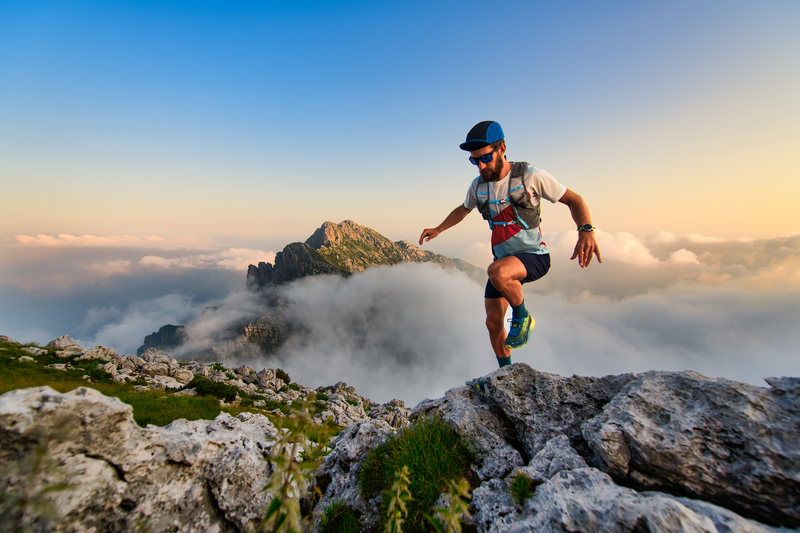
95% of researchers rate our articles as excellent or good
Learn more about the work of our research integrity team to safeguard the quality of each article we publish.
Find out more
OPINION article
Front. Environ. Sci. , 06 September 2022
Sec. Environmental Economics and Management
Volume 10 - 2022 | https://doi.org/10.3389/fenvs.2022.951306
This article is part of the Research Topic Evolution of Environmental Economics & Management in the Age of Artificial Intelligence for Sustainable Development View all 18 articles
Environmental business management is a special direction in the management of entrepreneurship within the framework of environmental legislation and corporate environmental responsibility. Despite the high importance for society (determines the contribution of business to the implementation of the SDGs) and business (forms loyalty to it), there are some difficulties in the area of environmental business management for two reasons, both of which (according to the hypothesis put forward in this article) can be overcome through the use of Big Data and AI (Lubis et al., 2019; Colvin et al., 2020).
The first reason is due to the fact that enterprises face a shortage of information about current ecological problems, ongoing initiatives to protect the environment and available opportunities to join them. Big Data make it possible to automatically collect, systematize and provide enterprises with all the necessary information (Popkova et al., 2020; Lamolinara et al., 2022; Mikac et al., 2022).
The second reason is that information about the state and protection of the environment has a large volume and is subject to constant changes. Processing and analysis of this information is not available for most enterprises, especially small and medium-sized ones. Artificial intelligence allows to quickly and accurately process a large amount of information and provide intelligent support for environmental decision-making in environmental business management (Parvathi Sangeetha et al., 2022; Popkova et al., 2022; Tian, 2022).
The purpose of the article is to substantiate the prospects and develop recommendations for improving environmental decision-making in environmental business-management using Big Data and AI. The originality of the article lies in providing clear justifications for the key role of technologies in the optimization of environmental decision-making in environmental business management in the AI era.
In their publications, Oteng et al. (2022), Pullin et al. (2022), and Tang et al. (2022) cite numerous advantages of using high technologies, in particular, AI and Big Data when making environmental decisions in environmental business management in industry. Among these advantages, it is necessary to indicate the possibility to fully take into account the totality of environmental parameters when making management decisions, as well as to search for the most optimal solutions from both an economic and environmental point of view.
The existing literature also reveals in sufficient detail the essence and features of agriculture in the era of artificial intelligence, embodied in the practice of AgroTech. In the works of Dai et al. (2022), Deng et al. (2022), Dupla et al. (2022), and Parmar et al. (2022), it is noted that as technological modernization strengthens, the gap between the economy and ecology increases. If this gap is quite large and obvious in industry, then in agriculture it has been most clearly manifested only in the process of the Fourth Industrial Revolution. Although high technologies make it possible to increase the volume of food production, achieve its climatic stability and other advantages, which is realized through the transition of the agro-industrial complex from an agrarian economy (based on agriculture producing eco-products) to an industrial economy (producing synthetic food with GMOs, food additives, etc.).
The works of Li et al. (2022), Ren et al. (2022), and Yew et al. (2022) indicate that high technologies require serious financial costs. Therefore, even with the support of the state, these technologies are either inaccessible to small and medium-sized businesses, or dictate the need to optimize costs. This hinders environmental decision-making in environmental business-management.
In this regard, a research question (RQ) arises about whether high technologies (Big data and AI) contribute to the improvement of processes of developing and making environmental decisions, and how this contribution can be maximized for the optimization of environmental business-management. Based on works by [FULL NAME], which point out the advantages of high technologies for environmental business-management, this article makes a hypothesis that high technologies (Big Data and AI) are instrumental in the optimality of environmental decision-making.
In industry, environmental decision-making is associated only with reducing the environmental costs of economic growth, but in agriculture, the prospects for environmental business-management are broader and also include the production of organic (natural) products and positive impact on the environment (for example, from planting plants and forests). Therefore, in order to find an answer to the RQ, this article pays special attention to the experience of AgroTech.
To determine the role of technologies in the optimization of environmental decision-making in environmental business-management in the AI era, the dynamics of startup financing in AgroTech in 2012–2020 and its contribution to the development of food production are analyzed using the example of AgroTech (based on Biotech, Robotics, AI and Big Data technologies) (Figure 1).
FIGURE 1. Financing of startups in AgroTech and its contribution to the development of food production in 2012–2020. Source: calculated and constructed by the authors based on the materials of the Queen (2022), Intellias (2022), and World Bank (2022).
As shown in Figure 1, AgroTech is actively financing startups, which increased more than 11 times in 2020 ($30.50 billion) compared to 2012 ($2.68 billion). The presented regression curves showed that as the amount of funding for startups in AgroTech increases, for every $1 billion, agriculture, forestry, and fishing value added increases by 0.0032% of GDP (correlation of 6.19%), and the food production index (2014–2016 = 100) increases by 0.0053 (correlation 86.60%).
According to the forecasts of Intellias (2022), by 2023, the amount of funding for startups in AgroTech will double by 2023, that is, it will amount to about $ 60 billion. Taking into account the established dependencies, it can be expected that this will ensure an increase in agriculture, forestry, and fishing value added by 2.26 of GDP and an increase in the food production index (2014–2016 = 100) by 14.02%. The obtained results indicate that high technologies (Big Data and AI) already play an important role in the optimization of environmental decision-making in environmental business-management in AgroTech.
Significant results of the use of Big Data and the use of AI in environmental decision-making were demonstrated in 2021 in Russia within the Year of Science and Technology. Application solutions that have been successfully implemented in the practice of Russian companies include smart forecasting of adverse climate change (forecasting weather and natural disasters)—in environmental science; automated search for new oil and gas fields, Smart Grid development—in the energy sector; creation of new materials and technologies, quality control—in materials science; optimization of urban space, control and intelligent support of sustainable and environmentally friendly management of natural resources—in the regional management (Ministry of Science and Higher Education of the Russian Federation, 2022).
The successfully implemented projects of using AI and Big Data for the improvement of environmental decision-making (Ministry of Science and Higher Education of the Russian Federation, 2022) in environmental science include: 1) Whirlwind forecasting based on Big Data and AI by the researchers of the laboratory of climate theory of the Obukhov Institute of Atmospheric Physics, RAS; 2) Fire forecasting by the research and information center of the Ministry of Emergency Situations of the Russian Federation based on the Thermal Points mobile application; 3) Flood forecasting by Sber in cooperation with the Moscow Aviation Institute and Ministry of Emergency Situations using online satellite images; 4) Reduction of the carbon footprint by the Research Center for Artificial Intelligence based on the Skolkovo Institute of Science and Technology, and carbon landfills.
In addition, many smart projects in the energy sector are being implemented in Russia (Ministry of Science and Higher Education of the Russian Federation, 2022): 1) Reducing energy consumption using the application developed by the PREDICT team from Mail. Ru Group; 2) Accounting for seismic activity in the development of Gazprom Neft fields; 3) Improving the safety of Rosatom’s alternative energy through the introduction of a system for monitoring the provision of employees with personal protective equipment at nuclear power plants based on computer vision algorithms.
Moreover, AI and Big Data are abundantly used in retail sales, ensuring the reduction of the environmental footprint of the economy. Thus, the Russian retail store network Lenta creates an environmentally-friendly (biodegradable) package, while the retail store network Rossmann in the Czech Republic and Germany has introduced the “filling stations” with detergents under the trademark Love Nature, an affiliated company of Henkel (Corporate accelerator GenerationS, 2022).
AI and Big Data help to combat climate change: 1) PJSC FGC UES (Russia) launched the process of development of main power transmission lines based on Artificial Intelligence technologies; 2) Conservation of land and marine biodiversity based on monitoring and control of ecosystems, e.g. using NASA Blue River Technology in the World ocean; 3) Water saving: Valor Water Analytics allows detecting leakages, analyzing water flows in real-time mode, and tracking utility meter malfunctions; 4) Improvement of the air quality: The IBM Green Horizons project brings together machine learning and the Internet of Things for the air quality forecasting. IBM and Microsoft tools combine conventional physical models of atmospheric and weather chemistry with machine learning models. Atmospheric air pollution monitoring stations are already operating in Moscow, Moscow Oblast, and several other regions. 5) Recycling development: Nevlabs waste sorting equipment based on Artificial Intelligence (recycling kiosks and smart waste bins), produced by WinBin and TrashBack (Softline, 2022).
To improve environmental decision-making in environmental business-management using Big Data and AI, the following recommendations are proposed. Firstly, consideration of sectoral characteristics of the use of high technologies in environmental decision-making to fully realize the development potential of environmental business management in each industry. So, in industry, the options of using Big Data and AI are being worked out in different aspects (both in theory and in practice) to improve economic production efficiency (for example, automation to increase production capacity and to benefit from the economies of scale), as well as environmental production efficiency (for example, “green” AI innovations).
In other industries, in particular, in agriculture, the focus is on the economic efficiency (for example, HRM or sales optimization) of the use of high technologies in entrepreneurship. The issues of environmental decision-making need independent and in-depth study in each industry, taking into account its characteristics. For example, in AgroTech, it is recommended to consider sustainable (responsible) environmental management as a separate economic practice and a separate area of environmental business management. In this area, independent automation of environmental decision-making can be carried out, while other areas (production and sales) may be less automated. This will reduce the costs of technological modernization of the business.
Secondly, the transition from individual to collective use of Big Data and AI in the practice of environmental decision-making in environmental business-management. Agriculture is strongly tied to the territory in which it is conducted. The planting of forests by one business structure will not noticeably improve the air quality in the territory, but if all enterprises join efforts and plant a large number of trees together, a serious contribution to the fight against climate change will be achieved.
In this regard, it is proposed to separate environmental business-management from other areas of corporate governance and separately automate environmental decision-making processes. This will help enterprises maintain economic independence and at the same time gain access to advanced technologies (Big Data and AI) for environmental business management, both acquired collectively and provided by local public authorities as a measure for the development of the ecological economy.
The contribution of the article to the literature consists in overcoming sectoral restrictions on the use of high technologies (Big Data and AI) when making environmental decisions in environmental business-management. Unlike Oteng et al. (2022), Pullin et al. (2022), and Tang et al. (2022), it has been proved that high technologies can be used in environmental business-management not only in industry, but also in agriculture (AgroTech). Thanks to this, a much greater potential for using Big Data and AI in environmental decision-making has been revealed, which is not limited to reducing the environmental costs of economic growth, but also includes improving the ecological balance.
In contrast to Dai et al. (2022), Deng et al. (2022), Dupla et al. (2022), and Parmar et al. (2022), it has been proved that technological modernization does not necessarily lead to a gap between the economy and ecology, but on the contrary, can help to bridge this gap. So, for example, the development of AgroTech simultaneously increases the added value created in the agro-industrial complex (economic advantage) and increases the productivity of natural (organic) agriculture (environmental advantage).
Unlike Li et al. (2022), Ren et al. (2022), and Yew et al. (2022), it has been demonstrated that high technologies (Big Data and AI) can (proved by the example of startups in AgroTech) and should be available to small and medium-sized businesses in various sectors of the AI economy. Authors’ framework practice-oriented recommendations have been proposed, which are universal and applicable in various sectors of the AI economy, aimed at diffusing high technologies (increasing accessibility) and increasing the efficiency of their use in environmental decision-making in environmental business management.
The result of the study was the confirmation of the correctness of the hypothesis put forward in the article, the justification of prospects, as well as the development of recommendations for improving environmental decision-making in environmental business-management using Big Data and AI. The key role of technologies in the optimization of environmental decision-making in environmental business-management in the AI era is revealed, associated with the harmonization and balancing of economic and environmental interests of business in decision-making, which has been proved in the example of AgroTech.
The uniqueness of the article and its contribution to the literature consist in the fact that it has bridged the gap between theory and practice, having demonstrated that high technologies (AI and Big Data) can be and are successfully used for the optimization of environmental decision-making in various sectors of the economy. For the first time, the article has proved through numerous empirical examples and the case experience of Russian companies, that high technologies have significant potential to improve the decision-making efficiency not only in the production sector but also in AgroTech and other sectors. To fulfill the identified potential to the fullest extent possible, it is recommended to take into account the industry specificity and make a transition from individual to shared use of Big Data and AI in the practice of environmental decision-making in environmental business-management.
The theoretical significance of the results obtained in the study is that they clarified organizational (in comparison with other areas of management) and industry (using the example of comparing industry and agriculture) features of technological modernization (automation based on Big data and AI) environmental business-management. The practical contribution of the conclusions and recommendations is due to the fact that the authors’ proposals for improving environmental decision-making using Big Data and AI can significantly expand the range of practical applications of environmental business-management from reducing environmental damage to maximizing the contribution of business to environmental protection and improving the environment by involving all sectors of the economy in environmental business-management.
SV, VK, and ZT contributed to conception and design of the study. ZT and TA performed the statistical analysis. SV wrote the first draft of the manuscript. SV, VK, ZT, and TA wrote sections of the manuscript. All authors contributed to manuscript revision, read, and approved the submitted version.
The authors declare that the research was conducted in the absence of any commercial or financial relationships that could be construed as a potential conflict of interest.
All claims expressed in this article are solely those of the authors and do not necessarily represent those of their affiliated organizations, or those of the publisher, the editors and the reviewers. Any product that may be evaluated in this article, or claim that may be made by its manufacturer, is not guaranteed or endorsed by the publisher.
Colvin, R. M., Witt, G. B., and Lacey, J. (2020). Power, perspective, and privilege: The challenge of translating stakeholder theory from business management to environmental and natural resource management. J. Environ. Manag. 271, 110974. doi:10.1016/j.jenvman.2020.110974
Corporate accelerator GenerationS, (2022). How Artificial Intelligence makes life more natural. Available at: https://new-retail.ru/tehnologii/kak_iskusstvennyy_intellekt_delaet_zhizn_estestvenney6681/(Accessed date: 09.08.2022).
Dai, X., Chen, Y., Wu, X., He, Y., and Wang, F. (2022). Are the agro-ecosystems sustainable? Measurement and evaluation: A case study of sichuan province, China. Front. Environ. Sci. 25. doi:10.3389/fenvs.2022.862740
Deng, X., Wang, G., Yan, H., Zheng, J., and Li, X. (2022). Spatial–temporal pattern and influencing factors of drought impacts on agriculture in China. Front. Environ. Sci. 31. doi:10.3389/fenvs.2022.820615
Dupla, X., Lemaître, T., Grand, S., Gondret, K., Charles, R., Verrecchia, E., et al. (2022). On-farm relationships between agricultural practices and annual changes in organic carbon content at a regional scale. Front. Environ. Sci. 24. doi:10.3389/fenvs.2022.834055
Intellias (2022). How to implement Big data Analytics in agriculture: 5 business use cases. Available at: https://intellias.com/how-to-encourage-farmers-to-use-big-data-analytics-in-agriculture/ (Accessed date: 17.05.2022).
Lamolinara, B., Pérez-Martínez, A., Guardado-Yordi, E., Dieguez-Santana, K., Diéguez-Santana, K., and Ruiz-Mercado, G. J. (2022). Anaerobic digestate management, environmental impacts, and techno-economic challenges. Waste Manag. 140, 14–30. doi:10.1016/j.wasman.2021.12.035
Li, B., Huang, Y., Guo, D., Liu, Z., Han, J. C., Wang, Z., et al. (2022). Environmental risks of disposable face masks during the pandemic of COVID-19: Challenges and management. Sci. Total Environ. 825, 153880. doi:10.1016/j.scitotenv.2022.153880
Lubis, Y., Nguyen, P. T., ThuyTo Nguyen, Q. L. H., Bich Huynh, V. D., and Fitrio, T. (2019). Study of green marketing: An environmentally friendly approach of business management. Test Eng. Manag. 81, 1080–1085.
Mikac, B., Abbiati, M., Adda, M., Desiderato, A., Pellegrini, M., Turicchia, E., et al. (2022). The environmental effects of the innovative ejectors plant technology for the eco-friendly sediment management in harbors. J. Mar. Sci. Eng. 10 (2), 182. doi:10.3390/jmse10020182
Ministry of Science and Higher Education of the Russian Federation (2022). Monthly digest within the year of science and technology in Russia – november 2021: Artificial intelligence. Available at: https://годнауки.рф/upload/iblock/336/5pxu3rd0i88mzxxpjc69soqbil7bd44v/11.2021.pdf (Accessed date: 09.08.2022).
Oteng, D., Zuo, J., and Sharifi, E. (2022). Environmental emissions influencing solar photovoltaic waste management in Australia: An optimised system network of waste collection facilities. J. Environ. Manag. 314, 115007. doi:10.1016/j.jenvman.2022.115007
Parmar, B., Vishwakarma, A., Padbhushan, R., Kumar, R., Kumari, R., Kaviraj, M., et al. (2022). Hedge and alder-based agroforestry systems: Potential interventions to carbon sequestration and better crop productivity in Indian sub-himalayas. Front. Environ. Sci. 10, 858948. doi:10.3389/fenvs.2022.858948
Parvathi Sangeetha, B., Kumar, N., Ambalgi, A. P., Thilagam, K., and Vijayakumar, P. (2022). IOT based smart irrigation management system for environmental sustainability in India. Sustain. Energy Technol. Assessments 52, 101973. doi:10.1016/j.seta.2022.101973
Popkova, E. G., Bogoviz, A. V., and Krivtsov, A. I. (2020). “Conclusions: Economic and legal management of modern economic systems' innovative development: A view into the future,” in The economic and legal foundations of managing innovative development in modern economic systems, 203–204. doi:10.1515/9783110643701-022
Popkova, E. G., De Bernardi, P., Tyurina, Y. G., and Sergi, B. S. (2022). A theory of digital technology advancement to address the grand challenges of sustainable development. Technol. Soc. 68, 101831. doi:10.1016/j.techsoc.2021.101831
Pullin, A. S., Cheng, S. H., Jackson, J. D. U., Envall, I., Fada, S. J., Twardek, W. M., et al. (2022). Standards of conduct and reporting in evidence syntheses that could inform environmental policy and management decisions. Environ. Evid. 11 (1), 16. doi:10.1186/s13750-022-00269-9
Queen, G. (2022). Total agri-food tech funding in 2020 to reach US$30.5 billion, new data shows. Available at: https://www.greenqueen.com.hk/total-agri-food-tech-funding-in-2020-to-reach-us30-5-billion-new-data-shows/ (Accessed date: 17.05.2022).
Ren, C., Zhang, X., Reis, S., and Gu, B. (2022). Socioeconomic barriers of nitrogen management for agricultural and environmental sustainability. Agric. Ecosyst. Environ. 333, 107950. doi:10.1016/j.agee.2022.107950
Softline (2022). Artificial Intelligence on guard of environment protection. Available at: https://slddigital.com/article/iskusstvennyj-intellekt-na-strazhe-ekologii/ (Accessed date: 09.08.2022).
Tang, Y. M., Chau, K. Y., Fatima, A., and Waqas, M. (2022). Industry 4.0 technology and circular economy practices: Business management strategies for environmental sustainability. Environ. Sci. Pollut. Res. 29, 49752–49769. doi:10.1007/s11356-022-19081-6
Tian, Y. (2022). The heterogeneous dynamic effect of financial development and environmental regulation on Chinese urban green technology management efficiency. Environ. Sci. Pollut. Res. 29 (21), 32032–32053. doi:10.1007/s11356-021-18320-6
World Bank (2022). Indicators: Agriculture & rural development. Available at: https://data.worldbank.org/indicator (Accessed date: 17.05.2022).
Keywords: environmental decision-making, environmental business management, technological modernization, automation, big data, AI
Citation: Vagin SG, Klimenko VA, Telegina ZA and Aleksashina TV (2022) Improving environmental decision-making in environmental business-management using big data and AI. Front. Environ. Sci. 10:951306. doi: 10.3389/fenvs.2022.951306
Received: 23 May 2022; Accepted: 15 August 2022;
Published: 06 September 2022.
Edited by:
Elena G. Popkova, Moscow State Institute of International Relations, RussiaReviewed by:
Ruchika Pharswan, Indian Institute of Technology Delhi, IndiaCopyright © 2022 Vagin, Klimenko, Telegina and Aleksashina. This is an open-access article distributed under the terms of the Creative Commons Attribution License (CC BY). The use, distribution or reproduction in other forums is permitted, provided the original author(s) and the copyright owner(s) are credited and that the original publication in this journal is cited, in accordance with accepted academic practice. No use, distribution or reproduction is permitted which does not comply with these terms.
*Correspondence: Sergei G. Vagin, dnNnNjNAaG90bWFpbC5jb20=
Disclaimer: All claims expressed in this article are solely those of the authors and do not necessarily represent those of their affiliated organizations, or those of the publisher, the editors and the reviewers. Any product that may be evaluated in this article or claim that may be made by its manufacturer is not guaranteed or endorsed by the publisher.
Research integrity at Frontiers
Learn more about the work of our research integrity team to safeguard the quality of each article we publish.