Corrigendum: What affects China’s high-tech industry land misallocation: Government behavior or enterprise behavior?
- 1School of Spatial Planning and Design, Zhejiang University City College, Hangzhou, China
- 2School of Public Administration, Zhejiang University of Finance and Economics, Hangzhou, China
- 3School of Global Studies, Tokai University, Hiratsuka, Japan
- 4TOKAI Research Institute for Environment and Sustainability (TRIES), Hiratsuka, Japan
The high-tech industry belongs to the core strategic national development category and is essential in promoting social future economic growth and scientific and technological progress. This paper constructs a theoretical framework for high-tech industry land misallocation based on the four-level institutional analysis method. The study selects Zhejiang Province of China as the research area. It uses the literature research method and multiple regression analysis methods to analyze the root causes and influence mechanism of land misallocation for the high-tech industry. The research shows that the land-use support policies for the high-tech industry can effectively alleviate the misallocation of land factors. In contrast, the government’s improper financial support measures and tax incentives increase the degree of land misallocation in the high-tech industry. The higher the R&D expenditure-to-income ratio and profitability, the lesser the land misallocation in the high-tech industry. Government behavior and enterprise behavior impact land misallocation in the high-tech industry, but government behavior has a major impact. Therefore, the government, enterprises, and the market should adopt an integrated strategy to reduce excessive government intervention in land resource allocation, allowing the market to play a decisive role in resource allocation, and stimulate the vitality of enterprise R&D and innovation. This study helps to reveal the deep motivation of land misallocation for high-tech industry and provides practical guidance for optimizing the high-tech industrial land allocation and promoting high-quality industrial development.
Introduction
The coronavirus disease 2019 (COVID-19) epidemic has delayed the return of personnel to work, caused a short-term impact on the supply side, and triggered fluctuations in the global supply chain, and the industry is facing the challenges of transformation and upgrading. The most crucial factor in industrial transformation and upgrading is agglomerating resource elements to the advanced technology industry. The high-tech industry is characterized by intelligence, innovation, and strategy, which can promote the production of intermediate products, increase the localization rate, and enhance the core competitiveness of the industry (Buhr et al., 2021). Countries worldwide are making the development of high-tech industries an important national development strategy (Zhang, 2020). The rational allocation of factors such as land, capital, and labor are important to the development of high-tech industries (Pruchnow et al., 2006). China’s high-tech industry has always maintained a healthy development trend (Novikov, 2019), which is seen as continuous growth in scale, continuous improvement in technical capacity (Tung and Yu, 2016), continuous optimization of layout, etc. However, it is also facing a decline in the growth rate, weak core technology capacity, insufficient utilization of cluster advantages, and other challenges (Novikov, 2019). The high-tech industry not only reflects the achievements of China’s cutting-edge technological innovation but also helps to achieve sustained economic growth in China in the future.
In 2018, the number of high-tech industry enterprises in China increased from 28,200 in 2010 to 33,600, and the main business revenue increased from 7,448.28 billion yuan in 2010 to 15,7001 billion yuan; its scale of high-tech industry output ranked second in the world, and its scale of exports ranked first in the world [calculated based on the World Input-Output Database (WIOD)]. For high-tech enterprises in need of key support, the enterprise income tax rate is reduced to 15%, and all levels of government pay great attention to the development of high-tech industries. The development of industries needs to be arranged on land (Sun et al., 2020). Due to the urbanization process, the land system in China shows a clear tendency toward industrialization, which promotes the extensive industrial use of land. There is also a significant structural imbalance in China’s industrial land supply. The proportion of the land supply available for emerging industries and high-tech industries is relatively low (Wang and Wang, 2020), and the phenomena of idle land and inefficient land use are prominent (Guanzhong and Yi, 2015). High-tech industries have strategic significance for national and regional development (Liang and Fan, 2020). Therefore, due to the dual intervention of the central and local governments, high-tech industrial land has been more seriously misallocated than traditional industrial land (Huang and Du, 2017; Guo, 2019).
Academia is increasingly concerned about the impact of land allocation on industrial development, mainly from the perspective of government-led or market-led policies (Wang et al., 2020a; Tsvetkova et al., 2020), to study the root causes (Lu et al., 2021), influencing factors (Liang et al., 2020), measurement methods (Chen et al., 2020), governance models (Adler et al., 2019), and governance strategies (Liu et al., 2018a) of land misallocation in high-tech industries. First, in terms of the root causes of the misallocation, domestic and foreign scholars believe that the main reasons for the misallocation of high-tech industrial land are market failure (Wang et al., 2017), government failure (Zeng, 2019), imperfect property rights system (Haohan et al., 2019), and local government competition (Coman and Ronen, 2007). The difference between domestic and foreign academics is that some foreign studies only consider land as a part of the capital, and the land misallocation problem in developed countries is not so prominent (Chen and Kangping, 2018). However, the uniqueness of China’s system determines the direction of industrial policy and the government’s dominant position in the land factor allocation, which makes land misallocation for high-tech industries relatively serious. Subsequently, land misallocation has become a key issue hindering the development of high-tech industries (Lu et al., 2022). Studies of the root causes of land misallocation for high-tech industries have been conducted from the perspectives of the government and the market and have explored the land factor misallocation caused by improper government intervention in the market and the defects of spontaneity, blindness, and lag in the market itself (Zhao, 2019; Kong et al., 2021). Second, in terms of influencing factors, the influencing factors mainly include institutional factors (Sick et al., 2019), socioeconomic factors (Yu et al., 2020), location factors (Liu et al., 2018a), and natural endowment factors (Lu et al., 2020a). Third, in terms of study methods, scholars mainly focus on the evaluation of land use efficiency, land conservation, and intensive use. Their methods mainly include input–output method (Bryan et al., 2015), DEA method (Han and Zhang, 2020), SFA method (Liu et al., 2020a), and evaluation method based on index system (Zheng et al., 2019). The measurement method of land misallocation in high-tech industries can learn from the measurement and evaluation method of resource allocation efficiency. Finally, from the perspective of governance models and strategies, they mainly include government-led governance models; market-led governance models; mixed governance models; and strategies such as clarifying the property rights of allocation, improving the institutional environment of allocation, correcting the distortion of allocation policies, and improving the management of allocation planning. In a word, there is a consensus regarding the existence of land misallocation. However, existing studies have paid relatively little attention to the issue of land misallocation in high-tech industries. At the same time, from the perspective of research, the existing studies focus on the perspective of allocation efficiency and agglomeration effects and lack research on the high-tech industry from the perspective of land misallocation. From the perspective of research content, the existing studies focus on the contribution of land factor investment to economic growth at the macro level, and the research on the mechanism of land misallocation in high-tech industries is relatively scarce. Research at the micro level, especially at the enterprise level, is mostly ignored. Empirical tests are relatively lacking.
The contribution of this study lies in that this study breaks through the existing research on utilization efficiency and an agglomeration perspective and conducts research based on the perspective of microenterprise total factor productivity. Theoretically, this study enriches and perfects the research on the optimal allocation model of high-tech industrial land. In practice, it provides practical guidance for optimizing the allocation of land for high-tech industries and promoting the high-quality development of industries. This study aims to use the high-tech enterprises in Zhejiang Province as the research object and, from the viewpoints of government behavior and enterprise behavior, to explore the impact mechanism and the root causes of land misallocation for the high-tech industry. To this end, it employs literature review methods and multivariate regression analysis to seek optimization strategies for land allocation in the high-tech industry. This study will provide a scientific basis and decision-making reference for the intensive and efficient use of industrial land, industrial transformation and upgrading, and the high-quality development of regional economies. The following section presents the theoretical basis and literature review. Then, the theoretical analysis framework for the study of land misallocation in the high-tech industry is presented. The Model and data section proposes hypotheses and constructs analytical models. In the Results and discussion section, the empirical results are discussed. The final section is the conclusion of this study.
Theoretical basis and literature review
Resource misallocation theory
Modern microeconomics emphasizes that resource allocation plays a key role in achieving efficiency and that improper resource allocation is an essential source of differences in productivity among countries (Liu et al., 2020b). Jones proposed an input–output economy with a Cobb–Douglas production function and consumption function and a distortionary wedge (Yin and Zhu, 2020). Bigio and La’O expanded on this by proposing input–output economies with Cobb–Douglas production and consumption functions with distortionary wedges and an elastic labor supply (JonesMisallocation, 2013). When an economy is distorted, meaning that the marginal product of inputs of each production unit is not equal, there will be resource misallocation. “Resource misallocation” refers to the existence of external factors that cause obstacles to the flow of resource elements, thus causing the unequal marginal product of resources, i.e., deviation from the Pareto optimality (Chen et al., 2019). Studies on resource misallocation in China mainly focus on the nature, connotations, form, cause, and impact of resource misallocation. Since China’s economic growth is mainly achieved through the promotion of industrialization, the industrial sector forms the core of China’s economy. Its output value has been maintained at approximately 50% of the total gross domestic product (GDP) (Lu et al., 2020b), and the high-tech industry is the pillar of China’s economy. Research on land resource misallocation in high-tech industries is the key to deftly promoting industrialization and ultimately achieving industrial transformation and upgrade in China. There are two main types of resource misallocation. One is misallocation in the intensive margin, in which the marginal return on resources is not equal across sectors; the other is misallocation in the extensive margin, in which the marginal output of all enterprise factors in the economy is equal, but the reallocation of factors could still lead to output increases (Yang and Shao, 2018).
Connotation of land misallocation in the high-tech industry
The earliest definition of high-tech industry describes it as a large-scale industry that uses cutting-edge technology and cutting-edge equipment (Banerjee and Moll, 2010). The high-tech industries addressed in this study are modern urban industries that meet the national classification standards. High-tech industry land includes the land used by units that meet the national high-tech industry classification and are recognized as high-tech enterprises or high-tech industrial parks by governments at all levels. Its output value is usually higher than that of general industries. From the perspective of economics, if production factors are not effectively allocated, and high productivity enterprises cannot get enough factor input, the overall productivity of the economy will be affected, and the resulting efficiency loss is called resource misallocation (Sun and Lv, 2020). At present, scholars at home and abroad have not formed a precise definition of the land misallocation of high-tech industries. Referring to the concept of “resource misallocation” (Lu et al., 2021), the land misallocation of high-tech industries is that the flow of factors is blocked, resulting in the deviation of the marginal output of resources from the Pareto optimal state. Due to the different levels of factor marginal output in different departments or regions, the actual allocation of high-tech industrial land will deviate from the optimal allocation equilibrium point under the intervention of the government and the market. This leads to the reduction of the output efficiency of high-tech industries, forming a certain output gap. The greater the difference of factor marginal output level in different departments or regions, the greater the degree of deviation from the optimal allocation, and the more serious the misallocation phenomenon. Therefore, under the influence of excessive government intervention and market failure, the allocation of high-tech industrial land by the main body of resource allocation deviates from the principle of fairness, resulting in the imbalance of the allocation of capital, labor, land, and other factors in various departments and regions (Li and Wang, 2021). The supply and demand of land for high-tech industries are in an unbalanced state, resulting in some high-tech industries in some regions being unable to develop due to the lack or backwardness of factor resources.
This study assumes that over a fixed period of time, the total area of industrial land is fixed, denoted by L; the industrial land is allocated to two specific sectors (enterprises), which are recorded as sector A and sector B, and their decision-making behavior is in line with the concept of the economic man. Under the same level of industrial land supply, the marginal benefit functions of industrial land for sector A and sector B are F1 and F2, respectively.
As shown in Figure 1, the marginal benefit functions F1 and F2 are placed in a box plot. The horizontal axis L′L represents the amount of industrial land allocated for sector A, and L″L represents the amount of industrial land allocated for sector B. Effective allocation should achieve equal marginal revenue (i.e., L* points). The two marginal benefit curves are divided into eight parts, denoted as M, M2, M1, Q1, Q2, Q3, Q4, and N. Under the condition of effective allocation, the economic output is expressed as:
Study review of land misallocation in the high-tech industry
Through combing the literature, it is found that foreign scholars began to study the high-tech industry in 1984. In order to restore the vitality of economic development after the 2008 financial crisis, the world’s economies paid more attention to the high-tech industry, which is a high-growth industry. They issued more supporting policies to promote its development. Foreign scholars’ study on land misallocation began in 1979, and its popularity has been growing slowly for a long time. The main reason for this is that foreign countries started early in the study of land resource utilization, and the development system is more perfect. Therefore, in the actual process of industrial growth, there is less large-scale and eye-catching industrial land misallocation. Domestic study on land use efficiency and high-tech industry began in 1984. Under the tide of industrial structure adjustment caused by the world’s new technological revolution, China has paid increasing attention to high-tech industrial development zones. Domestic research on high-tech industry reached its peak in 1999 and then showed a fluctuating downward trend. The study on land use efficiency has been growing slowly from the beginning to 2018. And as the national society pays more attention to the efficiency of industrial land use, it is expected that the efficiency of land use will become a research hotspot in the future.
Existing studies mainly focus on the root causes (Liu et al., 2018b; Yan, 2018; Agheyisi, 2019), influencing factors (Li et al., 2017; Chen et al., 2022), measurement methods (Zeng et al., 2019; Wang et al., 2020b; Wang and Tan, 2020), governance strategies (Ma and Zhao, 2015), and other aspects of land misallocation in high-tech industries. The root causes of land misallocation in high-tech industries can be summarized into five aspects. First, the imperfect land factor market (Agheyisi, 2019). For example, restrictions on transfer or lease will increase the transaction costs of business entities in production activities and hinder the flow of land elements to entities that use more efficiently. Second, excessive government intervention in the land market and market failure caused by monopoly of land supply are important reasons for the low efficiency of land allocation in high-tech industries (Agheyisi, 2019). The third is the imperfect property right system (Yan, 2018). Fourth, land regulation is one of the reasons for the imbalance between marginal income and cost of land (Yan, 2018). The fifth is the competition of local governments under the reform of tax sharing system (Liu et al., 2018b). The influencing factors of high-tech industrial land misallocation can be divided into institutional factors (Li et al., 2017), socioeconomic factors (Li et al., 2017), location factors (Chen et al., 2022), natural endowment factors (Chen et al., 2022), and so on. Among the institutional influencing factors, the existing studies mainly focus on the impact of institutional changes, land supply system reform, industrial land use system, and industrial policy. The influence of socioeconomic factors is mainly reflected in the impact of the degree of economic development and the imbalance of economic development on the space–time difference of high-tech industrial land allocation. Due to the great differences in the degree of regional economic development, the subsequent development opportunities are also different; therefore, the location factor is also an important reason. Natural endowment factors also have a certain degree of impact. Among them, many studies have pointed out that population changes, income levels and affluence, and technological progress are important influencing factors. Since the theoretical basis of high-tech industrial land misallocation is the theory of resource misallocation, the measurement method of high-tech industrial land allocation efficiency can learn from the measurement and evaluation method of resource allocation efficiency. There are mainly marginal output differences or productivity share covariance, which is the method of calculating the marginal efficiency output of the research object by constructing a covariance model (Zeng et al., 2019); using the relative productivity of factors, that is, restoring the relative productivity of factors through the calculation of the elasticity coefficient and distortion coefficient of production factors (Wang et al., 2020b) and based on the productivity gap caused by resource misallocation, that is, by calculating the distortion degree of resource misallocation, and then comparing it with the most efficient point, we can get the productivity gap (Wang and Tan, 2020). The optimal allocation of high-tech industrial land is committed to correcting the misallocation and improving the allocation efficiency by selecting and improving the governance structure. The misallocation of high-tech industrial land may be caused by the failure of government and market mechanism; therefore, the definition of the functional scope of government and market has become the governance core. The main modes of high-tech industrial land allocation governance include government governance mode, market governance mode, and mixed governance mode (Ma and Zhao, 2015).
Theoretical analysis framework for land misallocation in the high-tech industry
Four-level institutional analysis of Williamson’s framework
According to the institutional analysis theory of Williamson (2000), the roles of the four levels of social embeddedness, institutional environment, governance structure, and resource allocation are flipped from top to bottom. Social embeddedness (L1) has been changing for a long time, and its research scale is relatively macro. However, the scope of this study is limited to Zhejiang Province, and therefore, the impact of the first level is ignored at the institutional level. From the perspective of the institutional environment (L2), under the current fiscal decentralization system and in the face of regional competition and fiscal revenue and expenditure pressure, local governments tend to attract large capital-intensive or labor-intensive enterprises, and some traditional enterprises lose their vitality for development and become backward and inefficient. A large amount of inefficiently utilized industrial land cannot be put back into market transactions, which significantly reduces the land available for high-tech industry. The governance structure (L3) removes the transaction barriers that affect the normal operation of the market mechanism. The current allocation of high-tech industrial land is mainly affected by government behavior and enterprise behavior. The level of resource allocation (L4) manifests as government intervention in the normal land market through fiscal subsidies, preferential land supply, and other policy measures under the systems of fiscal decentralization and urban–rural division, resulting in marginal private costs that are lower than the marginal social costs for high-tech industry enterprises; consequently, high-tech enterprises have strong development motives. This level starts with marginal efficiency and is affected by the common influence of the first three institutional levels. The interaction among these levels is shown in Figure 2. However, the dual failure of the market and the government during the resource allocation process indicates that simply adjusting the price and quantity of land to improve the marginal efficiency of land use cannot correct the root cause of the misallocation problem. Therefore, it is necessary to explore the solution at a deeper level.
Theoretical framework of land misallocation for high-tech industry
Because the allocation of land for high-tech industry is affected by both government intervention and market failure (Ferrara et al., 2014), it is not ideal to explore this misallocation only from the perspective of efficiency allocation. To more clearly understand the mechanism of land misallocation for high-tech industry and clarify the interaction between land misallocation for high-tech industry and the behavior of government and enterprise, this study draws on the four-level institutional analysis of the Williamson framework and constructs a theoretical analysis framework for land misallocation in high-tech industries (Figure 3) according to the ideal of “institutional level—behavior model—transmission path—influence on outcome.”
First, changes in social embeddedness are slow and unpredictable. Therefore, only the second, third, and fourth levels are considered at the institutional level. The institutional environment is the structure that ensures the smooth operation of society, and it forms the basis for the allocation of industrial land. Governance structure represents implementation at the level of social institutions. Resource allocation includes production factors, pricing, and incentive adjustments. Currently, most studies on land allocation for high-tech industry focus on the fourth level of marginal analysis: resource allocation efficiency. Second, the behavioral model mainly analyses the behavior of government and enterprise in the land allocation process. In terms of government behavior, under the institutional environment of fiscal decentralization and urban–rural division, the government intervenes in the land market through policy governance measures such as fiscal subsidies and preferential land supply to attract more high-tech enterprises. From the perspective of enterprise behavior, the government’s financial subsidies and preferential policies cause the marginal private cost of high-tech enterprises to be smaller than the marginal social cost. Meanwhile, traditional inefficient enterprises have difficulty vacating, high-tech enterprises have difficulty entering, and the latter has poor operating conditions, which cause the distortion of land allocation for high-tech industries. Third, the transmission path analysis mainly explores how government behavior or enterprise behavior affects land misallocation for high-tech industries and the path through which they affect the efficiency of factor allocation. Insufficient land resource allocation for high-tech enterprises will result in the dispersion of the agglomeration of scientific research resources and production factors, which will increase the operating costs of enterprises. Excessive land resource allocation for high-tech enterprises leads to a large amount of capital being fixed in the land and unable to flow to more efficient sectors to create more value, thus creating a vicious circle. In addition, because the factors can be converted and circulated, enterprises can use the existing resources for mortgage loans to attract capital injection and can easily cover the land misallocation, which damages the development efficiency of other enterprises. Eventually, this causes misallocation in the high-tech industry.
Model and data
Theoretical assumptions
According to the theoretical analytical framework of land misallocation in the high-tech industry (Figure 3), government behavior is mainly reflected in the second and third levels. There is fierce competition among local governments, and land has become a tool that local officials use to develop the regional economy. In an institutional environment characterized by fiscal decentralization and urban–rural division, the local government, due to political promotional and fiscal incentives, excessively intervenes in the land market, causing severe distortions in the deviation of land prices from land values (Wei et al., 2019). High-tech industry enterprises exist in a distorted market environment. Therefore, there is a serious misallocation of land resources. In recent years, extensive land supply based on low land prices and the agreement-based transfer method has become the main means by which local governments attract investment. Based on this, this study proposes hypothesis 1: the greater the government intervention in high-tech industrial land, the more serious the land misallocation in the high-tech industry.
Enterprise behavior is mainly reflected in the fourth level. Local government competition for attracting investment in the land will lead to excessive investment by land-purchasing enterprises, which increases the degree of capital intensity but keeps employment absorption capacity and growth efficiency low (Huang and Du, 2017). Under solid government intervention, the marginal private cost of traditional industrial land is lower than the marginal social cost, and enterprise land has a relatively apparent negative externality. The spatial uniqueness of land resources leads to a minimal possibility for enterprises to exit after they have occupied the land while simultaneously increasing the cost of the land for new entrants and reducing the overall level of productivity, leaving enterprises in a dilemma. Based on this, this study proposes hypothesis 2: the more significant the negative externality of land use by high-tech enterprises, the greater the likelihood of land misallocation.
In fact, high-tech industrial land is allocated under the combined effect of macrocontrol and market mechanisms, and the study of misallocation should also consider these two aspects. First, the local government is both the land’s supply side and the macroregulator’s identity. Therefore, the local government has strong control over land allocation. Local government-led land allocation has a direct impact on the market demand for high-tech industrial land and restricts the signal transmission between price and supply and demand, which is the root cause of misallocation. Second, imperfect market mechanisms and incomplete regulatory functions directly impact enterprises’ unreasonable behavior, which in turn affects land allocation. In the game between the government and enterprises, the local government will provide land according to the demands of the land-using enterprises, and the land-using enterprises will determine the demand based on the land price. The vicious competition among local governments has severely depressed the price of industrial land. According to the theory of market supply–demand equilibrium, the decrease in land price will lead to an increase in the land demand of enterprises, which in turn promotes an increase in the supply of industrial land. Meanwhile, other investment promotion policies of local governments will also stimulate the land demand of high-tech enterprises, which may cause a large amount of land occupation and land hoarding behavior. This behavior prevents the enterprise’s own capital elements from circulating and hinders the entry of foreign high-tech enterprises. As a result, the allocation of land resource elements in high-tech industries is distorted. Based on this, this study proposes hypothesis 3: land misallocation in the high-tech industry is caused by both government behavior and enterprise behavior, but government behavior has a major impact on it.
Model construction
First, we examine whether government behavior impacts land misallocation in the high-tech industry. Hypothesis 1 is verified by examining the impact of indicators such as fiscal decentralization and fiscal subsidies on the explained variables. The model was established as follows:
In this model,
Second, we examine how enterprise behavior affects land misallocations in high-tech industries. To verify hypothesis 2, the model is established as follows:
In this model, the variables, asset–liability ratio (
Finally, the relationship between government behavior, enterprise behavior, and land misallocation in the high-tech industry was studied to clarify the underlying mechanism of land misallocation in high-tech industries. To verify hypothesis 3, the model is established as follows:
In this model, the first three variables, including the FIN, SUB, and POL, represent government behavior; the latter, including the ASS, PRO, and R&D, represent the behavior of high-tech industries.
Data description
The land misallocation in the high-tech industry is the core variable (i.e., dependent variable) to be explained in this study. Academia primarily uses the area of land leased by agreement/area of land leased through bidding or the average price of commercial land/average price of industrial land to characterize the degree of land misallocation, but the heterogeneity and differences among different industries are ignored. Based on misallocation theory, this study uses the traditional industrial land area/high-tech industrial land area ratio to express the core variable of land misallocation in high-tech industry. Government behavior and enterprise behavior are explanatory variables (i.e., independent variables). In the analysis of government behavior, the government behavior variables are measured using indicators such as fiscal decentralization, fiscal subsidies, and high-tech industrial land-use policy. In the analysis of enterprise behavior, high-tech enterprises’ operational management and revenue interact with the land misallocation in the high-tech industry (Liu et al., 2018a). Therefore, the enterprise behavior variables are measured using the ASS, PRO, R&D, and other indicators. For high-tech industry, the city’s economic development trend is the foundation of industrial development, and the quantity and quality of talent determine the speed of future development. Therefore, the control variables mainly include two aspects. One is the level of city development, including net population inflow, urban population density, and prefecture-level city GDP; the other is the supply of talent and technological innovation, including the number of college graduates and granted patents. The selection and composition of specific indicators are shown in Table 1.
Zhejiang Province plays an important role in the integration strategy of the Yangtze River Delta. The headquarters of high-tech enterprises with global influence, such as Alibaba and Ant Group, are in Zhejiang Province. Zhejiang Province has successively introduced a number of policies to support the development of high-tech enterprises. With the support of national strategic materials, Zhejiang Province will build a new science and technology city with scientific and technological innovation as its core and high-quality industrial development as its goal and will strive to explore development with specific characteristics. This has become a reproducible and promotable model development case. Although the high-tech industry in Zhejiang Province has developed rapidly, there is still a misallocation problem in the land for high-tech industries. From the perspective of factor input level (Figures 4, 5), the capital factor input has increased significantly, with a growth rate as high as 120% from 2014 to 2018, while the growth rate of the labor factor input and land factor input increased relatively less, only by 4% and 26% in 5 years, respectively. In terms of the output of high-tech industries, the province’s growth rate was 98%. Therefore, the output of high-tech industry enterprises in Zhejiang Province does not match the resource input, and the misallocation of labor factors and land factors is the most serious. This is not conducive to the long-term development of high-tech industry enterprises and society. According to the China Statistics Yearbook on High Technology Industry, Zhejiang Province’s high-tech industry investment in 2018 increased by 39.15% compared to 2015, the main business income increased by 41.70%, and the total profit increased by 45.36% (Figure 6). With the rapid development of the high-tech industry, a large number of high-efficiency and high-tech enterprises have emerged. In 2018, the number of high-tech enterprises in Zhejiang Province reached 2,785. Since inefficient, traditional enterprises did not establish an effective exit mechanism at the initial stage of their establishment, the environment of survival of the fittest created a dilemma that resulted in a prominent phenomenon of industrial land-use distortion. Therefore, it is typical to choose Zhejiang Province as the study area of this paper.
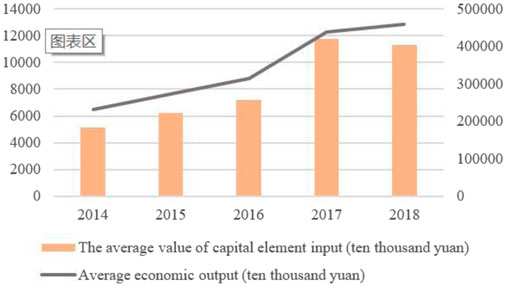
FIGURE 4. Average value of capital factor input and economic output of high-tech industrial enterprises in Zhejiang province in 2018.
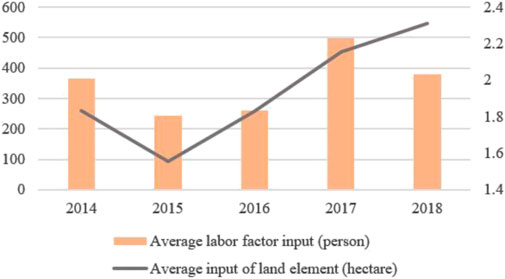
FIGURE 5. Average value of labor factor and land factor input of high-tech industrial enterprises in Zhejiang province in 2018.
The research subjects of this paper are listed high-tech enterprises in Zhejiang Province, with a total sample of 307. The detailed distribution is shown in Figure 7. High-tech industrial land-use data were taken from the Land China website and the Zhejiang Statistical Yearbook on Land Resources. Government behavior data were taken from the Zhejiang Statistical Yearbook and various local government websites. Given the availability and completeness of the data, the enterprise behavior data were compiled from the annual reports published by each listed high-tech enterprise from 2014 to 2018 and the science and technology statistical bulletins of each local government, and the data on control variables were obtained from the Zhejiang Statistical Yearbook (2014–2018). Based on the descriptive statistical results of the variables presented in Table 2, the most stable indicator is the number of college graduates, with a standard deviation of 0.0630. The indicator with the highest degree of dispersion is enterprise profitability, with a standard deviation of 2.5271. The average value of the SUB indicator is 22.4128, which is the highest variable, while the average value of the ASS indicator is −0.8826, the lowest variable. Indicators with the same observed values of the misallocation indicators included SUB, ASS, R&D, and PRO. In addition, the most recent year of the data is 2018; therefore, the timeliness of the data is relatively good, and all of the variables pass the logic test.
Results and discussion
Empirical results
The theoretical starting point for the land misallocation of high-tech industries is the difference in the marginal output of land. The greater the difference in the land factor’s marginal output, the greater the deviation of the factor allocation from the efficient subject or method (Baqaee and Farhi, 2020), which in turn indicates that the land misallocation in high-tech industries is more serious. As shown in Figure 8 Zhejiang Province shows an increase of 98% in the output level of high-tech industries. Ningbo has the largest absolute value of output, but its growth rate is only 33%, and Wenzhou has the smallest absolute value of output, but its growth rate is as high as 684%. Under the same input level, the output levels of different regions did not increase year-on-year, possibly due to the misallocation of factor resources.
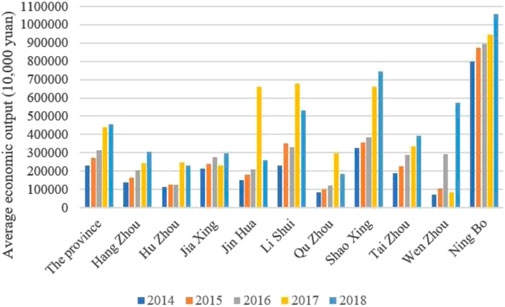
FIGURE 8. Variation of average economic output of high-tech industries in Zhejiang province from 2014 to 2018.
As shown in Table 3, the degree of correlation among government behavior variables, enterprise behavior variables, and mixed variables and the degree of fitting of the regression are high. The Durbin Watson (DW) value can be used to determine the autocorrelation status of the model. The DW test value is close to 2, indicating that no autocorrelation problem will occur. The significance is less than 0.01, indicating that the mechanisms of hypotheses 1, 2, and 3 can be well explained. The F-test value reached a significance level of 0.01, indicating that the explanatory power of the model was relatively good.
Under the government behavior model, the unstandardized coefficients of FIN and SUB are positive (Table 4), indicating that these two have a positive impact on the land misallocation in high-tech industry, and the unstandardized coefficient of POL is negative, indicating that there is a negative correlation between POL and land misallocation. The control variables were significant. The results in Table 4 show that land-use policy support can effectively alleviate the land misallocation in high-tech industry. The government’s financial subsidies for high-tech enterprises will attract more high-tech enterprises, but the higher the degree of fiscal decentralization, the greater the pressure on the local government to balance incomes and expenditures. Under conditions of fierce local competition, the government tends to invest resources in capital-intensive traditional industries. Thus inefficient, traditional industries continue to occupy a large amount of land resources, and high-tech enterprises have difficulty gaining entry, which will objectively increase the degree of land misallocation in high-tech industries. Hypothesis 1 was verified.
Under the enterprise behavioral model, the control variables were significant and land misallocation in high-tech industries is significantly inhibited by PRO and R&D (Table 5). The R&D investment of high-tech enterprises is directly related to their level of innovation and operating income. When R&D investment is insufficient, enterprises may use policy support to invest in land speculation and begin to hoard large amounts of land resources, resulting in land misallocation in high-tech industries. Profitability is the foundation of enterprise survival. In the case of excessive allocation of land factors, high land-holding costs will hinder the flow of capital elements to technology R&D, and enterprises will choose to concentrate productivity on low-end technology projects with easily produced outcomes, resulting in low efficiency. If land factors are not sufficiently allocated, enterprises that cannot obtain sufficient production space will be forced to reduce production efficiency, resulting in insufficient R&D revenue and profitability. The lack of internal revenue capacity among enterprises will in turn affect their investment in land factors, and this circular effect will deepen the land misallocation in high-tech industries. Hypothesis 2 was verified.
Under the hybrid model, the control variables were significant, and both government behavior and enterprise behavior will have an impact on the land misallocation in the high-tech industry. In the regression results, the larger the regression coefficient, the deeper the impact. Overall, the regression coefficient of the independent variable representing government behavior is greater than the regression coefficient of the independent variable representing enterprise behavior (Table 6), indicating that government behavior has the main impact. That is, hypothesis 3 is verified.
Discussion
First, land-use support policies for high-tech enterprises can effectively alleviate land misallocation, while excessive fiscal subsidies and tax incentives under fiscal decentralization will further increase land misallocation. Due to tax distribution reform and promotion incentives, the local government favors the development model of “operating the city with land” by virtue of an absolute monopoly over the land, which leads to the extensive use of industrial land by the local government and inhibits the transformation and upgrading of the industrial structure. Policies such as financial subsidies and tax incentives are the most important means by which local governments can attract investment, which can expand the economic benefits of the region in the short term and provide the government with fiscal revenue in the long term, thereby improving the performance of the government. High-tech enterprises are highly sensitive to production costs, and local governments usually choose to lower the price of industrial land and provide a large number of subsidies and preferential policies to attract high-tech enterprises. However, high-tech industries have high R&D costs, long payback periods, and high risks, and local governments tend to invest resources in capital-intensive traditional industries according to a performance evaluation system based on economic levels, making it difficult for traditional inefficient enterprises to vacate and for high-tech enterprises to enter. Therefore, the government should provide supportive policies for high-tech industries, such as land price concessions and land financial loans, and should rationally use tax incentives and financial subsidies. For advanced high-tech enterprises or projects with large output value per unit of land area that meets the requirements of government-supported key technologies, the reserve price for land transfer should be set at 70%–80% of the corresponding benchmark land price for the land class of the location. In addition, the transformation and upgrading of traditional industries should be accelerated to attract a new type of traditional industries and achieve the scaling of high-tech industries. Meanwhile, the proportion of economic output assessed in the performance evaluation should be reduced to establish a green performance evaluation system.
Second, the higher the R&D expenditure-to-income ratio and profitability, the lesser the land misallocation in high-tech industries. The ultimate goal of high-tech enterprises in carrying out scientific and technological R&D and technological innovation activities is to meet consumer demand by substantively improving old products and developing new products, thereby increasing the level of profitability and creating enterprise value. The higher the profitability of the enterprise, the better able it is to invest in scientific and technological R&D. Therefore, from the perspective of long-term development, high-tech enterprises should rationally use the government’s preferential support policies to intensively use the necessary land resources and invest more production factors in production R&D, thereby obtaining a higher level of profitability. Management should formulate a scientific and reasonable long-term development plan based on the enterprise’s actual situation to broaden the financing channels for enterprise technological innovation investment. The intellectual property protection system should be further improved to serve as the core competitive factor of high-tech enterprises. For traditional enterprises, land-use efficiency should be improved to curb the demand for new production land and alleviate the distorted allocation of high-tech industrial land. In addition, a perfect market operation mechanism is a key way to ensure the rational allocation of production factors, such as land resources, in the high-tech industry. The basic and decisive role of the market in the resource allocation should be effectively brought into action to establish an integrated regional market in Zhejiang Province and ensure the circulation of land factors.
Last, both government behavior and enterprise behavior have an impact on land misallocation in high-tech industry, but government behavior has a major impact. Therefore, the regulation of government behavior should be the focus. Excessive government intervention should be reduced to reverse the path of extensional expansion of land for development and achieve the transformation of economic development through the optimal allocation of land resources. On the other hand, competition among local governments in attracting investment should be constrained. However, it is unrealistic to rely solely on local governments to optimize the allocation of industrial land. Therefore, the constructive interaction between government subsidies and social capital should be explored, and government subsidies should be used to attract more social capital to alleviate the financial pressure on the government and increase government revenue. The government should guide high-tech enterprises by establishing an incentive mechanism for innovation investment and should stimulate enterprises’ independent R&D and innovation based on the development demands of Zhejiang Province. To correct the land misallocation of high-tech industries and improve the efficiency of industrial development, the most important step is to clarify the boundary between the government and the market. Based on the analysis of the theoretical framework of land misallocation in high-tech industries, the government should mainly play a role in institutional supply and governance structure, design a reasonable land supply system and a vacating mechanism for enterprises, provide space for more high-tech enterprises to enter, and avoid the accumulation of land that remains inefficient and idle for the long term. The market should give full play to its dominant position in resource allocation, and competitors should be encouraged to actively carry out scientific R&D and technological innovation.
Conclusion
This study used the research area of prefecture-level cities in Zhejiang Province and investigated 307 listed high-tech enterprises. The time span of the study was from 2014 to 2018. Based on the four-level institutional analysis method, a theoretical analysis framework for high-tech industry land misallocation was established to explore the mechanism underlying land misallocation in the high-tech industry. The hypothesis was proposed, and the regression analysis method was used for model calculation and hypothesis verification to provide a theoretical basis and practical guidance for the optimal allocation of land use. The main conclusions of this study are that, based on the government’s behavior, the land-use support policies for high-tech industries can effectively alleviate the misallocation of land factors, while the government’s improper financial support measures will increase the degree of land misallocation in high-tech industries. The greater the governmental intervention, the more serious the resulting misallocation problem. The government should formulate reasonable policies to support the land use of high-tech industries, increase efforts to promote the transformation and upgrading of traditional industries, and transform the government performance evaluation system that focuses on the economic level. Based on the level of enterprise behavior, when the R&D investment and profitability of high-tech enterprises are low, enterprises are more prone to low output efficiency and land misallocation. Enterprises should formulate long-term goals and plans, broaden the financing channels for scientific and technological R&D and technological innovation, improve their profitability, and form their core competitiveness. Based on multiple entities’ behavior, high-tech enterprises relied on a large number of government support measures to obtain a favorable development environment in the initial stage. Government behavior is the main cause of land misallocation in high-tech industries. Therefore, the government, enterprises, and the market should adopt an integrated strategy to reduce excessive government intervention in land resource allocation, allow the market to play a decisive role in resource allocation, and stimulate the vitality of enterprise R&D and innovation.
This study breaks from the traditional governance model, which uses only qualitative methods to explore the optimal land allocation for high-tech industries. Instead, this study uses the Williamson institutional analytical framework to construct a model and conducts empirical tests using regression analysis to reveal the internal mechanism of land misallocation of high-tech industries; additionally, it proposes corresponding governance strategies that account for the behavior of multiple entities, such as the government, the market, and high-tech enterprises. This study has high value as a reference for correcting the land misallocation of high-tech industries. However, due to data acquisition and field investigation limitations, the research area was Zhejiang Province, a developed eastern coastal province of China. The land misallocation of high-tech industries was mainly explored from the spatial perspective, ignoring the inherent differences within high-tech enterprises. Representative areas of the Central and Western regions can be included in later studies, and further research and validation can be conducted at the industry classification level to improve the applicability and rigor of the research conclusions.
Data availability statement
The original contributions presented in the study are included in the article/Supplementary Material, and further inquiries can be directed to the corresponding authors.
Author contributions
Conceptualization, XZ and LZ; methodology, LH and HB; validation, XZ, LZ, and FT-H; formal analysis, LZ; investigation, XZ; resources, LH and HB; data curation, XZ, LZ, and LH; writing—original draft preparation, LZ and XZ; writing—review and editing, LH and FT-H; supervision, HB; and funding acquisition, LH and HB. All authors have read and agreed to the published version of the manuscript.
Funding
This research was funded by the MOE (Ministry of Education in China) Project of Humanities and Social Sciences, grant number 21YJA630022, and Zhejiang Provincial Key Research Base of Philosophy and Social Science (Center for Economic Behavior Decision-making at Zhejiang University of Finance and Economics), Grant Number 20JDZD022; Grant-in-Aid for the Excellent Young Researcher of the Ministry of Education, Culture, Sports, Science and Technology of Japan (MEXT); and Grant-in-Aid for Young Scientists (No. 22K13432) of the Japan Society for the Promotion of Science (JSPS).
Conflict of interest
The authors declare that the research was conducted in the absence of any commercial or financial relationships that could be construed as a potential conflict of interest.
Publisher’s note
All claims expressed in this article are solely those of the authors and do not necessarily represent those of their affiliated organizations, or those of the publisher, the editors, and the reviewers. Any product that may be evaluated in this article, or claim that may be made by its manufacturer, is not guaranteed or endorsed by the publisher.
References
Adler, P., Florida, R., King, K., and Mellander, C. (2019). The city and high-tech startups: The spatial organization of Schumpeterian entrepreneurship. Cities 87, 121–130. doi:10.1016/j.cities.2018.12.013
Agheyisi, J. E. (2019). Inter-communal land conflicts in Benin city, Nigeria: Exploring the root causes in the context of customary land supply. Land Use Policy 83, 532–542. doi:10.1016/j.landusepol.2019.02.027
Banerjee, A. V., and Moll, B. (2010). Why does misallocation persist? Am. Econ. J. Macroecon. 2 (1), 189–206. doi:10.1257/mac.2.1.189
Baqaee, D., and Farhi, E. (2020). Productivity and misallocation in general equilibrium*. Q. J. Econ. 135 (1), 105–163. doi:10.1093/qje/qjz030
Bryan, B. A., Crossman, N. D., Nolan, M., Li, J., Navarro, J., and Connor, J. D. (2015). Land use efficiency: Anticipating future demand for land-sector greenhouse gas emissions abatement and managing trade-offs with agriculture, water, and biodiversity. Glob. Change Biol. 21 (11), 4098–4114. doi:10.1111/gcb.13020
Buhr, H., Funk, R. J., and Owen-Smith, J. (2021). The authenticity premium: Balancing conformity and innovation in high technology industries. Res. Policy 50 (1), 104085. doi:10.1016/j.respol.2020.104085
Chen, C., Restuccia, D., and Santaeulàlia-Llopis, R. (2022). The effects of land markets on resource allocation and agricultural productivity. Rev. Econ. Dyn. 45, 41–54. doi:10.1016/J.RED.2021.04.006
Chen, L., and Kangping, L. I. (2018). Administrative monopoly and resource misallocation from the perspective of fair competition review. Industrial Econ. Res (04), 113–123. doi:10.13269/j.cnki.ier.2018.04.010
Chen, W., Chen, W. J., Ning, S. Y., Liu, E. N., Zhou, X., Wang, Y. A., et al. (2019). Exploring the industrial land use efficiency of China's resource-based cities. Cities 93, 215–223. doi:10.1016/j.cities.2019.05.009
Chen, X. F., Liu, Z. Y., and Zhu, Q. Y. (2020). Reprint of "Performance evaluation of China's high-tech innovation process :Analysis based on the innovation value chain". Technovation 94-95, 102094–102153. doi:10.1016/j.technovation.2019.102094
Coman, A., and Ronen, B. (2007). Managing strategic and tactical constraints in the hi-tech industry. Int. J. Prod. Res. 45 (4), 779–788. doi:10.1080/00207540600597237
Ferrara, A., Salvati, L., Sabbi, A., and Colantoni, A. (2014). Soil resources, land cover changes and rural areas: Towards a spatial mismatch? Sci. Total Environ. 478, 116–122. doi:10.1016/j.scitotenv.2014.01.040
Guanzhong, W., and Yi, C. (2015). Government-led urban land use efficiency: Empirical results from China. Shanghai: Academic Monthly.
Guo, R. E. (2019). Effectuation, opportunity shaping and innovation strategy in high-tech new ventures. Md 57 (1), 115–130. doi:10.1108/MD-08-2017-0799
Han, H., and Zhang, X. (2020). Exploring environmental efficiency and total factor productivity of cultivated land use in China. Sci. Total Environ. 726, 138434. doi:10.1016/j.scitotenv.2020.138434
Haohan, W., Jianjun, X. U., Bo, L., Business, F. O., and University, N. (2019). Factor market distortion and growth of high-tech industry from the spatial perspective: An empirical test based on manufacturing of electronic and communication equipment. Econ. Geogr. 39 (09), 129–137. doi:10.15957/j.cnki.jjdl.2019.09.016
Huang, Z., and Du, X. (2017). Government intervention and land misallocation: Evidence from China. Cities 60, 323–332. doi:10.1016/j.cities.2016.10.006
JonesMisallocation, C. I. (2013). Misallocation, economic growth, and input-output economics. Adv. Econ. Econ. 10 (02), 419–456. doi:10.1017/CBO9781139060028.011
Kong, Q., Peng, D., Ruijia, Z., and Wong, Z. (2021). Resource misallocation, production efficiency and outward foreign direct investment decisions of Chinese enterprises. Res. Int. Bus. Finance 55, 101343. doi:10.1016/j.ribaf.2020.101343
Li, N. N., and Wang, Y. J. (2021). Estimating resource misallocation: Distinguishing factor market distortions from variable markups. Econ. Lett. 207, 110027. doi:10.1016/j.econlet.2021.110027
Li, Y., Luo, H., and Economics, S. O. (2017). Does land resource misallocation hinder the upgrading of industrial structure? Empirical evidence from Chinese 35 large and medium-sized cities. J. Finance Econ 43 (09), 110–121. doi:10.16538/J.CNKI.JFE.2017.09.009
Liang, L., and Fan, Z. Y. (2020). Resource misallocation threshold for high-tech industrial specialization on technological innovation performance for pharmaceutical manufacturing. Math. Problems Eng. 2020, 1–11. doi:10.1155/2020/6520240
Liang, L. W., Wang, Z. B., Luo, D., Wei, Y., and Sun, J. W. (2020). Synergy effects and it's influencing factors of China's high technological innovation and regional economy. PloS one 15 (5), e0231335. doi:10.1371/journal.pone.0231335
Liu, C. Y., Gao, X. Y., Ma, W. L., and Chen, X. T. (2020). Research on regional differences and influencing factors of green technology innovation efficiency of China's high-tech industry. J. Comput. Appl. Math. 369, 112597. doi:10.1016/j.cam.2019.112597
Liu, J., Jin, X., Xu, W., Gu, Z., Yang, Y., Ren, J., et al. (2020). A new framework of land use efficiency for the coordination among food, economy and ecology in regional development. Sci. Total Environ. 710 (2), 135670. doi:10.1016/j.scitotenv.2019.135670
Liu, Y., Geng, H., Sun, W., and Chuanwu, L. I. (2018). Which is more important between the price distortion and marginal production? Source decomposition of the resource misallocation in construction land. China Land Sci 32 (11), 44–49. doi:10.1080/09537325.2017.1337092
Liu, Z., Chen, X., Chu, J., and Zhu, Q. (2018). Industrial development environment and innovation efficiency of high-tech industry: Analysis based on the framework of innovation systems. Technol. Analysis Strategic Manag. 30 (4), 434–446. doi:10.1080/09537325.2017.1337092
Lu, S., Bai, B., Li, J. J., Lin, J. J., Li, W., and Lin, J. (2022). Impact of virtual water export on water resource security associated with the energy and food bases in Northeast China. Technol. Forecast. Soc. Change 180, 121635. doi:10.1016/j.techfore.2022.121635
Lu, S., Li, J., Xiao, B., and Guo, M. (2020). Analysis of standard accounting method of economic compensation for ecological pollution in watershed. Sci. Total Environ. 737, 138157. doi:10.1016/j.scitotenv.2020.138157
Lu, S., Zhang, X., and Tang, Y. (2020). Evolutionary analysis on structural characteristics of water resource system in basins of Northern China. Sustain. Dev. 1, 13. doi:10.1002/sd.2031
Lu, S., Lian, Z., Sun, H., Wu, X., Bai, X., and Wang, C. (2021). Simulating trans-boundary watershed water resources conflict. Resour. Policy 73 (73), 102139. doi:10.1016/j.resourpol.2021.102139
Ma, X. Y., and Zhao, X. (2015). Land use allocation based on a multi-objective artificial immune optimization model: An application in anlu county, China. Sustainability 7 (11), 15632–15651. doi:10.3390/su71115632
Novikov, S. V. (2019). State regulation and stimulation of high-tech industries. Amazon. Invest. 8 (19), 308–315. doi:10.17559/TV-20190215075504
Pruchnow, J., Schögel, M., Wentzel, D., and Weber, M. (2006). Success through differentiation in the high-tech industry. Thexis 23 (4), 2–5. doi:10.1007/BF03249124
Sick, N., Preschitschek, N., Leker, J., and Bröring, S. (2019). A new framework to assess industry convergence in high technology environments. Technovation 84-85, 48–58. doi:10.1016/j.technovation.2018.08.001
Sun, T. S., and Lv, Y. Q. (2020). Employment centers and polycentric spatial development in Chinese cities: A multi-scale analysis. Cities 99, 102617. doi:10.1016/j.cities.2020.102617
Sun, Y. F., Ma, A., Su, H. R., Su, S. L., Chen, F., Wang, W., et al. (2020). Does the establishment of development zones really improve industrial land use efficiency? Implications for China's high-quality development policy. Land Use Policy 90, 104265. doi:10.1016/j.landusepol.2019.104265
Tsvetkova, A., Conroy, T., and Thill, J. C. (2020). Surviving in a high-tech manufacturing industry: The role of innovative environment and proximity to metropolitan industrial portfolio. Int. Entrep. Manag. J. 16 (2), 501–527. doi:10.1007/s11365-019-00591-8
Tung, F. C., and Yu, T. W. (2016). Does innovation leadership enhance creativity in high-tech industries? Leadersh. Organ. Dev. J. 37 (5), 579–592. doi:10.1108/LODJ-09-2014-0170
Wang, K. J., Lestari, Y. D., and Tran, V. N. B. (2017). Location selection of high-tech manufacturing firms by a fuzzy analytic network process: A case study of taiwan high-tech industry. Int. J. Fuzzy Syst. 19 (5), 1560–1584. doi:10.1007/s40815-016-0264-z
Wang, L., Xue, Y. B., Chang, M., and Xie, C. (2020). Macroeconomic determinants of high-tech migration in China: The case of Yangtze River delta urban agglomeration. Cities 107, 102888. doi:10.1016/j.cities.2020.102888
Wang, L. Y., and Wang, B. H. (2020). Spatial statistical analysis and comprehensive evaluation of high-tech industry development. Ojs 10 (03), 431–452. doi:10.4236/ojs.2020.103028
Wang, R., and Tan, R. (2020). Efficiency and distribution of rural construction land marketization in contemporary China. China Econ. Rev. 60, 101223. doi:10.1016/j.chieco.2018.09.004
Wang, W., Wu, C., Yan, C., Guo, C., and Peng, D. (2020). China's local governments breaking the land use planning quota: A strategic interaction perspective. Land Use Policy 92, 104434. doi:10.1016/j.landusepol.2019.104434
Wei, Z. X., Han, B., Han, L., and Shi, Y. Y. (2019). Factor substitution, diversified sources on biased technological progress and decomposition of energy intensity in China's high-tech industry. J. Clean. Prod. 231, 87–97. doi:10.1016/j.jclepro.2019.05.223
Yan, J. (2018). Land supply regulation and residential land misallocation: An empirical study based on the city-level panel data. China Land Sci 32 (05), 15–22.
Yang, Y. L., and Shao, X. (2018). Understanding industrialization and employment quality changes in China: Development of a qualitative measurement. China Econ. Rev. 47, 274–281. doi:10.1016/j.chieco.2017.08.009
Yin, Z. H., and Zhu, S. J. (2020). Consistencies and inconsistencies in urban governance and development. Cities 106, 102930. doi:10.1016/j.cities.2020.102930
Yu, L., Liu, X. Q., Fung, H. G., and Leung, W. K. (2020). Size and value effects in high-tech industries: The role of R&D investment. North Am. J. Econ. Finance 51, 100853. doi:10.1016/j.najef.2018.10.001
Zeng, L. (2019). Analysing the high-tech industry with a multivariable grey forecasting model based on fractional order accumulation. K. 48 (6), 1158–1174. doi:10.1108/K-02-2018-0078
Zeng, L., Yan-Ling, L. I., and Liu, Y. F. (2019). The effect of land resource misallocation on urban agglomeration characteristics——From the perspective of industrial agglomeration and structure. Econ. Surv 36 (05), 104–111. doi:10.15931/j.cnki.1006-1096.20190624.013
Zhang, M. (2020). The analysis of the influencing factors of high-tech industry collaborative innovation efficiency in China based on two-stage DEA-Tobit model. Int. J. Front. Eng. Technol. 2 (1), 84–94. doi:10.25236/IJFET.2020.020108
Zhao, J. (2019). Chinese state-owned companies, misallocation and the reform policy. Chin. Polit. Sci. Rev. 4, 28–51. doi:10.1007/s41111-018-0112-4
Keywords: high-tech industry, land misallocation, government behavior, enterprise behavior, Williamson’s four-level institutional analysis framework
Citation: Han L, Zhou L, Bao H, Zeng X and Taghizadeh-Hesary F (2022) What affects China’s high-tech industry land misallocation: Government behavior or enterprise behavior?. Front. Environ. Sci. 10:959926. doi: 10.3389/fenvs.2022.959926
Received: 02 June 2022; Accepted: 21 July 2022;
Published: 23 August 2022.
Edited by:
Zhen Wang, Huazhong Agricultural University, ChinaReviewed by:
Yafeng Zou, Fuzhou University, ChinaAnlu Zhang, Huazhong Agricultural University, China
Copyright © 2022 Han, Zhou, Bao, Zeng and Taghizadeh-Hesary. This is an open-access article distributed under the terms of the Creative Commons Attribution License (CC BY). The use, distribution or reproduction in other forums is permitted, provided the original author(s) and the copyright owner(s) are credited and that the original publication in this journal is cited, in accordance with accepted academic practice. No use, distribution or reproduction is permitted which does not comply with these terms.
*Correspondence: Haijun Bao, YmFvaGFpanVuQHp1Y2MuZWR1LmNu; Farhad Taghizadeh-Hesary, ZmFyaGFkQHRzYy51LXRva2FpLmFjLmpw