- 1Guangdong Vocational Institute of Public Administration, Guangzhou, China
- 2Key Laboratory of the Sustainable Development of Xinjiang’s Historical and Cultural Tourism, Xinjiang University, Urumqi, China
- 3School of Tourism Management, SunYat-sen University, Guangzhou, China
Theorists have traditionally considered the effects of single rather than multiple landscape attributes on property prices, and ignored the interaction between landscape-specific housing attributes. The aim of this paper is to analyze the hedonic attributes of property prices and compare the effects on property prices of two key landscape attributes. Seventeen hedonic attributes are used to build a hedonic-pricing model with five dimensions: structure, neighborhood and community, location, environment, and time. A sample of 688 valid houses within 1,000 m of Xiangshan Park in the city of Zhuhai, China is collected. The selected hedonic attributes are found to have significant effects on property prices, explaining around 91% of the observed variation in price. Proximity to the sea has a greater positive influence on property prices than proximity to parks. In addition, people prefer to buy properties with sea views than to live close to the sea.
Introduction
Attracting immigrants as well as temporary visitors has become an important goal of many tourist-city authorities in the last few decades. The phenomenon of tourists’ residing for relatively long periods and even settling down fully in tourist cities has expanded from Western countries to developing countries such as China (Liang et al., 2021). Tourists and residents cohabit in many tourist cities, which creates policy issues (Rodriguez, 2001). The tourist city of Gold Coast in Australia’s “sun-belt” (Mullins, 1991) attracts a large number of long-distance immigrants seeking a better climate and lifestyle (Stimson and Minnery, 1998). The Costa del Sol in Spain, another famous tourist region, receives a massive volume of foreign tourists and immigrants, especially retired migrants (Rodriguez, 2001). The ancient town of Dali in southwestern China attracts both domestic and international immigrants. This new population growth has stimulated a wave of tourist-related real estate development in the last decade (Wu and Xu, 2010). Most new immigrants purchase or rent houses close to areas of natural beauty such as beaches, rivers, lakes and parks, or tourist attractions such as theme parks (Liang and Bao, 2015). Landscape plays a critical role in the decision to rent or purchase a house in a tourist city (Pitkanen, 2008).
The complicated relationship between property prices and landscape has become a very popular topic in the fields of tourism geography and leisure research in the last few decades. People who choose to live in tourist cities may prioritize housing and landscape features over more practical concerns such as the distance between home and the workplace (Chai et al., 2011; Li et al., 2013). Generally, people are willing to pay more for houses with more attractive environments and better facilities (Crompton, 2004). Research has shown that the price of both residential and commercial properties is significantly affected by the features of the surrounding landscape and related facilities, such as parks (Hammer et al., 1974; Schroeder, 1982; Crompton, 2001a; Crompton, 2005; Nicholls and Crompton, 2005), greenways (Mahan et al., 2000; Tyrväinen and Miettinen, 2000; Irwin, 2002; Nicholls and Crompton, 2005; Crompton and Nicholls, 2006; Shi and Zhang, 2010), rivers and other water bodies, such as lakes or the ocean (Leggett and Bockstael, 2000; Loomis and Feldman, 2003; Hui et al., 2007; Wu et al., 2008; Wen et al., 2012), golf courses (Crompton, 2000; Boswell and Crompton, 2007), shopping malls (Sirpal, 1994), schools (Mok et al., 1995; Mao et al., 2014), sport centers (Ahlfeldt and Maennig, 2007; Dehring et al., 2007), subways (Dewees, 1976; Knaap et al., 2001; Bae et al., 2003), as well as some negative attributes such as crime rate (Wu and Dong, 2014). However, previous researchers have investigated the effects of only one or a few landscape or facility attributes on property prices; the interaction between the determinants of property prices has been ignored.
However, prospective house buyers consider more than one landscape attribute before making their decisions. Zhuhai has consistently been ranked as the most “livable” tourist city in China, as indicated in a report on China Urban Livable Competitiveness (2014) published by the Chinese Academy of Social Sciences in 2015. In addition to its warm climate and relative lack of pollution, Zhuhai’s developed parks (such as theme parks), greenways, seaside corridors and many tourist facilities attract not only holiday makers but also seasonal and retired migrants, mainly from other regions of China. The permanent population of Zhuhai (defined as registered and non-registered individuals living in the city for more than 6 months per year) increased from 0.36 million in 1979 to 1.59 million in 2013. More than 0.36 million immigrants were registered as permanent residents between 2001 and 2013(Bureau, 2015). The number of non-registered immigrants is even greater. Most new immigrants (both registered and non-registered) purchase or rent houses close to the city’s parkland or seashore. Which type of landscape do they prefer? Do they intend to actively use these landscape features or simply wish to enjoy a good view? The answers to these questions not only offer insights into buyers’ motives and decision making but also guide urban planning and real-estate development within tourist cities.
Tourism landscape and property prices
It is widely accepted that many urban residents are willing to pay more for properties closer to attractive landscape features such as parks, beaches, and other open spaces. As a result, these landscape features increase the value of nearby properties. This process of capitalization is based on the proximate principle (Crompton, 2001), according to which the increasing value of nearby parkland enables real-estate developers to obtain returns on their investment or cover their management costs after construction. The proximate principle was first theorized in the first half of the 19th century, when city authorities in England began to create parks for a rapidly growing urban population (Crompton, 2004). For example, in the 1840s, Liverpool’s commissioners allocated 125 acres of land to Birkenhead Park in perpetuity, and sold 100 acres of the surrounding land for housing development. The costs of excavation, construction, planning, and management amounted to approximately £140,000, but the revenue obtained from selling the houses totaled £228,000 (Crompton, 2004). Therefore, the proximate principle repositions park expenditure as an investment rather than a cost. This idea traveled from Britain to the U.S., and eventually to the rest of the world. Today, urban real-estate development usually incorporates parks and other open spaces, or occurs close to seaside areas, rivers, greenways, lakes, golf courses, and other attractive features of the urban landscape.
In studies of the proximate principle, researchers have focused on identifying and evaluating influential variables. In early studies, the perception-based approaches and the comparison-based approach were widely used to estimate the effects of parks and other landscape features on property prices. Scholars using the perception-based approach estimated these effects based on the perceptions of respondents who owned houses in the surrounding area; and those using the comparison-based approach calculated the difference in local property prices before and after park construction. Although these two approaches are easily conducted, the results are approximate and relatively unreliable, as numerous uncontrolled variables may influence property prices. In the 1960s, the hedonic pricing method (HPM) was introduced to estimate the effects of parkland and other landscape features on property prices. This method is more reliable and accurate than either the perception-based approach or the comparison-based approach. Many case studies have since been conducted using the HPM, and increasing numbers of influential variables have been identified.
Research has shown that increasing proximity to parkland results in a statistically significant growth in land value (Hammer et al., 1974; Nicholls, 2002), which in turn increases local property prices. The effects of landscape on property prices differ both spatially and temporally, and weaken as the distance between key landscape features and property increases (Shi and Zhang, 2010). In a study of 336 properties surrounding a single park in Philadelphia, Hammer et al. (1974) found a positive relationship between property prices and properties located at the corners of the park or adjacent to the park. Nicholls and Crompton (2005a) further investigated this effect in three neighborhoods in Austin, and found that property prices in Barton, Lost Creek and Travis decreased by $13.51, $3.97, and $10.61, respectively, per foot from parkland or greenbelt. In addition, the effects of landscape on property prices have been shown to depend on the distance between a property and the relevant landscape site (e.g., a park or greenbelt) (Wolf, 2007). In an early study, Lyon claimed that the effect of landscape on property prices is strongest within approximately 600–800 feet of the given landscape feature (Lyon, 1972). Hammer, Coughlin and Horn proved that proximity to parkland accounts for 33% of the total land value at 40 feet, 9% at 1,000 feet, and 4.2% at 2,500 feet from the parkland (Hammer et al., 1974). More precise results were obtained by Bolitzer and Netusil (2000), who conducted a series of studies of 193 parks, and found that parkland has a 5.3%–7.6% effect on property sale prices within 100 feet, but only a 1.5%–3.8% effect between 1,301 and 1,500 feet. Yu et al. (2007) pointed out that a sea view adds an average of 15% to the price of a property. In addition, a significant and negative correlation has been found between increased distance from the exposed shoreline of the sea, a lake or a river and the average property-price premium (Loomis and Feldman, 2003; Sander and Polasky, 2009). Crompton summarized the findings of early studies in this area, and reported that properties “abutting or fronting” parks designed for passive use were 20% more expensive than equivalent properties located elsewhere (Crompton, 2001). He reported that this effect extended to 1,500–2,000 feet, but was concentrated within 600 feet, equivalent to a 3-min walk (Dines and Brown, 2001) or three blocks’ distance.
Some researchers have argued that no significant relationship exists between real-estate price (or value) and the proximity of parkland (Walls et al., 2013). In some cases, a negative relationship between the two variables has been found. The relationship between real-estate price (or value) and landscape/facilities usually depends on the nature of the landscape/facilities. Gartner et al. (1996) found no evidence to suggest that proximity to rivers, lakes, or public land significantly affects property prices. Walls et al. (2013) found that forest views negatively affect property prices, whereas views of farmland and grassy areas have positive effects. Proximity to large lakes (more than 500 acres) and medium lakes (25–100 acres) but not small lakes (fewer than 25 acres) has been shown to be significantly and positively correlated with property value (Gartner et al., 1996). In short, the effects of landscape on property value are closely related to the characteristics of the landscape. Different types of landscape have different effects. The average sale price of properties located within 1,500 feet of a natural park is higher than that of properties within the same distance from an urban park or a special-use park (Lutzenhiser and Netusil, 2001). The effect on property prices of open space undergoing conservation is significantly greater than that of developable farmland and forest (Irwin, 2002). The effect of parks designed for passive use is also significantly greater than that of parks designed for active leisure activities (More et al., 1982; Crompton, 2005). When a tourist city offers many kinds of landscape, which do people tend to choose? To answer this question, the effects of multiple local landscape features on the property-price premium should be considered.
Parks, beaches, and other types of open space provide opportunities for recreation, exercise, and social activities, whether active or passive, organized or spontaneous, individual or collective. Visiting parks and other attractive landscape features has been proven to have significant psychological and physiological benefits (Nicholls and Crompton, 2005b). However, it remains unclear whether people who purchase properties close to parkland intend to actively use this open space. Some studies have indicated that a view of attractive landscape features significantly affects the local property-price premium. Tyrväinen and Miettinen (2000) found that properties with a forest view were on average 4.9% more expensive than properties with otherwise similar characteristics. In a study of Lake Erie, Bond, Seiler and Seiler (2002) found that a lake view was sufficiently desirable to add 89.9% to the value of a property. People are also willing to pay more for properties with sea views (Schroeder, 1982; Mok et al., 1995; Hui et al., 2007). Therefore, it remains unclear whether people who purchase properties close to attractive landscape features intend to actively use these features or simply wish to enjoy a good view.
Methodology
Study area
Zhuhai, which adjoins Macao, has been ranked as the most “livable” tourist city in China, attracting not only urban tourists but immigrants from Hong Kong and Macao, where property prices are high, and China’s northern provinces, where winters are cold. Many of these immigrants choose to purchase houses near Xiangshan Park (a large city park with free entry). As well as providing convenient transport links to all of Zhuhai’s tourist attractions, the area around Xiangshan Park offers warm weather in winter, relative low property prices, developed living amenities and, most importantly, a rich urban landscape. As shown in Figure 1, there are three city parks in this area (Xiangshan Park, Huafa Exercise Park, and Martyrs’ Park), along with a seafront road (Qinglv Road), many bus stations and numerous types of living facilities such as hospitals, community-service centers, shopping malls, supermarkets, food markets, convenience stores and cultural and entertainment facilities. The 1,400-acre Xiangshan Park is the most important landmark; Huafa Exercise Park and Martyrs’ Park are much smaller, and thus unlikely to have a significant effect on house-purchasing decisions. Nevertheless, all three parks provide opportunities for residents to exercise, hike and walk. Qinglv Road is the area’s most famous sea-view “corridor,” extending for 28 km. Following government-led development in 2015 to make the seafront more cosmopolitan and attractive, Qingly Road has become an important tourist attraction. It has recently been used as the Zhuhai International Half-Marathon race-track, and provides a good open space for sightseeing, recreation and exercise. Samples of property located within 1,000 m of Xiangshan Park were selected for analysis.
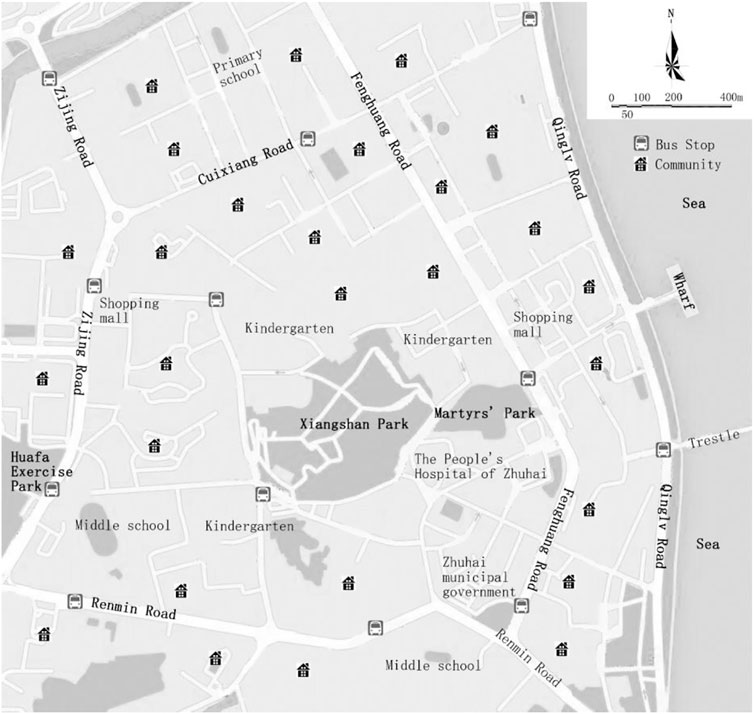
FIGURE 1. The site of Xiangshan Park Area, Zhuhai city, China. Source: Created based on the planning map of GoogleMaps [digital data]. Base map available at: http://ditu.google.cn.
HPM
The HPM was the core analytical tool used in our study. This method was first developed by Lancaster (Lancaster, 1966), and then refined and revised by Rosen (Rosen, 1974). Using the HPM, the property price is modeled as the set of all its characteristics, denoted by x = (x1, x2,…, xn). Next, a functional relationship between the price (y) and the above vector is formed, as purchasing a property is assumed to be equivalent to purchasing a set of characteristics (Hite et al., 2001; Hui et al., 2007). The intangible characteristics or attributes of the property are reflected in the final price people are willing to pay. The HPM works best when housing can be located and paired (Gartner et al., 1996). The factors that influence property prices are complicated. Those most frequently cited are related to location, structure and neighborhood (Mok et al., 1995). Nicholls (2002) extended the list of factors and divided them into six groups: 1) the structural features of property, such as lot size and quality of decoration; 2) neighborhood conditions, such as property management; 3) community conditions, such as the quality of local living facilities; 4) locational factors, such as the distance to a given landscape feature; 5) environmental factors, such as noise and air pollution; and 6) time-related factors, such as the age of the property at the time of sale.
Most researchers have chosen a small number of factors relevant to the study setting without allocating them to groups (Bolitzer and Netusil, 2000; Leggett and Bockstael, 2000; Hite et al., 2001). However, based on the six groups of factors suggested by Nicholls (2002), and considering the characteristics of the Xiangshan Park area in Zhuhai, 17 factors were chosen as independent variables, and property sale price was used as the dependent variable in the HPM. Table 1 illustrates the variables used in the study, along with their predicted signs and relevant methods of non dimensionization.
Data collection and processing
The selling season of houses in Zhuhai for domestic buyers is normally between May and July before summer holiday, and between October and December before winter vacation. Therefore, the sale prices of all properties sold in the area under study from 15 May 2015 to 6 June 2015 were collected from the websites of two large real-estate agencies: Anjuwang (www.anjuke.com) and Soufang (www.fang.com). These properties contain all types of dwelling ranging from single apartment, two-room house, to multiple bedroom villa. These companies monopolize the real-estate market in Zhuhai, and provide data on more than 80% of their transactions online. Of 851 samples collected, 688 from 22 residential areas within 1,000 m of Xiangshan Park were analyzed; the remaining 163 samples were deleted due to duplication (details provided on both websites) or abnormality (extreme or unreliable values). Data on the independent variables—structural factors, community-/neighborhood-related factors and time-related factors—and the dependent variable of sale price were obtained from the two websites and verified by field research. The field research was conducted in June 2015 to compare the data obtained from the websites, fill in missing values and, most importantly, assess the quality of the properties’ decoration, living facilities, community management, and community environment. The variables quality of community management and quality of environment were examined by observation and through interviews with residents. The linear location variables were determined using Google Maps (www.google.cn/maps).
As the variables were either continuous variables (measured in actual values), dummy variables (1 or 0) or ordinal variables (1–5), nondimensionalization was performed to reduce the influence of unit or magnitude difference. The HPM distinguishes between four functional forms: a linear form, a logarithmic form, a linear logarithmic form and a log-linear form. The dependent variable used in the study was the natural logarithm of sale price; the independent variables concerning distance and area were logarithmic; and Z-scores were used to non dimensionalize the ordinal variables—property-management fees and number of bus lines—and the dummy variables with actual values. In addition, average values were calculated to replace missing data. Table 2 shows the key descriptive statistics obtained from the data processing.
Results
Effects of urban landscape on property prices
The correlation coefficients obtained for the three key landscape variables—sea view (SEAVIEW), distance from park (DPAR) and distance from sea (DSEA)—and the dependent variable, property sale price, were examined. The three landscape variables were found to be significantly correlated with the dependent variable. Forced entry multiple linear regressions were conducted using the software package SPSS 20.0. To prevent multicollinearity and determine the effect of each landscape variable on property sale price, a separate regression with controlled variables was carried out (see Model 1, Table 3), followed by a regression for each landscape variable (see Models 2–4, Table 3). Finally, a separate regression with controlled variables and all of the landscape variables (Model 5) was conducted, as shown in Table 3.
The results of regressing Model 1 indicated that 12 controlled variables significantly influenced property prices. LOT, FEE, LFAC, PUBS, MANA, DECORATION, and POOL had positive and significant effects on property price, while AGE, DEXE, and DCEM had a negative and significant influence. These results were as expected, and in line with the findings of most early studies. However, some results were inconsistent with our predictions. TRSP and PARKING had negative rather than positive effects on property prices, while neither LIFT nor CENV had a significant effect on property prices. Property prices are generally assumed to increase with the number of accessible bus lines and the availability of parking spaces. However, although public bus services offer convenient transport links, they also increase noise and traffic congestion. As properties near transportation hubs are subject to noise, air pollution and traffic congestion on a daily basis, their sale price will be lower (Wu et al., 2008; Wen et al., 2012). The field investigation conducted during this study revealed that car-parking spaces and residential properties in the Xiangshan Park area of Zhuhai are sold separately; as a result, the cost of parking does not contribute directly to property price. Xiangshan Park is located in the old city center, where car-parking spaces are always insufficient, despite the construction of new residential communities in recent years. Most new parking facilities have been constructed in older communities with lower property prices, where there is more public space and land value is lower, making parking spaces cheaper. In short, more parking is available near properties with lower prices. Overall, Model 1 performed well, with an adjusted R2 value of 0.916 (F = 534.331, p = 0.00).
The regression results for Models 2 to 4 indicate the effects of individual landscape variables, namely DPAR, DSEA, and SEAVIEW, on property sale price. Model 1 (a separate regression with controlled variables only) was compared with Models 1 to 4, and DPAR (Model 2), DSEA (Model 3), and SEAVIEW (Model 4) were found to increase the original model’s explanatory power by 0.1%, 0.1%, and 0.2%, respectively. Model 2 performed well, with an adjusted R2 value of 0.917 (F = 505.367, p = 0.00). The regression results indicate that for every 1% increase in distance from Xiangshan Park, property prices rise by 0.056%. The finding that proximity to Xiangshan Park negatively affects property prices was contrary to our expectation. Model 3 also performed well, with an adjusted R2 value of 0.917 (F = 508.761, p = 0.00). With the exception of distance to Martyrs’ Cemetery and access to elevators, all of the independent variables in Model 3 significantly influenced the sale price of housing in the area under study. According to the main regression results, for every 1% increase in DSEA, property prices dropped by 0.055%. As expected, therefore, proximity to the seashore had a significant and positive influence on property prices. Model 4 also performed well, with an adjusted R2 value of 0.918 (F = 514.323, p = 0.00). All the independent variables in Model 4 significantly affected property prices. The main regression results indicated that a 1% increase in the likelihood of a sea view increased property prices by 0.059%. As expected, therefore, the variable Seaview was found to have a significant positive influence on property prices.
Model 5, which included the controlled variables and all the landscape variables, performed well, with an adjusted R2 value of 0.918 (F = 484.039, p = 0.00). When all three landscape variables were considered, only DSEA and SEAVIEW were found to have a significant influence on property prices; the effect of DPAR was not significant. For every 1% increase in the likelihood of a sea view, property prices rose by 0.072%. For every 1% increase in DSEA, property prices fell by 0.040%. People are most likely to buy properties near the sea or with sea views. In addition, comparison of the results for Model 3 and Model 4, with the same controlled variables, revealed that Model 4 (adjusted R2 = 0.918, F = 514.323, p = 0.00) had greater explanatory power than Model 3 (adjusted R2 = 0.917, F = 508.761, p = 0.00). Even in Model 5, the standardized regression coefficient of SEAVIEW (β = 0.072) was greater than that of DSEA (β = −0.040), which indicates that sea views have a greater influence on property prices than proximity to the sea. Together, these results confirm that people prefer to own properties with sea views rather than to live close to the sea.
Property-price premium
In the logarithmic model, the regression coefficients of each continuous variable corresponded to the price elasticity of housing characteristics; that is, when other variables remained constant, a 1% change in a given variable was assumed to result in a 1% change in housing price. Due to the presence of discontinuous variables, unstandardized regression coefficients cannot be used under conditions of semi-elasticity, so anti-logarithmic regression coefficients were obtained. The results are presented in Table 4.
The results for the individual landscape variables are as follows: 1) Proximity to Xiangshan Park had a negative effect on property values: every 1% increase in distance from the park led to a 0.056% increase in property prices. 2) Proximity to the seashore had a positive influence on property prices: every 1% increase in distance from the sea decreased property prices by 0.055%. 3) A sea view increased total property prices by 9.308%. 4) Proximity to Huafa Exercise Park, one of the three open spaces in the area under study, also had a significant effect on property prices. Every 1% increase in distance to Huafa Exercise Park led to a 0.033% drop in property prices. Of all of the landscape variables, only distance to Xiangshan Park (DPAR) had a significant negative effect on property prices. There are three reasons for this finding. First, Xiangshan Park is located in the old center of Zhuhai, and properties closer to the park are sold at lower prices. Second, as the park is the area’s main open space, it is noisy and compromises the privacy of surrounding properties. Third, a large proportion of the properties sampled in this study were located between Xiangshan Park and Qinglv Road, as in Figure 1, so properties closer to the park were farther from the sea. This also explains a key finding from Model 5 (Table 3): when all of the landscape variables were considered in a single regression, distance to Xiangshan Park (DPAR) had a positive but insignificant effect on property price.
Using average housing prices and hedonic attributes as benchmarks, the marginal prices of the hedonic property attributes were estimated. As shown in the descriptive statistics in Table 2, the average logarithmic price was 4.82. As shown in Table 4, the marginal prices of the logarithmic variables were obtained from the elastic coefficient, and the marginal prices of the other variables were derived from the semi-elastic coefficient. As indicated in Table 4, 1) each additional 100 m from Xiangshan Park increased property prices by 10,550 yuan RMB; 2) each additional 100 m from the seashore decreased property prices by 7,640 yuan RMB; 3) a sea view increased total property prices by 1,27,280 yuan RMB, which indicates that the landscape variable Seaview had a significant positive influence on property prices; and 4) each additional 100 m to Huafa Exercise Park decreased property prices by 3,760 yuan RMB.
Conclusion and discussion
Tourist cities in China today, benefiting from several tourist attractions, generally offer a livable environment and the facilities required to attract not only temporary visitors, but also new-rich immigrants from the northern provinces, Macao and Hong Kong. However, prospective house buyers consider a series of property-related factors, such as landscape variables, before making a purchase decision. The aim of this study was to determine the effects of landscape characteristics on the market price of residential properties in a mature tourist city. A larger set of landscape attributes than used in most previous studies—several of which investigated only one attribute—was examined. The HPM was used to analyze 688 properties sampled from 22 residential areas within 1,000 m of Xiangshan Park, offering valuable insights into the effects of multiple landscape features on the property-price premium. More specifically, an important theoretical question is answered: how much is a tourist-city immigrant willing to spend on various landscape attributes of a property? The major findings are as follows. First, LOT, FEE, LFAC, PUBS, MANA, DECORATION, and POOL had significant positive effects on property prices, while AGE, DEXE, and DCEM had significant negative effects. Contrary to expectation, TRSP and PARKING had a negative influence on property prices, and neither LIFT nor CENV had a significant effect on property prices. These controlled independent variables explained 91.6% of the observed variation in property prices. Second, people were found to prefer properties close to the sea, especially having a sea view than those close to large parks.
However, it remains unclear whether immigrants purchase properties close to attractive landscape features primarily to enjoy a good view or to use the available open space for leisure activities such as exercise, hiking, riding, and walking. In many early studies, the effects of landscape and other facilities on property prices were found to vary substantially according to the usage of these facilities. Some early evidence was obtained that parks designed for passive use have a greater positive influence on property prices than parks designed for active use (More et al., 1982; Crompton, 2005). Crompton’s review of the literature on the effects of landscape features on property prices revealed that properties “abutting or fronting a passive park” are of particular value (Crompton, 2001a). A passive area is generally an undeveloped space or environmentally sensitive area in which minimal human activity occurs. Passive areas do not usually provide sports infrastructure or other opportunities to engage in strenuous physical activity, although they may contain open space. For a property buyer, a passive area is a quiet landscape that provides a good view rather than an opportunity for recreation. Therefore, properties with a good view of the surrounding landscape, especially sea views, are attractive to people who wish to immigrate to a tourist city.
To conclude, this study increases understanding of property-price determination in tourist cities. The results are useful not only to immigrants who plan to purchase properties with various landscape options in tourist cities, but also for city governments or planning authorities seeking to make tourist cities more attractive. First, as houses with sea view are more attractive, the development of urban residential areas should take full advantage of the desirability of a sea view. Second, proximity to large parks has a significant positive influence on property prices. Passive parks are preferred. To increase the sale price of real estate surrounding a park, the park should provide a quiet, clean, and comfortable environment for relaxation rather than offering substantial sports infrastructure or other opportunities to engage in strenuous physical activity.
The research has several limitations that may be considered as future research directions. First, although the study used a representative case area, future research ought to be conducted in other case sites with different potential house buyers. Moreover, the study can be advanced by exploring several other house variables, such as education factor (e.g., school district), distance to airport or high-speed rail station, which may provide more fruitful insights into understanding the casual relationships certified in this research.
Data availability statement
The raw data supporting the conclusion of this article will be made available by the authors, without undue reservation.
Author contributions
ZL conceived the conceptual design and methodology of the article. DS offered research funding, edited, and improved the final manuscript, YZ and ZL carried out the investigation, formal analysis, and wrote the first research draft. All authors contributed to the article and approved the submitted version.
Funding
This work was supported by grants from the Youth Innovation Talent Project of Guangdong Ordinary Colleges and Universities (No. 2019GWQNCX051) and Zhuhai Tourism Academy.
Acknowledgments
The authors thank Shuhui Yang, Aoxiang Yang, Huitian Wu, Yiling Zhu, Tingting Yang, Ling Liu, and Min Wang for their help in data collection and data analysis.
Conflict of interest
The authors declare that the research was conducted in the absence of any commercial or financial relationships that could be construed as a potential conflict of interest.
Publisher’s note
All claims expressed in this article are solely those of the authors and do not necessarily represent those of their affiliated organizations, or those of the publisher, the editors and the reviewers. Any product that may be evaluated in this article, or claim that may be made by its manufacturer, is not guaranteed or endorsed by the publisher.
References
Ahlfeldt, G. M., and Maennig, W. (2010). Impact of sports arenas on land values: Evidence from Berlin. Ann. Reg. Sci. 44 (2), 205–227. doi:10.1007/s00168-008-0249-4
Bae, C. H. C., Jun, M. J., and Park, H. (2003). The impact of Seoul's subway Line 5 on residential property values. Transp. policy 10 (2), 85–94. doi:10.1016/S0967-070X(02)00048-3
Bolitzer, B., and Netusil, N. R. (2000). The impact of open spaces on property values in Portland, Oregon. J. Environ. Manag. 59 (3), 185–193. doi:10.1006/jema.2000.0351
Boswell, W. J., and Crompton, J. L. (2007). A city’s strategy to fund a golf course by developing homes on proximate property. J. Park Recreat. Adm. 25 (1), 115–127.
Chai, Y. W., Zhang, Y., and Liu, Z. l. (2011). Spatial differences of home-work separation and the impacts of housing policy and urban sprawl: Evidence from household survey data in Beijing. Acta Geogr. Sin. 66 (2), 157–166. doi:10.11821/xb201102002
Crompton, J. L. (2000). Designing golf courses to optimize proximate property values. Manag. Leis. 5 (4), 192–199. doi:10.1080/13606710010001770
Crompton, J. L. (2001b). Perceptions of how the presence of greenway trails affects the value of proximate properties. J. Park Recreat. Adm. 19 (3), 114–132.
Crompton, J. L. (2001a). The impact of parks on property values: A review of the empirical evidence. J. Leis. Res. 33 (1), 1–31. doi:10.1080/00222216.2001.11949928
Crompton, J. L. (2005). The impact of parks on property values: Empirical evidence from the past two decades in the United States. Manag. Leis. 10 (4), 203–218. doi:10.1080/13606710500348060
Crompton, J. L. (2004). The proximate principle: The impact of parks, open space and water features on residential property values and the property tax base. Second ed. Virginia: National Recreation and Park Association.
Crompton, J., and Nicholls, S. (2006). An assessment of tax revenues generated by homes proximate to a greenway. J. Park Recreat. Adm. 24 (3), 103–108.
Dehring, C. A., Depken, C. A., and Ward, M. R. (2007). The impact of stadium announcements on residential property values: Evidence from a natural experiment in Dallas‐Fort Worth. Contemp. Econ. Policy 25 (4), 627–638. doi:10.1111/j.1465-7287.2007.00077.x
Dewees, D. N. (1976). The effect of a subway on residential property values in Toronto. J. Urban Econ. 3 (4), 357–369. doi:10.1016/0094-1190(76)90035-8
Dines, T. N., and Brown, K. (2001). Landscape architect's portable handbook. New York: McGraw-Hill Professional Publishing, 27–44.
Gartner, W. C., Chappelle, D. E., and Girard, T. C. (1996). The influence of natural resource characteristics on property value: A case study. J. Travel Res. 35 (1), 64–71. doi:10.1177/004728759603500110
Hammer, T. R., Coughlin, R. E., and Horn IV, E. T. (1974). The effect of a large urban park on real estate value. J. Am. Inst. Planners 40 (4), 274–277. doi:10.1080/01944367408977479
Hite, D., Chern, W., Hitzhusen, F., and Randall, A. (2001). Property-value impacts of an environmental disamenity: The case of Landfills. J. Real Estate Finance Econ. 22 (2-3), 185–202. doi:10.1023/A:1007839413324
Hui, E. C., Chau, C. K., Pun, L., and Law, M. Y. (2007). Measuring the neighboring and environmental effects on residential property value: Using spatial weighting matrix. Build. Environ. 42 (6), 2333–2343. doi:10.1016/j.buildenv.2006.05.004
Irwin, E. G. (2002). The effects of open space on residential property values. Land Econ. 78 (4), 465–480. doi:10.2307/3146847
Knaap, G. J., Ding, C., and Hopkins, L. D. (2001). Do plans matter? The effects of light rail plans on land values in station areas. J. Plan. Educ. Res. 21 (1), 32–39. doi:10.1177/0739456X0102100103
Lancaster, K. J. (1966). A new approach to consumer theory. J. political Econ. 74 (2), 106–107. doi:10.1007/978-3-642-51565-1_34
Leggett, C. G., and Bockstael, N. E. (2000). Evidence of the effects of water quality on residential land prices. J. Environ. Econ. Manag. 39 (2), 121–144. doi:10.1006/jeem.1999.1096
Li, X. G., Qiu, D. C., Li, F., and Zhen, Z. (2013). Matching analysis of the job and residence space of residents in the public rental housing community in Chongqing. Geogr. Res. 32 (8), 1457–1466. CNKI:SUN:DLYJ.0.2013-08-011.
Liang, Z., and Bao, J. (2015). Tourism gentrification in Shenzhen, China: Causes and socio-spatial consequences. Tour. Geogr. 17 (3), 461–481. doi:10.1080/14616688.2014.1000954
Liang, Z., Luo, H., and Hui, T. (2021). Moving for a good life: Tourism mobility and subjective well-being of Chinese retirement migrants. Tour. Geogr., 1–19. doi:10.1080/14616688.2021.1990385
Loomis, J., and Feldman, M. (2003). Estimating the benefits of maintaining adequate lake levels to homeowners using the hedonic property method. Water Resour. Res. 39 (9), 1–6. doi:10.1029/2002WR001799
Lutzenhiser, M., and Netusil, N. R. (2001). The effect of open spaces on a home's sale price. Contemp. Econ. Policy 19 (3), 291–298. doi:10.1093/cep/19.3.291
Lyon, D. W. (1972). The spatial distribution and impact of public facility expenditures. Berkeley, California: Department of City and Regional Planning, University of California.
Mahan, B. L., Polasky, S., and Adams, R. M. (2000). Valuing urban wetlands: A property price approach. Land Econ. 76 (1), 100–113. doi:10.2307/3147260
Mao, F. F., Luo, G. F., and Pan, J. S. (2014). The effect of elementary education on the price of Hangzhou school district housing. Urban Environ. Stud. 1 (2), 53–64. CNKI:SUN:CSHY.0.2014-02-006.
Mok, H. M., Chan, P. P., and Cho, Y. S. (1995). A hedonic price model for private properties in Hong Kong. J. Real Estate Finan. Econ. 10 (1), 37–48. doi:10.1007/BF01099610
More, T. A., Stevens, T., and Allen, P. G. (1982). The economics of urban parks: A benefit/cost analysis. Parks Recreat. 17 (8), 31–33.
Mullins, P. (1991). Tourism urbanization. Int. J. Urban Reg. Res. 15 (3), 326–342. doi:10.1111/j.1468-2427.1991.tb00642.x
Nicholls, S., and Crompton, J. L. (2005a). Impacts of regional parks on property values in Texas. J. Park Recreat. Adm. 23 (2), 87–108.
Nicholls, S., and Crompton, J. L. (2005b). The impact of greenways on property values: Evidence from Austin, Texas. J. Leis. Res. 37 (3), 321–341. doi:10.1080/00222216.2005.11950056
Nicholls, S. (2002). Does open space pay? Measuring the impacts of green spaces on property values and the property tax base. College Station, Texas: Texas A&M University.
Pitkanen, K. (2008). Second-home landscape: The meaning(s) of landscape for second-home tourism in Finnish Lakeland. Tour. Geogr. 10 (2), 169–192. doi:10.1080/14616680802000014
Rodriguez, V. (2001). Tourism as a recruiting post for retirement migration. Tour. Geogr. 3 (1), 52–63. doi:10.1080/14616680010008702
Rosen, S. (1974). Hedonic prices and implicit markets: Product differentiation in pure competition. J. Polit. Econ. 82 (1), 34–55. doi:10.1086/260169
Sander, H. A., and Polasky, S. (2009). The value of views and open space: Estimates from a hedonic pricing model for Ramsey County, Minnesota, USA. Land Use Policy 26 (3), 837–845. doi:10.1016/j.landusepol.2008.10.009
Schroeder, T. D. (1982). The relationship of local public park and recreation services to residential property values. J. Leis. Res. 14 (3), 223–234. doi:10.1080/00222216.1982.11969519
Shi, Y. S., and Zhang, R. (2010). Temporal-spatial impact effects of large-scale parks on residential prices: Exemplified by the Huangxing Park in Shanghai. Geogr. Res. 29 (3), 510–520. doi:10.11821/yj2010030014
Sirpal, R. (1994). Empirical modeling of the relative impacts of various sizes of shopping centers on the values of surrounding residential properties. J. Real Estate Res. 9 (4), 487–505. doi:10.1080/10835547.1994.12090766
Stimson, R. J., and Minnery, J. (1998). Why people move to the 'sun-belt': A case study of long-distance migration to the Gold Coast, Australia. Urban Stud. 35 (2), 193–214. doi:10.1080/0042098984943
Tyrväinen, L., and Miettinen, A. (2000). Property prices and urban forest amenities. J. Environ. Econ. Manag. 39 (2), 205–223. doi:10.1006/jeem.1999.1097
Walls, M., Kousky, C., and Chu, Z. (2013). Is what you see what you get? The value of natural landscape views. Land Econ. 91 (10), 1–19. doi:10.3368/le.91.1.1
Wen, H. Z., Bu, X. Q., and Qin, Z. F. (2012). The spatial effect of urban lakes on housing prices: The case of the West Lake in Hangzhou. Econ. Geogr. 32 (11), 58–64. CNKI:SUN:JJDL.0.2012-11-009.
Wen, H. Z., Li, X. N., and Zhang, L. (2012). Impacts of the urban landscape on the housing price: A case study in hangzhou. Geogr. Res. 31 (10), 1806–1814. doi:10.11821/yj2012100007
Wu, D. M., Guo, Z. X., and Chen, H. G. (2008). Impact of lake landscape on urban residential property values in Nanjing. Resour. Sci. 30 (10), 1503–1510.
Wu, W., and Dong, G. (2014). Valuing the “green” amenities in a spatial context. J. Regional Sci. 54 (4), 569–585. doi:10.1111/jors.12099
Wu, Y. F., and Xu, H. G. (2010). A study on the development and socio-cultural impact of tourism real estate in the ancient town of Dali. Hum. Geogr. 25 (4), 67–71. CNKI:SUN:RWDL.0.2010-04-015.
Keywords: landscape, property prices, hedonic analysis, urban tourism, Zhuhai city
Citation: Shi D, Zhang Y and Liang Z (2022) The effects of park and sea landscape on property value in a tourist city. Front. Environ. Sci. 10:967094. doi: 10.3389/fenvs.2022.967094
Received: 12 June 2022; Accepted: 12 September 2022;
Published: 27 September 2022.
Edited by:
Rita Yi Man Li, Hong Kong Shue Yan University, Hong Kong SAR, ChinaReviewed by:
David Brasington, University of Cincinnati, United StatesMohd Faris Dziauddin, Sultan Idris University of Education, Malaysia
Copyright © 2022 Shi, Zhang and Liang. This is an open-access article distributed under the terms of the Creative Commons Attribution License (CC BY). The use, distribution or reproduction in other forums is permitted, provided the original author(s) and the copyright owner(s) are credited and that the original publication in this journal is cited, in accordance with accepted academic practice. No use, distribution or reproduction is permitted which does not comply with these terms.
*Correspondence: Zengxian Liang, liangzx3@mail.sysu.edu.cn