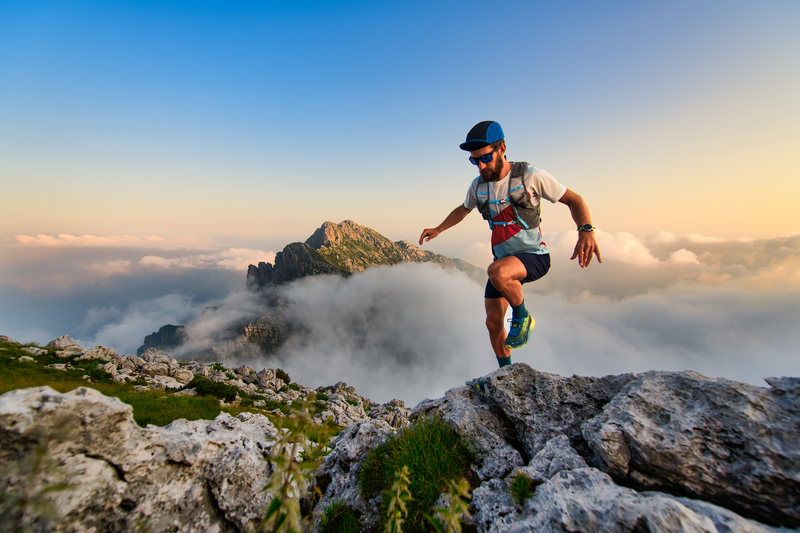
95% of researchers rate our articles as excellent or good
Learn more about the work of our research integrity team to safeguard the quality of each article we publish.
Find out more
BRIEF RESEARCH REPORT article
Front. Environ. Sci. , 10 October 2022
Sec. Environmental Economics and Management
Volume 10 - 2022 | https://doi.org/10.3389/fenvs.2022.966656
This article is part of the Research Topic Green Energy Technologies for Environmental Sustainability: Drivers, Challenges, and Future Aspects View all 27 articles
The economic progress of China cannot be denied; however, deteriorating environmental quality is the primary concern to be addressed. Since the last few years, China’s legislators have intended to facilitate green transformation by sustaining natural resources and ensuring renewable energy consumption. Therefore, this study explores the impact of renewable energy, natural resource green innovation, and economic growth on ecological footprints in China. The bootstrap autoregressive distributed lag (ARDL) model has been deployed by taking the quarterly data ranging between 1990Q1 and 2020Q4. The results reveal that by enhancing green innovation, China can transform its economy into a clean environment. Similarly, the effective utilization of renewable energy consumption is indispensable to reducing the ecological footprint in the long run. Moreover, the Error Correction Term (ECT) is significantly negative, confirming a stable long-run equilibrium relationship between model variables. Similar results are found in the short run; however, the marginal impact of all variables is stronger in the long run. It implies that long-term planning is imperative in China for optimal benefits from renewable energy and green technologies.
The deteriorating environment is becoming a global threat to humans’ survival as more than three-fourths of the world faces these challenges. It is one of the primary hurdles in maintaining societal growth (Umar et al., 2021). Amid rising ecological concerns, protecting environmental quality is on the utmost agenda of all countries (Zhang et al., 2022). The abusive utilization of natural resource rents, deforestation, and increasing pollution are the key sources of environmental destruction (Zuo et al., 2021). As reported by C-W. Su et al. (2021), several countries are adopting the environmental regulations proposed by the United Nations Conference in 1972 through practical policy implications. To achieve economic growth, numerous developing countries like China contribute adversely to the environment through industrialization and manufacturing processes. It has been stated by Khan M. A. et al. (2020) that China is sharing 30% of the global expansion in the economy, reflecting the fastest growing country in the world. However, due to the expansion of the economy utilizing the export and manufacturing sector, China is leaving negative impressions on the environment (Zhang et al., 2022). Matuštík and Kočí (2021) argued that China requires two more planets to absorb their waste material and regenerate their natural resources. China is plagued by severe ecological distresses that could affect its economic growth. Thus, it seems to be an essential concern to analyze the hazardous consequences of determinants such as renewable energy, green innovation, and natural resources on the environment in the context of China.
Providing the hazardous impact of economic advancement on the atmosphere and seeking the answers to the pollution problems linked with the industrial revolution has become the basis of green development. The emerging concept of the green economy presented by the United Nations in 2008 is dynamically implemented at the national level and has also been appreciated and improved by UN officials (Zhang et al., 2022). Based on the framework of 17 objectives, the UN sustainable development goals for 2030 were also proposed in 2015, having the critical aspect of eco-friendly regulations. The well-being of the environment is hindered in China due to the vast underdeveloped regions, as these areas are straining natural resources. China is eager to devise all possible regulations to achieve sustainable goals so far by implementing the objectives for sustainable development into the country’s development strategy (Khan et al., 2022). Green innovation and investment, natural resources, growth, and renewable energy are imperative to address climate vulnerabilities, while the 14th 5-year plan of China is continuously enhancing the need to implement the green economy at the national level (Su et al., 2021). On the contrary, Z-W. Su et al. (2021) reveal that enhancing green innovations for sustainable development is crucial for society.
Undoubtedly, society and the environment are correlated; social and natural health is not separate. Consequently, nature and economic development may conserve in a parallel direction. Salah and El-Haggar (2007) stated that misuse of natural resources causes environmental hazards. For several reasons, careless utilization of resources by humans poses a threat to the depletion of natural resources. The manufacturing ability of natural resources exceeds; as a result, the earth is propelled towards destruction (Umar et al., 2021). Such an imbalance of demand and supply between resources and human needs is cumulating greenhouse emissions and destroying the planet’s ecosystem (Zhang et al., 2022). Since the adequate availability of natural resources and their ecological footprints has been a long debate for many years (Johnsson et al., 2019), such association complies that ecological footprints are increasing due to industrialization, which causes the exploitation of natural resources leading to the country’s economic growth. Henceforth green innovation and natural resource rent seem to be the key determinant in accessing the environment’s quality for the developing sector. It is also noted that green technology innovation is not easy to adopt in developing nations as it is crucial to favor low-carbon sustainability (Jun et al., 2015; Baloch et al., 2019). Yet, green technology innovation is helping to utilize energy and natural resources more efficiently, advancing the mode of energy conservation and improving the ecological ambiance of emerging economic systems.
Energy and natural resources are consumed through a weak operation level and reverse convention method in China (Su, 2021). Therefore, economic development is dependent on energy and natural resources, resulting in colossal wastage and pollution. Understanding the importance of economic development with ecological safety, Chen et al. (2012) emphasized that the existing economic systems should revisit the association between growth and usage of natural resources, consider their expected outcomes, and devise the efficiency of green innovation. Accordingly, the association between environmental degradation and green innovation is of paramount concern in achieving the sustainable development goals of 2030. It improves the use and allocation of natural resources and helps to increase resources’ ability to achieve sustainable development goals. The green economy is a healthy global ecosystem (Wang et al., 2020). The reasons above are thus justified for the present study to include economic growth, natural resource rent, renewable energy consumption, and green innovation to observe their influence on the ecological footprint of China.
The current study contributes to prevailing literature in various aspects. It utilizes quarterly data from 1990 to 2020 to explore the dynamic linkages between renewable energy, green innovation, resource consumption, and ecological footprints in China. Recently, Su et al. (2021) have identified the association between human, social, and economic development and the environment’s quality, along with various studies that discussed green development from a social standpoint (Wang et al., 2020). However, no noticeable research has been found that presents these variables in a multivariate framework. Hence, the current study contributes to the extant literature by exploring the dynamic assessment of green innovation along with natural resource rent, economic development, and renewable energy consumption with the environmental quality in China. It utilized the novel bootstrap autoregressive distributed lag (BARDL) model to fulfill the required objectives. BARDL is more flexible than the traditional ARDL model as it provides various statistical assumptions relaxation compared to conventional ARDL.
The rest of the study is systematized as follows. The second section describes the critical and systematic review of the literature with the possible gaps, and the third section explains the methodological framework of the study, followed by results and discussion in the fourth section. The study will be concluded with policy implications and suggested future recommendations in the last section.
The seminal work of Wackernagel et al. (1999) motivated the researcher’s attention toward the ecological footprint as their study analyzed the ecological traces of 52 countries worldwide. Afterward, Wu et al. (2013) and Ying et al. (2012) explored the environmental hazards by taking the ecological footprint as an indicator in two provinces of China. Similarly, Hubacek et al. (2009) and Peng et al. (2019) are some of the prominent early contributions to the literature on ecological footprint under the scenario of China.
The most recent study by Zhang et al. (2022) focused on the non-linear association between green investment, economic growth, natural resources, and green innovation with the ecological footprint of China by applying the NARDL estimation method. Observing the increasing pattern of ecological footprints in China, the motivation of the study is based on the enhancement of green development to control the destruction of the environment. Limited data from 2000 to 2018 have been considered for concluding the research. The results of NARDL revealed that China should opt for policies favoring green development in the country, as economic progress with the exploitation of natural resources and misuse of energy consumption is adversely affecting the environment. Another study by Afshan and Yaqoob (2022) employed the Quantile Autoregressive Distributive Lag (QARDL) model to observe the conditional distribution of ecological footprint by taking eco-innovation, economic growth, natural resource rent, and financial development as covariates. The annual data from 1995 to 2017 have been considered in the study. As a principal emitter of greenhouse gas emissions, China observed a comprehensive picture of the ecological footprint at several quantiles. Results revealed that natural resource rent poses a positive while financial development negatively impacts China’s ecological footprints. The U-shaped inverted relationship was also validated through the economic growth variable. Overall, the modeling strategy provides fruitful insights into exploring the nexus between independent variables and conditional quantiles of environmental degradation. Furthermore, considering the BRICS nations, Lin and Wang (2020) studied the nexus between natural resource rent, renewable energy, and urbanization with ecological footprint through a panel data setting. The empirical results portrayed the positive impact of renewable energy consumption, natural resource rent, and urbanization on the quality of the environment.
After the efforts of Grossman and Krueger (1991) in testing the EKC hypothesis, numerous studies have validated it for various countries. Taking the economic growth and natural resources as a determinant of ecological footprint, Hassan et al. (2019) studied the validity of EKC for the emerging economy of Pakistan. The conventional time series ARDL model has been deployed to scrutinize the long- and short-run dynamics between the variables on yearly data from 1970 to 2014. The study's outcomes affirm the EKC hypothesis, and bidirectional causality has also been observed. Moreover, natural resources leave a positive effect on the environment’s quality. Likewise, Ahmed et al. (2020) considered the impact of natural resources, technological innovations, and growth of 22 emerging economies in the panel setting. The historical data on an annual basis from 1984 to 2016 were under consideration. The panel data technique of Cross-section Autoregressive Distributed Lag model (CS-ARDL) is employed for the desired objective of the study. Inclusively, the outcome concludes that technological innovation must be improved as it is impacted positively on the ecological footprint. Natural resources should be preserved through effective policy implications in the said regions. Moving towards the same list of variables, Ahmed et al. (2021) also included urbanization and globalization for G7 countries and concluded that urbanization exerts pressure on the environment. Utilizing the Cross-section Autoregressive Distributed Lag model (CS-ARDL), the EKC hypothesis has been confirmed for G7 countries. The outcomes of the study also recommended that globalization, promoting innovation policies, improving the urbanization structure, and hence progressing economically, become the significant components of sustainable development. Similarly, Erdoğan et al. (2021) assessed the impact of natural resource rent, globalization, urbanization, and human capital on the ecological footprint of 23 African countries and revealed that human capital and globalization positively impact the environment of the countries under study. In the same way, Danish & Khan (2019), Khan A. et al. (2020), and Zafar et al. (2019) are also prominent studies in the context of natural resources and ecological footprint. Regarding the green economic system, Saberi et al. (2018) assert that green innovation positively facilitates the environment while providing eco-friendly solutions (Cohen et al., 2016) for sustainable development. According to Song and Yu (2018), green innovation enhancement in production leads to sustainable development. Industries adopting green technology contribute to an efficient ecological economic system compared to traditional manufacturing methods (Sun et al., 2017). Thus, several studies like Priadi (2021) and Ji et al. (2021) encourage the implementation of the green innovation system to protect the environment from destruction.
The above-discussed literature possesses a few gaps that need to be filled. First, none of the previous studies have applied any advanced single-country methodology to observe environmental degradation. In contrast, the current research aims to deploy the novel statistical technique of bootstrap ARDL to access the dynamic association among the variables. Second, the impact of green investment was not enhanced more broadly. However, this study attempts to explain the long- and short-run effects of the covariates on the quality of the environment.
This study converted annual data into a quarter using a match-sum approach. This method is adopted to apply the bootstrap ARDL model and explore the dynamic linkages between ecological footprint, green innovation, renewable energy, natural resource rent, and economic growth. The data frequency ranges from 1990Q1 to 2020Q4. A detailed depiction of the included variables and sources is presented in Table 1.
The current study applies the bootstrap ARDL (Autoregressive Distributive Lag) estimation technique devised by McNown et al. (2018). The ARDL model of Pesaran and Shin (1999) is sensitive to sample size and power features, whereas the bootstrap ARDL effectively overcame the said issues. The novel method of bootstrap ARDL is an extended version of the conventional one as it is based on the robust estimation of F- and t-tests. It has also been observed that the ARDL model needs error correction terms to be significant along with the coefficients associated with lagged covariates (Pesaran et al., 2001). The significance of the error correction term depends on the order of integration, i.e., variables under study are restricted to be I (1); while testing the lagged coefficients of independent variables, the bounds are evaluated, but practically, both conditions cannot be fulfilled efficiently for every case. Goh et al. (2017) directed toward the concerns of the ARDL model and suggested that the bootstrap ARDL method increases the efficiency of F- and t-tests from innovative cointegration tests. Thus the reason behind the deployment of the bootstrap model is conclusive, i.e., it allows for analysis of the cointegration among the variables if they are not I (1) or even if they possess mixed order of integration (McNown et al., 2018). The following is the baseline model used to apply the bootstrap ARDL model:
where Yt is the predicted variable, whereas
The coefficients of the ECT model can be comprised function of
Once the hypothesis is formulated, the cointegration relationship between the independent variables can be established. The required three hypothesis statements are as follows: The first F-test (F1) employed on the ECTs parameters with the null hypothesis (Ho:
The second F-test applied to the coefficients of explanatory variables along with the hypothesis statement (Ho:
The third F-test is valid on the lagged values of the dependent variable with a null hypothesis (Ho:
The main ground to employ the bootstrap ARDL approach is that it provides a critical bounds test statistics of all the above-mentioned tests. At the same time, it brings out some robust estimates (McNown et al., 2018), which the traditional ARDL cannot make possible as it only gives an insight into the critical bound testing for F- and t-tests.
Before embarking on the formal cointegration test, the order of integration must be investigated. The study applied the ADF and ZA unit root tests with and without structural breaks in data. The outcomes of the test are stated in Table 2. It can be viewed that all variables are I (1), i.e., stationary at first difference. The outcomes are justified in applying the bootstrap ARDL methodology as the method is robust in capturing the structural breaks.
We move to the cointegration analysis now as variables get stationary at first difference. Employing the cointegration test, the dynamic association between renewable energy, natural resource rent, economic growth, and green innovation with the ecological footprint of China has been examined. Shahbaz et al. (2020) emphasized the use of bootstrap ARDL compared to ARDL since it offers the test statistics of F-test and t-test at all lags of the variables and lags of the dependent variable, respectively. Moreover, it also provides the innovative t value at the lags of the covariates, which is more robust in identifying the cointegration among the said variables. The lag selection criteria of Akaike Information Criteria (AIC) have been employed due to its high efficiency as bootstrap ARDL is sensitive to the lag orders (Lütkepohl., 2006). Table 3 presents the selected lag length and the cointegration results. The F- and t-test values are bootstrapped under the framework of analysis and are also reported in Table 3. The significant values of the F- and t-test indicate that the null hypothesis of no cointegration has been rejected at the significance level of 1% and concluded that renewable energy, natural resource rent, economic growth, and green innovation are independent. The innovative t-test retrieved at lags of covariates confirmed the cointegration among the variables. These outcomes appear to be similar to the findings of Zhang et al. (2022), who found non-linear cointegration among natural resource rent, green innovation, and economic growth with the ecological footprint of China. The test also finds the break date of the suggested model, i.e., 2007Q2. To validate the robustness of the model, diagnostic measures are also performed. The Jarque–Bera (JB) test supports the normality of the model residuals, the Langrange multiplier test (LM) confirms that the residuals are free from multicollinearity, while insignificant Q-stat portrays that model errors are homoscedastic.
The long-run assessment of the variables has been done as cointegration is confirmed. The outcomes are exhibited in Table 4. The long-run elasticities show that a 1% increment in natural resource rent and economic growth increases ecological footprint by 0.156% and 0.620%. In contrast, a 1% increase in renewable energy consumption and green innovation decreases ecological footprints by 0.102% and 0.272%. Furthermore, renewable energy consumption is found to be negative and significant, implying a negative association with the ecological footprint in China. This outcome is consistent with the study by Lin and Wang (2020) performed for BRICS nations. Similarly, the marginal effect of green innovation on environmental quality is negative as the associated coefficient appears negative and significant. The findings suggest that China should invest in green technology as the environmental distress is increasing due to the abusive treatment of natural resources. In the context of China, this result parallels the study of Zhong et al. (2022) and Alvarado et al. (2021). However, both natural resource rent and economic growth are positive and significant. It indicates that China is not utilizing its natural resources effectively. Nathaniel et al. (2021) found that misuse of natural resource rent results in environmental degradation in the BRICS region. In terms of economic growth, the findings are consistent with the study of Ahmed et al. (2020, 2021).
The short-run estimates obtained through bootstrap ARDL are presented in Table 5. It has been observed from the findings that a 1% increase in natural resource rent and economic growth increases ecological footprint by 0.093% and 0.380%; on the contrary, a 1% increase in renewable energy consumption and green innovation decreases ecological footprint by 0.058% and 0.075%. The signs of the coefficients appear to be similar to long-run estimates, though the magnitude of the coefficient is less than that of the long-run findings. The outcomes reveal that green innovation and renewable energy are important determinants in decreasing the ecological footprint in China. Proceeding to more outcomes, it has been shown that the selected dummy variable is also significant, suggesting the dynamic impact of the break date on the ecological footprints of China.
The coefficient of the speed of adjustment associated with the error correction term is found to be negative and significant. It can be seen that the coefficient is 0.368 indicating that the equilibrium adjustment, in the long run, can be adjusted by 36.8% annually. The ECM value also confirms the equilibrium relationship among the variables. This analysis is novel as the traditional time series model allows the establishment of the relationship between the variables after ignoring the previous year’s effect, which harms the efficiency of the current year’s estimates. It has also been considered that current ecological footprints depend upon past lag; thus, the lag effect concerning time is important to incorporate in the analysis to avoid the overestimation of the coefficients. The overall diagnostic tests are applied, and the results are reported in Table 6. The model residuals do not suffer from heteroscedasticity, autocorrelation, and multicollinearity problem, while the CUSUM test shows stable results.
The study aims to analyze the influence of green innovation, renewable energy, natural resource rent, and economic growth on the ecological footprint of China. The quarterly data spanning from 1990Q1 to 2020Q4 have been retrieved for the empirical analysis. Efforts of work have been done to explore the significant determinants in explaining their effects on the ecological footprint in the scenario of China. The novel technique of bootstrap ARDL has been employed to fulfill the objectives of the study. The order of integration of the variables under study has been confirmed using unit root test with and without structural breaks. Both tests confirm that variables are I (1); thus, the analysis moves toward the quest of cointegration. Overall, the model confirms the cointegration among the variables at the break date of 2009Q3. Green innovation and renewable energy possess negative and significant effects, while growth and energy positively impact China’s ecological footprint. The error correction term is negative and significant, whereas the break dummy is also significant. Overall, the bootstrap ARDL technique supports the testing hypothesis of the study.
Understanding the importance of the outcomes revealed by the study, several implications can be explained in the context of China. As a developing country, China should reinforce its links with other emerging economies and promote its investment direction to renewable energy and green innovation. The Chinese government may take actions to foster their industries regulated for green technology instead of carbon-releasing conventional processes. Moreover, the policies must be designed to achieve long-term benefits in China with the desirable improvement of ecological traces within the country. China should transform its development strategy headed toward a carbon-free zone. Thus, the result obtained from the dynamic relationship appears to be valuable for the policymakers to improve the quality of the environment in China.
Although the comprehensive outcomes are acquired in the current study, there are still few limitations. First, the study is a single-country analysis, and environmental degradation is not a matter of a single country. Thus, taking more countries with the same variables can be accessed in either panel data setting or sophisticated econometric modeling methods. Second, the historical data might be more useful in generating insights related to ecological footprints. Lastly, more independent variables concerning economic, financial, and political aspects seem to be productive in explaining the distributional assumptions of the ecological footprint.
The original contributions presented in the study are included in the article/Supplementary Material; further inquiries can be directed to the corresponding authors.
DJ: conceptualizing, writing, and original draft preparation. HS: data collection and empirical estimations. YF: conceptualizing, review, and editing. YD: data collection and empirical estimations. HL: Reviewing and editing.
The authors thank the financial support from the National Statistical Science Research Project (2022LY067).
The authors declare that the research was conducted in the absence of any commercial or financial relationships that could be construed as a potential conflict of interest.
All claims expressed in this article are solely those of the authors and do not necessarily represent those of their affiliated organizations, or those of the publisher, the editors, and the reviewers. Any product that may be evaluated in this article, or claim that may be made by its manufacturer, is not guaranteed or endorsed by the publisher.
Afshan, S., and Yaqoob, T. (2022). The potency of eco-innovation, natural resource and financial development on ecological footprint: A quantile-ARDL-based evidence from China. Environ. Sci. Pollut. Res. 1–11. doi:10.1007/s11356-022-19471-w
Ahmad, M., Jiang, P., Majeed, A., Umar, M., Khan, Z., and Muhammad, S. (2020). The dynamic impact of natural resources, technological innovations and economic growth on ecological footprint: An advanced panel data estimation. Resour. Policy 69, 101817. doi:10.1016/j.resourpol.2020.101817
Ahmad, M., Jiang, P., Murshed, M., Shehzad, K., Akram, R., Cui, L., et al. (2021). Modelling the dynamic linkages between eco-innovation, urbanization, economic growth and ecological footprints for G7 countries: Does financial globalization matter? Sustain. Cities Soc. 70, 102881. doi:10.1016/j.scs.2021.102881
Alvarado, R., Tillaguango, B., Dagar, V., Ahmad, M., Işık, C., Méndez, P., et al. (2021). Ecological footprint, economic complexity and natural resources rents in Latin America: Empirical evidence using quantile regressions. J. Clean. Prod. 318, 128585. doi:10.1016/j.jclepro.2021.128585
Baloch, M. A., Zhang, J., Iqbal, K., and Iqbal, Z. (2019). The effect of financial development on ecological footprint in BRI countries: Evidence from panel data estimation. Environ. Sci. Pollut. Res. 26 (6), 6199–6208. doi:10.1007/s11356-018-3992-9
Chen, Y., Jayaprakash, C., and Irwin, E. (2012). Threshold management in a coupled economic-ecological system. J. Environ. Econ. Manag. 64 (3), 442–455. doi:10.1016/j.jeem.2012.04.003
Cohen, M. C., Lobel, R., and Perakis, G. (2016). The impact of demand uncertainty on consumer subsidies for green technology adoption. Manag. Sci. 62 (5), 1235–1258. doi:10.1287/mnsc.2015.2173
Danish, U. R., and Khan, S. U. D. (2019). Determinants of the ecological footprint: Role of renewable energy, natural resources, and urbanization. Sustain Cities Soc. 54, 101996.
Erdoğan, S., Çakar, N. D., Ulucak, R., and Kassouri, Y. (2021). The role of natural resources abundance and dependence in achieving environmental sustainability: Evidence from resource-based economies. Sustain. Dev. 29 (1), 143–154.
Goh, S. K., Yong, J. Y., Lau, C. C., and Tang, T. C. (2017). Bootstrap ARDL on energy-growth relationship for 22 OECD countries. Appl. Econ. Lett. 24 (20), 1464–1467. doi:10.1080/13504851.2017.1284980
Grossman, G. M., and Krueger, A. B. (1991). Environmental impacts of a North American free trade agreement.
Hassan, S. T., Xia, E., Khan, N. H., and Shah, S. M. A. (2019). Economic growth, natural resources, and ecological footprints: Evidence from Pakistan. Environ. Sci. Pollut. Res. 26 (3), 2929–2938. doi:10.1007/s11356-018-3803-3
Hubacek, K., Guan, D., Barrett, J., and Wiedmann, T. (2009). Environmental implications of urbanization and lifestyle change in China: Ecological and water footprints. J. Clean. Prod. 17 (14), 1241–1248. doi:10.1016/j.jclepro.2009.03.011
Ji, X., Umar, M., Ali, S., Ali, W., Tang, K., and Khan, Z. (2021). Does fiscal decentralization and eco-innovation promote sustainable environment? A case study of selected fiscally decentralized countries. Sustain. Dev. 29 (1), 79–88. doi:10.1002/sd.2132
Johnsson, F., Kjärstad, J., and Rootzén, J. (2019). The threat to climate change mitigation posed by the abundance of fossil fuels. Clim. Policy 19 (2), 258–274. doi:10.1080/14693062.2018.1483885
Jun, S., Zhilong, T., and Qing, X. (2015). How does government make policies for strategic emerging industry? Forum on Science and Technology in China.
Khan, A., Chenggang, Y., Hussain, J., Bano, S., and Nawaz, A. (2020b). Natural resources, tourism development, and energy-growth-CO2 emission nexus: A simultaneity modeling analysis of bri countries. Resour. Policy 68, 101751. doi:10.1016/j.resourpol.2020.101751
Khan, K., Su, C. W., and Khurshid, A. (2022). Do booms and busts identify bubbles in energy prices? Resour. Policy 76, 102556. doi:10.1016/j.resourpol.2022.102556
Khan, M. A., Khan, M. A., Ali, K., Popp, J., and Oláh, J. (2020a). Natural resource rent and finance: The moderation role of institutions. Sustainability 12 (9), 3897. doi:10.3390/su12093897
Kim, D., and Perron, P. (2009). Unit root tests allowing for a break in the trend function at an unknown time under both the null and alternative hypotheses. J. Econ. 148 (1), 1–13. doi:10.1016/j.jeconom.2008.08.019
Lin, B., and Wang, M. (2020). How to boost energy productivity in China's industrial sector: An integrated decomposition framework based on multi-dimensional factors. J. Clean. Prod. 259, 120902. doi:10.1016/j.jclepro.2020.120902
Lütkepohl, H. (2006). Structural vector autoregressive analysis for cointegrated variables. Allg. Stat. Arch. 90 (1), 75–88.
Matuštík, J., and Kočí, V. (2021). What is a footprint? A conceptual analysis of environmental footprint indicators. J. Clean. Prod. 285, 124833.
McNown, R., Sam, C. Y., and Goh, S. K. (2018). Bootstrapping the autoregressive distributed lag test for cointegration. Appl. Econ. 50 (13), 1509–1521. doi:10.1080/00036846.2017.1366643
Nathaniel, S. P., Yalçiner, K., and Bekun, F. V. (2021). Assessing the environmental sustainability corridor: Linking natural resources, renewable energy, human capital, and ecological footprint in BRICS. Resour. Policy 70, 101924. doi:10.1016/j.resourpol.2020.101924
Peng, B., Li, Y., Elahi, E., and Wei, G. (2019). Dynamic evolution of ecological carrying capacity based on the ecological footprint theory: A case study of jiangsu province. Ecol. Indic. 99, 19–26. doi:10.1016/j.ecolind.2018.12.009
Pesaran, M. H., Shin, Y., and Smith, R. J. (2001). Bounds testing approaches to the analysis of level relationships. J. Appl. Econ. 16 (3), 289–326. doi:10.1002/jae.616
Priadi, R. (2021). Enhancing sustainable ecological footprints through climate funds, green technology investments and research and development EXPENDITURE: A panel data analysis of asean countries. KUMPULAN BERKAS KEPANGKATAN DOSEN.
Saberi, S., Cruz, J. M., Sarkis, J., and Nagurney, A. (2018). A competitive multiperiod supply chain network model with freight carriers and green technology investment option. Eur. J. Operational Res. 266 (3), 934–949. doi:10.1016/j.ejor.2017.10.043
Salah, E. H., and El-Haggar, P. E. (2007). Sustainability of agricultural and rural waste management. Sustain. Ind. Des. Waste Manage, 223–260.
Shahbaz, M., Raghutla, C., Song, M., Zameer, H., and Jiao, Z. (2020). Public-private partnerships investment in energy as new determinant of CO2 emissions: The role of technological innovations in China. Energy Econ. 86, 104664. doi:10.1016/j.eneco.2020.104664
Song, W., and Yu, H. (2018). Green innovation strategy and green innovation: The roles of green creativity and green organizational identity. Corp. Soc. Responsib. Environ. Mgmt. 25 (2), 135–150. doi:10.1002/csr.1445
Su, C. W., Xie, Y., Shahab, S., Faisal, C., Hafeez, M., Qamri, M., et al. (2021). Towards achieving sustainable development: Role of technology innovation, technology adoption and CO2 emission for BRICS. Ijerph 18 (1), 277. doi:10.3390/ijerph18010277
Sun, L. Y., Miao, C. L., and Yang, L. (2017). Ecological-economic efficiency evaluation of green technology innovation in strategic emerging industries based on entropy weighted TOPSIS method. Ecol. Indic. 73, 554–558. doi:10.1016/j.ecolind.2016.10.018
Sun, Y., Anwar, A., Razzaq, A., Liang, X., and Siddique, M. (2022d). Asymmetric role of renewable energy, green innovation, and globalization in deriving environmental sustainability: Evidence from top-10 polluted countries. Renew. Energy 185, 280–290. doi:10.1016/j.renene.2021.12.038
Sun, Y., Guan, W., Razzaq, A., Shahzad, M., and Binh An, N. (2022b2022). Transition towards ecological sustainability through fiscal decentralization, renewable energy and green investment in OECD countries. Renew. Energy 190, 385–395. doi:10.1016/j.renene.2022.03.099
Sun, Y., and Razzaq, A. (2022). Composite fiscal decentralisation and green innovation: Imperative strategy for institutional reforms and sustainable development in OECD countries. Sustain. Dev. 1–14. doi:10.1002/sd.2292
Sun, Y., Razzaq, A., Sun, H., and Irfan, M. (2022c2022). The asymmetric influence of renewable energy and green innovation on carbon neutrality in China: Analysis from non-linear ARDL model. Renew. Energy 193, 334–343. doi:10.1016/j.renene.2022.04.159
Umar, M., Ji, X., Kirikkaleli, D., and Alola, A. A. (2021). The imperativeness of environmental quality in the United States transportation sector amidst biomass-fossil energy consumption and growth. J. Clean. Prod. 285, 124863. doi:10.1016/j.jclepro.2020.124863
Wackernagel, M., Onisto, L., Bello, P., Callejas Linares, A. C., Susana López Falfán, I. S. L., Méndez Garcı́a, J. M., Isabel Suárez Guerrero, M. G. S., and Guadalupe Suárez Guerrero, M. (1999). National natural capital accounting with the ecological footprint concept. Ecol. Econ. 29 (3), 375–390. doi:10.1016/s0921-8009(98)90063-5
Wang, L., Su, C. W., Ali, S., and Chang, H. L. (2020). How China is fostering sustainable growth: The interplay of green investment and production-based emission. Environ. Sci. Pollut. Res. 27 (31), 39607–39618. doi:10.1007/s11356-020-09933-4
Wu, Z., Shi, X., Shi, Q., and Pan, X. (2013). The effect of nighttime temperature increase on the photosynthesis and activity of protective enzyme of different early indica rice varieties at filling stage. Acta Agric. Univ. Jiangxiensis 35 (6), 1119–1125.
Ying, L., Guo, T. T., and Li, P. P. (2012). Study on calculation of ecological footprint model parameters of beijing urban water resources. Adv. Mater. Res. 374. Trans Tech Publications Ltd, 105–108.
Zafar, M. W., Zaidi, S. A. H., Khan, N. R., Mirza, F. M., Hou, F., and Kirmani, S. A. A. (2019). The impact of natural resources, human capital, and foreign direct investment on the ecological footprint: The case of the United States. Resour. Policy 63, 101428. doi:10.1016/j.resourpol.2019.101428
Zhang, H., Shao, Y., Han, X., and Chang, H. L. (2022). A road towards ecological development in China: The nexus between green investment, natural resources, green technology innovation, and economic growth. Resour. Policy 77, 102746. doi:10.1016/j.resourpol.2022.102746
Keywords: renewable energy, green innovation, natural resources, climate change, bootstrap ARDL, China
Citation: Jiang D, Shu H, Fan Y, Dong Y and Li H (2022) Influence of renewable energy and natural resources on climate change: The role of green innovation in China. Front. Environ. Sci. 10:966656. doi: 10.3389/fenvs.2022.966656
Received: 11 June 2022; Accepted: 09 September 2022;
Published: 10 October 2022.
Edited by:
Asif Razzaq, Ilma University, PakistanReviewed by:
Najia Saqib, Prince Sultan University, Saudi ArabiaCopyright © 2022 Jiang, Shu, Fan, Dong and Li. This is an open-access article distributed under the terms of the Creative Commons Attribution License (CC BY). The use, distribution or reproduction in other forums is permitted, provided the original author(s) and the copyright owner(s) are credited and that the original publication in this journal is cited, in accordance with accepted academic practice. No use, distribution or reproduction is permitted which does not comply with these terms.
*Correspondence: Haiwei Li, bGlod2huQHRqY3UuZWR1LmNu
Disclaimer: All claims expressed in this article are solely those of the authors and do not necessarily represent those of their affiliated organizations, or those of the publisher, the editors and the reviewers. Any product that may be evaluated in this article or claim that may be made by its manufacturer is not guaranteed or endorsed by the publisher.
Research integrity at Frontiers
Learn more about the work of our research integrity team to safeguard the quality of each article we publish.