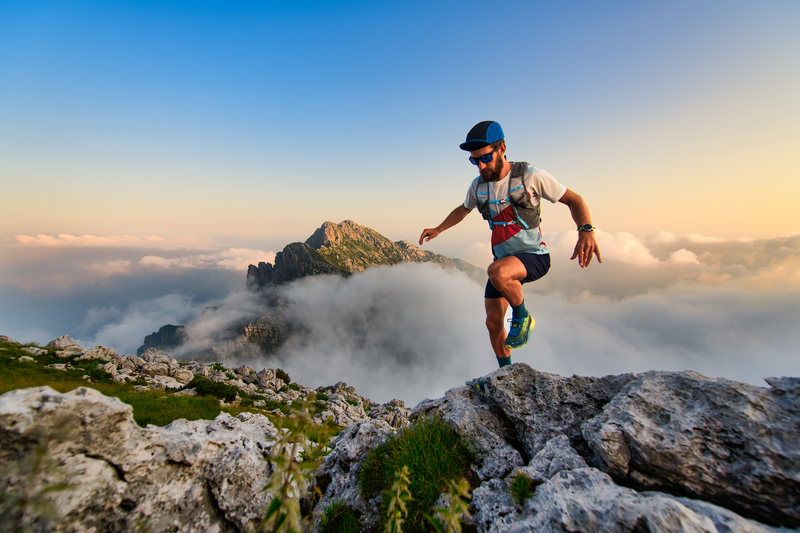
94% of researchers rate our articles as excellent or good
Learn more about the work of our research integrity team to safeguard the quality of each article we publish.
Find out more
ORIGINAL RESEARCH article
Front. Environ. Sci. , 13 July 2022
Sec. Environmental Economics and Management
Volume 10 - 2022 | https://doi.org/10.3389/fenvs.2022.953070
This article is part of the Research Topic Accentuating the Effects of Digital Circular Economy Transformations on Environmental Sustainability View all 25 articles
The digital economy has introduced far-reaching innovations in the fields of government governance, enterprise production, and social operation. How to motivate the economic development mode towards a low-carbon and greenway transformation through the digital economy is a major issue concerning the Chinese government. However, there is scarce evidence to interpret the role mechanism of the digital economy on carbon emission efficiency from the factor misallocation scenario. Taking a database from 30 provincial-level administrative regions for the period from 2011 to 2019 in China as an example, the paper examines the effect of the digital economy on carbon emission efficiency, as well as explores its role mechanism deeply in terms of factor misallocation (capital misallocation and labor misallocation). The results suggest that there is a significant potential for the digital economy to contribute to carbon emission efficiency, as well as this finding, is valid when considering both the endogeneity issue and a series of robustness checks. Also, the digital economy can significantly contribute to carbon efficiency in both southern and northern regions, but more strongly in the northern region. Besides, the digital economy can inhibit the factor misallocation (labor misallocation and capital misallocation) level which ultimately improves carbon emission efficiency. Finally, as a digital economy, it can positively impact carbon efficiency in the long run by mitigating factor misallocation (labor misallocation and capital misallocation).
Global warming has already emerged as an extremely serious impediment to low-carbon development, and how effectively controlling and reducing greenhouse gas emissions (GHG), mainly carbon dioxide, has proven to be a major issue in front of mankind. As China’s industrialization and urbanization continuously advance, the rapid economic growth and social productivity continue to rise, while energy consumption emits enormous amounts of carbon dioxide (Wang et al., 2019; Wang et al., 2020a). Excessive GHG emissions which result in increasingly frequent extreme and severe weather phenomena also have an adverse impact on production, economic development, and physical and mental health, and consequently, the resulting ecological concerns are already inflicting incalculable economic losses (Deng et al., 2019; Jia et al., 2022; Li Z. et al., 2021). As evidenced by data published by the International Energy Agency (IEA), China’s carbon emissions surged dramatically since the 21st century, with its total carbon emissions surging from 8.83 billion tons to 9.9 billion tons over the past decade, rendering it the world’s largest carbon emitter1. In response to the increasingly challenging climate environment, the Chinese government has successively enacted several policies to shoulder its share of responsibility in the developing process (Yang et al., 2022; Ren et al., 2021). China, for example, has made clear that it will contribute more autonomously by aiming for “peak carbon emissions by 2030 and carbon neutrality by 2060” (Hao et al., 2021; Fang et al., 2022; Xin et al., 2022). Nevertheless, as China strives to fulfill its carbon emission reduction goals, the most immediate dilemma is the need to optimize the ecosystem by boosting carbon emission efficiency while guaranteeing stable and healthy economic development (Meng et al., 2021). Carbon emission efficiency is considered as one of the parameters to evaluate low carbon economy level, which is essentially a production technology efficiency considering carbon emission that can reflect the resource utilization efficiency of production activities as well as the carbon utilization capacity (Shi et al., 2022). Therefore, an in-depth discussion on carbon emission efficiency not only helps analyze the scope for carbon emission reduction improvement in each area, but also contributes to the early achievement of the double carbon goal.
Since the double carbon goal is proposed, how to strengthen carbon emission efficiency has become a hot topic for scholars, and scholars have conducted investigations on carbon emission efficiency-related issues from various fields. As a newly emerging economic phenomenon, the digital economy has been continuously elevating the digitalization, networking, and intelligentization of the economy and society through digital industrialization and industrial digitization, effectively driving economic development (Li J. et al., 2021). Meanwhile, the digital economy reacts directly to the huge changes in the internal endowment and external environment of the economy under its high penetration, scale effect, and network effect. The White Paper on the Development of China’s Digital Economy covers the Chinese digital economy scale from RMB 9.5 trillion in 2011 to RMB 39.2 trillion in 2020, as well as the share of GDP accounted for by the digital economy at 38.6% in 2020, with a 9.7% growth rate, significantly faster than the nominal GDP growth rate in the same period2. Consequently, the digital economy has experienced rapid growth with continuous attention from academia (Wang et al., 2021a). Among them, the impact of the digital economy on carbon emission efficiency is one of the key points of academic interest. Some scholars consider that the digital economy can directly or indirectly influence the carbon emissions generated by energy activities and thus the carbon emission efficiency through its wide application in the chain of energy production, consumption, transmission, operation, management, measurement, and trading. Other scholars believe that the e-commerce industry, big data industry, communication and Internet industry in the digital economy, as environmentally-friendly industries, can crush highly energy-consuming and high-emission industries through the crowding-out effect and optimize the industrial structure, which in turn affects carbon emission efficiency.
Moreover, lagging factor market reform is a critical factor in China’s slow marketization process, which is largely reflected in the excessive intervention of local governments in factor trading activities (Yang et al., 2021a). Such excessive intervention can distort factor prices and consequently trigger factor misallocation (Wang et al., 2020c; Wang et al., 2021b; Wu et al., 2022). The Chinese government, suggests that the proliferation and transmission of resource allocation distortions have brought about economic and structural problems. However, market-based factor allocation is the fundamental way to drive high-quality economic development. Simultaneously, the spread of digital infrastructure and digital technology has a huge impact on economic development. Regarding the allocation of resources, the digital economy can alleviate information asymmetry, which is an essential factor affecting the flow and allocation of labor and capital. However, few existing studies seek to quantify the impact of the digital economy on carbon emission efficiency by considering factor misallocation. So, what is the impact of the evolving digital economy on carbon emission efficiency, and how does this impact differ from a regional heterogeneity perspective? What is the role of the impact of the digital economy on carbon efficiency in factor misallocation scenarios? Responses to the above questions are not only informative and instructive for realizing a green and low-carbon economic transition by raising carbon emission efficiency, but also serve as policy guidance for emerging economies with similar development to China. Therefore, this paper incorporates factor misallocation into the research framework of the impact of the digital economy on carbon emission efficiency, systematically discusses the role mechanism and heterogeneity of the digital economy on carbon emission efficiency under factor misallocation, and quantifies the dynamic effect of the digital economy and factor misallocation on carbon emission efficiency. This study aims to provide a scientific basis and theoretical reference for improving the construction of the digital economy, reducing factor misallocation, and facilitating the steady improvement of carbon emission efficiency through rigorous empirical analysis.
This paper primarily supplements the current research in the following dimensions. First, because of its high penetration, the digital economy is highly integrated with other industries, so the results of directly measuring the value-added to the digital economy or using the satellite account method may underestimate the scale of the digital economy. Comparatively, utilizing the input-output method of national accounting to evaluate the value-added to the digital economy may be nearer to the real value. Therefore, this paper adopts the input-output method to estimate the digital economy to develop the existing content of the research on measuring the digital economy. Second, this paper examines the role mechanism of the digital economy in influencing carbon emission efficiency according to the current literature, and then investigates the nexus that emerges between the digital economy and carbon emission efficiency with the help of an econometric model, as well as demonstrating the heterogeneity of the relationship between the two based on geographical location, which is conducive to the development of related theories. Thirdly, the intrinsic mechanism of interaction between the digital economy and carbon emission efficiency is further fully dissected from the factor misallocation perspective, which is empirically inspected by constructing a mediating effect on the basis of measuring the degree of resource misallocation. Finally, in conjunction with the findings of the study, this paper gives specific policy implications for the better role of the digital economy in factor allocation improvement and carbon emission efficiency enhancement, and policy rationale for digital economy development following specific local conditions.
Judging from the available studies, those highly linked to the digital economy, factor misallocation, and carbon emission efficiency can be largely categorized as three categories as follows. The first category is concerned with the discussion of the digital economy measurement and its economic and social effects (Li Y. et al., 2021). Digital technologies, such as cloud computing, the Internet of Things, as well as artificial intelligence, have been continuously integrated with public administration, logistics, transportation, and traditional manufacturing industries in recent years, empowering traditional industries with a digital economy to achieve high-quality economic development has become a topical issue (Corbett, 2018). Since its birth, the concept of the digital economy has gone through roughly the stages of an information economy, internet economy, and digital economy (Mesenbourg, 2001; Ma et al., 2022). As the digital economy develops and evolves, scholars have gradually widened the differences in their understanding of the digital economy (Bowman, 1996; Moulton, 2000; Turcan and Juho, 2014). As a result, there are also significant differences in the measurement methods of the digital economy. The current calculation of the digital economy can be categorized in four ways, which are national economic accounting methods, studying the value-added of the digital economy, measuring satellite account construction, and indexing (Acevedo Ruiz and Pena-Lopez, 2017; Jolliff and Nicholson, 2019; Ojanperä et al., 2019). Li J. et al. (2021), for example, measure the digital economy in terms of the Internet dimension using the entropy value method. Li Y. et al. (2021) take “Digital Inclusive Finance Index of China Peking University” as benchmark indicators to construct the digital economy index. Although scholars are yet to develop a unified concept of the digital economy, they have affirmed its positive effects on economic and social development. Labaye and Remes (2015) demonstrate that digital technologies have significantly contributed to China’s economic growth. Goldfarb and Tucker (2019) argue that the digital economy is a manifestation of the marketization of a new generation of digital technologies, which have natural advantages in reducing data-processing costs, transaction costs, and optimizing resource allocation. Ghasemaghaei and Calic (2019) describe the digital economy in terms of data that can improve the quality and efficiency of traditional factors such as labor and capital, which in turn can contribute to economic growth.
The second category is the research associated with carbon emissions. In line with the increasing prominence of climate issues, scholars have conducted increasingly profound investigations on carbon emissions, mainly concentrating on two dimensions of carbon emission level measurement and influence factor exploration (Xu et al., 2014). Ran et al. (2015) and Tang et al. (2020), for example, investigate carbon emissions in the Yellow River basin and the Yangtze River basin, respectively. Zheng et al. (2022) use multi-source data to investigate carbon emissions from energy consumption in Beijing as well as formulate a novel framework to calculate carbon emissions from houses and urban facilities. Cheng et al. (2018) account for the total factor carbon emission efficiency of the industrial sector separately by provincial administrative regions, and carbon emission efficiency has a significant growth trend. Shi et al. (2017) examine the factors influencing carbon emissions in the building industry, which shows that the energy intensity are the largest positive and negative roles on carbon emissions increase in the building industry, respectively. Zhou et al. (2020) analyze that regarding the association with industrial structure upgrading and carbon emission efficiency from a coupling perspective, discovering that a significant dynamic asymmetry is observed between carbon emission efficiency and industrial structure upgrading in most regions. Fang et al. (2022) utilizes the ICPP emission inventory method to estimate carbon emissions in eight industries in China and predicts that it is highly improbable that agriculture, building, manufacturing and transportation emissions will peak in 2030, while emissions from power and mining could peak in 2030.
The third category is the research associated with resource misallocation. It is an extremely crucial issue in economics research and one of the most critical issues via economic development in the world economy, thus, it has been a hot issue in the scientific community. A typical study on resource misallocation is Hsieh and Klenow’s (2009) computational index of resource misallocation and productivity gap estimation model, which measures such factors as labor and capital that are not optimally allocated and the resulting efficiency deficit. Additionally, Banerjee and Moll (2010) demonstrate that reallocating factors under conditions where the marginal output of all firms’ factors are all equivalent can still contribute to higher output. A study by Ljungwall and Tingvall (2015) highlights the insufficient R&D spending in China owing to the presence of more severe factor market distortions, causing a lag in innovation capacity. Examining 30 provincial administrative regions in China, Brandt et al. (2013) report that total factor productivity losses in China primarily originate from the misallocation of capital among provinces and the public and non-public sectors. Dollar and Wei (2007), using a study of more than 12,000 Chinese firms, suggest that improvement in capital distortion can increase GDP by 5% with constant inputs. Li and Du (2021) examine the micro-firm level to reveal that, in Chinese firms, internet development can contribute to energy efficiency by mitigating resource misallocation, resulting in energy savings. Yang et al. (2022) identify a significant spatial distribution heterogeneity of energy misallocation in China, which is strongly linked to the degree of energy internet development. Wu et al. (2022) assert that the distortions of capital distortions and output distortions to total factor productivity in the thermal power industry are more significant in hydropower plants in hydro-rich regions.
An overview of the existing studies suggests that the study on the role influence of the digital economy on carbon emission efficiency is just in its initial stage, especially few reports investigating the role mechanism of the digital economy on carbon emission efficiency from the consideration of factor misallocation. On the one hand, on the basis of a continuing study of the existing literature, this paper further theorizes how the digital economy can mitigate factor misallocation and thus contribute to carbon emission efficiency. On the other hand, utilizing a database from 30 provincial-level administrative regions for the period from 2011 to 2019 in China as an example, the capital misallocation index and labor misallocation index are introduced as mediating variables to deeply analyze the transmission mechanism and impact effect of factor misallocation between the digital economy and carbon emission efficiency.
The effect of the digital economy on carbon emission reduction efficiency is manifested in the three major aspects as follows. First, the digital industry represented by electronic equipment manufacturing and information technology services has all the attributes of an environmentally friendly industry. Digital industries are generally greener than traditional industries, whose development is less damaging to the environment (Ghobakhloo and Ching, 2019). Second, digital industrialization can help traditional industries improve their carbon emission efficiency. With the continuous promotion of digital industrialization, digital technologies represented by big data and artificial intelligence will continuously integrate with traditional industries. This will not only help their industries to gradually transform into digital, intelligent, and green, ones but also reduce energy consumption and carbon emissions while improving industrial added value (Qin and Cheng, 2017). Third, the establishment of a carbon emission market encourages enterprises to carry out environmental technology innovation. And the development of the digital economy helps to solve a series of key technical problems that plague the establishment of the carbon market, such as carbon emission monitoring, reporting, and verification (Weng and Xu, 2018). Based on this, this paper proposes hypothesis H1.
Hypothesis H1: The digital economy can significantly improve carbon emission efficiency.
Moreover, as the digital economy develops, the cost of information search decreases significantly, which reduces the information asymmetry between supply and demand, thus improving the price mechanism and reducing the degree of factor misallocation. Simultaneously, the digital economy has substantially strengthened market competition (Chen, 2020). On the one hand, because of the improved efficiency of information access, both the entry barrier of producers and the bargaining power of consumers have been lowered, thus significantly enhancing competition. On the other hand, the rise of the platform economy has blurred the concept of geography and intensified the cross-regional competition of enterprises. It gradually eliminates the less efficient enterprises in the continuous fierce competition, thus realizing the reallocation of factors. Third, the digital economy has obvious high permeability and high synergy. The combination of information, data, and other factors with traditional factors has substantially improved the productivity and allocation efficiency of factors (Zhai et al., 2022). The rise of the digital economy has also transformed traditional organizational structures and business processes towards networking, flattening, and flexibility, thereby improving the degree of factor misallocation (Kretschmer and Khashabi, 2020). Based on this, this paper proposes hypothesis H1.
Hypothesis H2: The digital economy can significantly inhibit factor misallocation.
The digital economy itself has such characteristics as scale effect, network effect, and platform effect, and thus it creates new channels and platforms for the orderly and reasonable flow of production factors associated with the rapid development of the digital economy, which in turn has an indirect role in promoting carbon emission efficiency. Digital economy development assists in lowering information and transaction costs as well as regional industrial upgrading (Lin and Chen, 2018, which in turn contributes to the carbon emission efficiency. On the one hand, the spatio-temporal compression characteristics of the digital economy effectively alleviate information asymmetry, and significantly reduce information costs and transaction costs, thus expanding the market scope while reducing industry and transaction barriers, prompting production factors and commodities to flow in a larger space in an orderly and reasonable manner, thus alleviating the problem of factor misallocation. On the other hand, the digital economy boosts the digital and intelligent transformation of industries, thus optimizing resource allocation. For example, the digital economy eliminates backward enterprises and industries such as low efficiency and high energy consumption through competitive effects and promotes new industries and new business models in the form of digital and intelligent transformation of traditional enterprises (Mattauch et al., 2015). This not only broadens the flow channels and platforms of production factors and improves factor allocation efficiency, but also promotes carbon emission efficiency.
Hypothesis H3: The digital economy improves carbon emission efficiency by ameliorating factor misallocations.
The theoretical mechanism of this paper is illustrated on the basis of the above theoretical analysis (Figure 1).
Referring to Wang et al. (2022), this paper develops the following model to examine the role of the digital economy on carbon efficiency. Specific model setting is given in Eq. 1:
where
Next, the following equation is developed to examine whether the digital economy can contribute to carbon emission efficiency by mitigating factors misallocation degree.
Step 1: The estimating equations of the digital economy and factor misallocation (including capital misallocation and labor misallocation) is constructed (See Eqs. 2 and 3).
where
Step 2: To inspect whether the digital economy can contribute to carbon emission efficiency by mitigating factor misallocation (capital factor misallocation and labor factor misallocation) (See Eqs. 4 and 5).
Referring to the test of Yang et al. (2021b), the significant
Following the research method of Wang et al. (2021b), this paper employs the scale of the digital economy to characterize the digital economy development degree. Under the definition of the connotation of the digital economy, the digital economy scale is estimated by using digital industrialization and industrial digitization as two parts of accounting for the scale of the digital economy (Li Y. et al., 2021; Wen et al., 2021). Among them, digital industrialization scale is mainly denoted by the added value of the information and communication technology industry
Step 1: Digital industrialization scale
where
Step 2: Industry digitalization scale, i.e., the value of
where the subscript
This paper employs the super-efficient Slack Based Measure (SBM) analysis to measure carbon emission efficiency. Tone (2002) first proposes the SBM model, which is a data envelopment analysis (DEA) model based on slack variables. It has two major advantages over the traditional DEA in that it treats the undesired output more appropriately and can solve the difficulty of comparing multiple efficient units with each other. The equations are set in the following form.
This paper considers capital stock, energy consumption, and labor as factor inputs, GDP as desired output, and total carbon emissions as non-desired output. Where each province’s capital stock was capitalized with 2010 as the base period. The number of urban year-end employment is labor input. Total energy consumption is energy input. Desired output is the real provincial equivalent of GDP transformed taking 2010 years into the base period. Undesired output is the carbon emissions of each province. Drawing on the research methodology of Zhao et al. (2022) and Su et al. (2021), the capital stock is estimated. The specific setting is shown in Eq. 11.
where
Referring to Li Y. et al. (2021) and Wang et al. (2020b), the IPCC approach is adopted for calculation. The specific calculation process is shown in Eq. 12.
Among them,
Referring to Hao et al. (2020), this paper introduces the capital misallocation index and labor misallocation index to estimate factor misallocation. The specific accounting techniques are shown in Eqs. 13–15:
Equation 13,
where
Referring to Yang et al. (2022), the Solow residual approach is employed to account for the factor distortion index. The production function in this paper is hypothesized to be a C-D production function that has fixed returns to scale. The specific form is shown in Eq. 15.
where
Since carbon emission efficiency is influenced by many factors, to be precise and reliable for the study, following Ren et al. (2021), and Hao et al. (2021), this paper identifies energy mix (
This paper selects balanced panel data of 30 Chinese provincial administrative region during the period from 2010 to 2019 as the study sample. The fundamental data are derived from the China EPS database, China Population, and Employment Statistical Yearbook, the Statistical Yearbooks of each province, and relevant data published by the National Bureau of Statistics in each years. Descriptive statistics are presented in Table 3.
Table 3 reports the statistical results of the digital economy on carbon emission efficiency. Columns (1) and (3) of Table 4 demonstrate the estimation results without the inclusion of control variables, columns (2) and (4) demonstrate the estimation results with the inclusion of control variables, columns (1) and (2) use OLS models to estimate the baseline regression model, and columns (3) and (4) use fixed effects models to estimate the baseline regression model. Table 5 reports that there is a significant positive regression coefficient for the digital economy. (p-value < 0.05), implying that the digital economy significantly drivers carbon emission efficiency. Our results have supported the view of Han et al. (2022), Zhang et al. (2022) and Ma et al. (2022) that digital economy will significantly inhibit carbon emissions and thus influence carbon emission efficiency. Hypothesis 1 is verified. It is easy to interpret that compared with traditional industries, digital industries are emphasized by high added value and environmental friendliness, thus the rise of digital industries has contributed to carbon emission efficiency (Han et al., 2022). Moreover, the development of the digital industry is the basis for the digitization of traditional industries. Along with the leapfrog growth of the digital industry, the digital industry has fuelled the transformation of traditional industry to digitalization, intelligence, and greening (Ma et al., 2022). Meanwhile, the digital economy has solved some technical problems faced by market-based environmental regulation, such as monitoring and verification, which has effectively facilitated the establishment of a carbon market and advanced carbon emission efficiency (Zhang et al., 2022).
The above results demonstrate that the digital economy significantly positive impact on carbon emissions efficiency. However, the above estimation methods may also have some endogeneity problems that cause the results to be biased. Also, the endogeneity problem arising from omitted variables is addressed by adding relevant variables to the control variables as far as possible in this paper. Moreover, considering that carbon emission efficiency represents the production and technology ability of the region while higher production and technology ability may contribute to the digital economy development in the region. Thus, there may be an inverse causality of carbon emission efficiency on digital economy. A properly selected instrumental variable is the most common strategy to tackle the endogeneity problem due to bi-directional causality. Referring to Maydeu-Olivares et al. (2019), this paper re-estimates the baseline regression results using two-stage least squares (TSLS) on the basis of internet penetration employed to generate the instrumental variable for the digital economy (see Table 5). Judging from the first-stage results, the coefficients of the key variables are significant (p-value < 0.01), so that the instrumental variable correlation requirement is achieved. Judging from the second-stage results, the coefficients of the digital economy are still significant (p-value < 0.01),l, and the F-statistics of the weak instrumental variable test are 192.54 and 89.03 (all of them are greater than 10), indicating that there is no weak instrumental variable issue. Consequently, Table 5 suggests that the digital economy can enhance carbon emissions efficiency when endogenous issues are addressed.
To investigate that the regression results are stable, this paper employs the robustness tests via replacing core explanatory variables, estimating sample subintervals, eliminating extreme values by shrinking tails, and removing municipalities (See Table 6). First, the explanatory variables are replaced. To avoid biased estimation results due to the selection of measurement methods, the carbon emissions efficiency of each province is re-measured by using the ratio of GDP to carbon emissions (see column (1) of Table 6). Second, municipalities directly under the central government are eliminated from the sample. Since municipalities directly under the central government and other provincial regions still have significant differences in various aspects, this paper excludes four samples of municipalities directly under the central government, namely Beijing, Shanghai, Tianjin, and Chongqing, to test again above effect (see column (2) of Table 6). Third, the data are subjected to a tailoring process. This paper recalculates above effect based on a 2% tailoring of each variable to remove extreme values (see column (3) of Table 6). Fourth, the sample subinterval is estimated. As China enters a new stage of digital economy development in 2015, this paper excludes the data from 2010 to 2014 to observe above effect in the sub-sample interval (see column (4) of Table 6). Table 6 demonstrates that the regression coefficients for the digital economy are not significantly different from the baseline regression results in the case of significance and direction, proving that the previous results are robust.
Given the enormous geographical boundaries in China and the significant diversity among regions in terms of natural endowments and industrial distribution. To elucidate the effects of the digital economy on the heterogeneity of carbon emission efficiency in different regions, referring to Liu et al. (2022), the results are re-estimated by dividing the study region into the south and the north following the Qinling-Huai River boundary. Table 7 reports that the coefficients of the digital economy are all significantly positive, but the digital economy has a higher effect on carbon emission efficiency in such a region in the north where energy-producing regions and heavy industries are relatively concentrated. The traditional manufacturing industry in the northern region is relatively concentrated, and its innovation and technology level is comparatively lagging in development. The major advantage of the digital economy is the compressibility of temporal and spatial, which greatly reduces the innovation barriers and R&D costs in the region. This not only provides an effective boost to the green upgrading in the case of the traditional manufacturing industry in the northern region, but also contributes to the economic development and ecological construction. In addition, the economic and social development level of the southern region is generally better than that of the northern region. The innovation-driven and ecological protection strategies are also implemented earlier, resulting in much higher carbon emission efficiency in the southern region than in the northern region.
In order to investigate the role mechanism of the digital economy on carbon emission efficiency, this paper examines them in the light of factor misallocations (labor factor misallocation and resource factor misallocation) (See Table 8). Column (1) of Table 8 reveals that the digital economy significantly enhances carbon emissions efficiency, ie., for every unit of growth in the digital economy, there will be a significant 8.5% increase in carbon emission efficiency. Columns (2) and (4) of Table 8 suggest that the digital economy significantly inhibits capital misallocation and labor misallocation, respectively. Columns (3) and (5) in Table 9 show that capital misallocation and labor misallocation significantly inhibit carbon emission efficiency. Therefore, the above results confirm that the digital economy can significantly contribute to carbon emissions efficiency via inhibiting factor misallocation (capital misallocation and labor misallocation). Hypotheses 2 and 3 are tested. We can explain the above results from the following aspects. Labor misallocation and capital misallocation reduce the production cost of backward enterprises, so that inefficient enterprises can continue to survive or even expand their scale, resulting in a decrease in carbon emission efficiency. Also, factor misallocation causes low labor and capital prices, which hinders enterprises’ technological innovation, and generates an inefficient and low-technology “lock-in effect” (Wang et., 2018). Moreover, the digital economy diminishes the cost of information search, eliminates information asymmetry in the market, and enhances the matching between the supply and demand of labor and capital. Meanwhile, the e-commerce model formed based on digital technology blurs the concept of geography, which strengthens the bargaining ability of consumers. This not only intensifies market competition and accelerates the elimination of less efficient enterprises, but also enables the reallocation of capital and labor, reducing the degree of the misallocation. Finally, the digital economy has significantly improved the productivity of labor and capital by empowering traditional factors with data, thereby improving their misallocation levels.
This paper further analyzes the dynamic effect of the digital economy on carbon emission efficiency in the light of factor misallocations (labor factor misallocation and resource factor misallocation) using impulse response functions based on a static study of the effects. The impulse function examines the impact of a random error term of an endogenous variable subjected to a shock of one standard deviation size on the current and future values of all endogenous variables. It describes the trajectory of mutual shocks and responses among the variables in the system, reflecting the dynamic influence relationship among the variables. Referring to Wu et al. (2021), unit root tests are conducted for the target variables using the LLC test, IPS test, and ADF-Fisher test (see Table 9). Table 9 indicates that the first-order difference terms of the digital economy, factor misallocation, and carbon emission efficiency pass the significance test, i.e., the digital economy, factor misallocation, and carbon emission efficiency are smooth. To assure the estimated parameters validity, the number of lags of the impulse response model needs to be determined. Further, three methods, AIC, BIC, and HQIC, are used for the lag order test (see Table 10). If the lag order is too high, it will diminish model degrees of freedom and cause unnecessary loss of model data. Conversely, if the lag order is too low, it will reduce the accuracy of the model test results. The final lag order is dominated by the Bayesian criterion, so the lag term orders are chosen as 4th order, 1st order, 1st order, 1st order, and 3rd order, respectively.
Figure 2 illustrates that the horizontal axis reveals the lag order, the vertical axis indicates the response degree, the red solid line in the middle is the impulse response function, and the width between the green solid line and the blue solid line indicates the positive and negative double standard deviation bands. Figure 2A shows that the impulse response function curve of the digital economy on carbon emission efficiency is a “U" shape. When the digital economy is subjected to an external positive shock, the change in carbon emission efficiency covers a trend of increasing and then decreasing, and the impact gradually converges to zero from the fourth period. It suggests that the digital economy has a longer-term influence on carbon emission efficiency after an external shock, with a relatively stable impact. Figures 2B,C illustrate that the digital economy produces a negative shock to capital (labor)misallocation, and such variation is only present in the first two periods, which exhibits a decreasing trend. A positive shock to the digital economy is transmitted to the capital (labor) misallocation and brings a negative shock to the capital (labor)misallocation, indicating that a positive shock to the digital economy can mitigate the degree of capital (labor) misallocation and that the impact is short term persistent. Figure 2D illustrates that after a positive shock to the capital misallocation, the carbon emission efficiency shows a negative change in the current period, but the change in carbon emission efficiency fluctuates up and down around the horizontal axis from the first lag and gradually converges to zero in the third period, indicating that a positive shock to the degree of capital misallocation in the short term may inhibit the improvement of carbon emission efficiency. Figure 2E illustrates that after a positive shock is given to labor misallocation, the amount of carbon emission efficiency change fluctuates up and down around the horizontal axis from the lagged period and gradually converges to zero from the eighth period, indicating that a positive shock to the degree of capital misallocation will influence carbon emission efficiency in the long run. In general, the digital economy can contribute to carbon emission efficiency in a certain period and inhibit factor misallocation. As capital misallocation can affect carbon emission efficiency in the short term, while labor misallocation can affect carbon emission efficiency in the longer term. The dynamic analysis results are consistent with the previous analysis, implying that the digital economy can contribute to carbon efficiency by inhibiting capital misallocation and labor misallocation.
The Chinese economy is in a critical transition period, facing economic pains caused by growth rate shift, structural adjustment, and the absorption of previous stimulus policies, in addition to coping with climate warming due to huge carbon emissions. Therefore, as a new economic form, how to promote economic growth and reduce carbon emissions through the digital economy has become an urgent issue to be investigated. Taking a database from 30 provincial-level administrative regions for the period 2011 to 2019 from 2011 to 2019 in China as an example, the paper examines the effect of the digital economy on carbon emission efficiency, as well as explores its role mechanism deeply in terms of factor misallocation. The results demonstrate that the digital economy can significantly contribute to the improvement of carbon emission efficiency, and this finding is valid when considering both the endogeneity issue and a series of robustness checks. The heterogeneous results show that the digital economy can significantly contribute to carbon efficiency in both southern and northern regions, but more strongly in the northern region. The role mechanism suggests that the digital economy can inhibit the factor misallocation (labor misallocation and capital misallocation) level by reducing the cost of information collection, strengthening enterprise competition, and promoting the integration of information, data, and other high-end data with traditional data, which ultimately enhances carbon emission efficiency. Finally, the dynamic effect results reveal that the digital economy can positively affect carbon efficiency in the long run by mitigating factor misallocation (labor misallocation and capital misallocation). As far as the above findings, this paper provides some policy implications for promoting carbon emission efficiency through the digital economy in the following three aspects.
First, policymakers should further enhance the digital economy scale. While continuously refining the fundamental digital technology, policymakers should strive to expand digital infrastructure construction, accelerate the expansion of the application scenarios of the new generation of digital technology, deepen the integration of the digital economy with the real economy, thereby providing an effective path to fulfil double carbon goal.
Secondly, policymakers should advance the coordinated development of the digital economy among regions by taking into account local conditions and zoning policies. Policymakers, for example, should not only fully balance the heterogeneity between different regions in terms of natural endowment and industrial distribution, but also promote digital industrialization or industrial digitization with a focus on different regions, so as to continuously improve carbon efficiency on the whole.
Finally, policymakers should maximize the factor allocation effect of the digital economy. While utilizing the spatial and temporal compression effect of the digital economy, policymakers should further accelerate the flow of information. By improving the price mechanism, the digital economy is adopted to promote the effective allocation of labor and capital among industries and regions, reduce the degree of factor mismatch, and improve allocation efficiency.
This paper confirms that the digital economy can positive impact on carbon emission efficiency by inhibiting factor misalignment. However, the role paths of the digital economy on carbon emission efficiency are complex and diverse, and some other factors may exist, such as technological innovation, industrial structure, and market segmentation. Scholars in the future can explore more potential paths for the digital economy to influence carbon emission efficiency from the above perspectives.
The original contributions presented in the study are included in the article/Supplementary Material, further inquiries can be directed to the corresponding authors.
WG: Conceptualization, Project administration, Writing - review andamp; editing, Writing - original draft. GL: Formal analysis, Data curation. LL: Software, Visualization. YX: Writing - original draft, Writing - review andamp; editing, Formal analysis. BS: Methodology, Data curation.: Writing - review andamp; editing, Validation. XS: Writing - review andamp; editing, Validation. QR: Writing - review andamp; editing, Writing - original draft, Conceptualization, Methodology, Funding acquisition, Supervision. XY:Writing - review andamp; editing.
The authors declare that the research was conducted in the absence of any commercial or financial relationships that could be construed as a potential conflict of interest.
All claims expressed in this article are solely those of the authors and do not necessarily represent those of their affiliated organizations, or those of the publisher, the editors and the reviewers. Any product that may be evaluated in this article, or claim that may be made by its manufacturer, is not guaranteed or endorsed by the publisher.
The authors acknowledge the financial support from the National Natural Science Foundation of China (71463057), and the Special Research Project on Science and Technology Innovation Strategy of Xinjiang Uygur Autonomous Region (2021B04001-4), and the graduate research and innovation project of Xinjiang University (XJ2021G014, XJ2021G013, XJ2020G020), and a special project of the School of Business and Economics, Shanghai Business School “Research on the Impact of Digital Economy on High-Quality Economic Development” (SWJJ-GYZX-2021-06). The usual disclaimer applies.
The Supplementary Material for this article can be found online at: https://www.frontiersin.org/articles/10.3389/fenvs.2022.953070/full#supplementary-material
1See more detail: https://www.bp.com/content/dam/bp/business-sites/en/global/corporate/pdfs/energy-economics/statistical-review/bp-stats-review-2021-full-report.pdf.
2See more detail: http://m.caict.ac.cn/yjcg/202007/P020200703318256637020.pdf.
Acevedo Ruiz, M., and Pena-Lopez, I. (2017). Evaluation of the Open Data for Development Program. Final report. Ottawa: IDRC. Available at: http://ictlogy.net/articles/20170815_acevedo_pena-lopez_-_evaluation_of_the_open_data_for_development_program_final_report.pdf .
Banerjee, A. V., and Moll, B. (2010). Why Does Misallocation Persist? Am. Econ. J. Macroecon. 2 (1), 189–206. doi:10.1257/mac.2.1.189
Bowman, J. P. (1996). The Digital Economy: Promise and Peril in the Age of Networked Intelligence. New York.
Brandt, L., Tombe, T., and Zhu, X. (2013). Factor Market Distortions across Time, Space and Sectors in China. Rev. Econ. Dyn. 16 (1), 39–58. doi:10.1016/j.red.2012.10.002
Cao, J., Law, S. H., Samad, A. R. B. A., Mohamad, W. N. B. W., Wang, J., and Yang, X. (2021). Impact of Financial Development and Technological Innovation on the Volatility of Green Growth-Evidence from China. Environ. Sci. Pollut. Res. 28 (35), 48053–48069. doi:10.1007/s11356-021-13828-3
Chen, Y. (2020). Improving Market Performance in the Digital Economy. China Econ. Rev. 62, 101482. doi:10.1016/j.chieco.2020.101482
Cheng, Z., Li, L., Liu, J., and Zhang, H. (2018). Total-factor Carbon Emission Efficiency of China's Provincial Industrial Sector and its Dynamic Evolution. Renew. Sustain. Energy Rev. 94, 330–339. doi:10.1016/j.rser.2018.06.015
Corbett, C. J. (2018). How Sustainable Is Big Data? Prod. Oper. Manag. 27 (9), 1685–1695. doi:10.1111/poms.12837
Deng, M., Zhong, S., and Xiang, G. (2019). Carbon Emission Reduction Effect of China's Final Demand Structure Change from 2013 to 2020: a Scenario-Based Analysis. Carbon Manag. 10 (4), 387–404. doi:10.1080/17583004.2019.1620036
Dollar, D., and Wei, S. J. (2007). Das (Wasted) Kapital: Firm Ownership and Investment Efficiency in China.Cambridge.
Fang, K., Li, C., Tang, Y., He, J., and Song, J. (2022). China's Pathways to Peak Carbon Emissions: New Insights from Various Industrial Sectors. Appl. Energy 306, 118039. doi:10.1016/j.apenergy.2021.118039
Ghasemaghaei, M., and Calic, G. (2019). Does Big Data Enhance Firm Innovation Competency? the Mediating Role of Data-Driven Insights. J. Bus. Res. 104, 69–84. doi:10.1016/j.jbusres.2019.07.006
Ghobakhloo, M., and Ching, N. T. (2019). Adoption of Digital Technologies of Smart Manufacturing in SMEs. J. Industrial Inf. Integration 16, 100107. doi:10.1016/j.jii.2019.100107
Goldfarb, A., and Tucker, C. (2019). Digital Economics. J. Econ. Literature 57 (1), 3–43. doi:10.1257/jel.20171452
Han, D., Ding, Y., Shi, Z., and He, Y. (2022). The Impact of Digital Economy on Total Factor Carbon Productivity: the Threshold Effect of Technology Accumulation. Environ. Sci. Pollut. Res., 1–16. doi:10.1007/s11356-022-19721-x
Hao, Y., Ba, N., Ren, S., and Wu, H. (2021). How Does International Technology Spillover Affect China's Carbon Emissions? A New Perspective through Intellectual Property Protection. Sustain. Prod. Consum. 25, 577–590. doi:10.1016/j.spc.2020.12.008
Hao, Y., Gai, Z., and Wu, H. (2020). How Do Resource Misallocation and Government Corruption Affect Green Total Factor Energy Efficiency? Evidence from China. Energy Policy 143, 111562. doi:10.1016/j.enpol.2020.111562
Hsieh, C.-T., and Klenow, P. J. (2009). Misallocation and Manufacturing TFP in China and India*. Q. J. Econ. 124 (4), 1403–1448. doi:10.1162/qjec.2009.124.4.1403
Jia, S., Yang, C., Wang, M., and Failler, P. (2022). Heterogeneous Impact of Land-Use on Climate Change: Study from a Spatial Perspective. Front. Environ. Sci. 510. doi:10.3389/fenvs.2022.840603
Jolliff, W., and Nicholson, J. R. (2019). Measuring the Digital Economy: An Update Incorporating Data from the 2018 Comprehensive Update of the Industry Economic Accounts. US Bureau of Economic Analysis. Washington, D.C., April, 2019-04.
Kretschmer, T., and Khashabi, P. (2020). Digital Transformation and Organization Design: An Integrated Approach. Calif. Manag. Rev. 62 (4), 86–104. doi:10.1177/0008125620940296
Labaye, E., and Remes, J. (2015). Digital Technologies and the Global Economy's Productivity Imperative. Digiworld Econ. J. 100, 47.
Li J, J., Chen, L., Chen, Y., and He, J. (2021). Digital Economy, Technological Innovation, and Green Economic Efficiency—Empirical Evidence from 277 Cities in China. Manag. Decis. Econ. 43(3):616–629. doi:10.1002/mde.3406
Li, M., and Du, W. (2021). Can Internet Development Improve the Energy Efficiency of Firms: Empirical Evidence from China. Energy 237, 121590. doi:10.1016/j.energy.2021.121590
Li Y, Y., Yang, X., Ran, Q., Wu, H., Irfan, M., and Ahmad, M. (2021). Energy Structure, Digital Economy, and Carbon Emissions: Evidence from China. Environ. Sci. Pollut. Res. 28 (45), 64606–64629. doi:10.1007/s11356-021-15304-4
Li Z, Z., Li, N., and Wen, H. (2021). Digital Economy and Environmental Quality: Evidence from 217 Cities in China. Sustainability 13 (14), 8058. doi:10.3390/su13148058
Lin, B., and Chen, Z. (2018). Does Factor Market Distortion Inhibit the Green Total Factor Productivity in China? J. Clean. Prod. 197, 25–33. doi:10.1016/j.jclepro.2018.06.094
Liu, J., Pan, X., Qu, Y., Wang, C., and Wu, W. (2022). Air Pollution, Human Capital and Corporate Social Responsibility Performance: Evidence from China. Appl. Econ. 54 (4), 467–483. doi:10.1080/00036846.2021.1963413
Ljungwall, C., and Tingvall, P. G. (2015). Is China Different? A Meta-Analysis of the Growth-Enhancing Effect from R&D Spending in China. China Econ. Rev. 36, 272–278. doi:10.1016/j.chieco.2015.10.001
Ma, Q., Tariq, M., Mahmood, H., and Khan, Z. (2022). The Nexus between Digital Economy and Carbon Dioxide Emissions in China: The Moderating Role of Investments in Research and Development. Technol. Soc. 68, 101910. doi:10.1016/j.techsoc.2022.101910
Mattauch, L., Creutzig, F., and Edenhofer, O. (2015). Avoiding Carbon Lock-In: Policy Options for Advancing Structural Change. Econ. Model. 50, 49–63. doi:10.1016/j.econmod.2015.06.002
Maydeu-Olivares, A., Shi, D., and Rosseel, Y. (2019). Instrumental Variables Two-Stage Least Squares (2SLS) vs. Maximum Likelihood Structural Equation Modeling of Causal Effects in Linear Regression Models. Struct. Equ. Model. A Multidiscip. J. 26 (6), 876–892. doi:10.1080/10705511.2019.1607740
Meng, Y., Liu, L., Wang, J., Ran, Q., Yang, X., and Shen, J. (2021). Assessing the Impact of the National Sustainable Development Planning of Resource-Based Cities Policy on Pollution Emission Intensity: Evidence from 270 Prefecture-Level Cities in China. Sustainability 13 (13), 7293. doi:10.3390/su13137293
Mesenbourg, T. L. (2001). Measuring the Digital Economy. Maryland: US Bureau of the Census, 1, 1–19.
Moulton, B. R. (2000). GDP and the Digital Economy: Keeping up with the Changes. MIT Press, Cambridge, Massachusetts, United States, 35–48.
Ojanperä, S., Graham, M., and Zook, M. (2019). The Digital Knowledge Economy Index: Mapping Content Production. J. Dev. Stud. 55 (12), 2626–2643. doi:10.1080/00220388.2018.1554208
Qin, S.-F., and Cheng, K. (2017). Future Digital Design and Manufacturing: Embracing Industry 4.0 and beyond. Chin. J. Mech. Eng. 30 (5), 1047–1049. doi:10.1007/s10033-017-0176-3
Ran, L., Lu, X. X., Yang, H., Li, L., Yu, R., Sun, H., et al. (2015). CO2outgassing from the Yellow River Network and its Implications for Riverine Carbon Cycle. J. Geophys. Res. Biogeosci. 120 (7), 1334–1347. doi:10.1002/2015jg002982
Ren, S., Hao, Y., Xu, L., Wu, H., and Ba, N. (2021). Digitalization and Energy: How Does Internet Development Affect China's Energy Consumption? Energy Econ. 98, 105220. doi:10.1016/j.eneco.2021.105220
Shi, Q., Chen, J., and Shen, L. (2017). Driving Factors of the Changes in the Carbon Emissions in the Chinese Construction Industry. J. Clean. Prod. 166, 615–627. doi:10.1016/j.jclepro.2017.08.056
Shi, X., and Xu, Y. (2022). Evaluation of China's Pilot Low-Carbon City Program: A Perspective of Industrial Carbon Emission Efficiency. Atmos. Pollut. Res. 13 (6), 101446. doi:10.1016/j.apr.2022.101446
Su, Y., Li, Z., and Yang, C. (2021). Spatial Interaction Spillover Effects between Digital Financial Technology and Urban Ecological Efficiency in China: an Empirical Study Based on Spatial Simultaneous Equations. Ijerph 18 (16), 8535. doi:10.3390/ijerph18168535
Tang, D., Zhang, Y., and Bethel, B. J. (2020). A Comprehensive Evaluation of Carbon Emission Reduction Capability in the Yangtze River Economic Belt. Ijerph 17 (2), 545. doi:10.3390/ijerph17020545
Tang, J., Zhong, S., and Xiang, G. (2019). Environmental Regulation, Directed Technical Change, and Economic Growth: Theoretic Model and Evidence from China. Int. Regional Sci. Rev. 42 (5-6), 519–549. doi:10.1177/0160017619835901
Tone, K. (2002). A Strange Case of the Cost and Allocative Efficiencies in DEA. J. Operational Res. Soc. 53 (11), 1225–1231. doi:10.1057/palgrave.jors.2601438
Turcan, R. V., and Juho, A. (2014). What Happens to International New Ventures beyond Start-Up: An Exploratory Study. J. Int. Entrep. 12 (2), 129–145. doi:10.1007/s10843-014-0124-6
Wang, G., Cui, L., Zheng, J., and Wang, C. (2021a). Digital Economy Empowers the Transformation and Upgrading of Manufacturing Industry: Heterogeneity Influence Mechanism and Effect. J. Statistics (05), 9–23. in Chinese. doi:10.19820/j.cnki.ISSN2096-7411.2021.05.002
Wang, J., Wang, W., Ran, Q., Irfan, M., Ren, S., Yang, X., Wu, H., and Ahmad, M. (2022). Analysis of the Mechanism of the Impact of Internet Development on Green Economic Growth: Evidence from 269 Prefecture Cities in China. Environ. Sci. Pollut. Res. 29 (7), 9990–10004. doi:10.1007/s11356-021-16381-1
Wang, S.-H., Song, M.-L., and Yu, T. (2019). Hidden Carbon Emissions, Industrial Clusters, and Structure Optimization in China. Comput. Econ. 54 (4), 1319–1342. doi:10.1007/s10614-016-9570-7
Wang, S., Chen, M., and Song, M. (2018). Energy Constraints, Green Technological Progress and Business Profit Ratios: Evidence from Big Data of Chinese Enterprises. Int. J. Prod. Res. 56 (8), 2963–2974. doi:10.1080/00207543.2018.1454613
Wang, S., Sun, X., and Song, M. (2021b). Environmental Regulation, Resource Misallocation, and Ecological Efficiency. Emerg. Mark. Finance Trade 57 (3), 410–429. doi:10.1080/1540496x.2018.1529560
Wang, S., Tang, Y., Du, Z., and Song, M. (2020a). Export Trade, Embodied Carbon Emissions, and Environmental Pollution: An Empirical Analysis of China's High- and New-Technology Industries. J. Environ. Manag. 276, 111371. doi:10.1016/j.jenvman.2020.111371
Wang, S., Wang, X., and Tang, Y. (2020b). Drivers of Carbon Emission Transfer in China-An Analysis of International Trade from 2004 to 2011. Sci. Total Environ. 709, 135924. doi:10.1016/j.scitotenv.2019.135924
Wang, S., Zhao, D., and Chen, H. (2020c). Government Corruption, Resource Misallocation, and Ecological Efficiency. Energy Econ. 85, 104573. doi:10.1016/j.eneco.2019.104573
Wen, H., Lee, C.-C., and Song, Z. (2021). Digitalization and Environment: How Does ICT Affect Enterprise Environmental Performance? Environ. Sci. Pollut. Res. 28 (39), 54826–54841. doi:10.1007/s11356-021-14474-5
Weng, Q., and Xu, H. (2018). A Review of China's Carbon Trading Market. Renew. Sustain. Energy Rev. 91, 613–619. doi:10.1016/j.rser.2018.04.026
Wu, H., Hao, Y., Ren, S., Yang, X., and Xie, G. (2021). Does Internet Development Improve Green Total Factor Energy Efficiency? Evidence from China. Energy Policy 153, 112247. doi:10.1016/j.enpol.2021.112247
Wu, H., Hao, Y., and Weng, J.-H. (2019). How Does Energy Consumption Affect China's Urbanization? New Evidence from Dynamic Threshold Panel Models. Energy policy 127, 24–38. doi:10.1016/j.enpol.2018.11.057
Wu, X., Zhao, J., Zhang, D., Lee, W.-C., and Yu, C.-H. (2022). Resource Misallocation and the Development of Hydropower Industry. Appl. Energy 306, 118024. doi:10.1016/j.apenergy.2021.118024
Xin, D., Zhi Sheng, Z., Jiahui, S., Wang, H., Xiao, H., and Yan, X. (2022). How Technological Innovation Influences Environmental Pollution: Evidence from China. Complexity 2022, 1–9. doi:10.1155/2022/5535310
Xu, S.-C., He, Z.-X., and Long, R.-Y. (2014). Factors that Influence Carbon Emissions Due to Energy Consumption in China: Decomposition Analysis Using LMDI. Appl. Energy 127, 182–193. doi:10.1016/j.apenergy.2014.03.093
Yang, X., Wang, J., Cao, J., Ren, S., Ran, Q., and Wu, H. (2021a). The Spatial Spillover Effect of Urban Sprawl and Fiscal Decentralization on Air Pollution: Evidence from 269 Cities in China. Empir. Econ., 1–29. doi:10.1007/s00181-021-02151-y
Yang, X., Wang, W., Wu, H., Wang, J., Ran, Q., and Ren, S. (2021b). The Impact of the New Energy Demonstration City Policy on the Green Total Factor Productivity of Resource-Based Cities: Empirical Evidence from a Quasi-Natural Experiment in China. J. Environ. Plan. Manag., 1–34. doi:10.1080/09640568.2021.1988529
Yang, X., Su, X., Ran, Q., Ren, S., Chen, B., Wang, W., et al. (2022). Assessing the Impact of Energy Internet and Energy Misallocation on Carbon Emissions: New Insights From China. Environ. Sci. Pollut. Res. 29 (16), 23436–23460. doi:10.1007/s11356-021-17217-8
Zhai, H., Yang, M., and Chan, K. C. (2022). Does Digital Transformation Enhance a Firm's Performance? Evidence from China. Technol. Soc. 68, 101841. doi:10.1016/j.techsoc.2021.101841
Zhang, W., Liu, X., Wang, D., and Zhou, J. (2022). Digital Economy and Carbon Emission Performance: Evidence at China's City Level. Energy Policy 165, 112927. doi:10.1016/j.enpol.2022.112927
Zhao, S., Cao, Y., Feng, C., Guo, K., and Zhang, J. (2022). How Do Heterogeneous R&D Investments Affect China's Green Productivity: Revisiting the Porter Hypothesis. Sci. Total Environ. 825, 154090. doi:10.1016/j.scitotenv.2022.154090
Zheng, Y., Du, S., Zhang, X., Bai, L., and Wang, H. (2022). Estimating Carbon Emissions in Urban Functional Zones Using Multi-Source Data: A Case Study in Beijing. Build. Environ. 212, 108804. doi:10.1016/j.buildenv.2022.108804
Zhong, S., Xiong, Y., and Xiang, G. (2021). Environmental Regulation Benefits for Whom? Heterogeneous Effects of the Intensity of the Environmental Regulation on Employment in China. J. Environ. Manag. 281, 111877. doi:10.1016/j.jenvman.2020.111877
Keywords: digital economy, factor misallocation, carbon emission efficiency, regional heterogeneity, economic development
Citation: Ge W, Xu Y, Liu G, Shen B, Su X, Liu L, Yang X and Ran Q (2022) Exploring the Impact of the Digital Economy on Carbon Emission Efficiency Under Factor Misallocation Constraints: New Insights From China. Front. Environ. Sci. 10:953070. doi: 10.3389/fenvs.2022.953070
Received: 25 May 2022; Accepted: 24 June 2022;
Published: 13 July 2022.
Edited by:
Muhammad Mohsin, Hunan University of Humanities, Science and Technology, ChinaReviewed by:
Ying Zhu, Xi’an University of Architecture and Technology, ChinaCopyright © 2022 Ge, Xu, Liu, Shen, Su, Liu, Yang and Ran. This is an open-access article distributed under the terms of the Creative Commons Attribution License (CC BY). The use, distribution or reproduction in other forums is permitted, provided the original author(s) and the copyright owner(s) are credited and that the original publication in this journal is cited, in accordance with accepted academic practice. No use, distribution or reproduction is permitted which does not comply with these terms.
*Correspondence: Yang Xu, eWFuZ3h1eGplZHVAMTI2LmNvbQ==; Qiying Ran, cmFucXl4amVkdUAxMjYuY29t
Disclaimer: All claims expressed in this article are solely those of the authors and do not necessarily represent those of their affiliated organizations, or those of the publisher, the editors and the reviewers. Any product that may be evaluated in this article or claim that may be made by its manufacturer is not guaranteed or endorsed by the publisher.
Research integrity at Frontiers
Learn more about the work of our research integrity team to safeguard the quality of each article we publish.