- 1School of Government, Sun Yat-sen University, Guangzhou, Guangdong, China
- 2School of Business, Sun Yat-sen University, Guangzhou, Guangdong, China
- 3School of Business, The University of Sydney, Sydney, NSW, Australia
- 4School of Sociology and Anthropology, Sun Yat-sen University, Guangzhou, China
This paper aims to explore the effectiveness of carbon emissions trading system by using mixed method research. In the past 5 years, the development level of carbon finance in Hubei Province is in the first tier. Hubei carbon emission trading center was established in September 2012, and now ranks top in the trading volume and total transaction amount of China’s seven pilot exchanges. Therefore, choosing Hubei Province as a sample to study the carbon emissions trading system has good externalities, that is, the research conclusions are highly representative. In order to make an in-depth analysis of the system construction and operation effect of the environmental equity trading market of carbon emission rights, this paper uses the closure of Hubei Province as an instrumental variable to alleviate the endogeneity in previous studies, and makes an empirical study on the effectiveness of the carbon emission trading system. The study finds that: after alleviating endogeneity, the current carbon emissions trading has reduced air quality. At the same time, this paper theoretically and statistically verifies the correlation and exogenous of the instrumental variable of city closure to ensure the credibility of the results. This paper further analyzes and finds that the lower the degree of industrialization and the worse the air quality, the higher the degree of air quality reduction. In addition, this paper conducted a series of robustness tests, such as adding new traffic related control variable, replacing variable indicators of air quality, conducting placebo test, and conducting lag regression, which verifies that the benchmark regression results are still valid. In the mechanism part, this paper uses the methods of interview and case analysis to analyze the causal mechanism of this phenomenon from the perspective of the system itself and the challenges in the implementation process. As far as the system itself is concerned, this paper believes that the current carbon emissions trading system does not cover a comprehensive range, there are defects in the way of carbon quota allocation, and the accuracy of the current verification method needs to be tested; In the process of implementation, there are “local protectionism” and information asymmetry.
1 Introduction
Existing research demonstrates that the negative impact of air pollution is multi-faceted, mainly from economic development and social life. At the economic level, when air pollution levels increase, the “pessimistic expectations” of managers of polluting firms and their investors about future environmental policies lead to a decrease in government spending, which in turn leads to a decline in corporate investment activity and financing capacity (Cheng et al., 2022; Feng et al., 2022). Further, when urban air quality deteriorates, the number of tourists and tourism revenue decrease accordingly (Ma et al., 2021). At a societal level, air pollution can greatly harm public health. Research has found that people’s memory declines in areas with higher levels of NO2 and PM10 (Powdthavee and Oswald, 2020). Besides, the prevalence of asthma among children was higher in major air pollution areas with higher PM10 (Norbäck et al., 2019). With the deepening of research on the hazards related to air pollution, the public has a deeper understanding of the hazards of air pollution; and has begun to realize the importance of air quality (Li X. et al., 2022). The advancement of industrialization has vigorously promoted social and economic development (Sikder et al., 2022). Nonetheless, the resulting high-emission manufacturing industry has led to the deterioration of the urban environment (Fang et al., 2022). Societies cannot hinder economic development from improving ambient air quality. Hence, how to contribute to sustainable economic and social development has become one of the hot topics in recent years, and the carbon emission trading market has emerged as the times require. Figure 1 shows the development history and prospect of China”s carbon emissions trading market.
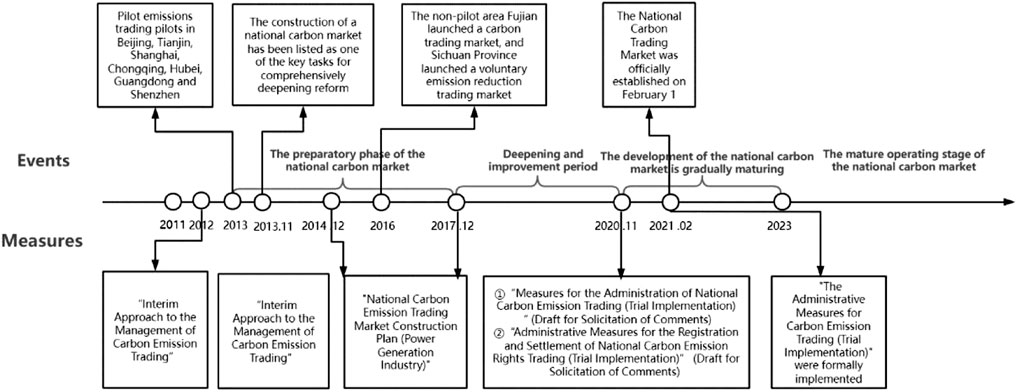
FIGURE 1. Development history and prospect of China’s carbon emissions trading market. Source: Handmade according to the national development and reform commission, the general office of ecology and environment and other relevant departments.
The carbon financial market mainly consists of carbon trading, investment and financing, and carbon intermediation (Jijian et al., 2021), among which the most important one is carbon emissions trading. The American economist Dales first proposed the concept of “carbon credits” in 1968. Its prototype originated from the Pigou tax theory, which refers to solving the problem of environmental pollution by taxing enterprises with large pollution emissions, internalizing negative externalities, thus reducing environmental pollution to the Pareto optimal level (Kouznetsov et al., 2019). This proposition offers an excellent solution to the “free-rider” behavior of high carbon emission enterprises brought by the non-exclusive and competitive nature of carbon emission reduction. Since then, the Pyrrhic tax has shown a market trend (Liu et al., 2021). The government or the state artificially sets a total amount of pollutant emissions and endows this emission rights with scarcity characteristics so that it can be traded as an intangible commodity, and finally use the market price adjustment mechanism and other means to achieve the purpose of controlling enterprise pollution emissions (Du and Li, 2021; Wang and Zhang, 2022), which is also the current rules of the carbon emission trading market. Figure 2 shows 2020 national carbon emission rights registration, trading and settlement management mechanism.
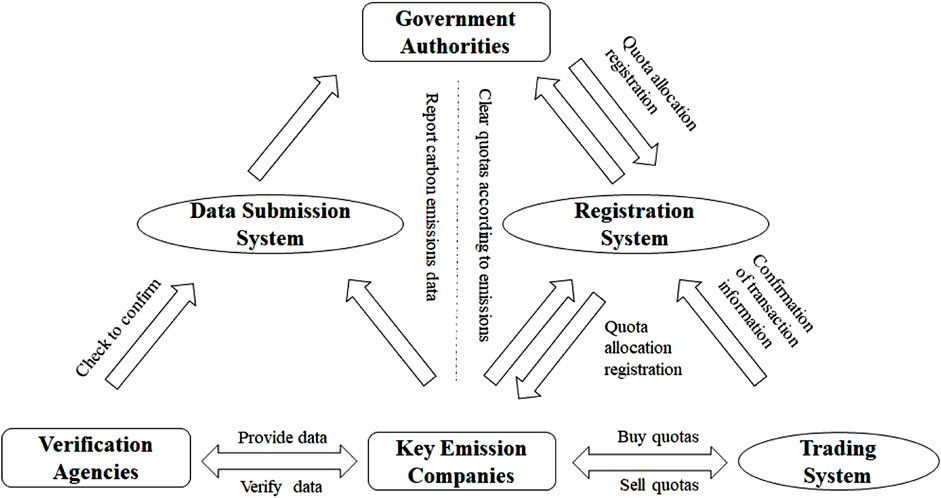
FIGURE 2. 2020 National carbon emission rights registration, trading and settlement management mechanism. Source: Compiled and produced according to the 2021 measures for the administration of carbon emission trading issued by the general office of ecology and environment (trial implementation) and other relevant materials.
As a result, it can be found that the premise of carbon trading is that the revenue brought by the increase in the production of products with pollutant emissions is greater than the increase in the cost brought by the internalization of carbon emission costs; and the difference between the revenue and the cost is what leads firms to purchase allowances in the carbon emission market (Peng et al., 2021a; Peng et al., 2022; Zou and Zhong, 2022). Compared with the relevant environmental departments’ leading role in formulating clear carbon emission reduction tasks or implementing mandatory pollutant emission taxation, the implementation of carbon emissions trading can store enterprises’ voluntary emission reduction behavior (Li et al., 2021; Peng et al., 2021b), which enables market players to make behavioral decisions after weighing profit and loss through market signals, and uses market regulation to allow enterprises to pursue private benefits while objectively achieving environmental policy goals. The market regulation allows firms to achieve optimal resource allocation flexibly and efficiently.
Compared with developed countries in Europe and the United States, China’s carbon emission market started relatively late (Yu et al., 2021). It was not until 2013-2014 that China began to pilot carbon emissions trading. As a supplementary mechanism for the carbon trading market, carbon allowances can be used for trading and greenhouse gas emission credits for emission control enterprises and other products such as voluntary emission reductions CCER1, have strongly promoted the development of the domestic carbon emission market (Gu et al., 2022). This study uses empirical evidence to explore the effectiveness of the carbon emissions trading system (Wang et al., 2022). Among the first seven pilot transactions established in China, this study selects Hubei Province as the research object. Hubei is located in the central south of China and the middle reaches of Yangtze River. Its economic development ranks among the top in the country, and its urban greening construction and industrial development are representative. Figure 3 demonstrates the carbon emissions trading in pilot markets nationwide as of August 2020.
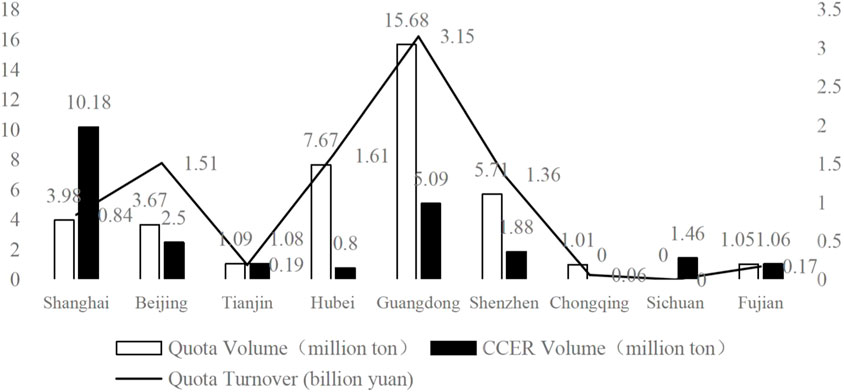
FIGURE 3. Carbon emissions trading in pilot markets nationwide as of August 2020. Source: Carbon emission volume data comes from the wind database.
Moreover, in the “Thirteenth Five-Year Plan,” Hubei’s representative proposes that “We should deeply promote carbon emission trading and improve the carbon emission trading system. Vigorously promote the construction of Hubei Carbon Emission Rights Trading Center and strengthen the connection with the national carbon market.” Additionally, in the past 5 years, the development level of carbon finance in Hubei has been in the first echelon (Cao et al., 2021); Hubei Carbon Emission Rights Trading Center was established earlier, in September 2012, and currently ranks the top of the seven pilot exchanges in China in terms of volume and total transaction amount. Thus, as a sample to study the carbon emission trading system, Hubei exhibits a good externality, which can improve the representativeness and validity of this study.
This study has three main contributions. First, the existing literature on carbon emissions is limited, and there are certain theoretical gaps in this field, and the research of this study enriches the theory of carbon emission system research. Second, in terms of research methods, this study creatively uses the exogenous impact of city closure as an instrumental variable to alleviate the endogeneity of two-way causality, which has certain empirical innovation. This study uses both the interview and case methods to explore the mechanism and the mixed research method science. Third, China’s carbon emissions trading system will be gradually improved, and it is expected to achieve “peak carbon” by 2030 and “carbon neutral” by 2060. This study empirically studies the effectiveness of the current system and explores the mechanism, providing certain experience for the next development and adjustment, which has strong practical significance.
The study is organized as follows: Part II is the literature review and research hypothesis; Part III is the research design; Part IV is the empirical results and analysis; Part V is further analysis; and Part VI is the conclusion.
2 Literature review and research hypothesis
Regarding the factors affecting air quality, in terms of economics, the Environmental Kuznets Curve (EKC) points out that air quality (environmental pollution) and economic growth have an “inverted U” shaped relationship (Chen and Taylor, 2020; Xu et al., 2020; Zhang et al., 2021). Moreover, economic growth and factors such as urbanization rate and industrial structure impact air quality with reference to the extended environmental Kuznets curve (Xu et al., 2019; Wu et al., 2022). The air quality has a positive relationship between urban population size and urban environmental pollution level.
Economic development can increase the degree of environmental pollution to a certain extent (Sun et al., 2021). Hence, “green development” is an important way to deal with the relationship between the environment and the economy by integrating “green development” into the overall economic development strategy (Tang P. et al., 2021). Green development has become the main theme of the development of the new era of socialism with Chinese characteristics and is bound to become the code of conduct guiding China’s future economic and social development (Vickers, 2009; Gunnarsdottir et al., 2021). China’s economy has shifted from a stage of high-speed growth to a stage of high-quality development, which needs to rely on green finance and other financial assistance to promote a new economic growth mode.
As for the operational utility of carbon finance, there are various existing evaluation systems (Polzin and Sanders, 2020; Chen et al., 2021; Nechifor et al., 2022). One is economic indicators, such as testing whether the pilot mechanism of carbon emission trading rights can improve the total factor productivity of industry in the pilot region (Li C. et al., 2022; Wu and Wang, 2022). The second is market indicators, such as using the rescaled gradient analysis proposed by Hurst to test the fluctuation of the daily carbon price consisting of the carbon price time series (Li J. et al., 2022). The third is environmental indicators, which can be used to examine the total emissions of three industrial pollutants such as carbon dioxide, industrial wastewater, industrial sulfur dioxide, and industrial solid waste (McGrath et al., 2022; Sahin and Esen, 2022). To deeply analyze the institutional construction and operational effects of carbon emission rights, and environmental equity trading market, this study focuses on the emission reduction effects of carbon emission trading policies from the original purpose of carbon market establishment. Environmental indicators are adopted to study the utility of the carbon market. The operation of carbon markets that can significantly reduce carbon emissions has constructed theoretical logic from the perspectives of corporate costs, technological innovation, and consumer preferences (Peng et al., 2022; Sovacool et al., 2021). Carbon price as the production cost of enterprises, and argue that enterprises improve production technology and promote emission reduction to reduce this cost, which in turn reduces social costs (Shi et al., 2021; Zhang and Wang, 2021; Wang and Zhang, 2022). The party selling carbon emission rights, carbon emission trading policy promotes the realization of carbon emission through market revenue inducement, technological innovation incentive effect, and government support effect reduction (Wang et al., 2018; Yao et al., 2020; Tang W. et al., 2021). While for the party who buys carbon emission rights, carbon emission trading achieves carbon emission reduction through the effects of enterprise cost pressure effect, process innovation motivation effect, and market guidance effect, thus improving air quality (Li G. et al., 2022). Besides, from the perspective of consumers, carbon emission trading can motivate consumers to choose low-carbon products, thus reducing carbon emissions. Hence, we assume that:
Hypothesis 1. Carbon emissions trading decreases air pollution and improve air quality.In contrast, there are negative attitudes toward the emission reduction effect of carbon markets, and they mostly argue their views from the perspective of imperfect institutional mechanism of carbon market (Cong and Lo, 2017; Song et al., 2018; García and Torvanger, 2019). China’s carbon finance suffers from low participation of citizens, an inadequate legal system, and an imperfect pricing mechanism, leading to its inability to achieve its original operational goals (Carrai, 2021; Zhang et al., 2020). Specifically, enterprises pursuing economic benefits and responding to the pressure of assessment may lack the concept of long-term sustainable development to cope with the needs of assessment (Tu and Wu, 2021; Appolloni et al., 2022; Bai and Satir, 2022). Coupled with the fact that the content of China’s system is mostly expressed as comprehensive and principled, and there is room for operation in the actual implementation, and it may have negative utility. Thus, we posit:
Hypothesis 2. Carbon emissions trading increases air pollution and worsens air quality.
3 Study design
3.1 Sample selection
In order to take advantage of the exogenous shock of epidemic city closure as an instrumental variable for carbon emissions trading and eliminate the impact of different months and seasons on air quality; based on the fact that most cities have lifted the city closure in mid-March, this study selected Hubei’s city-day data from 1 January to 31 March 2020, and the same period last year, 1 January to 31 March 2019, as samples for research. Among them, the daily air quality data of each city were obtained from China Air Quality Online Monitoring and Analysis Platform using python crawler; the epidemic closure period of each city in Hubei in 2020 was manually collected from the portal of each city’s government; the daily data of weather conditions such as temperature, humidity, air pressure, sunshine hours, and average wind speed were obtained from China Meteorological Science Data Center; the data of fixed asset on the completion of investment in each province were collected from the provincial statistical bureaus; the data on the proportion of secondary production to GDP in each province were collected manually from the National Bureau of Statistics; the daily data on the daily turnover of carbon emission rights were collected from the Wind database; the total number of emission control enterprises in each city were collected manually from the official website of the Development and Reform Commission of Hubei Province. After completing data collection, we matched the air quality data, meteorological data, economic data, and instrumental variables and excluded the samples from non-carbon trading days. Finally, we obtained 546 city-day observations for eight cities, namely Enshi, Jingzhou, Shiyan, Suizhou, Wuhan, Xiangyang, Xiaogan, and Yichang.
3.2 Variables and model settings
(1) Air quality: Domestic scholars mostly use air quality-related indicators to measure the effectiveness of carbon emissions trading. For example, Li et al. (2020) uses the indicators of carbon dioxide, industrial wastewater, industrial sulfur dioxide, and industrial solid waste emissions to assess the effect of carbon emissions trading policies on regional pollution emissions. The Air Quality Index (AQI) describes the degree of clean or polluted air, and the pollutants involved in this evaluation index are sulfur dioxide (SO2), nitrogen dioxide (NO2), respirable particulate matter (PM10), ozone (O3), carbon monoxide (CO), and fine particulate matter (PM2.5). This indicator can reflect the air quality more comprehensively and has been used as the main indicator of urban air quality in the literature, which is also chosen as the dependent variable.
In particular, a larger AQI indicates poorer air quality. Specifically, AQI between 0 and 50 indicates excellent air quality, between 51 and 100 indicates good air quality, between 101 and 150 indicates light pollution, between 151 and 200 indicates moderate pollution, between 201 and 300 indicates heavy pollution, and above 300 indicates severe pollution. This study used the variable lnAQI as the dependent variable after taking logarithms to eliminate heteroskedasticity.
(2) Carbon Emissions Trading: The research on carbon emissions trading in China is still developing, while more scholars focused on normative research; fewer scholars conducted relevant empirical research, while fewer scholars constructed the measurement indexes for carbon emissions trading. This study collected the daily carbon emission trading volume of each city but considering the large differences in the number of emission control enterprises in different cities, it was easy to ignore the influence of the original volume of emission enterprises in each city by simply using the trading volume. Accordingly, this study drawed on the latest study by Chen et al. (2020), which uses the evaluation index system of carbon finance development level constructed to measure the degree of local carbon emissions trading using the market depth evaluation factor targeting carbon emissions trading (CT). Further, considering the distribution characteristics and size of the total number of emission control enterprises, we used the logarithm of the total number of emission control enterprises and the daily volume of carbon emission allowances traded in each city in Hubei to generate a market depth variable. We divided each city’s daily carbon emission quota trading volume by the number of emission control enterprises in each city disclosed on the official website of Hubei Provincial Development and Reform Commission, took the logarithm, and generate lncet to measure the degree of carbon emission trading.
(3) Control variables: Drawing on previous related literature, there are three general categories of control variables related to air quality, the first being temporal trend fluctuations. Previous research has mostly explored air quality changes over longer time scales, setting seasonal dummy variables to control fixed temporal effects. In this study, we selected daily data to explore while controlling daily temporal fixed effects.
The second category is meteorological level variables closely related to air quality. In this study, urban average temperature, relative humidity, average air pressure, sunshine hours, and average wind speed were selected as meteorological class control variables, all of which were daily data.
The third category is the control variables of social and economic categories. Based on the availability of data and the rationality of data structure, we selected the share of secondary industry in GDP and fixed asset investment in secondary industry as the control variables of social and economic categories. Among them, the share of secondary industry in GDP is quarterly data, and the investment in secondary industry fixed assets was monthly data.
(4) This study manually collected the city closure and unclosure dates for each city in Hubei during the epidemic and set the closure dummy variable lockdown, where the lockdown value is 1 if a city was under closure and 0 if the city was not under closure or has been unsealed.
The specific variable settings and data sources for each variable are shown in Tables 1, 2.
3.2.1 Model settings
Based on the above variable design, the study constructed a panel two-way fixed effects model as follows.
Where i represents the city and t represents the date, lnAQIit is the core dependent variable. Because considering the lagged effect of carbon emissions trading on air quality, the carbon emissions trading on the same day is unlikely to impact the air quality on the same day. This study took lncetit-1 with a one-period lag as the core independent variable; Xit represents all the control variables mentioned above, μi represents the city fixed effect, δt represents the time fixed effects, and εit is the random error term.
Additionally, previous studies have shown that air quality can also impact carbon emissions trading. For example, carbon emissions trading is more frequent in areas with better air quality itself; therefore, there is a two-way causal relationship between environmental factors and carbon finance development (Ruan, 2020). Therefore, in terms of endogeneity, this study used the “city closure” policy as an instrumental variable to study the impact of the operation of carbon markets on air quality. The COVID-19 in 2020 led to the closure of several high-risk areas in Hubei. The government hopes to control the spread of novel coronavirus by restricting the flow of people between cities, supplemented by traffic control (Zhang et al., 2020). The closure of cities caused the shutdown of enterprises and hindered normal economic development, thus reducing the activity of allowance trading in the carbon emission market and affecting the development of carbon finance. While the direction of capital allocation and investment decisions in the financial sector impact the changes in the ecological environment, impact, affects air quality. However, the city closure as a good exogenous shock is not directly related to air quality (the city closure only controls the flow of people as well as traffic in and out of the city, and does not form a physical barrier to inhibit the flow of polluting gases outside the region) The empirical results show that there is a significant change in the daily degree of carbon emission trading in the carbon emission trading market in Hubei before and after the city closure. Thus, the instrumental variable of city closure can better alleviate the two-way causality problem in previous literature, studying the effect of carbon markets on air quality and identifying the effect of carbon emissions trading on air quality.
Therefore, this study used lockdown as an instrumental variable and constructs a two-stage regression model to alleviate the potential endogeneity problem.
The model represents that the first stage regression regresses the endogenous variable lncetit-1 on the instrumental variable lockdownit-1 (to satisfy the correlation, the closure of the city is only related to the carbon emissions trading on that day) to generate the predicted values of the endogenous variable. Afterward, the second stage regression regresses lnAQIit on the predicted values of the endogenous variables. According to the two-stage instrumental variables regression, the regression coefficients at this point are consistent estimates that better mitigate the endogeneity results.
4 Empirical results and analysis
4.1 Descriptive statistics
Table 3 reports the results of the descriptive statistics. The mean value of InAQI is 4.281 with a standard deviation of 0.43, indicating that the observations have a better overall air quality (AQI is 50–100) with less variation. The mean value of lncet is 3.51 with a standard deviation of 2.63, indicating that the observations have more variation in carbon emissions trading. This variation, which may come from different cities, may also come from different days. The carbon emissions trading market shows a more unstable and uneven characteristic. The mean value of lockdown is 0.22, indicating that 22% of the total sample is in the closed city-state; namely, the number of closed days accounts for 22% of the total number of days in the sample. For the control variables, each variable’s data are consistent with existing studies.
4.2 Baseline regression
Table 4 reports the results of the baseline regressions. To mitigate possible autocorrelation or heteroskedasticity problems, the regressions in this study are all adjusted for clustering at the city level and control the city and date fixed effects. Column (1) is the regression of model (1) without lagging carbon emissions trading and control variables, and the result is negatively insignificant. Column (2) shows the regression results of model (1) without control variables, where the relationship is negatively significant. In Column (3), the regression of model (1) without lagged carbon trading but with control variables is negatively significant but less significant than the lagged regression. Column (4) shows the regression results of model (1), which shows that carbon emissions trading reduces AQI values, (i.e., improving air quality). All four results columns verify Hypothesis 1 and justify the regression on the lag of carbon emissions trading well by comparison.
Column (5) presents the result of the first stage regression of the instrumental variables in the model (2). The result shows that the city closure significantly reduces carbon emissions trading, a finding that we can easily explain after the city closure, the production in the cities stagnates, which makes the firms that originally exceeded the carbon emissions limit no longer need to buy allowances from the firms with low carbon emissions. The lack of market demand leads to a decrease in carbon emissions trading. In summary, we demonstrate that the instrumental variable of sealing city has a good correlation from both theoretical and empirical aspects. Column (6) shows the result of the second stage regression of the instrumental variable, which is the regression of model (3). In this regression, according to the instrumental variable, we better mitigate the endogeneity problem mainly due to the two-way causality. We found that carbon emissions significantly increase the AQI values, namely, decreasing air quality, verifying Hypothesis 2.
For this result, this study suggests the following possible explanations: political tournament. Since China’s incentives stem from a combination of economic decentralization and a vertical political governance system, local governments are more committed to developing the local economy for political progress. Therefore, by lowering environmental regulatory standards or using local governments’ autonomy in environmental regulation, it may benefit foreign capital or other firms to attract more foreign capital and other liquidity elements (Zhu et al., 2011). Which lead to an incomplete implementation of the carbon market mechanism and ultimately make the carbon market ineffective in achieving its initial purpose. Specifically, local governments tend to use their autonomy to grant carbon emission quotas to enterprises with high production value and high pollution in the region to get promoted in the circuit of tournaments, thus developing the economy and reducing the air quality.
The benchmark results in this study demonstrate two opposing conclusion. First, based on the consideration of endogeneity issues, this study prefers to believe the results of the second-stage regression of instrumental variables. A possible explanation for this result is that there is a serious two-way causality problem between carbon emissions trading and air quality, namely, regions with originally poor air quality are likely to be the result of excessive pollutant emissions from industrial enterprises due to excessive economic development. Therefore, these regions also have a greater need to establish a sound carbon emissions trading system and a greater demand for the market. The instrumental variables regression in this study, on the other hand, uses the policy of city closure, an exogenous shock, which can mitigate endogeneity and obtain more reliable and consistent estimates. In summary, the baseline regressions in this study support Hypothesis 2, that carbon emissions trading reduces air quality.
4.3 Instrumental variables exogeneity discussion
An ideal instrumental variable needs to satisfy both the conditions of correlation and exogeneity. Correlation refers to the fact that the instrumental variable needs to be correlated with the endogenous variable, and exogeneity refers to the fact that the instrumental variable is independent of the nuisance term. For the discussion of correlation, theoretical and empirical tests have been given in this study in the section on analyzing the first-stage regression results of the benchmark regression instrumental variables. Therefore, the following discussion addresses exogeneity.
Regarding the exogeneity of instrumental variables, scholars tend to focus on theoretical analysis because direct statistical tests can only be conducted when over-identification (the number of instrumental variables is greater than the number of endogenous explanatory variables). Nevertheless, selecting instrumental variables require inspiration, and it is more difficult to come up with multiple instrumental variables for endogenous variables simultaneously. In this study, we test the instrumental variables theoretically and empirically.
Generally speaking, the instrumental variables can be divided into aggregated data, “physiological phenomena” such as weather patterns, birth, age, sickness, and death, variables from “social space” such as distance and price, and experimental variables from natural and virtual experiments (Chen, 2012). In this study, we consider the epidemic in Hubei. The policy of city closure in Hubei due to the epidemic is considered an exogenous shock, providing a quasi-natural experiment for our study and creating experimental conditions. In terms of policy motivation, the purpose of city closures is to control people’s travel and block the spread of the epidemic, there is no directly relevant impact on air quality, which is why city closures are considered a good exogenous shock experiment in our study. Therefore, we argue that the city closure policy satisfies the exogeneity requirement of the instrumental variable from the theoretical analysis.
To more rigorously demonstrate the exogeneity of the city closure policy, this study draws on Chen and Taylor (2020) and introduces another instrumental variable related to carbon emissions trading but not air quality to achieve an over-identification test. In previous studies, He and Wu (2015) measured the degree of marketization of a region using the share of private sector employment in the region’s total employment. In general, the more developed a region is in marketization, the lower its transaction costs (Chu and Liu, 2014), thus suppressing to a certain extent the excessive price of allowances (Liu, 2020) and making carbon emissions trading more active. It is also evident that the share of regional private employment in the total population does not affect air quality. Therefore, this study argues that the level of regional marketization measured by the private employment/total population construct variable satisfies the instrumental variable.
Additionally, we obtained the number of private and self-employed persons in each city from the China Urban Statistical Yearbook and the population data of each city in Hubei from the Hubei Statistical Yearbook. We then divide the two to get the marketability variable Proportion_pe, which is used as another instrumental variable for the two-stage regression results of the instrumental variable, as shown in Table 5.
As shown in Table 5, the second-stage regression results remain robust after adding a new instrumental variable, supporting Hypothesis 2. Meanwhile, the F-test value of the first-stage regression is 15.1197, which is greater than 10, and there is no weak instrumental variable problem. Most importantly, the p-value corresponding to the chi-square value in the over-identification test is 0.1094, which is higher than 0.1, so we can assume that the instrumental variable selected in this study satisfies exogeneity.
4.4 Heterogeneity analysis
4.4.1 Path dependency: Industrialization and carbon emissions trading
Gao et al. (2019) found that carbon emissions trading policies ultimately have a suppressive effect on the carbon emissions intensity of construction land through a relative decrease in energy intensity by examining panel data of 30 provincial units in China from 2007 to 2016. In contrast, Li (2015) argues that industrialization is essentially a process of continuous optimization and upgrading of advanced industrial structures; he verifies the conjecture that the increase in industrialization level can effectively improve energy intensity based on the data of 30 Chinese provinces from 2005 to 2013. On the other hand, carbon emission trading systems originate from solving the carbon emission problem caused by enterprise production. Therefore, this study argues that different levels of regional industrialization can cause differences in the effectiveness of carbon emission trading systems.
To investigate the differences in the impact of carbon emissions trading on air quality among regions with different degrees of industrialization, this study follows the control variable indus used above. First, it generates a dummy variable indus0 for the full sample according to whether it is higher than the mean value of indus, which is recorded as 1 if it is higher than the mean value of indus and 0 otherwise. After that, the interaction term lncet with indus0 has generated lncet_indus. lncet_indus is added to the instrumental variable regression model with indus0 in this study, and the same lagged period is regressed.
Columns (1) and (2) of Table 6 show the results of the second-stage regression of instrumental variables without and with the inclusion of control variables, respectively. The lagged term of lncet_indus is significantly negative after including control variables, indicating that the degree of regional industrialization negatively moderates the effect of carbon emissions on air quality. The higher the degree of industrialization, the weaker the effect of carbon emission trading activities on air quality reduction. The more experienced and efficient an industrialized region is with regard to energy use, the more efficiently its energy use is integrated. However, high industrialization increases energy intensity, reducing the magnitude of the reduction in local energy intensity that carbon emissions trading has, affecting the effectiveness of carbon emissions trading in improving air quality.
4.4.2 The heavy lift: Air quality and carbon emissions trading
Li et al. (2014) point out that there is a phenomenon that environmental regulation variables and regional eco-efficiency variables determine each other simultaneously; in other words, the eco-efficiency of a region before the introduction of an environmental regulation affects the effect of the implementation of that environmental regulation. Therefore, when exploring the effect of carbon emissions trading, the previous environmental level of each region cannot be ignored. Is this effect related to the original air quality level of each city? To further investigate this issue, the eight cities are grouped according to whether the average AQI value exceeds 80 during the sample period. This grouping has two bases: 1. the average AQI value of the whole sample is 81, which is close to 80. 2. The AQI value of 80 is used as the boundary to divide the sample, which gives exactly four cities in each of the two groups. The difference in the number of samples between groups is small, making the comparison more convincing. Among them, the cities with the AQI mean value of less than 80 are Enshi, Shiyan, Wuhan, and Xiaogan, and there are 304 samples in this group; the cities with the AQI mean value greater than 80 are Jingzhou, Suizhou, Xiangyang, and Yichang, and there are 233 samples in this group.
Table 7 reports the results of the second-stage regression of the grouped instrumental variables, and we can see that they are insignificant when no control variables are included. The inclusion of control variables shows that the cities with lower original air quality are more negatively affected. This shows that society should pay enough attention to the deterioration of air quality; otherwise the “green mountain and clear water” will be gone forever.
4.5 Robustness test
To ensure the empirical results’ robustness, this study has done the following robustness tests.
1. Although this study mitigated the endogeneity of two-way causality by using city closure as an instrumental variable, there was a problem of omitted variables. We attempted to collect relevant city-level variables when selecting control variables. However, based on the “city-day” data structure of this study and the requirements of the 2019-2020 data interval; data unavailability is high thus there were fewer control variables. On the other hand, considering the serious traffic of the epidemic and the close correlation between traffic data and air quality, we attempted to use the available data to construct the transportation variable transport for each city to join the instrumental variables in the second stage regression to alleviate the endogeneity problem of omitted variables, which was constructed as follows.
We obtained the data of “regional road passenger and freight transport volume and turnover” from CSMAR database, the population data of each city in Hubei from Hubei Statistical Yearbook, and the epidemic-related data of each city in Hubei from CEIC economic database and constructed the transportation variable transport = the resident population of each city/the province’s resident population * the province’s transportation from January to March 2019. Transportation variable transport = resident population of each city/resident population of the province * passenger transport volume of the province. At the same time, considering the impact of the epidemic on transportation in 2020, this study constructed the transportation variable transport = resident population of each city/resident population of the province * (1 − cumulative confirmed new crown cases in each city/resident population of each city) * province for January-March 2020 passenger traffic to incorporate the impact of the epidemic on transportation.
2. We considered changing the variable indicator of the dependent variable air quality by replacing lnAQI with PM2.5 and PM10 for regression, respectively. Second, in terms of independent variables, this study used each city’s daily carbon emission trading volume for measurement without considering the influence of the number of emission control enterprises.
3. The sample interval was widened by complementing and using the daily data of each city in April 2020 and 2019 for the placebo test. Because the cities were unsealed in April 2020, the validity of city closure as an instrumental variable would gradually disappear. If the instrumental variables in this study were valid, the second-stage regression of the instrumental variables in this period would not be significant.
4. We previously generated lncet variables based on data characteristics, and now regressions are performed without taking logarithms using cet as the independent variable.
5. In this study, considering that there is a time lag in the impact of carbon emissions trading on air quality, the lncet is lagged one period as the independent variable and is now regressed two and three periods, respectively.
Table 8 reports the results of the robustness tests of this study, and all robustness tests of this study report the results of the second-stage regression of the instrumental variables. Among them, column (1) reports robustness test 1, column (2), (3), (4) reports robustness test 2, column (5) reports robustness test 3, column (6) reports robustness test 4, and column (7), (8) report robustness test 5. From the regression results of the robustness tests, all of them support Hypothesis 2.
5 Mechanism analysis
Since qualitative research is more powerful than quantitative research in explaining the mechanism, this paper chooses qualitative research methods to explore the causal mechanism of benchmark regression results. Based on the conclusion that “carbon emissions trading will aggravate air pollution,” this study further suggests the possible reasons and transmission paths behind it, including the system’s current problems, the challenges encountered in the implementation process, and the impact on the overall macro environment. The analysis is shown as Figure 4.
5.1 Inadequacy of the system itself
To begin with, in terms of the design of the carbon emission trading system, China’s system is roughly as follows, this study believes that there are many problems.
The coverage is not comprehensive. The carbon emission system was established to mitigate global warming and help green development, so controlling greenhouse gas emissions is the most important part of its work. Human activities or naturally occurring greenhouse gases are mainly composed of six gases, including carbon dioxide, methane, nitrous oxide, hydrofluorocarbons, perfluorocarbons, and sulfur hexafluoride. However, according to the data released by the research team of Huabao Securities in May 2021 on Carbon Emissions Trading Online, all pilot regions in China, except Chongqing, use carbon dioxide emissions as the only measure of carbon emissions. Besides, the proportion of greenhouse gases covered in Hubei is only 42%, far below the national average for the pilot regions, limiting the role that carbon emissions control can play.
The carbon allowance allocation method is flawed. In formulating carbon quotas for enterprises, the government uses the benchmarking method, historical intensity method, and historical method to allocate quotas according to the quality of different industries’ respective carbon emission data. Although these three methods are distinguished in specific arithmetic, their final numerical indicators are determined from historical data. For the benchmarking method, the development of technology and changes in the macroeconomic situation is likely to make the benchmark value lack the necessary binding effect, resulting in a tendency for the carbon trading market to be inherently quota lose. The historical method is riskier in causing discriminatory allocation among trading subjects. In the specific distribution method, China is currently dominated by the government’s free allocation, which is difficult to reflect the value of carbon emission rights as a scarce resource and is not conducive to forming a normal market price mechanism. It is worth noting that China has not adopted the internationally practiced capping policy on total emissions. Instead, it adopts the method of the first allocation and then adjustment, first allocating part of the allowances to enterprises and finally adjusting them according to the actual emissions. Although this form gives more flexibility to the policy, it also gives more room for enterprises to manipulate. This ultimately leads to an excessive and unfair issuance of allowances and makes it difficult for carbon emissions trading to be carried out effectively. And in communication with a staff member in charge of carbon emission affairs within Huadian Group, he also talked about it as follows.
Now the government issued at the beginning of the issuance of quotas is still relatively generous, so we actually do not need to purchase from other enterprises this carbon emission rights In particular, large enterprises with relatively standardized management have made a lot of technological transformation in all aspects of energy conservation and carbon reduction in the early stage, so on the whole, they are basically in a surplus state. However, private enterprises such as paper mills and chemical plants with their own power plants are also high energy consumption and high emissions, and their quotas are relatively small according to the calculation method in the accounting guide (Interview No. 20211012A).
The accuracy of the current verification method is yet to be tested. China’s carbon emission control regions, including Hubei, have adopted the emission factor method recommended by the United Nations Intergovernmental (IPCC) as the mainstream measurement method. However, since developed countries (e.g., the United States and the United Kingdom) started carbon emission control earlier, they have a full international voice in constructing carbon emission accounting method systems and databases. Therefore, the IPCC default values established on this basis have deviations from China’s national conditions, and it is difficult to estimate the real carbon emissions situation accurately. However, China has not yet established a unified set of carbon emission factor calculation standards, and the national and provincial energy consumption statistics differ greatly, creating obstacles for the carbon emission accounting work in China. In addition, the emission factor method is essentially a theoretical calculation method. Considering the variability and complexity of the actual operation, it is still debatable whether the theoretical values obtained can truly reflect the actual emission situation.
5.2 Challenges of the concrete implementation process
In the process of implementing this system, there are also various challenges.
First, there is a tendency of “local protectionism” in many places. That local government can use their autonomy to relax the total quota or give more quotas to enterprises with high GDP contributions to protecting local industries. The government may also directly prohibit local enterprises from granting emission targets to other regions, thus creating segregation of carbon markets in different regions and making it difficult to establish a national carbon emissions trading market. In addition, when it comes to compliance, local governments may not impose severe penalties on enterprises that fail to meet the carbon emission standards; or the penalties may be single and insufficient to serve as a warning to enterprises. The reasons for this phenomenon are, on the one hand, governments’ protection of local enterprises with a high output value. On the other hand, enterprises have to bargain or lobby with local governments in the face of high violation penalties, forcing governments to make certain compromises. Consequently, the lack of strict compliance leads to the carbon emissions trading system being a formality and unable to play a real binding role on enterprises.
Second, there is information asymmetry between enterprises and the government and between the central and local governments regarding carbon market policies, carbon emission data, emission boundaries, and production technology and output. On the one hand, the historical emission data of enterprises are not open to the public and society, and each pilot can only rely on the emission reports provided by enterprises on their initiative to determine the quotas. And although enterprises have the complete advantage of their information, most do not provide the complete and true data to the government and third-party institutions when they report for profit. At the same time, due to the lack of understanding of the internal production process and product structure of enterprises, it is difficult for outsiders to fully grasp the real emission situation of enterprises, thus resulting in unequal information between the two sides. This not only brings obstacles to the government in allocating carbon emission quotas but also risks to banks and other financial institutions in the market, reducing their enthusiasm for promoting green credit and financial services. It can be said that the accuracy of data is undoubtedly the basis for the carbon trading market to function. If a large amount of data is unreliable, emission reduction leads to a loss in its basic guarantee. On the other hand, the carbon emissions trading market currently suffers from inadequate information disclosure. The disclosure of emission control enterprises’ quota allocation, carbon asset trading, and total carbon emissions is neither standardized nor sufficient, the public data on the official website is very limited, and many specifics are unverifiable. The lack of market information further hinders investors’ decision-making, making it difficult for the carbon emissions trading system to produce practical effects.
At present, the price of carbon per ton is not very expensive. It is only about 30 or 40 yuan. At present, the volume of state-owned enterprises is relatively large, so some costs incurred in such transactions actually have no impact. But in the future, the impact may be greater because of the national goals of “carbon peak” in 2030 and “carbon neutralization” in 2060 (Interview No. 20211012A).
6 Conclusion
In this study, we use exogenous shocks provided by epidemic closure as a quasi-natural experiment for instrumental variables regression to explore the pros and cons of carbon emissions trading systems with a sample of eight cities in Hubei. We empirically obtain the conclusion that carbon emissions trading reduces air quality. In this study, we believe that there are the following reasons: first, China’s carbon financial market has just emerged, the trading system is still not mature enough and needs to be improved, and the market has factors such as unequal distribution of carbon quotas and uncertainty of trading, which lead to its failure to achieve the expected effect; several enterprises are worried about the risk; second, it is also possible that the volume of carbon emission rights trading and air quality shows a U-shaped relationship, and in the early stage of its development, the number of exchanges was small, and the trading volume was small, and the trading of carbon emission rights made enterprises that could not emit originally could buy more emission rights, and the limit of carbon emission was set high, which led to the price adjustment mechanism could not function well, and finally led to the further aggravation of environmental pollution.
This study has two major contributions. First, in terms of urban governance, we provide a new perspective for urban air pollution management, reflecting on the possible drawbacks of using market-based means for environmental governance. Then, in the green finance aspect, this study fills in the research on green finance represented by carbon finance in China and offers relevant experience for the perfect development of green finance. Although China is now in the process of improving the development of carbon finance systems, it is only restricted by the Interim Measures of Carbon Emission Trading Management to each pilot carbon emission trading market, but the formal supporting laws are not perfect, which leads to the low efficiency of the market in China. Our research provides recommendations and directions for the development of the future financial system.
This study has a few main limitations. First, in the empirical analysis, due to the high requirement of data availability at the city-degree level, this study selects several representative available data when choosing control variables by referring to existing literature, but there are still more omissions. At the same time, due to the data structure limitation, this study cannot make a rigorous mechanism investigation at present. Further, the main empirical innovation of this study lies in selecting instrumental variables, but a statistical test for the exogeneity of the instrumental variables in the aptly identified cases has not yet emerged. Although there are still many deficiencies, this study refers to the existing literature, attempts to add another instrumental variable, and conducts empirical tests, contributing to the carbon literature.
Data availability statement
Publicly available datasets were analyzed in this study. This data can be found here: https://www.aqistudy.cn/historydata/weixin.php; http://fgw.hubei.gov.cn/; http://www.stats.gov.cn/; http://tjj.hubei.gov.cn/; https://geomodeling.njnu.edu.cn/dataItem/hub/5f50a9d60e989758900e8730.
Ethics statement
Written informed consent was obtained from the individual(s) for the publication of any potentially identifiable images or data included in this article.
Author contributions
YJ contributed to the thesis conception, research design, and empirical writing. JY contributed to the literature review, data collection, and theoretical writing. RG contributed to the validation, formal analysis, investigation, resources, supervision, funding acquisition, and supervision. JC contributed to the interview research and mechanism writing.
Conflict of interest
The authors declare that the research was conducted in the absence of any commercial or financial relationships that could be construed as a potential conflict of interest.
Publisher’s note
All claims expressed in this article are solely those of the authors and do not necessarily represent those of their affiliated organizations, or those of the publisher, the editors and the reviewers. Any product that may be evaluated in this article, or claim that may be made by its manufacturer, is not guaranteed or endorsed by the publisher.
Footnotes
1Voluntary emission reductions (CCER) from emission reduction projects such as new energy can be used to offset up to 5% of their verified emissions (1 unit of CCER can offset 1 ton of CO2-equivalent emissions) or sold for additional revenue.
References
Appolloni, A., Chiappetta Jabbour, C. J., D’Adamo, I., Gastaldi, M., and Settembre-Blundo, D. (2022). Green recovery in the mature manufacturing industry: the role of the green-circular premium and sustainability certification in innovative efforts. Ecol. Econ. 193, 107311. doi:10.1016/j.ecolecon.2021.107311
Bai, C., and Satir, A. (2022). A critical content-analysis of sustainable supplier development literature and future research directions. J. Clean. Prod. 365, 132443. doi:10.1016/j.jclepro.2022.132443
Cao, J., Ho, M. S., Ma, R., and Teng, F. (2021). When carbon emission trading meets a regulated industry: evidence from the electricity sector of China. J. Public Econ. 200, 104470. doi:10.1016/j.jpubeco.2021.104470
Carrai, M. A. (2021). Adaptive governance along Chinese-financed BRI railroad megaprojects in East Africa. World Dev. 141, 105388. doi:10.1016/j.worlddev.2020.105388
Chen, Y. S. (2012). Logic, imagination, and interpretation: the application of instrumental variables to causal inference in social sciences. Sociol. Stud. 27, 192–216. doi:10.19934/j.cnki.shxyj.2012.06.009.x
Chen, Q., and Taylor, D. (2020). Economic development and pollution emissions in Singapore: Evidence in support of the Environmental Kuznets Curve hypothesis and its implications for regional sustainability. J. Clean. Prod. 243, 118637. doi:10.1016/j.jclepro.2019.118637
Chen, X., Li, Z., Gallagher, K. P., and Mauzerall, D. L. (2021). Financing carbon lock-in in developing countries: bilateral financing for power generation technologies from China, Japan, and the United States. Appl. Energy 300, 117318. doi:10.1016/j.apenergy.2021.117318
Chen, Z. Y., Xu, L., and Qian, C. X. (2020). Measurement and dynamic evolution of China's carbon finance development level. J. Quant. Technical Econ. 37, 62–82. doi:10.13653/j.cnki.jqte.2020.08.004
Cheng, S., Wang, P., Chen, B., and Fan, W. (2022). Decoupling and decomposition analysis of CO2 emissions from government spending in China. Energy 243, 122741. doi:10.1016/j.energy.2021.122741
Chu, M. Q., and Liu, Z. B. (2014). Equipment manufacturing scale, transaction costs and externalization of production services. J. Financ. Econ. 40, 108–118. doi:10.16538/j.cnki.jfe.2014.07.010
Cong, R., and Lo, A. Y. (2017). Emission trading and carbon market performance in Shenzhen, China. Appl. Energy 193, 414–425. doi:10.1016/j.apenergy.2017.02.037
Du, W., and Li, M. (2021). The impact of land resource mismatch and land marketization on pollution emissions of industrial enterprises in China. J. Environ. Manag. 299, 113565. doi:10.1016/j.jenvman.2021.113565
Fang, G., Gao, Z., Tian, L., and Fu, M. (2022). What drives urban carbon emission efficiency? spatial analysis based on nighttime light data. Appl. Energy 312, 118772. doi:10.1016/j.apenergy.2022.118772
Feng, H., Liu, Z., Wu, J., Iqbal, W., Ahmad, W., Marie, M., et al. (2022). Nexus between Government spending’s and Green Economic performance: role of green finance and structure effect. Environ. Technol. Innov. 27, 102461. doi:10.1016/j.eti.2022.102461
Gao, Y. L., Dong, J., Li, L., and Li, H. B. (2019). Study on the effectiveness and mechanism of carbon emission right trading policy: based on the perspective of inter-provincial difference in the construction land carbon emission intensity. Environ. Yangtze Basin. 28, 783–793. doi:10.11870/cjlyzyyhj201904005
García, J. H., and Torvanger, A. (2019). Carbon leakage from geological storage sites: implications for carbon trading. Energy Policy 127, 320–329. doi:10.1016/j.enpol.2018.11.015
Gu, G., Zheng, H., Tong, L., and Dai, Y. (2022). Does carbon financial market as an environmental regulation policy tool promote regional energy conservation and emission reduction? Empirical evidence from China. Energy Policy 163, 112826. doi:10.1016/j.enpol.2022.112826
Gunnarsdottir, I., Davidsdottir, B., Worrell, E., and Sigurgeirsdottir, S. (2021). Sustainable energy development: history of the concept and emerging themes. Renew. Sustain. Energy Rev. 141, 110770. doi:10.1016/j.rser.2021.110770
He, G. Y., and Wu, X. G. (2015). The inequality between marketization, economic development and gender income in Chinese cities. Sociol. Stud. 30, 140–165. doi:10.19934/j.cnki.shxyj.2015.01.007
Jijian, Z., Twum, A. K., Agyemang, A. O., Edziah, B. K., and Ayamba, E. C. (2021). Empirical study on the impact of international trade and foreign direct investment on carbon emission for belt and road countries. Energy Rep. 7, 7591–7600. doi:10.1016/j.egyr.2021.09.122
Kouznetsov, A., Kim, S., and Wright, C. (2019). An audit of received international business corruption literature for logic, consistency, completeness of coverage. J. Int. Manag. 25 (4), 100688. doi:10.1016/j.intman.2019.100688
Li, B., Wu, J., and Chen, S. X. (2015). Urbanization, industrialization, informatization and Chinese energy intensity. Chin. J. Popul. Resour. Environ. 25, 69–76. doi:10.3969/j.issn.1002-2104.2015.08.009
Li, C., Qi, Y., Liu, S., and Wang, X. (2022). Do carbon ETS pilots improve cities’ green total factor productivity? Evidence from a quasi-natural experiment in China. Energy Econ. 108, 105931. doi:10.1016/j.eneco.2022.105931
Li, G., Ruonan, L., Yingdan, M., and Xiaoli, Z. (2022). Improve technical efficiency of China’s coal-fired power enterprises: taking a coal-fired-withdrawl context. Energy 252, 123979. doi:10.1016/j.energy.2022.123979
Li, J., Liu, R., and Xie, Q. (2022). The price fluctuation in Chinese carbon emission trading market: new evidence from adaptive Fourier decomposition. Procedia Comput. Sci. 199, 1095–1102. doi:10.1016/j.procs.2022.01.139
Li, X., Hu, Z., Cao, J., and Xu, X. (2022). The impact of environmental accountability on air pollution: A public attention perspective. Energy Policy 161, 112733. doi:10.1016/j.enpol.2021.112733
Li, S. L., and Lin, P. N. (2020). Research on the effect of CHina’s carbon emission trading policy in perfecting and promoting regional pollution reduction: difference-in-differences analysis based on provincial panel data. J. Sun Yatsen Univ. 60, 182–194. doi:10.13471/j.cnki.jsysusse.2020.05.022
Li, S. L., Shan, B., and Shen, C. (2014). Local government competition, environmental regulation and regional ecological efficiency. J. World Econ. 37, 88–110.
Liu, T. (2020). Research on the impact of blockchain technology on carbon trading price mechanism. Price: Theory & Practice, 54–57. doi:10.19851/j.cnki.CN11-1010/F.2020.08.311
Li, Y., Liu, T., Song, Y., Li, Z., and Guo, X. (2021). Could carbon emission control firms achieve an effective financing in the carbon market? A case study of China’s emission trading scheme. J. Clean. Prod. 314, 128004. doi:10.1016/j.jclepro.2021.128004
Liu, J., Liu, Y., Wang, F., Cui, Y., and Han, L. (2021). Influence of free-market competition and policy intervention competition on enterprise green evolution. J. Clean. Prod. 325, 129225. doi:10.1016/j.jclepro.2021.129225
Ma, S., He, Y., and Gu, R. (2021). Joint service, pricing and advertising strategies with tourists’ green tourism experience in a tourism supply chain. J. Retail. Consumer Serv. 61, 102563. doi:10.1016/j.jretconser.2021.102563
McGrath, T., Blades, L., Early, J., and Harris, A. (2022). UK battery electric bus operation: Examining battery degradation, carbon emissions and cost. Transp. Res. Part D Transp. Environ. 109, 103373. doi:10.1016/j.trd.2022.103373
Nechifor, V., Basheer, M., Calzadilla, A., Obuobie, E., and Harou, J. J. (2022). Financing national scale energy projects in developing countries – an economy-wide evaluation of Ghana’s Bui Dam. Energy Econ. 111, 106065. doi:10.1016/j.eneco.2022.106065
Norbäck, D., Lu, C., Zhang, Y., Li, B., Zhao, Z., Huang, C., et al. (2019). Sources of indoor particulate matter (PM) and outdoor air pollution in China in relation to asthma, wheeze, rhinitis and eczema among pre-school children: synergistic effects between antibiotics use and PM10 and second hand smoke. Environ. Int. 125, 252–260. doi:10.1016/j.envint.2019.01.036
Peng, H.-R., Cui, J., and Zhang, X. (2022). Does China emission trading scheme reduce marginal abatement cost? A perspective of allowance allocation alternatives. Sustain. Prod. Consum. 32, 690–699. doi:10.1016/j.spc.2022.05.021
Peng, H., Qi, S., and Cui, J. (2021a). The environmental and economic effects of the carbon emissions trading scheme in China: the role of alternative allowance allocation. Sustain. Prod. Consum. 28, 105–115. doi:10.1016/j.spc.2021.03.031
Peng, H., Shen, N., Ying, H., and Wang, Q. (2021b). Can environmental regulation directly promote green innovation behavior? based on situation of industrial agglomeration. J. Clean. Prod. 314, 128044. doi:10.1016/j.jclepro.2021.128044
Polzin, F., and Sanders, M. (2020). How to finance the transition to low-carbon energy in Europe? Energy Policy 147, 111863. doi:10.1016/j.enpol.2020.111863
Powdthavee, N., and Oswald, A. J. (2020). Is there a link between air pollution and impaired memory? Evidence on 34, 000 English citizens. Ecol. Econ. 169, 106485. doi:10.1016/j.ecolecon.2019.106485
Ruan, C. H. (2020). China’s carbon finance development: whether it depends on economy or environment?: based on structural equation model. Journal of Beijing College of Finance and Commerce 36, 24–30.
Sahin, H., and Esen, H. (2022). The usage of renewable energy sources and its effects on GHG emission intensity of electricity generation in Turkey. Renew. Energy 192, 859–869. doi:10.1016/j.renene.2022.03.141
Shi, Y., Wei, Z., Shahbaz, M., and Zeng, Y. (2021). Exploring the dynamics of low-carbon technology diffusion among enterprises: an evolutionary game model on a two-level heterogeneous social network. Energy Econ. 101, 105399. doi:10.1016/j.eneco.2021.105399
Sikder, M., Wang, C., Yao, X., Huai, X., Wu, L., KwameYeboah, F., et al. (2022). The integrated impact of GDP growth, industrialization, energy use, and urbanization on CO2 emissions in developing countries: Evidence from the panel ARDL approach. Sci. Total Environ. 837, 155795. doi:10.1016/j.scitotenv.2022.155795
Song, Y., Liang, D., Liu, T., and Song, X. (2018). How China’s current carbon trading policy affects carbon price? An investigation of the shanghai emission trading scheme pilot. J. Clean. Prod. 181, 374–384. doi:10.1016/j.jclepro.2018.01.102
Sovacool, B. K., Hess, D. J., and Cantoni, R. (2021). Energy transitions from the cradle to the grave: a meta-theoretical framework integrating responsible innovation, social practices, and energy justice. Energy Res. Soc. Sci. 75, 102027. doi:10.1016/j.erss.2021.102027
Sun, Y., Li, Y., Yu, T., Zhang, X., Liu, L., Zhang, P., et al. (2021). Resource extraction, environmental pollution and economic development: evidence from prefecture-level cities in China. Resour. Policy 74, 102330. doi:10.1016/j.resourpol.2021.102330
Tang, P., Jiang, Q., and Mi, L. (2021). One-vote veto: the threshold effect of environmental pollution in China’s economic promotion tournament. Ecol. Econ. 185, 107069. doi:10.1016/j.ecolecon.2021.107069
Tang, W., Li, H., and Chen, J. (2021). Optimizing carbon taxation target and level: enterprises, consumers, or both? J. Clean. Prod. 282, 124515. doi:10.1016/j.jclepro.2020.124515
Tu, Y., and Wu, W. (2021). How does green innovation improve enterprises’ competitive advantage? The role of organizational learning. Sustain. Prod. Consum. 26, 504–516. doi:10.1016/j.spc.2020.12.031
Vickers, E. (2009). Selling ‘socialism with Chinese characteristics’ ‘thought and politics’ and the legitimisation of China’s developmental strategy. Int. J. Educ. Dev. 29 (5), 523–531. doi:10.1016/j.ijedudev.2009.04.012
Wang, C., Wang, Z., Ke, R.-Y., and Wang, J. (2018). Integrated impact of the carbon quota constraints on enterprises within supply chain: direct cost and indirect cost. Renew. Sustain. Energy Rev. 92, 774–783. doi:10.1016/j.rser.2018.04.104
Wang, W., and Zhang, Y.-J. (2022). Does China’s carbon emissions trading scheme affect the market power of high-carbon enterprises? Energy Econ. 108, 105906. doi:10.1016/j.eneco.2022.105906
Wang, X.-Q., Su, C.-W., Lobonţ, O.-R., Li, H., and Nicoleta-Claudia, M. (2022). Is China’s carbon trading market efficient? Evidence from emissions trading scheme pilots. Energy 245, 123240. doi:10.1016/j.energy.2022.123240
Wu, Q., and Wang, Y. (2022). How does carbon emission price stimulate enterprises’ total factor productivity? Insights from China’s emission trading scheme pilots. Energy Econ. 109, 105990. doi:10.1016/j.eneco.2022.105990
Wu, Y., Zong, T., Shuai, C., Liao, S., Jiao, L., Shen, L., et al. (2022). Does resource environment carrying capacity have a coercive effect on urbanization quality? Evidence from the Yangtze river economic belt, China. J. Clean. Prod. 365, 132612. doi:10.1016/j.jclepro.2022.132612
Xu, C., Yiwen, Z., Cheng, B., Li, L., and Zhang, M. (2020). Study on environmental Kuznets curve for noise pollution: a case of 111 Chinese cities. Sustain. Cities Soc. 63, 102493. doi:10.1016/j.scs.2020.102493
Xu, S.-C., Miao, Y.-M., Gao, C., Long, R.-Y., Chen, H., Zhao, B., et al. (2019). Regional differences in impacts of economic growth and urbanization on air pollutants in China based on provincial panel estimation. J. Clean. Prod. 208, 340–352. doi:10.1016/j.jclepro.2018.10.114
Yao, X., Zhang, X., and Guo, Z. (2020). The tug of war between local government and enterprises in reducing China’s carbon dioxide emissions intensity. Sci. Total Environ. 710, 136140. doi:10.1016/j.scitotenv.2019.136140
Yu, P., Cai, Z., and Sun, Y. (2021). Does the emissions trading system in developing countries accelerate carbon leakage through OFDI? Evidence from China. Energy Econ. 101, 105397. doi:10.1016/j.eneco.2021.105397
Zhang, G., Jia, Y., Su, B., and Xiu, J. (2021). Environmental regulation, economic development and air pollution in the cities of China: spatial econometric analysis based on policy scoring and satellite data. J. Clean. Prod. 328, 129496. doi:10.1016/j.jclepro.2021.129496
Zhang, M., Lian, Y., Zhao, H., and Xia-Bauer, C. (2020). Unlocking green financing for building energy retrofit: a survey in the Western China. Energy Strategy Rev. 30, 100520. doi:10.1016/j.esr.2020.100520
Zhang, Y.-J., and Wang, W. (2021). How does China’s carbon emissions trading (CET) policy affect the investment of CET-covered enterprises? Energy Econ. 98, 105224. doi:10.1016/j.eneco.2021.105224
Zhu, P. F., Zhang, Z. Y., and Jiang, G. L. (2011). Empirical study of the relationship between FDI and environmental regulation: an intergovernmental competition perspective. Econ. Res. J. 46, 133–145.
Keywords: carbon emissions trading, green finance, carbon neutrality, instrumental variable regression, mixed method research
Citation: Jiang Y, Yao J, Gao R and Chen J (2022) Why the “journey” of carbon neutrality is a long one—a mixed method research based on carbon emission trading in hubei province. Front. Environ. Sci. 10:984434. doi: 10.3389/fenvs.2022.984434
Received: 04 July 2022; Accepted: 11 July 2022;
Published: 30 August 2022.
Edited by:
Xuefeng Shao, University of Newcastle, AustraliaCopyright © 2022 Jiang, Yao, Gao and Chen. This is an open-access article distributed under the terms of the Creative Commons Attribution License (CC BY). The use, distribution or reproduction in other forums is permitted, provided the original author(s) and the copyright owner(s) are credited and that the original publication in this journal is cited, in accordance with accepted academic practice. No use, distribution or reproduction is permitted which does not comply with these terms.
*Correspondence: Jiayi Yao, YmFpbHlqbzMyeUAxNjMuY29t; Ruosu Gao, cmdhbzM5MjBAdW5pLnN5ZG5leS5lZHUuYXUmI3gwMjAwYTs=