- 1School of Economics, Tianjin University of Commerce, Tianjin, China
- 2School of Management, National Defense University, Beijing, China
The implementation of the low-carbon city pilot policy is an important measure to reduce carbon emissions and promote low-carbon economic development in China. However, the resulting fiscal pressure may be counterproductive. The aim of this paper is to investigate the impacts of the low-carbon city pilot policy and fiscal pressure on carbon productivity. Based on the data of 282 cities in China over the period 2005 to 2017, this paper uses the staggered difference-in-differences (DID) model to identify the causal relationship among the low-carbon city pilot policy, fiscal pressure, and carbon productivity. The results show that this pilot policy can significantly improve carbon productivity and that the improvement effect presents a dynamic and persistent feature. However, the fiscal pressure resulting from this pilot policy can reduce carbon productivity, and the degree of reduction depends on the status of fiscal pressure. Increased fiscal pressure has a negative impact on carbon productivity, which is heterogeneous with different levels of economic development. Moreover, the mediation effect analysis finds that this pilot policy affects carbon productivity by adjusting the energy production and consumption structure, enhancing green technology innovation capabilities, and increasing the number of low-carbon-type enterprises entering the market. This paper provides new ideas for improving carbon productivity without increasing fiscal pressure. It also recommends that fiscal pressure cannot be ignored in the implementation of the low-carbon city pilot policy.
1 Introduction
In recent years, extreme weather conditions related to global warming have frequently occurred, highlighting the challenges to high-quality economic development. Therefore, effective strategies to improve carbon productivity and reduce carbon emissions have elicited increasing attention. The goal of the low-carbon policy is to promote carbon productivity and enhance the development of a low-carbon economy. However, Tao and Li (2021) found that China’s low-carbon city pilot policy had a negatively impact on economic growth and fiscal revenue, thus aggravating the fiscal pressure on local governments. To alleviate fiscal pressure, local governments may relax environmental regulations to induce more pollution-intensive enterprises, thereby increasing the degree of industrial pollution (Wang and Zhang, 2017; Bai et al., 2019; Huang and Zhou, 2020). This raises the question of whether the fiscal pressure brought about by the low-carbon city pilot policy reduces carbon productivity. Under the condition of controlling for the impact of fiscal pressure brought about by this pilot policy, can the low-carbon city pilot policy still improve carbon productivity?Clarifying the above problems will not only unveil the internal relationship between the low-carbon city pilot policy and carbon productivity but also provide policy implications for further improving the latter.
The low-carbon city pilot policy is an important way to significantly reduce carbon emissions (Huo et al., 2022; Liu et al., 2022; Ren et al., 2022), and it has positive spillover effects (Liu, 2022). Most of the literatures have focused on the ways in which the low-carbon policy affects carbon emissions. First, the low-carbon city pilot policy can promote urban green total factor productivity (Cheng et al., 2019; Qiu et al., 2021). Second, the low-carbon city pilot policy can promote the green technology innovations of enterprises, and its green innovation effect is reflected in patent applications for energy conservation and alternative energy production (Chen et al., 2022; Pan et al., 2022). An increase in the use of clean energy has been shown to reduce coal-fired power generation and carbon emissions (Fell and Kaffine, 2018; Huo et al., 2022; Zhou et al., 2022). Third, carbon tax increases the cost of carbon consumption and significantly reduces carbon emissions (Nordhaus, 2006; Andersson, 2019). Moreover, carbon tax and research and development (R&D) subsidies can encourage companies to innovate clean technologies, thereby reducing pollutant emissions and promoting output growth (Acemoglu et al., 2016; Aghion et al., 2016). Fourth, the carbon emission trading system is also conducive to low-carbon technology innovation and carbon emission reduction (Calel and Dechezleprêtre, 2016; Li et al., 2022).
However, in the implementation of the low-carbon city pilot policy, local governments may directly shutdown high-energy-consuming enterprises, which can lead to reductions in the tax base and tax revenue. In turn, this can increase the fiscal pressure on local governments. On the one hand, to alleviate fiscal pressure, local governments can increase the number of industrial enterprises and relax environmental regulations to promote the proliferation of more pollution-intensive enterprises, thus increasing the degree of industrial pollution (Wang and Zhang, 2017; Huang and Zhou, 2020). On the other hand, local governments can increase fiscal revenues through non-tax methods, which will increase the actual non-tax burden of enterprises (Peng et al., 2020; Zhao and Fan, 2020). In both cases, regardless of which way is chosen to alleviate fiscal pressure, it may have a negative impact on enhancing carbon productivity.
In general, the above literature contribute to the impacts and mechanisms of the low-carbon city pilot policy on carbon emissions, as well as the rise in fiscal pressure, leading to the relaxation of low-carbon environmental regulations by local governments. However, there are still some shortcomings. First, the relationship among the low-carbon city pilot policy, fiscal pressure, and carbon productivity has not been established. In fact, the low-carbon city pilot policy may lead to increased fiscal pressure that will prompt the local government loosening its environmental regulations, which may increase carbon emissions and reduce carbon productivity. Second, the existing literature does not consider the impact of fiscal pressure brought about by the low-carbon city pilot policy. This not only not only has the problem of biased estimation results caused by the omission of variables, but also fails to fully understand the role of the low-carbon city pilot policy. Only perceiving the positive aspects of the pilot policy will not be conducive to providing effective strategies for solving fiscal pressure problems. It will have a perverse effect on reducing carbon emissions and improving carbon productivity.
The aims of this paper are twofold. First, it investigates whether the low-carbon city pilot policy and fiscal pressure brought about by the low-carbon city pilot policy affect carbon productivity. This will not only establish a full understanding of the impact of the low-carbon city pilot policy but also resloves the problem of estimation bias caused by the omission of variables to avoid only seeing the positive impacts of the pilot policy. Second, this study deconstructs the mediation mechanism of the low-carbon city pilot policy on carbon productivity and discusses the heterogeneity of the impact of the pilot policy between different regions and economic development levels, which will enhance the understanding of the impacts of the low-carbon city pilot policy.
Based on the background of the low-carbon city pilot policy and the proposed hypothesis, this paper obtains data from 282 cities in China over the period 2005–2017 and then uses the staggered difference-in-differences (DID) model to identify the relationships among the low-carbon city pilot policy, fiscal pressure, and carbon productivity. The results are as follows:1) the low-carbon city pilot policy can significantly improve carbon productivity, and the impact is persistent; 2) the fiscal pressure resulting from the low-carbon city pilot policy will reduce carbon productivity, and the degree of decline depends on the status of fiscal pressure; (3)increased fiscal pressure will reduce carbon productivity, and the degree of reduction is heterogeneous with different levels of economic development; and 4) the mediation effect analysis shows that the low-carbon city pilot policy affects carbon productivity by adjusting the structure of energy production and consumption, enhancing the ability for green technology innovation and increasing the number of low-carbon-type enterprises entering the market. In this sense, this paper delivers new ideas and supplements the existing literature on the impacts of such a pilot policy.
Compared to the existing literature, the marginal contributions of this paper are as follows. First, most existing studies focus on whether the low-carbon city pilot policy can reduce carbon emission and the negative relationship between this pilot policy and economic growth. This paper incorporates fiscal pressure into the analysis of the impacts of such a pilot policy on carbon productivity, which not only provides an explanation for the negative relationship between the pilot policy and economic growth (Tao and Li, 2021) but also solves the estimation bias problem caused by the omission of variables. In addition, without controlling for the impact of fiscal pressure brought about by this pilot policy, the size of the impact of the low-carbon city pilot policy is similar to that reported by Huo et al. (2022) and Liu (2022). However, after controlling for the impact of the fiscal pressure brought about by the pilot policy, the impact of the low-carbon city pilot policy is greater, which is significantly different from that of Huo et al. (2022) and Liu (2022). Meanwhile, the impact of the fiscal pressure brought about by this pilot policy is the opposite; the ultimate impact of the low-carbon city pilot policy also depends on the status of fiscal pressure. Second, studies on the effects of the low-carbon city pilot policy usually use technological innovation and energy structure as mediation variables to analyze the mediation effect (Huo et al., 2022; Liu et al., 2022). Apart from these, this paper adds the registration number of low-carbon-type enterprises and fiscal pressure brought about by this pilot policy as additional mediation variables, thus expanding the literature on the impact path of the low-carbon city pilot policy on carbon productivity. Third, in terms of policy implications, this paper evidences that the fiscal pressure brought about by the implementation of the low-carbon city pilot policy will reduce carbon productivity. However, if the implementation of this policy can raise the low-carbon access standards for new enterprises, optimize the low-carbon technology level of existing enterprises, and adjust the energy structure, this policy will not increase the fiscal pressure. On the contrary, it will help improve carbon productivity and resolve fiscal pressure.
The remainder of this paper is organized as follows. Section 2 presents the background of the low-carbon city pilot policy and proposes the theoretical hypotheses. Section 3 describes the empirical model and data. Section 4 delivers the results of the estimation and robustness tests. Section 5 discusses the impacts of heterogeneity and the mediation effects. Finally, Section 6 provides the conclusions and policy implications.
2 Background of the low-carbon city pilot policy and theoretical hypothesis
2.1 Background of the low-carbon city pilot policy
China is the largest manufacturing country globally; hence, it is considered “the world’s factory.” However, the rapid development of the manufacturing industry has been inevitably accompanied by massive energy consumption, and many industries that use traditional fossil fuel energy have emitted large amounts of carbon dioxide (CO2). To reduce carbon emissions, the Chinese government has actively adopted measures and committed to the realization of global carbon emission reduction. At the 15th Session of the Conference of the Parties to the United Nations Framework Convention on Climate Change held in Copenhagen, Denmark in 2009, the Chinese government pledged that by 2020, the country’s carbon dioxide emissions per unit of gross domestic product (GDP) would be reduced by 40–45% compared to 2005 figures, Such an effort represents a huge contribution to emission reduction efforts. In particular, Chairman Xi Jinping proposed that China would strive to achieve a carbon peak by 2030 and carbon neutrality by 2060 during the general debate held at the 75th United Nations General Assembly in September 2020, thus representing China’s action plan for achieving its carbon emission reduction targets.
After the Chinese State Council proposed the goal of controlling greenhouse gas (GHG) emissions in November 2009, the National Development and Reform Commission (NDRC) announced its plan to reduce carbon emissions by issuing the “Notice on the Implementation of thePilot Policy for Low-Carbon Provinces, Regions, and Low-carbon Cities” (Development and Reform Climate [2010] No. 1587) in July 2010. This policy requires low-carbon pilot cities to formulate their own action goals, key tasks, and response measures to control GHG emissions, advocate low-carbon production and consumption, and formulate supporting policies conducive to achieving low-carbon and green development. This policy also aimed to establish an industrial system characterized by low carbon emissions to reduce the intensity of carbon emissions and promote carbon productivity. The first batch of selected low-carbon cities consisted of eight cities, including Tianjin and Chongqing, which were further expanded in 2012. In april 2012, the Climate Department of the NDRC issued the “Notice on Organizing the Recommendation and Declaring the Second Batch of low-carbon Pilot Provinces and Cities” (Development and Reform Climate [2012] No. 3760). This batch comprised 28 cities, including Beijing and Shanghai. After that, the NDRC issued the “Notice on Carrying Out the Third Batch of National Low-carbon City Pilot Policy Work” (Development and Reform Climate [2017] No. 66) in January 2017, this time including 45 cities (e.g., Wuhai City) in the third batch of selected areas.
Thus far, a total of 81 cities have been selected as low-carbon city pilot policy areas. In terms of geographical distribution, these areas include cities in the Eastern, Central, and Western regions, such as cities in the south and north of the Qinling Mountains and the Huai River. From the perspective of location, the selected areas include provincial capital cities, municipalities directly under the Central Government, and general prefecture-level cities. With respect to economic development level, the list comprises cities in both economically developed and underdeveloped areas in the Central and Western regions. Generally, the selected areas for the low-carbon city pilot thoroughly represent a broad spectrum of Chinese cities with varying characteristics.
2.2 Theoretical hypothesis
The low-carbon city pilot policy affects carbon productivity in several ways. First, traditional fossil energy sources, such as raw coal and coke, have high CO2 emissions. Low-carbon cities reduce the proportion of traditional fossil energy use, increase the use of clean energy (e.g., hydropower, wind power, and solar energy), and reduce carbon emission by adjusting their respective energy structures (Liu et al., 2022). Second, the low-carbon city pilot policy requires the acceleration of low-carbon technology demonstration, promotion, and application. In terms of policy, carbon tax and R&D subsidies can encourage companies to invest in cleaner production and technological innovations, reduce carbon emissions, increase carbon productivity, and promote low-carbon economy growth (Acemoglu et al., 2016; Aghion et al., 2016). In other words, such a policy can promote the progress of low-carbon technology and increase the number of green patent applications, thus improving carbon productivity. Third, different from Acemoglu et al.(2016), Huo et al.(2022) and Liu et al. (2022) argued that to reduce carbon emissions by adjusting the energy structure and promoting low-carbon technology innovation, if the local government improves the low-carbon technology access standards of enterprises and expands the number of low-carbon-type enterprises entering the market, it can reduce the overall carbon emission intensity and improve carbon productivity. Accordingly, the first hypothesis is proposed:
Hypothesis 1. The low-carbon city pilot policy can improve carbon productivity, and the mediation effect of this pilot policy operates by adjusting the energy structure, increasing the number of low-carbon-type enterprises entering the market, and enhancing green technology innovation.To alleviate fiscal pressure, local governments may adopt two measures. First, they could increase the number of high-energy-consuming industrial enterprises and relax environmental regulations, thus increasing the number of pollution-intensive enterprises (Wang and Zhang, 2017; Huang and Zhou, 2020). Although this will increase fiscal revenue and alleviate fiscal pressure, it will also increase carbon emissions and lead to a decline in carbon productivity. Second, they could alleviate fiscal pressure by increasing the actual non-tax burden of enterprises (Peng et al., 2020; Zhao and Fan, 2020). Such an increase leads to a concomitant increase in policy uncertainty and operating costs for enterprises. Excessive non-tax burdens lead to insufficient funds for low-carbon technological innovation. At the same time, enterprises may cease operations due to excessive non-tax burdens, which is seriously detrimental to carbon emission reduction and carbon productivity improvement. In addition, strengthening tax collection and management and increasing the non-tax burden will have a distorting effect on investment in capital factors and reduce the efficiency of capital allocation (Huang and Deng, 2020), which are also not conducive to improving carbon productivity. Unlike Huang and Zhou (2020), Zhao and Fan (2021), and other studies, we link fiscal pressure to carbon productivity, recognizing the role of fiscal pressure. Accordingly, the second hypothesis is proposed:
Hypothesis 2. An increase in fiscal pressure leads to the decline of carbon productivity.During the implementation of the low-carbon city pilot policy, some enterprises with high energy and resource consumption may be shut down, thereby reducing government revenues and increasing fiscal pressure. To alleviate the fiscal pressure, local governments either increase the intensity of tax collection and management(Huang and Zhou, 2020) or increase non-tax collection efforts(Peng et al., 2020; Zhao and Fan, 2020), or both, which will increase the actual burden on enterprises. As a result, enterprises do not have enough funds for the research and development and use of low-carbon clean technologies, which will not help reduce carbon emissions and improve carbon productivity. When the tax and non-tax burdens of enterprises have reached high levels, and there is no way to further increase the intensity of tax and non-tax collection, local governments have to relax environmental regulations again and increase the entry of high-energy-consuming enterprises, resulting in increased carbon emissions and lower carbon productivity.By contrast, during the implementation of the low-carbon city pilot policy, if the local government provides policy support for the low-carbon clean technology transformation of stock enterprises (note: high-polluting enterprises must be closed), such action can stabilize the tax base and improve carbon productivity. In addition, the low-carbon technology access standards for new enterprises should be improved to enable them to meet the requirements of low-carbon development, thereby expanding the tax base and reducing the intensity of carbon emissions. The above measures will not increase fiscal pressure but will alleviate fiscal pressure and improve carbon productivity. Accordingly, the third hypothesis is proposed:
Hypothesis 3. The fiscal pressure brought about by the low-carbon city pilot policy will hinder the improvement of carbon productivity. However, if the local government increases the policy support for the optimization of low-carbon technologies of existing enterprises and raises the low-carbon access standards for new enterprises, such a pilot policy will not lead to increased fiscal pressure. Moreover, carbon productivity will be reduced.
3 Methodology
3.1 Empirical model
This paper examines the impacts of the low-carbon city pilot policy in 2010 and 2012. Referring to the methods of Angrist and Pischke (2008), Callaway and Sant’Anna (2021), and Baker et al. (2022), the staggered DID model is used for estimation. The benchmark model is set as follows:
where
where
To test whether the staggered DID model satisfies the assumption of parallel trends and to examine the dynamic effects of the low-carbon city pilot policy over time, this paper draws on the methods of Jacobson et al. (1993), Freyaldenhoven et al. (2019), and Sun and Abraham (2021) and adopts the event analysis method for estimation. The model is set as follows:
where
3.2 Variables
3.2.1 Dependent variable
This paper refers to the method of Shao et al. (2014) and Hu and Liu (2016) to measure carbon productivity as follows:
where
3.2.2 Independent variables
According to the basic steps of the staggered DID model, two dummy variables related to the low-carbon city pilot policy are constructed. The first one consists of the group dummy variable of the low-carbon city pilot policy (
The low-carbon city pilot policy(
Fiscal pressure (
The fiscal pressure brought about by the low-carbon city pilot policy(
3.2.3 Control variables
With reference to the research of Liu (2022), Huo et al. (2022), and Ren et al. (2022). The selected control variables are as follows: investment growth rate (
3.3 Data
Fixed asset investment, population growth rate, GDP, general budget expenditure, human capital, and trade import and export data were sourced from the China Statistics for Regional Economy Database and China City Statistics Database of the EPS DATA platform. CO2 emission data came from the China Carbon Accounting Database (CEADS), while the tax revenue data were sourced from the China Statistics for Regional Economy Database of the EPS DATA platform and China Economic Database of the CEIC data platform. For these different sources of data, we matched by city and year to obtain the required data.
After obtaining the data, we further processed them according to the following principles: 1) For any city with missing GDP data for 5 years or more, we deleted the sample data of the city. 2) Next, we deleted city samples whose numbers of corresponding years in the city code were less than 14 (i.e., the data whose statistical year was less than 14 years). 3) We used the three-period moving average method to make up for missing data. 4) After filling in the missing data, if the sample number of all indicators in any city was less than 13, the sample data of that city was deleted. 5) Except for the dummy variables of the low-carbon city pilot policy, other variables were winsorized at the level of 5%. 6) Given that Jiyuan City is a county-level city and the Daxing’an Mountains region has missing data, the samples from these two regions were deleted, leaving a total of 34 low-carbon pilot cities. In this way, we obtained the sample data for 282 cities in China over the period 2005–2017. The variables and their descriptive statistical characteristics are shown in Table 1.
Subsequently, we divided the whole sample of carbon productivity into two sample groups: the low-carbon city group and the non-low-carbon city group. Then, we calculated the average value of the carbon productivity in each group every year. Finally, we plotted them. The trend chart of carbon productivity is displayed in Figure 1. In addition, we plotted a scatter diagram to present the relationship between fiscal pressure and carbon productivity, as shown in Figure 2.
Figure 1 indicates that before 2012, carbon productivity met the parallel assumption in both low-carbon and non-low-carbon cities. However, since 2012, the changes in the carbon productivity of the low-carbon cities have become significantly higher than those of non-low-carbon cities. These results initially reflect that the low-carbon city pilot policy strongly promotes carbon productivity. The results in Figure 2 demonstrate that a negative relationship between fiscal pressure and carbon productivity, that is, the greater the fiscal pressure, the lower the carbon productivity.
4 Empirical result analysis and robustness test
4.1 Parallel trend and heterogeneous treatment effect test
When using the staggered DID model to estimate the impacts of the low-carbon city pilot policy and fiscal pressure on carbon productivity, one necessary condition is that the control and treatment groups must meet the parallel trend assumption. If there is a difference in the time trend of carbon productivity between low-carbon and non-low-carbon cities before the launch of the low-carbon city pilot policy, it can be inferred that the difference is not caused by this pilot policy. In addition, although the staggered DID model estimates the average treatment effect of the low-carbon city pilot policy, the impacts of this policy in different years are not shown. Therefore, to test whether the staggered DID model is a suitable method, we used the event analysis method to test the parallel trends and dynamic effects of the low-carbon city pilot policy. This specific method involves the following procedure: first, generate the interaction term of a low-carbon city pilot policy group dummy variable and the year dummy variable, then estimate the coefficient of the interaction term to obtain the dynamic effect of the low-carbon city pilot policy. The results are shown in Figure 3.
Figure 3 plots the estimated results of the coefficients of the interaction term between the low-carbon city pilot policy group dummy variable and the year dummy variable under a 95% confidence interval (CI). Evidently, the coefficients of the interaction term are all around 0 in the years before the start of the low-carbon city pilot policy, suggesting that before the pilot policy was started, there was no significant difference between the treatment and control groups. Hence, the conditions for the assumption of parallel trends are established. After the launch of the pilot policy, the coefficient is positive and increases significantly, showing a stable trend. At this point, the estimated coefficients of the control and treatment groups are significantly different, which indicates that the implementation of the low-carbon city pilot policy significantly improves the carbon productivity of low-carbon cities.
The two-way fixed effects (FE) regression of the staggered DID model is a popular method to evaluate treatment effects. However, if the error term is not mean zero conditional on group and period, this can lead to the problem of heterogenous treatment effects (Gardner, 2021). de Chaisemartin and D’Haultfoeuille (2020) demonstrated that in several DID models, the two-way FE estimator is a weighted sum of the treatment effect in each group and period. If the average treatment effects (ATEs) are heterogeneous across groups or periods, it will result in the question of negative weights. The two-way FE estimators of the staggered DID model do not identify the typical effect of the treatment. To test whether heterogeneous treatment effects exist, de Chaisemartin and D’Haultfoeuille (2020) recommended computing the weights attached to the two-way FE regression of the staggered DID model and calculating the ratio of the coefficient of variable and the standard deviation of the weights. If many weights are negative and if the ratio is small, we should use the new method to estimate the staggered DID model. Thus, referring to the method of de Chaisemartin and D’Haultfoeuille (2020), we computed the weight of the treated group and periods. The results are shown in Table 2.
In Table 2, the results indicate that under the condition of the estimation of the two-way FE of the staggered DID model, there are 220 positive weights and 0 negative weights. Moreover, the standard deviation of the treatment effect across the low-carbon cities and years is 0.879, which is greater than 0. Thus, we can use the two-way FE regression of the staggered DID model to estimate the treatment effect of the low-carbon city pilot policy.
4.2 Baseline DID model estimation
As a major policy measure to promote carbon productivity, the low-carbon city pilot policy advocates low-carbon production and consumption, which can undoubtedly reduce CO2 emissions and improve the quality of economic development. Therefore, the implementation of this pilot policy provides a quasi-natural experiment for this study. We used a staggered DID model to estimate the impacts of the policy and fiscal pressure on carbon productivity. The results are provided in Table 3.
In Table 3, Columns (1)–3) show the results of the staggered DID estimation under the conditions of controlling for only the year FE, only the city FE, and both types of FE, respectively. Columns (4)–6) present the effects of one period lag of the low-carbon city pilot policy. The results indicate that the coefficients pass the significance test after controlling for both the city and year FE, regardless of whether it is the current period or one lag period.
Specifically, Column 3) shows that when the city and year FE are controlled simultaneously, the coefficient of
4.3 Robustness test
To verify whether the relationship among the low-carbon city pilot policy, fiscal pressure, and carbon productivity is robust, we test the robustness of the conclusions by taking a series of measures, including index substitution, the Tobit-DID model of data merging processing, and counterfactual testing methods.
4.3.1 Robustness analysis after carbon productivity Is substituted by lnCO2
A similar indicator to carbon productivity is carbon emissions. We use the logarithm of the total carbon emissions (lnCO2) as an alternative indicator of carbon productivity and then estimate the model. In doing so, it is also helpful to compare the connections and differences between this paper and the study by Huo et al. (2022) and Liu et al. (2022) and explore whether the fiscal pressure brought about by the low-carbon city pilot policy is a factor that cannot be ignored. The estimated results are provided in Table 4.
Columns (3)–4) in Table 4 indicate that, without controlling for the effects of the interaction term of the low-carbon city pilot policy and fiscal pressure(
At the same time, compared to those that do not control for the influence of the interaction term of the low-carbon city pilot policy and fiscal pressure(
4.3.2 Robustness analysis after substituted by secondary industry and industrial carbon productivity
The low-carbon city pilot policy advocates low-carbon production and consumption. Secondary industry, especially the industrial sector, is the main source of CO2 emissions. This means that if the secondary industry and the industrial sector carbon productivity in a low-carbon city are significantly improved, then the pilot policy will be able to promote carbon productivity; otherwise, the pilot policy is invalid. We used the ratio of secondary industry output value (unit: yuan) and industrial output value (unit: yuan) to CO2 emissions(unit: kilogram) to measure the secondary industry and industrial sector carbon productivity. Subsequently, the GDP carbon productivity in the staggered DID model in Eq. 2 was substituted by the secondary industry carbon productivity and industrial sector carbon productivity. After controlling for the city FE and the year FE, we estimated the impacts of the low-carbon city pilot policy and fiscal pressure on carbon productivity. To examine the continuous effects of the low-carbon city pilot policy, we estimated the impact of the one period lag of interaction term(
Table 5 presents the estimated results after replacements with secondary industry carbon productivity. In Columns 1) and (2), the coefficient of
In Column (3), after substituting carbon productivity with industrial sector, the coefficient of
4.3.3 Censored data and tobit-DID estimation
To deal with the possible adverse effects of variable singular values, we used the winsorize method to deal with all variables at the 5% level, which resulted in the problem of censored data. Although we observed the data for all samples, data with variable values below the 5% level were censored to the 5% level, and those with values above the 95% level were censored to the 95% level. However, the potential issue with censored data is that the estimates of the staggered DID model with two-way FE are inconsistent. To solve this problem, the method of censored regression (i.e., Tobit model estimation) was adopted. Therefore, we used the panel Tobit-DID model to estimate the impacts of the low-carbon city pilot policy and fiscal pressure on carbon productivity. The estimation results are provided in Table 6.
In Table 6, Columns 1) and 2) indicate that, without controlling for the influence of other variables, the coefficients of both current period
4.3.4 Counterfactual testing
We tested the relationship among the low-carbon city pilot policy, fiscal pressure, and carbon productivity through indicator substitution and Tobit-DID methods, all of results reveal that the conclusions are robust. However, a further placebo test is needed to determine whether counterfactual experiments in different cities and in different periods can yield consistent conclusions. The basic idea involved the selection of a total of 34 cities as low-carbon cities in 2010 and 2012, with 248 unselected cities. Initially, there were 36 low-carbon pilot cities, but Jiyuan City is a county-level city and the Daxinganling area has missing data. Thus, the samples from these two pilot areas were deleted. Next, we randomly selected 34 cities from 282 cities as “pseudo-low-carbon cities.” Assuming that these 34 cities were selected as low-carbon cities, namely, the treatment group, and the other cities comprised the control group, then more than 500 random samples were taken to complete the placebo test.
However, the pilot policy times of the two batches of selected low-carbon cities vary, and this factor cannot be ignored in counterfactual experiments. Therefore, in the quasi-natural experiment using the staggered DID model to evaluate the effects of the low-carbon city pilot policy, it is necessary to use the placebo test method to conduct the counterfactual experiments. The specific methods are as follows. First, we used the “sample”command to randomly select a year as a pseudo-low-carbon city pilot policy year, after which we randomly selected 34 cities as pseudo-low-carbon cities and kept the city code and year from which the sample was drawn. Then, the sample was matched with the original data, and the cities that were successfully matched were classified as the pseudo-low-carbon cities (i.e., the samples of the treatment group); the cities that were not matched became the samples of the control group. Then, the dummy variable of the pseudo-low-carbon city pilot policy was generated by comparing the sequential relationship between each period and the pseudo-low-carbon city pilot policy year. Based on this estimation, the estimated coefficient, standard error, and p-value of the pseudo-low-carbon city pilot policy dummy variable were obtained. We repeated this process 1,000 times to obtain the results of the placebo effect test of the low-carbon city pilot policy. The detailed results are shown in Figure 4.
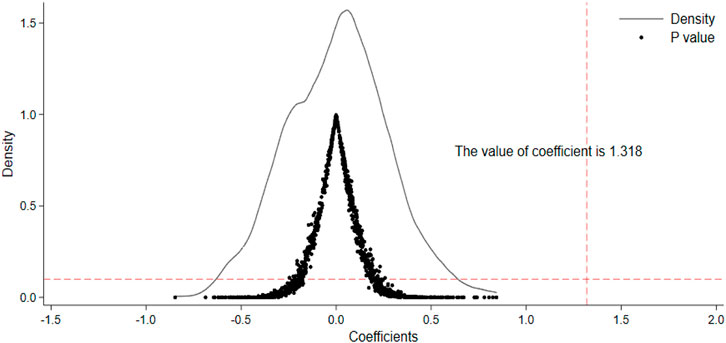
FIGURE 4. The placebo effects of thelow-carbon city pilot policy. Note: The figures show the distribution of estimated coefficients and corresponding p-values of the pseudo-low-carbon city pilot dummy variables sampled 1,000 times. The vertical dashed line represents the estimated value of the true coefficient, while the horizontal dashed line represents a value of 0.1.
The results in Figure 4 reveal that the estimated coefficients of the pseudo-low-carbon city pilot policy are basically around 0, and the corresponding p-values are all greater than 0.1. Hence, the estimated value of the low-carbon city pilot policy in the real staggered DID model is not obtained by chance or coincidence, so the influence of random factors or other similar policies can almost be ignored. This finding further illustrates that the estimated coefficient of the low-carbon city pilot policy is robust, which evidences that the pilot policy effectively promotes carbon productivity.
5 Discussion of the heterogeneity and mediation effects
In this section, we carry out two aspects of research. First, we conduct a heterogeneous analysis of the effects of the low-carbon city pilot policy and fiscal pressure on carbon productivity and test whether this effect is the same under different conditions of geographic location and economic development levels. Second, we perform a test of the effect of the mediation mechanism, explore the mechanism by which the pilot policy and fiscal pressure affect carbon productivity, and provide multiple mediation mechanisms for the impact of the low-carbon city pilot policy on carbon productivity.
5.1 Analysis of heterogeneity
There may be differences in the effects of the low-carbon city pilot policy under different geographic locations and economic development levels. Thus, we conducted heterogeneity analyses based on these aspects and discussed the reasons for any potential differences.
5.1.1 Heterogeneity test in different geographical locations
To distinguish the impact of geographic location on the effect of the low-carbon city pilot policy, we divided the sample based on two geographic locations. First, we divided the entire sample into cities from the Eastern region and those from the Central and Western regions. The Eastern region included 11 Eastern provinces and cities, such as Beijing and Tianjin. The corresponding city samples were the samples of the Eastern region. The corresponding city samples of other provinces, municipalities, and districts comprised the samples of the Central and Western regions. Second, we divided the entire sample into groups consisting of samples from the southern and northern cities. China was divided into Northern and Southern regions along the Qinling–Huai River line. Most of the cities in Jiangsu, most of the cities in Anhui, and a small part of the cities in Shaanxi, Chongqing, Sichuan, and 17 other provinces, cities, and districts comprised the group of southern cities. Other provinces, municipalities, and districts made up the northern region. The cities corresponding to other provinces, municipalities, and districts were comprised northern cities. After dividing the sample groups, we adopted the staggered DID model for estimation. The results are provided in Table 7.
Table 7 presents the estimation results of the staggered DID model under different regional conditions. In Columns 1) and (2), it is apparent that the coefficients of
At the same time, the results show that the coefficients of
5.1.2 Heterogeneity of the difference in the level of economic development
One fact that cannot be ignored is that the level of economic development itself may also have an influence on carbon productivity, which may affect the effective of the low-carbon city pilot policy. To characterize such an impact, we adopted the following methods. First, we examined whether there are differences in the effects of the low-carbon city pilot policy under different conditions of GDP size. To this end, we calculated the average GDP of each city in the sample period, sorted the averaged GDP according to size and divided the sorted data into two groups: the cities with small GDPs and those with large GDPs. Then, we used the staggered DID model to perform the estimation. Second, we also investigated whether there are differences in the effects of the low-carbon city pilot policy under different per capita GDP levels. For this, we calculated the average per capita GDP of each city in the sample period, sorted the average per capita GDP, and divided the sorted per capita GDP into city groups with low and high per capita GDP. Finally, we performed the estimation after grouping. The results are displayed in Table 8.
Table 8 presents the estimated results of the low-carbon city pilot policy effects under different sizes of GDP and levels of per capita GDP. Columns 1) and 2) show that in the city group with small GDPs, the coefficients of
The results in Columns 3) and 4) in Table 8 indicate that under the condition of cities with low levels of per capita GDP, the coefficient of
5.2 Test of the mediation effect.
The impacts of the low-carbon city pilot policy and fiscal pressure on carbon productivity are mainly achieved through technological innovation and progress, closing or restricting the production of high-energy-consuming enterprises, restricting new high-energy-consuming enterprises, and increasing the use of clean energy. At the same time, closing down enterprises will increase fiscal pressure. To resolve this problem, some local governments will partially relax restrictions on the development of high-energy-consuming industries. In view of this, we introduced four mediation variables: “energy structure,”“the number of green patent applications,”“the number of registrations of low-carbon-type enterprises,” and “fiscal pressure brought about by the low-carbon city pilot policy.” We constructed the mediation effect model of the low-carbon city pilot policy and fiscal pressure on carbon productivity and tested the mediation effect of the policy.
Among them, the number of green patent applications (
where
We refer to the methods of Baron and Kenny (1986), Imai et al. (2010), and Hicks and Tingley (2011) to construct a model of the mediation effect of the low-carbon city pilot policy on carbon productivity:
where
5.2.1 Mediation effect based on energy structure
In the process of implementing the low-carbon city pilot policy, there is a need to involve the change of energy structure. This is because raw coal, coking coal, and other traditional fossil energy sources not only contain high sulfur elements but also cause high CO2 carbon emissions. However, the use of electrical energy, especially hydropower, wind, and solar power, can effectively reduce carbon dioxide emissions. As for the change of energy structure, it is assumed that for energy consumption, the proportion of electric energy in total energy consumption will increase. For energy production, there will be a big push to develop clean energy sources, such as hydro, wind, and solar, which means that non-thermal power generation will increase. This development will effectively boost carbon productivity. Based on the mediation variables of electricity consumption ratio and non-thermal power generation, the stepwise regression coefficient method was used to estimate the mediation effect model based on the energy structure, after which we calculated the size of the mediation effect. The results are shown in Table 9.
In Table 9, when examining the mediation channels for the proportion of electricity consumption, Column 1) indicates that the coefficient of
5.2.2 Mediation effect based on the number of green patent applications
The low-carbon city pilot policy advocates low-carbon production and consumption. In the production field, this effort will inevitably lead to greener and cleaner production technological progress, which is reflected in the expansion of green patent applications. At the same time, the expansion of the number of green patents will produce corresponding rewards, such as improved clean production capacity, lower carbon emissions, and higher carbon productivity. Therefore, based on the number of green patent applications, we used the stepwise regression coefficient method to estimate the mediation effect model and then calculated the size of the mediation effect. The results are provided in Table 10.
In Table 10, without controlling for the influence of other variables, Column 1) shows that the coefficient of
5.2.3 Mediation effect based on the number of registrations of low-carbon-type enterprises
One of the most direct ways for the government to implement the low-carbon city pilot policy is to restrict the development of high-energy-consuming industries. Specifically, local governments can reduce their approval for high-carbon-type enterprises and expand access for low-carbon-type enterprises. Thus, the number of registrations of high-carbon-type enterprises will be declined, and those of low-carbon-type enterprises will be increased. If the low-carbon city pilot policy increases the number of registrations of low-carbon-type enterprises, then the pilot policy supports the development of low-carbon enterprises, in turn, improves carbon productivity. In view of this, we estimated the mediation mechanism effect model based on the number of registrations of low-carbon-type enterprises under the conditions of not controlling and controlling for the influences of other variables. The results are shown in Table 11.
In Table 11, without controlling the influence of other variables, Column 2) shows that the coefficient of
5.2.4 Mediation effect based on fiscal pressure brought about by the low-carbon city pilot policy
In the actual implementation of the low-carbon city pilot policy, some high-energy-consuming enterprises will inevitably be shut down, which will eliminate some tax sources. In the case of rigid growth of government expenditure, the fiscal expenditure gap will increase, as will fiscal pressure on the local government. Especially in the case of economic downturns and fiscal difficulties, local governments are likely to respond to fiscal pressure by loosening restrictions on the development of high-energy-consuming enterprises, which will adversely affect carbon productivity. In this regard, it is necessary to minimize the adverse effects. This paper used the interaction term between the dummy variable of the low-carbon city pilot policy and the fiscal pressure to describe the fiscal pressure brought about by such a pilot policy, after which we estimated the mediation effects based on this mediation variable. The results are provided in Table 12.
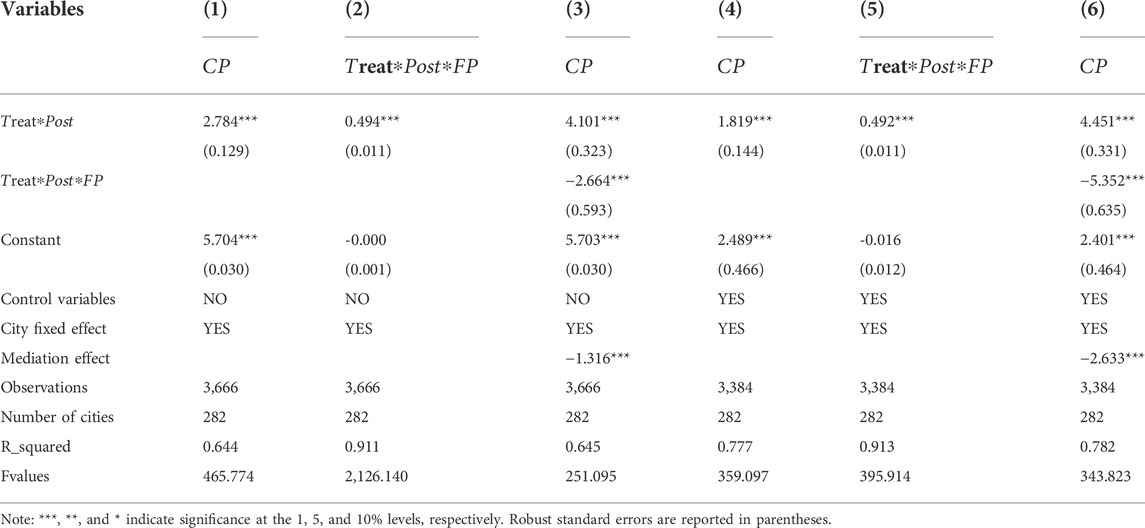
TABLE 12. Estimation results based on the fiscal pressure brought about by the low-carbon city pilot policy.
In Table 12, under the condition of controlling for the influence of other variables, Column 2) reports that the coefficient of
6 Conclusion and policy implications
The low-carbon city pilot policy is an important measure for China to promote carbon productivity, as it provides a certain degree of experience and demonstrations for China to achieve a carbon peak in 2030. At the same time, the low-carbon city pilot policy will affect the development of high-energy-consuming enterprises and the tax base to some extent, which may lead to an increase in fiscal pressure. Several results were obtained in this paper. First, the low-carbon city pilot policy can significantly improve carbon productivity, and the improvement effect presents a dynamic and persistent feature. Second, the fiscal pressure resulting from the low-carbon city pilot policy will reduce carbon productivity, and the degree of reduction depends on the status of fiscal pressure. Third, an increase in fiscal pressure will significantly decrease carbon productivity, which is heterogeneous with different levels of economic development. Finally, the examination of the mediation effect found that the low-carbon city pilot policy improves carbon productivity by affecting the energy structure, green and low-carbon technological progress, and the entry of low-carbon-type enterprises. However, the fiscal pressure brought about by the low-carbon city pilot policy has a negative impact on the improvement of carbon productivity, whose influence we cannot ignore. Therefore, the issue of how to improve carbon productivity without affecting fiscal pressure is a scientific problem. In relation to this, optimizing the low-carbon clean technology of existing enterprises and raising the low-carbon technology access standards of new enterprises may be a feasible strategy.
The conclusions drawn from the above research can offer inspiration to developing countries for improving carbon productivity in several ways. First, these countries can expand the scope of their low-carbon city pilot policy and provide policy support for improving carbon productivity. In the process of expanding the scope of such policies, the central government must strictly select the criteria, clarify the conditions and requirements for selection, and plan the strategic tasks for the development of low-carbon cities. Second, the low-carbon technology level of existing enterprises must be optimized, and the low-carbon technology access standards of new enterprises must be raised. A fact that cannot be ignored is that in the process of promoting a low-carbon economy, some local governments still need to bridge the gap between their policy approaches and local economic development conditions and resource endowments. Solving one problem can lead to the emergence of another problem. Therefore, in the process of improving carbon productivity, attention should be allocated to resolving fiscal pressure and preventing fiscal risks. Third and finally, there is a need to adjust the energy structure and improve energy efficiency. The use of traditional energy sources, such as thermal power, raw coal, crude oil, and gasoline, will lead to the emission ofmassive amounts of CO2. However, emerging energy sources, such as hydropower, wind power, and solar energy, do not generate carbon emissions. This means that if the structure of energy production and consumption can be improved to produce and use cleaner energy, which can directly reduce carbon emissions and promote carbon productivity. At the same time, even if the energy structure remains unchanged, improving energy efficiency can also promote carbon productivity.
Although our research has given rise to valuable conclusions, it still has certain limitations. First, in view of the limitations involving carbon emission data, the latest annual data were not obtained for analysis. Second, an analysis based on micro-level (enterprise) data has not been performed. In the future, we will expand and analyze these two aspects to provide strong evidence for the relationship among the low-carbon city pilot policy, fiscal pressure, and carbon productivity.
Data availability statement
The original contributions presented in the study are included in the article/supplementary material further inquiries can be directed to the corresponding author.
Author contributions
YY contributed to methodology, funding acquisition, writing—original draft preparation; CP contributed to conceptualization, writing—review, editing. All authors contributed to the article and approved the submitted version.
Funding
This paper was sponsored by the Launching Project of New Staff in Social Science of Tianjin University of Commerce (2022) (Grant No. 22KYQD14).
Acknowledgments
The authors would like to thank the editor for their attentive and friendly service, and also thank the reviewers for their constructive comments and suggestions in this paper.
Conflict of interest
The authors declare that the research was conducted in the absence of any commercial or financial relationships that could be construed as a potential conflict of interest.
Publisher’s note
All claims expressed in this article are solely those of the authors and do not necessarily represent those of their affiliated organizations, or those of the publisher, the editors and the reviewers. Any product that may be evaluated in this article, or claim that may be made by its manufacturer, is not guaranteed or endorsed by the publisher.
References
Acemoglu, D., Akcigit, U., Hanley, D., and Kerr, W. (2016). Transition to clean technology. J. Political Econ. 124 (1), 52–104. doi:10.1086/684511
Aghion, P., Dechezleprêtre, A., Hemous, D., Martin, R., and Van Reenen, J. (2016). Carbon taxes, path dependency, and directed technical change: Evidence from the auto industry. J. Political Econ. 124 (1), 1–51. doi:10.1086/684581
Andersson, J. J. (2019). Carbon taxes and CO2 emissions: Sweden as a case study. Am. Econ. J. Econ. Policy 11 (4), 1–30. doi:10.1257/pol.20170144
Angrist, J. D., and Pischke, J. S. (2008). Mostly harmless econometrics. New Jersey: Princeton University Press.
Bai, J., Lu, J., and Li, S. (2019). Fiscal pressure, tax competition and environmental pollution. Environ. Resour. Econ. (Dordr). 73 (2), 431–447. doi:10.1007/s10640-018-0269-1
Baker, A. C., Larcker, D. F., and Wang, C. C. Y. (2022). How much should we trust staggered difference-in-differences estimates? J. Financial Econ. 144 (2), 370–395. doi:10.1016/j.jfineco.2022.01.004
Baron, R. M., and Kenny, D. A. (1986). The moderator–mediator variable distinction in social psychological research: Conceptual, strategic, and statistical considerations. J. Personality Soc. Psychol. 51 (6), 1173–1182. doi:10.1037/0022-3514.51.6.1173
Calel, R., and Dechezleprêtre, A. (2016). Environmental policy and directed technological change: Evidence from the European carbon market. Rev. Econ. Statistics 98 (1), 173–191. doi:10.1162/REST_a_00470
Callaway, B., and Sant’Anna, P. H. C. (2021). Difference-in-differences with multiple time periods. J. Econ. 225 (2), 200–230. doi:10.1016/j.jeconom.2020.12.001
Chen, C., Lin, Y., Lv, N., Zhang, W., and Sun, Y. (2022). Can government low-carbon regulation stimulate urban green innovation? Quasi-experimental evidence from China’s low-carbon city pilot policy. Appl. Econ. 2022, 1–21. doi:10.1080/00036846.2022.2072466
Cheng, J., Yi, J., Dai, S., and Xiong, Y. (2019). Can low-carbon city construction facilitate green growth? Evidence from China’s pilot low-carbon city initiative. J. Clean. Prod. 231, 1158–1170. doi:10.1016/j.jclepro.2019.05.327
de Chaisemartin, C., and D'Haultfoeuille, X. (2020). Two-way fixed effects estimators with heterogeneous treatment effects. Am. Econ. Rev. 110 (9), 2964–2996. doi:10.1257/aer.20181169
Fell, H., and Kaffine, D. T. (2018). The fall of coal: Joint impacts of fuel prices and renewables on generation and emissions. Am. Econ. J. Econ. Policy 10 (2), 90–116. doi:10.1257/pol.20150321
Freyaldenhoven, S., Hansen, C., and Shapiro, J. M. (2019). Pre-event trends in the panel event-study design. Am. Econ. Rev. 109 (9), 3307–3338. doi:10.1257/aer.20180609
Gardner, J. (2021). Two-stage differences in differences. USA: University of Mississippi. Working paper.
Hicks, R., and Tingley, D. (2011). Causal mediation analysis. Stata J. 11 (4), 605–619. doi:10.1177/1536867X1201100407
Hu, X., and Liu, C. (2016). Carbon productivity: A case study in the Australian constr-uction industry. J. Clean. Prod. 112, 2354–2362. doi:10.1016/j.jclepro.2015.10.042
Huang, S., and Deng, Y. (2020). Fiscal pressure, distortion of enterprise factor input and economic performance. Fiscal Res. 3, 85–100. doi:10.19477/j.cnki.11-1077/f.2020.03.007
Huang, Y., and Zhou, Y. (2020). How does vertical fiscal imbalance affect environmental pollution in China? New perspective to explore fiscal reform’s pollution effect. Environ. Sci. Pollut. Res. 27 (25), 31969–31982. doi:10.1007/s11356-020-09072-w
Huo, W., Qi, J., Yang, T., Liu, J., Liu, M., and Zhou, Z. (2022). Effects of China's pilot low-carbon city policy on carbon emission reduction: A quasi-natural experiment based on satellite data. Technol. Forecast. Soc. Change 175, 121422. doi:10.1016/j.techfore.2021.121422
Imai, K., Keele, L., and Tingley, D. (2010). A general approach to causal mediation analysis. Psychol. Methods 15 (4), 309–334. doi:10.1037/a0020761
Jacobson, L. S., LaLonde, R. J., and Sullivan, D. G. (1993). Earnings losses of displaced workers. Am. Econ. Rev. 83 (4), 685–709.
Li, C., Qi, Y., Liu, S., and Wang, X. (2022). Do carbon ETS pilots improve cities' green total factor productivity? Evidence from a quasi-natural experiment in China. Energy Econ. 108, 105931. doi:10.1016/j.eneco.2022.105931
Li, X., and Gong, L. (2019). Correction and fitting of night light images of DMSP/OLS and VIIRS/D-NB. Bull. Surv. Mapp. 7, 138–146. doi:10.13474/j.cnki.11-2246.2019.0236
Liu, F. (2022). The impact of China’s low-carbon city pilot policy on carbon emissions: Based on the multi-period DID model. Environ. Sci. Pollut. Res. Int. doi:10.1007/s11356-022-20188-z
Liu, W. (2019). Enterprise-Registration-Data-of-ChineseMainland[EB/OL]. Available at: https://github.com/imhuste-r/Enter-prise-Registration-Data-of-Chinese-Mainland, 2019-06-01.
Liu, X., Li, Y., Chen, X., and Liu, J. (2022). Evaluation of low carbon city pilot policy effect on carbon abatement in China: An empirical evidence based on time-varying DID model. Cities 123, 103582. doi:10.1016/j.cities.2022.103582
Nordhaus, W. D. (2006). After kyoto: Alternative mechanisms to control global warming. Am. Econ. Rev. 96 (2), 31–34. doi:10.1257/000282806777211964
Pan, A., Zhang, W., Shi, X., and Dai, L. (2022). Climate policy and low-carbon innovation: Evidence from low-carbon city pilots in China. Energy Econ. 112, 106129. doi:10.1016/j.eneco.2022.106129
Peng, F., Xu, W., Lu, P., and Wu, H. (2020). Unexpected shocks of non-tax burdens: A study based on the "VAT reform. Econ. Res. 55 (11), 67–83.
Qiu, S., Wang, Z., and Liu, S. (2021). The policy outcomes of low-carbon city construction on urban green development: Evidence from a quasi-natural experiment conducted in China. Sustain. Cities Soc. 66, 102699. doi:10.1016/j.scs.2020.102699
Ren, H., Gu, G., and Zhou, H. (2022). Assessing the low-carbon city pilot policy on carbon emission from consumption and production in China: How underlying mechanism and spatial spillover effect? Environ. Sci. Pollut. Res. Int. doi:10.1007/s11356-022-21005-3
Shao, C., Guan, Y., Wan, Z., Guo, C., Chu, C., and Ju, M. (2014). Performance and decomposition analyses of carbon emissions from industrial energy consumption in Tianjin, China. J. Clean. Prod. 64, 590–601. doi:10.1016/j.jclepro.2013.08.017
Sun, L., and Abraham, S. (2021). Estimating dynamic treatment effects in event studies with heterogeneou-s treatment effects. J. Econ. 225 (2), 175–199. doi:10.16/j.jeconom.2020.09.006
Tao, D., and Li, C. (2021). Environmental regulation, local fiscal pressure and actual corporate tax burden. Econ. Sci. 3, 83–95. doi:10.12088/PKU.jjkx.2021.03.07
Wang, R., and Zhang, Q. (2017). Local governments' fiscal pressure and the dependence on polluting industries in China. China & World Econ. 25 (4), 109–130. doi:10.1111/cwe.12209
Zhao, R., and Fan, Z. (2020). Pension reform, collection and management incentives and corporate contri-bution rates. China Ind. Econ. 9, 61–79. doi:10.19477/j.cnki.11-1077/f.2021.06.003
Keywords: low-carbon city pilot, fiscal pressure, carbon productivity, staggered DID, mediation effect
Citation: Yang Y and Peng C (2022) Low-carbon city pilot policy, fiscal pressure, and carbon productivity: Evidence from china. Front. Environ. Sci. 10:978076. doi: 10.3389/fenvs.2022.978076
Received: 25 June 2022; Accepted: 30 July 2022;
Published: 06 September 2022.
Edited by:
Ayman Elshkaki, Key Laboratory of Resource Utilization and Environmental Restoration, Institute of Geographic Sciences and Natural Resources Research (CAS), ChinaReviewed by:
Luigi Aldieri, University of Salerno, ItalyElchin Suleymanov, Baku Enginering University, Azerbaijan
Copyright © 2022 Yang and Peng. This is an open-access article distributed under the terms of the Creative Commons Attribution License (CC BY). The use, distribution or reproduction in other forums is permitted, provided the original author(s) and the copyright owner(s) are credited and that the original publication in this journal is cited, in accordance with accepted academic practice. No use, distribution or reproduction is permitted which does not comply with these terms.
*Correspondence: Chunli Peng, pcl-1990@163.com; Yun Yang, yangyunpaper@126.com