse impact of the latter on the former may cause simultaneous bias. The endogeneity problem, and ignoring the spatial spillover effect of the two and the interaction effect between regions may cause the problem of missing variables, so choosing a single-equation model may lead to biased or even inconsistent results due to the endogeneity of variables. Therefore, this thesis constructs a spatial simultaneous equation model and draws on the experience of Zhang and Wang (2014) to establish the following equations:
In Eqs 3, 4, Aggit and GTFPit represent the financial agglomeration and the green technology innovation level of i province in tth year respectively,
Indicator measure and notation description
Green technology innovation measurement system
This thesis selects year-end electricity consumption, capital stock, fulltime equivalent of R&D personnel and R&D internal expenditure as input indicators from three aspects of capital investment, energy investment and scientific research investment. As the expected output to measure the total factor productivity of green technology innovation, the total amount of carbon dioxide emissions, industrial solid waste emissions, industrial carbon dioxide emissions and industrial chemical oxygen demand for water are taken as the undesired output. Besides, the capital stock can be calculated by “Perpetual inventory system” and the carbon dioxide emissions are obtained by calculating the relevant energy consumption using the carbon emission coefficient released by IPCC (Liang et al., 2019; Liu Y. et al., 2021). The completed measurement system is shown in Table 1.
Financial agglomeration
Provincial financial agglomeration is the agglomeration of financial industry and financial capital at the provincial level. At present, scholars usually use the Herfindahl index, spatial Gini coefficient, location entropy and other indicators to measure the level of financial agglomeration. However, the Herfindahl index does not take into account the differences in the spatial distribution of financial resources and the size of geographical regions, the spatial Gini coefficient does not take into account the differences in the scale of enterprises in various industries, and the location entropy index also lacks consideration of the differences in the scale of financial institutions. Therefore, based on the existing research and the availability of data, this thesis selects the ratio of the loan scale of provincial financial institutions to the local GDP to represent the level of financial agglomeration.
Among them,
Data source and symbol description
The data in this article are mainly from China Statistical Yearbook, China Urban Statistical Yearbook, China Environment Yearbook, China Energy Statistical Yearbook, National Patent Search System, and 2006–2021 Statistical Yearbooks or Statistical Bulletins of 30 provinces. In order to alleviate the multicollinearity problem, the non-proportional indicators selected in this thesis are all logarithmic in the model. All data information can be found in Table 2.
Empirical analysis
Stability test
Panel data needs to be tested for stationarity before operation and modeling. This thesis mainly chooses LLC test, IPS test and ADF test. Three test methods are used to comprehensively judge whether the data in this thesis is stable and whether the next regression can be carried out.
It can be seen the test result from Table 3 that under the three inspection methods, except for the technical market turnover, all the other indicators have two or more inspection methods that are significant at the 10% level. According to the principle of comparison and selection, all indicators except the technical market turnover are stable. The technical market turnover is all significant at the 1% level under the LLC test. Therefore, the data trend chart is drawn. From Figure 1, it can be seen that the data of the technical market turnover in each province shows a regular upward trend. Therefore, it is considered that the overall trend of technical market turnover is stable, and the conclusions in Table 3 can be confirmed.
Analysis of the status quo of each province
Based on the stationarity analysis above, it is considered that the data selected in this thesis are valid data and do not need differential processing. In order to observe the development of green technology innovation and financial agglomeration level in various provinces in China more intuitively, this thesis uses ArcGIS 10.8 to draw the level of green technology innovation and financial agglomeration in 2020 and the average level of green technology innovation and financial agglomeration 2006 to 2020 in the form of a heat map, as a basis for the overall analysis of green technology innovation and financial agglomeration in various provinces.
Development of China’s provincial green technology innovation level
From the annual average value of total factor productivity of green technology innovation in Figure 2 the southeast coastal areas has always been at a relatively high level. Except for Qinghai Province, the total factor productivity of green technology innovation in the southwest and northwest regions is at a low level, and the north and northeast regions have also remained at medium-low levels. Compared with the total factor productivity heat map of green technology innovation in 2020, it can be seen that Jiangxi, Qinghai, Sichuan, Inner Mongolia and other provinces have improved the level of green technology innovation index and green technology development started earlier. However, the level of green technology innovation is declining year by year. The main reason for this phenomenon is that the development speed of green technology in these provinces is lower than the average progress speed of green technology innovation in China. The development of green technology in Tianjin, Zhejiang, Anhui, Guangdong, Hainan and other provinces has always been at the forefront of the country in terms of starting time and speed of progress, which can radiate the surrounding areas through high-level green technology innovation capabilities, and achieve the goal of common progress in green technology in all provinces in China.
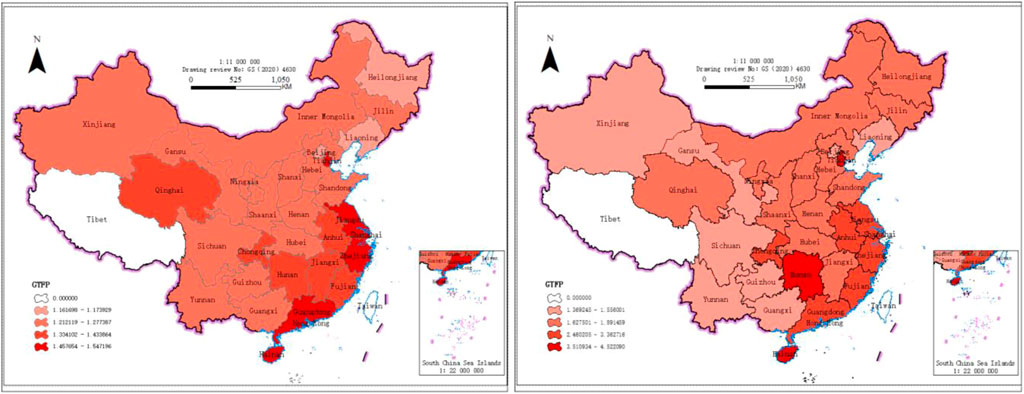
FIGURE 2. The average level of green technology innovation in each province from 2006 to 2020 (left) and the level of green technology innovation in 2020 by province (right).
Development of provincial financial agglomeration in China
It can be seen from Figure 3 that China has formed financial regions centered on Beijing-Tianjin, Shanghai-Zhejiang, Jilin-Liaoning, Guangdong-Guangxi-Guizhou, and Qinghai-Gansu, showing a trend of spreading from the center to the periphery. Comparing these two figures, we can see that the level of financial agglomeration in each province has not changed significantly. Although the level of financial agglomeration in each province has been continuously improving, because few provinces have experienced rapid agglomeration or transfer of financial capital, the level of financial agglomeration in each province has remained close to the level of the average level, and there are no outliers.
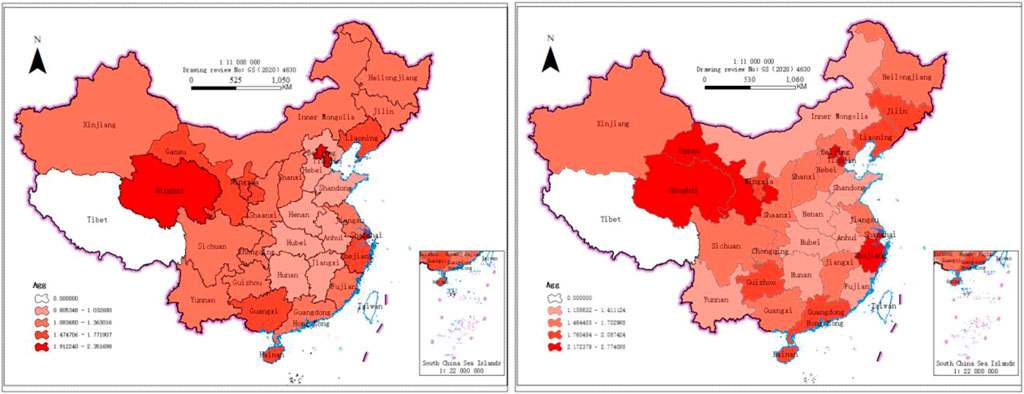
FIGURE 3. The average level of financial agglomeration in each province from 2006 to 2020 (left) and the financial agglomeration level in 2020 by province (right).
Based on the calculation results of the total factor productivity level and financial agglomeration level of green technology innovation in each province, this part draws a provincial heat map, which can more accurately control the development and changes of the overall green technology and financial agglomeration in each province. The interaction and spatial relationship between innovation and financial agglomeration pave the way.
Spatial correlation analysis between green technology innovation and financial agglomeration
In order to further understand the spatial spillover and changes of green technology innovation and financial agglomeration among provinces in China, this thesis takes the inverse distance spatial weight matrix as an example to calculate the global Moran index from 2006 to 2020. The results are shown in Table 4.
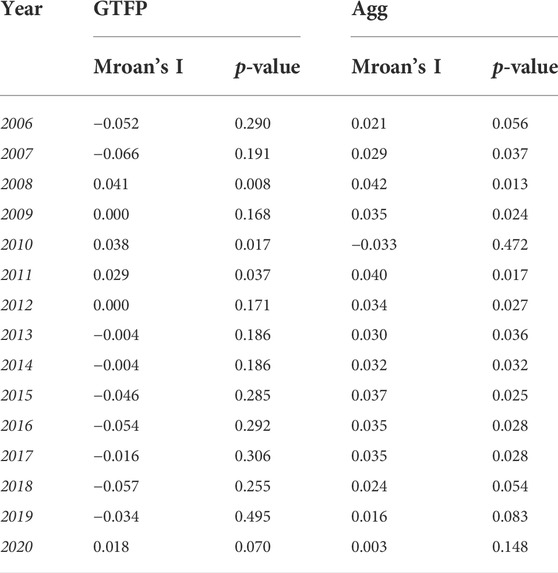
TABLE 4. Moran index of Chinese green technology innovation and financial agglomeration from 2006 to 2020.
From the global Moran index and its p-value over the years, it can be seen that the global Moran index of green technology innovation is only positive and significant in 2008, 2010, 2011 and 2020, and the other years are not significant and the Moran index is negative. Therefore, this thesis It is inferred that the inter-provincial exchanges of China’s overall green technology innovation are infrequent and highly random, and the exchange of green technology and the circulation of green elements in China lacks standardized and normalized policies. In contrast, the Moran Index of the National Financial Agglomeration Global Index failed the significance test only in 2010 and 2020. Through the analysis of the value of the Moran index, it can be seen that since 2017, the overall Moran index in China has shown a downward trend, that is, the impact of financial agglomeration on the surrounding provinces has gradually weakened.
Interaction and spatial relationship between green technology innovation and financial agglomeration
On the basis of spatial correlation analysis, a system of spatial simultaneous equations is constructed. Considering that there may be a two-way endogenous relationship between green technology innovation and financial agglomeration, and the endogenous relationship may be heterogeneous, the square terms GTF2 and Agg2 of green technology innovation and financial agglomeration are added to eliminate the random disturbance of variables and equations. W_Agg and W_GTFP are the spatial lag terms of financial agglomeration and green technology innovation in the spatial simultaneous model, respectively. To eliminate the problem of heteroskedasticity and dimension as much as possible, the spatial correlation of the terms was used to estimate the simultaneous equation system using the generalized spatial three-stage least squares (GS3SLS) method. The results are shown in Table 5.
Model 1 is the estimation result of the financial equation. First, we observe the total factor productivity of green technology innovation and its square term. It can be seen that the two regression coefficients have different signs and are both significant at the 5% level, indicating that when the level of green technology innovation is low, its impact on green technology innovation is low. The influence of financial agglomeration is mainly dominated by inhibition. With the improvement of the level of green technology, it will gradually have a positive impact on the deepening of financial agglomeration. Comparing the coefficient of green technology innovation and its square term under the geographical inverse distance space weight matrix: −9.9046 and 2.0413, it can be seen that the primary item of green technology innovation has a much greater inhibitory effect on financial agglomeration than the secondary item. It is not difficult to draw the conclusion that green technology innovation takes a long time to break through the threshold to promote the development of regional financial under-takings. In the initial stage of development, green technology innovation requires a lot of resource investment, and the initial green technology is often at the expense of its own profits. With the continuous improvement of the level of green technology, high-level green technology promotes the deepening of the process of provincial financial agglomeration by optimizing the industrial structure, restraining the negative effects of undesired output, obtaining policy preferences and so on.
In the financial agglomeration equation, the regression coefficient of the lag term of green technology innovation is positively significant at the 1% level, and the lag term of financial agglomeration is positively significant at the 10% level. In the financial agglomeration equation, the regression coefficient of the lag term of green technology innovation is positive and significant at the 1% level, and the lag term of financial agglomeration is significant at the 10% level, which indicates that green technology innovation activities in adjacent areas have promoted financial agglomeration in the region. The innovation of technology itself has the property of spatial overflow. The circulation of factors between adjacent provinces is relatively free, and the mobility of talents is relatively high. Moreover, the development direction of technology and the characteristics of resources between provinces are quite different, so it is easier to realize the exchange of technology and the flow of resources. Complement each other’s advantages and promote the diversified development of green technology in adjacent areas. The significance level of the spatial lag term of financial agglomeration is relatively low, indicating that although there are exchanges of financial institutions and transfers of financial capital between provinces, the spatial relationship of financial agglomeration is relatively weak due to the large differences in policy priorities and business environments.
Model 2 is the estimation result of the green technology innovation equation. The regression coefficient of the square term of financial agglomeration is analyzed, which is in the opposite direction to the regression coefficient of financial agglomeration and is statistically significant at the 10% level. The mechanism of action is similar. The industrial structure of low-developed areas is generally dominated by “low-efficiency, high-power consumption” industries. The rapid accumulation of capital in such areas is often based on the further development of their own industrial structure. Vigorously developing green technology at this stage does not meet the needs of the region. As the level of financial agglomeration increases, educational resources, technological talents, and financial capital gradually gather, and the industrial structure gradually tends to be diversified, which helps to reduce R&D costs, broaden innovative ideas, and play a positive role in green technology innovation. The above analysis of the interaction between green technology innovation and financial agglomeration can confirm hypotheses H1 and H2.
In the green technology innovation equation, the spatial lag term of green technology innovation is significantly positive at the 1% significance level, indicating that under different spatial correlations, green technology innovation in surrounding provinces has a significant role in promoting the province. Taking the calculation results under the inverse distance space weight matrix of Model 2 as an example, for every 1 percentage point increase in the level of green technology innovation in the surrounding provinces, the total factor productivity of green technology innovation in the province increases by 0.96 percentage points. Comparing the global Moran index of green technology innovation, it is found that the spatial autocorrelations measured by the two are inconsistent. By contrast, it can be known that the data structures used to find the two are different. The global Moran index of the explained variable is based on cross-sectional data, while the lagged terms for core variables are generally based on panel data. Besides, the usage of the two model is different. The global Moran index of the explained variable is the slope formed by the explained variable as the x-axis value and the weight vector multiplied by the explanatory variable as the y-axis value; and the lagged terms for core variables are the spatial autoregressive coefficient after adding the control variable. In other words, the global Moran index is the result of one-to-one spatial autoregression, while the lagged terms for core variables are the result of many-to-one spatial autoregression. Adding control variables not only affects the value of the regression coefficients, but sometimes also the signs of the regression coefficients. Taking the space lag term of green technology innovation in Model 2 as an example, Model 2 regards financial agglomeration as an endogenous variable, and the remaining control variables are set as instrumental variables to comprehensively consider the spatial correlation of green technology innovation. In the context of the rapid development of the Internet, a region can quickly obtain innovative elements and knowledge spillovers from neighboring provinces through the Internet, and compare its own green technology development to complement its advantages. The spatial lag term of financial agglomeration on green technology innovation is significant at the 10% level, which proves that financial agglomeration in spatially related provinces provides advantageous resources for the development of green technology in the province, which is consistent with the previous theoretical analysis. The above analysis of green technology innovation and the spatial lag term of financial agglomeration can prove the accuracy of assumptions H3 and H4. So far, all the hypotheses in this thesis have been confirmed.
Comparing the regression results of the system of spatial simultaneous equations under three different spatial weight matrices, it can be seen that the direction and significance of the regression coefficients of each variable are basically consistent. Therefore, it is considered that the spatial simultaneous model formed by Model 1 and Model 2 is robust, and the estimation results have good reference value and guiding significance for studying the development trend and mechanism of green technology innovation and financial agglomeration.
Conclusions and implications
On the basis of the existing research, this thesis constructs a green technology innovation measurement system based on carbon emissions. On the basis of the system, we study the direct impact and spatial relationship between green technology innovation and financial agglomeration. The research found that: 1) Since 2006, the level of financial agglomeration in China’s provinces has been relatively stable, forming a typical multi-core “spillover” pattern, while the regional changes in the level of green technology innovation have been relatively large. In 2020, the level of green technology innovation in each province will seriously deviate from the average level. Although some central and western provinces started early in green technology, the eastern and southeastern provinces developed rapidly in green technology, much faster than the national average. 2) There is a significant nonlinear interaction relationship between green technology innovation and financial agglomeration, and the effect change mechanism is similar, that is, it is inhibited at a low level and promoted at a high level. 3) In terms of single variable, the overall spatial dependence of green technology innovation is not significant, while financial agglomeration shows obvious spatial dependence between provinces. 4) From a two-way endogenous perspective, the spatial relationship of financial agglomeration among regions is significantly positive, but the impact of financial agglomeration on green technology innovation in other provinces is not significant. Green technology innovation has a significant role in promoting the spatial impact of green technology innovation and financial agglomeration in other provinces.
This thesis has important theoretical guidance and policy implications by studying the current interaction and spatial relationship between China’s provincial green technology innovation and financial agglomeration:
Firstly, strengthen work communication and policy exchanges in neighboring provinces, and improve the flow mechanism of green technology and financial capital, so as not to be “stuck” by policies. From the regression results of simultaneous equations, it can be seen that exchanges and cooperation between different regions can achieve a win-win effect. Therefore, Chinese provinces need to make full use of the spatial effect of financial activities to promote inter-regional economic and technological exchanges. In addition, the inter-provincial green technology development plan and the financial development plan should be consistent in direction, form a unified strategic goal, and be supplemented by a “cooperative” common development policy. Only by maintaining a consistent strategy can we successfully absorb the remaining resources and spillover enterprises from neighboring provinces, complement each other’s advantages, make up for the shortcomings of the province, and achieve all-round and three-dimensional development.
Secondly, increase the support of financial institutions for green technology innovation, and realize a sustainable development model of mutual support, mutual benefit and win-win between financial agglomeration and green technology innovation. The emphasis and investment of financial institutions on green technology innovation is a real market demand and an important factor in mobilizing R&D enthusiasm, and green technology innovation is the only way to achieve sustainable development strategies and “dual carbon” goals. It is an important way for the high-quality development of Chinese society. Therefore, in order to improve the coordinated development system of green technology innovation and financial agglomeration, the government should provide policy subsidies to encourage enterprise innovation, and strengthen the connection between scientific research institutes and financial institutions, so that both parties can strengthen green technology innovation and financial capital according to their own advantages. internal connection.
Thirdly, increase investment in education, extend the number of years of education, and make the promotion of education equality and inclusiveness the focus of future education work. As a populous country, China’s educational resources are in short supply. In this situation of scarcity of educational resources, the allocation of educational resources also has problems such as regional inequality and urban-rural inequality, which limits China’s talent training from many aspects (Gao et al., 2016; Peng et al., 2020). However, the development of education and the birth of new blood are the foundation for the steady development of Chinese society and the vitality of society. Therefore, in addition to paying attention to education investment, it is necessary to pay more attention to the quality of education and the cultivation of students’ quality, to extend the years of education, to improve the fairness of access to educational resources, and to make China truly achieve “successors.”
Finally, improve the green technology review mechanism and the fair financial market competition mechanism, so that the fittest will survive, and no “face project” will be carried out. In order to pursue the so-called “innovation ability”, some enterprises falsify the achievements of green technology development, reuse and declare existing or similar achievements, ignore the transformation of green technology achievements, and regard the achievements as performance figures. The fairness of the market is related to the stability of the industry and even the society, and supervision and development should be combined to make the market operate reasonably within the scope of the “law.”
Data availability statement
The original contributions presented in the study are included in the article/Supplementary Material, further inquiries can be directed to the corresponding authors.
Author contributions
ZH: conceptualization, methodology, software, data curation, formal analysis, visualization, and writing—original draft. XL: writing—review and editing, supervision, and funding acquisition. ZY: writing—review and editing, and supervision. KZ: data curation and writing—review and editing.
Funding
This work was supported by the Pearl River-Xijiang River Economic Belt Development Research Institute Scientific Research Grants for the Key Research Bases of Humanities and Social Sciences of Guangxi Colleges and Universities (ZX2022002), The Science and technology project of Guangxi (Guike AD21220114), National Natural Science Fund of China (11901124), Guangxi University Fundamental Ability Improvement Project for Young and Middle-aged Teachers of China (2021KY1798) and Innovation Project of Guangxi Graduate Education (XYCSZ2022006).
Conflict of interest
The authors declare that the research was conducted in the absence of any commercial or financial relationships that could be construed as a potential conflict of interest.
Publisher’s note
All claims expressed in this article are solely those of the authors and do not necessarily represent those of their affiliated organizations, or those of the publisher, the editors and the reviewers. Any product that may be evaluated in this article, or claim that may be made by its manufacturer, is not guaranteed or endorsed by the publisher.
References
Chang, J. (2022). The role of digital finance in reducing agricultural carbon emissions: Evidence from China’s provincial panel data. Environ. Sci. Pollut. Res. Int., 1–16. doi:10.1007/s11356-022-21780-z
Chen, W., Pan, L., Lin, C., Zhao, M., Li, T., and Wei, X. (2021). Efficiency evaluation of green technology innovation of China’s industrial enterprises based on SBM model and EBM model. Math. Problems Eng. 2021, 1–11. doi:10.1155/2021/6653474
Du, K., Li, P., and Yan, Z. (2019). Do green technology innovations contribute to carbon dioxide emission reduction? Empirical evidence from patent data. Technol. Forecast. Soc. Change 146, 297–303. doi:10.1016/j.techfore.2019.06.010
Faber, A., and Frenken, K. (2009). Models in evolutionary economics and environmental policy: Towards an evolutionary environmental economics. Technol. Forecast. Soc. Change 76 (4), 462–470. doi:10.1016/j.techfore.2008.04.009
Gao, Y., He, Q., Liu, Y., Zhang, L., Wang, H., and Cai, E. (2016). Imbalance in spatial accessibility to primary and secondary schools in China: Guidance for education sustainability. Sustainability 8 (12), 1236. doi:10.3390/su8121236
Guo, W., and Wang, W. (2018). On spatial spillover effects of financial agglomeration on science and technology innovation and heterogeneity in Guangdong-Hong Kong-Macao greater Bay area. J. Guangdong Univ. Finance Econ. 33 (02), 12.
Hall, B. H., and Helmers, C. (2013). Innovation and diffusion of clean/green technology: Can patent commons help? J. Environ. Econ. Manage. 66 (1), 33–51. doi:10.1016/j.jeem.2012.12.008
Hao, A., Tan, J., Ren, Z., and Zhang, Z. (2022). A spatial empirical examination of the relationship between agglomeration and green total-factor productivity in the context of the carbon emission peak. Front. Environ. Sci. 343, 829160. doi:10.3389/fenvs.2022.829160
He, W., Li, W., and Deng, M. (2022). Human capital, green technology innovation, and total factor carbon emission efficiency in the Yangtze River economic Belt. Sci. Technol. Prog. Countermeas. 39 (09), 23–32. doi:10.6049/kjjbydc.C202108003
Hirsch-Kreinsen, H. (2011). Financial market and technological innovation. Ind. Innov. 18 (4), 351–368. doi:10.1080/13662716.2011.573954
Kindleberger, C. P. (1973). The formation of financial centers: A study in comparative economic history. Princeton New Jersey: University Press.
Li, C., Liu, X., Bai, X., and Umar, M. (2020). Financial development and environmental regulations: The two pillars of green transformation in China. Int. J. Environ. Res. Public Health 17, 9242. doi:10.3390/ijerph17249242
Li, Y., and Chen, Y. (2021). Development of an SBM-ML model for the measurement of green total factor productivity: The case of Pearl River Delta urban agglomeration. Renew. Sustain. Energy Rev. 145, 111131. doi:10.1016/j.rser.2021.111131
Liang, Z., Chiu, Y. H., Li, X., Guo, Q., and Yun, Y. (2019). Study on the effect of environmental regulation on the green total factor productivity of logistics industry from the perspective of low carbon. Sustainability 12 (1), 175. doi:10.3390/su12010175
Liu, D., Zhu, X., and Wang, Y. (2021a). China's agricultural green total factor productivity based on carbon emission: An analysis of evolution trend and influencing factors. J. Clean. Prod. 278, 123692. doi:10.1016/j.jclepro.2020.123692
Liu, G., Wang, B., and Zhang, N. (2016). A coin has two sides: Which one is driving China’s green TFP growth? Econ. Syst. 40 (3), 481–498. doi:10.1016/j.ecosys.2015.12.004
Liu, Y., Ji, D., Zhang, L., An, J., and Sun, W. (2021b). Rural financial development impacts on agricultural technology innovation: Evidence from China. Int. J. Environ. Res. Public Health 18 (3), 1110. doi:10.3390/ijerph18031110
Liu, Y., Yang, Y., Zheng, S., Xiao, L., Gao, H., and Lu, H. (2022). Dynamic impact of technology and finance on green technology innovation efficiency: Empirical evidence from China’s provinces. Int. J. Environ. Res. Public Health 19 (8), 4764. doi:10.3390/ijerph19084764
Meirun, T., Mihardjo, L. W., Haseeb, M., Khan, S. A. R., and Jermsittiparsert, K. (2021). The dynamics effect of green technology innovation on economic growth and CO2 emission in Singapore: New evidence from bootstrap ARDL approach. Environ. Sci. Pollut. Res. 28 (4), 4184–4194. doi:10.1007/s11356-020-10760-w
Muhsin, K., Nazlioglu, S., and Agir, H. (2011). Financial development and economic growth nexus in the MENA countries: Bootstrap panel granger causality analysis. Econ. Model. 29 (3), 68 693. doi:10.1016/j.econmod.2010.05.015
Peng, H., Qi, L., Wan, G., Li, B., and Hu, B. (2020). Child population, economic development and regional inequality of education resources in China. Child. Youth Serv. Rev. 110, 104819. doi:10.1016/j.childyouth.2020.104819
Shi, Q., and Lai, X. (2013). Identifying the underpin of green and low carbon technology innovation research: A literature review from 1994 to 2010. Technol. Forecast. Soc. Change 80 (5), 839–864. doi:10.1016/j.techfore.2012.09.002
Shijin, S. H. U. A. I., Zhi, W. A. N. G., Xiao, M. A., Hongming, X. U., Xin, H. E., and Jianxin, W. A. N. G. (2021). Low carbon and zero carbon technology paths and key technologies of ICEs under the background of carbon neutrality. J. Automot. Saf. Energy 12 (4), 417. doi:10.3969/j.issn.1674-8484.2021.04.001
Sun, P., Liu, L., and Qayyum, M. (2021). Energy efficiency comparison amongst service industry in Chinese provinces from the perspective of heterogeneous resource endowment: Analysis using undesirable super efficiency SBM-ML model. J. Clean. Prod. 328, 129535. doi:10.1016/j.jclepro.2021.129535
Tone, K. (2004). Dealing with undesirable outputs in dea: A slacks-based measure (SBM) approach. Toronto: Presentation At NAPW III, 44.
Wang, H., Cui, H., and Zhao, Q. (2021a). Effect of green technology innovation on green total factor productivity in China: Evidence from spatial Durbin model analysis. J. Clean. Prod. 288, 125624. doi:10.1016/j.jclepro.2020.125624
Wang, X., Hu, L., and Fan, G. (2021b). Marketization index report by provinces in China. Beijing: Social Sciences Academic Press.
Wang, Y., Xie, L., Zhang, Y., Wang, C., and Yu, K. (2019). Does FDI promote or inhibit the high-quality development of agriculture in China? An agricultural GTFP perspective. Sustainability 11 (17), 4620. doi:10.3390/su11174620
Wang, Y., Zhang, Y., Li, Q., Zhou, X., Chen, W., and Niu, Y. (2004) The interaction of financial agglomeration and green development: Evidence from 284 cities in China. Available at SSRN 4122783. Amsterdam: Elsevier. doi:10.2139/ssrn.4122783
Wen, Y., Zhao, M., Zheng, L., Yang, Y., and Yang, X. (2021). Impacts of financial agglomeration on technological innovation: A spatial and nonlinear perspective. Tech. Analysis. Strategic Manag. 365, 1–13. doi:10.1080/09537325.2021.1963702
Xie, H., Ouyang, and Z., and Choi, Y. (2020). Characteristics and influencing factors of green finance development in the Yangtze River Delta of China: Analysis based on the spatial Durbin model. Sustainability 12, 9753. doi:10.3390/su12229753
Xie, R., Fu, W., Yao, S., and Zhang, Q. (2021). Effects of financial agglomeration on green total factor productivity in Chinese cities: Insights from an empirical spatial Durbin model. Energy Econ. 101, 105449. doi:10.1016/j.eneco.2021.105449
Xue, Q., Feng, S., Chen, K., and Li, M. (2022). Impact of digital finance on regional carbon emissions: An empirical study of sustainable development in China. Sustainability 14 (14), 8340. doi:10.3390/su14148340
Ye, Q., and Cheng, C. (2019). Green technological innovation efficiency and financial ecological environment. Open J. Soc. Sci. 7 (12), 132–151. doi:10.4236/jss.2019.712011
Ye, T., Zheng, H., Ge, X., and Yang, K. (2021). Pathway of green development of Yangtze River economics Belt from the perspective of green technological innovation and environmental regulation. Int. J. Environ. Res. Public Health 18 (19), 10471. doi:10.3390/ijerph181910471
Zhang, K., and Wang, D. (2014). The interaction and spatial spillover between agglomeration and pollution. China Ind. Econ. 06, 70–82. doi:10.19581/j.cnki.ciejournal.2014.06.007
Zhao, H., Yang, Y., Li, N., Liu, D., and Li, H. (2021). How does digital finance affect carbon emissions? Evidence from an emerging market. Sustainability 13 (21), 12303. doi:10.3390/su132112303
Keywords: the goal of “double carbon”, green technology innovation, generalized three-stage least squares method, spatial simultaneous equations, financial agglomeration
Citation: Han Z, Li X, Yan Z and Zhong K (2022) Interaction and spatial effects of green technology innovation and financial agglomeration: Empirical evidence from China under the goal of “double carbon”. Front. Environ. Sci. 10:984815. doi: 10.3389/fenvs.2022.984815
Received: 02 July 2022; Accepted: 25 July 2022;
Published: 26 August 2022.
Edited by:
Irfan Ullah, Nanjing University of Information Science and Technology, ChinaReviewed by:
Baoliu Liu, Beijing University of Technology, ChinaDong Mei, Institute of Agricultural Resources and Regional Planning (CAAS), China
Copyright © 2022 Han, Li, Yan and Zhong. This is an open-access article distributed under the terms of the Creative Commons Attribution License (CC BY). The use, distribution or reproduction in other forums is permitted, provided the original author(s) and the copyright owner(s) are credited and that the original publication in this journal is cited, in accordance with accepted academic practice. No use, distribution or reproduction is permitted which does not comply with these terms.
*Correspondence: Xingyi Li, bGl4aW5neWlpcmVuZUAxNjMuY29t; Zhen Yan, emhlbnlhbkBneG51LmVkdS5jbg==